Algorithm Efficiency.md
Document Details
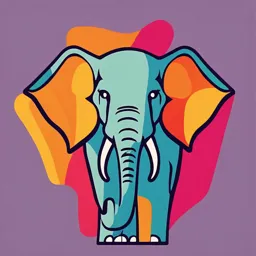
Uploaded by RestfulPersonification8326
Full Transcript
# What is an Algorithm? ## A Little Preface When students first learn to code, their focus is—and should be—on getting their code to run properly. Their code is measured using one simple metric: does the code work? But as you gain experience, you should begin to learn about additional layers and nu...
# What is an Algorithm? ## A Little Preface When students first learn to code, their focus is—and should be—on getting their code to run properly. Their code is measured using one simple metric: does the code work? But as you gain experience, you should begin to learn about additional layers and nuances regarding the quality of their code. Very often there can be two snippets of code that both accomplish the same task, but that one snippet is better than the other. There are numerous measures of code quality. One important measure is code maintainability. Maintainability of code involves aspects such as the readability, organization, and modularity of one’s code. However, there’s another aspect of high-quality code, and that is code efficiency. ## Which code runs faster than the other? ![[Code Example 1.png]] VS. ![[Code Example 2.png]] ## Algorithms - De-Mystified Although the word algorithm sounds like something complex, it really isn’t. An algorithm is simply a set of instructions for completing a specific task. For example, Even a process as simple as preparing a bowl of cereal is technically an algorithm, as it involves following a defined set of steps to achieve the task at hand. The cereal-preparation algorithm follows these four steps (for me, at least): 1.Grab a bowl. 2.Pour cereal into the bowl. 3.Pour milk into the bowl. 4.Dip a spoon into the bowl. By following these steps in this particular order, we can now enjoy our breakfast. When applied to computing, an algorithm refers to the set of instructions given to a computer to achieve a particular task. When we write any code, then, we’re creating algorithms for the computer to follow and execute. Algorithms can also be expressed using plain English to set out the details of the instructions we plan on providing to the computer. # Arrays ## The Array: Foundational Data Structure The array is one of the most basic data structures in computer science. I assume you have worked with arrays before, so you are aware that an array is a list of data elements. The array is versatile and can serve as a useful tool in many situations, but let’s look at one quick example. If you are looking at the source code for an application that allows users to create and use shopping lists for the grocery store, you might find code like this: ![[Array Example.png]] ![[Arrays continued Example.png]] ## Operations To understand the performance of any data structure—such as the array—we need to analyze the common ways our code might interact with that data structure. **Read**: Reading refers to looking something up at a particular spot within the data structure. With an array, this means looking up a value at a particular index. For example, looking up which grocery item is located at index 2 would be reading from the array. **Search**: Searching refers to looking for a particular value within a data structure. With an array, this means looking to see if a particular value exists within the array, and if so, at which index. For example, looking up the index of "dates" in our grocery list would be searching the array. **Insert**: Insertion refers to adding a new value to our data structure. With an array, this means adding a new value to an additional slot within the array. If we were to add "figs" to our shopping list, we’d be inserting a new value into the array. **Delete**: Deletion refers to removing a value from our data structure. With an array, this means removing one of the values from the array. For example, if we removed "bananas" from our grocery list, this value would be deleted from the array. ## Measuring Speed So, how do we measure the speed of an operation? When we measure how “fast” an operation takes, we do not refer to how fast the operation takes in terms of pure time, but instead in ==how many steps it takes==. We’ve seen this earlier in the context of printing the even numbers from 2 to 100. The second version of that function was faster because it took half as many steps as the first version did. ## Why measure code’s speed in terms of steps? We do this because we can never say definitively that any operation takes, say, five seconds. While a piece of code may take five seconds on a particular computer, that same piece of code may take longer on an older piece of hardware. For that matter, that same code might run much faster on the supercomputers of tomorrow. Measuring the speed of an operation in terms of time is undependable, since the time will always change depending on the hardware it is run on. However, we can measure the speed of an operation in terms of how many computational steps it takes. If Operation A takes 5 steps, and Operation B takes 500 steps, we can assume that Operation A will always be faster than Operation B on all pieces of hardware. Measuring the number of steps is, therefore, the key to analyzing the speed of an operation. ## Read (fastest) A computer can read from an array in just one step. This is because the computer can jump to any index in the array and peer inside. In our example of `["apples", "bananas", "cucumbers", "dates", "elderberries"]`, if we looked up index 2, the computer would jump right to index 2 and report that it contains the value "cucumbers". How is the computer able to look up an array’s index in just one step? Let’s see how. A computer’s memory can be viewed as a giant collection of cells. In the following diagram, you can see a grid of cells in which some are empty, and some contain bits of data: ![[Read Picture.png]] When a program declares an array, it allocates a contiguous set of empty cells for use in the program. So, if you were creating an array meant to hold five elements, your computer would find a group of five empty cells in a row and designate it to serve as your array. Now, every cell in a computer’s memory has a specific address. It’s sort of like a street address (for example, 123 Main St.), except that it’s represented with a number. Each cell’s memory address is one number greater than the previous cell’s address. ![[Read Example 2.png]] Let's get back to our declared array and visualize it with its indexes and memory addresses. When the computer reads a value at a particular index of an array, it can jump straight to that index because of the combination of the following facts about computers: 1.A computer can jump to any memory address in one step. For example, if you asked a computer to inspect whatever’s at memory address 1063, it can access that without having to perform any search process. As an analogy, if I ask you to raise your right pinky finger, you wouldn’t have to search all your fingers to find which one is your right pinky. You’d be able to identify it immediately. 2.Whenever a computer allocates an array, it also makes note at which memory address the array begins. So, if we asked the computer to find the first element of the array, it would be able to instantly jump to the appropriate memory address to find it. ![[Read Example 3.png]] Now, these facts explain how the computer can find the first value of an array in a single step. However, a computer can also find the value at any index by performing simple addition. If we asked the computer to find the value at index 3, the computer would simply take the memory address at index 0 and add 3. (Memory addresses are sequential, after all.) Reading from an array is, therefore, an efficient operation, since the computer can read any index by jumping to any memory address in one step. Naturally, an operation that takes just one step is the fastest type of operation. Besides being a foundational data structure, arrays are also a very powerful data structure because we can read from them with such speed. ==READ - 1 step process== ==O(1) == ## Search As I stated previously, searching an array means looking to see whether a particular value exists within an array and if so, at which index it’s located. In a sense, it’s the inverse of reading. Reading means providing the computer an index and asking it to return the value contained there. Searching, on the other hand, means providing the computer a value and asking it to return the index of that value’s location. Unlike Reading, Searching, is tedious, since the computer has no way to jump to a particular value. This is an important fact about computers: a computer has immediate access to all of its memory addresses, but it has no idea offhand what values are contained at each memory address. Let’s take our earlier array of fruits and veggies, for example. The computer can’t immediately see the actual contents of each cell. To search for a fruit within the array, the computer has no choice but to inspect each cell one at a time. The following diagrams demonstrate the process the computer would use to search for "dates" within our array. First, the computer checks index 0. Since the value at index 0 is "apples", and not the "dates" we’re looking for, the computer moves on to the next index. This continues until “dates” is found. ![[Search Example.png]] In this example, because the computer had to check four different cells until it found the value we were searching for, we’d say that this operation took a total of four steps. Now, what is the maximum number of steps a computer would need to perform to conduct a linear search on an array? If the value we’re seeking happens to be in the final cell in the array (like "elderberries"), then the computer would end up searching through every cell of the array until it finally finds the value it’s looking for. Also, if the value we’re looking for doesn’t occur in the array at all, the computer likewise would have to search every cell so that it can be sure the value doesn’t exist within the array. So, it turns out that for an array of five cells, the maximum number of steps linear search would take is five. For an array of 500 cells, the maximum number of steps linear search would take is 500. Another way of saying this is that for N cells in an array, linear search would take a maximum of N steps. In this context, N is just a variable that can be replaced by any number. ==Always worst case scenario- if array size is N, the number of steps is N == ==O(N)== ## Insert The efficiency of inserting a new piece of data into an array depends on where within the array you’re inserting it. Let’s say we want to add "figs" to the end of our shopping list. Such an insertion takes just one step. This is true due to another fact about computers: when allocating an array, the computer always keeps track of the array’s size. When we couple this with the fact that the computer also knows at which memory address the array begins, computing the memory address of the last item of the array is a cinch: if the array begins at memory address 1010 and is of size 5, that means its final memory address is 1014. So, to insert an item beyond that would mean adding it to the next memory address, which is 1015. Once the computer calculates which memory address to insert the new value into, it can do so in one step. ![[Insert Example.png]] But there’s one hitch. Because the computer initially allocated only five cells in memory for the array, and now we’re adding a sixth element, the computer may have to allocate additional cells toward this array. In many programming languages, this is done under the hood automatically, but each language handles this differently, so I won’t get into the details of it. We’ve dealt with insertions at the end of an array, but inserting a new piece of data at the beginning or in the middle of an array is a different story. In these cases, we need to shift pieces of data to make room for what we’re inserting, leading to additional steps. To do this, we need to move "cucumbers", "dates", and "elderberries" to the right to make room for "figs". This takes multiple steps, since we need to first move "elderberries" one cell to the right to make room to move "dates". We then need to move "dates" to make room for "cucumbers". ![[Insert Example 2.png]] Notice that in the preceding example, insertion took four steps. Three of the steps involved shifting data to the right, while one step involved the actual insertion of the new value. We can say that insertion in a worst-case scenario can take N + 1 steps for an array containing N elements. This is because we need to shift all N elements over, and then finally execute the actual insertion step. ==Inserting at the end of an array --> 1 step== ==Inserting at the beginning of an array --> shift everything one step to the right --> N+1 steps== ## Delete Deletion from an array is the process of eliminating the value at a particular index. Let’s return to our original example array and delete the value at index 2. In our example, this value is "cucumbers". While the actual deletion of "cucumbers" technically took just one step, we now have a problem: we have an empty cell sitting smack in the middle of our array. An array is not effective when there are gaps in the middle of it, so to resolve this issue, we need to shift "dates" and "elderberries" to the left. This means our deletion process requires additional steps. ![[Delete Example.png]] It turns out that for this deletion, the entire operation took three steps. The first step involved the actual deletion, and the other two steps involved data shifts to close the gap. Like insertion, the worst-case scenario of deleting an element is deleting the very first element of the array. This is because index 0 would become empty, and we’d have to shift all the remaining elements to the left to fill the gap. For an array of five elements, we’d spend one step deleting the first element, and four steps shifting the four remaining elements. For an array of 500 elements, we’d spend one step deleting the first element, and 499 steps shifting the remaining data. We can say then, that for an array containing N elements, the maximum number of steps that deletion would take is N steps. ==Go to last memory block and remove, 1 step process== ==Can't have unoccupied blocks, therefore first number removed is N step== # O YES! BIG O NOTION ## What is Big O notation? So, in the previous slides we discussed that the primary factor in determining an algorithm’s efficiency is the number of steps it takes. However, we can’t simply label one algorithm a “22-step algorithm” and another a “400-step algorithm.” This is because the number of steps an algorithm takes cannot be pinned down to a single number. Let’s take linear search, for example. The number of steps linear search takes varies, as it takes as many steps as there are elements in the array. If the array contains 22 elements, linear search takes 22 steps. If the array contains 400 elements, however, linear search takes 400 steps. The more effective way, then, to quantify the efficiency of linear search is to say that linear search takes N steps for N elements in the array. That is, if an array has N elements, linear search takes N steps. Now, this is a wordy way of expressing this concept. To help ease communication regarding time complexity, computer scientists have borrowed a concept from the world of mathematics to describe a concise and consistent language around the efficiency of data structures and algorithms. Known as Big O Notation, this formalized expression of these concepts allows us to easily categorize the efficiency of a given algorithm and convey it to others. While Big O Notation comes from the math world, we are going to leave out all the mathematical jargon and explain it as it relates to computer science. Additionally, we are going to begin by explaining Big O Notation in simple terms and then continue to refine it over the next few weeks. ## Big O: How Many Steps Relative to N Elements? Big O achieves consistency by focusing on the number of steps an algorithm takes, but in a specific way. Let’s start off by applying Big O to the algorithm of linear search. In a worst-case scenario, linear search will take as many steps as there are elements in the array. As we’ve previously phrased it: for N elements in the array, linear search can take up to N steps. The appropriate way to express this in Big O Notation is O(N). Here’s what the notation means. It expresses the answer to what we’ll call the “key question.” The key question is: if there are N data elements, how many steps will the algorithm take? O(N) says that the answer to the key question is that the algorithm will take N steps. ==O(N) is also known as having linear time==. ## Big O: Read an Element from an Array Reading from an array takes just one step, no matter how large the array is. To figure out how to express this in Big O terms, we are going to again ask the key question: if there are N data elements, how many steps will reading from an array take? The answer is that reading takes just one step. So, we express this as O(1). This means, That, no matter how many elements an array has, reading from the array always takes one step. And this is why O(1) is considered the “fastest” kind of algorithm. Even as the data increases, an O(1) algorithm doesn’t take any additional steps. The algorithm always takes a constant number of steps no matter what N is. In fact, an O(1) algorithm can also be referred to as having constant time. ## The Soul of Big O Let’s say we have an algorithm that always takes three steps no matter how much data there is. That is, for N elements, the algorithm always takes three steps. How would you express that in terms of Big O? Based on everything you’ve learned up to this point, you’d probably say that it’s O(3). However, it’s actually O(1). And that’s because of the next layer of understanding Big O, which we will reveal now. The soul of Big O is what Big O is truly concerned about: how will an algorithm’s performance change as the data increases? This is the soul of Big O. Big O doesn’t want to simply tell you how many steps an algorithm takes. It wants to tell you the story of how the number of steps increase as the data changes ## Algorithm - O(N) An algorithm that is O(N), is an algorithm whose performance is affected as we increase the data. More specifically, it’s the kind of algorithm whose steps increase in direct proportion to the data as the data increases. This is the story O(N) tells. It tells you about the proportional relationship between the data and the algorithm’s efficiency. It describes exactly how the number of steps increase as the data increases. To understand better, Look at how these two types of algorithms are plotted on a graph: Notice that O(N) makes a perfect diagonal line. This is because for every additional piece of data, the algorithm takes one additional step. Accordingly, the more data, the more steps the algorithm will take. Contrast this with O(1), which is a perfect horizontal line. No matter how much data there is, the number of steps remain constant. ![[Algorithm Graph.png]] ==N step is O(N) and 1 step is O(1)== Let pause here and work on a group activity now. As a group of 3 people, analyze this graph and do a small write up on what you can deduce from this graph. ![[Algorithm Graph 2.png]] ## Same Algorithms, Different Scenarios Linear search isn’t always O(N). It’s true that if the item we’re looking for is in the final cell of the array, it will take N steps to find it. But when the item we’re searching for is found in the first cell of the array, linear search will find the item in just one step. So, this case of linear search would be described as O(1). If we were to describe the efficiency of linear search in its totality, we’d say that linear search is O(1) in a best-case scenario, and O(N) in a worst-case scenario. While Big O effectively describes both the best- and worst-case scenarios of a given algorithm, Big O Notation generally refers to the worst-case scenario unless specified otherwise. This is why most references will describe linear search as being O(N) even though it can be O(1) in a best-case scenario. ## Algorithm - O(log N) - Binary Search Before trying to understand O(log N), let us try to understand the Binary Search Algorithm and logarithm. First, What is Binary Search Algorithm? You’ve probably played this guessing game as a child: I’m thinking of a number between 1 and 100. Keep guessing which number I’m thinking of, and I’ll let you know whether you need to guess higher or lower. You may know intuitively how to play this game. You wouldn’t begin by guessing number 1. Instead, you’d probably start with 50, which is smack in the middle. Why? Because by selecting 50, no matter whether I tell you to guess higher or lower, you’ve automatically eliminated half the possible numbers! If you guess 50 and I tell you to guess higher, you’d then pick 75, to eliminate half of the remaining numbers. If after guessing 75, I told you to guess lower, you’d pick 62 or 63. You’d keep on choosing the halfway mark in order to keep eliminating half of the remaining numbers. ![[Binary Search Picture.png]] ## Binary Search ![[Binary Search 1.png]] ![[Binary Search 2.png]] ![[Binary Search 3.png]] ## Logarithms Let’s examine why algorithms such as binary search are described as O(log N). What is a log, anyway? Log is shorthand for logarithm. The first thing to note is that logarithms have nothing to do with algorithms, even though the two words look and sound so similar. Logarithms are the inverse of exponents. Here’s a quick refresher on what exponents are: 23 is the equivalent of: 2 * 2 * 2 which just happens to be 8. Now, log2 8 is the converse. It means: how many times do you have to multiply 2 by itself to get a result of 8? Because you must multiply 2 by itself 3 times to get 8, log2 8 = 3. ## O(log N) In computer science, whenever we say O(log N), it’s actually shorthand for saying O(log2 N). We just omit that small 2 for convenience. O(log N) means that for N data elements, the algorithm would take log2 N steps. If there are 8 elements, the algorithm would take three steps, since log2 8 = 3. This is exactly what happens with binary search. As we search for a particular item, we keep dividing the array’s cells in half until we narrow it down to the correct number. Said simply: ==O(log N) means the algorithm takes as many steps as it takes to keep halving the data elements until we remain with 1.== ## O(log N) vs O(N) This table demonstrates a striking difference between the efficiencies of O(N) and O(log N): While the O(N) algorithm takes as many steps as there are data elements, the O(log N) algorithm takes just one additional step each time the data is doubled. ![[O(log N) vs O(N) Table.png]] ## More Big O Notations We discussed the basic building blocks for other Big O notations such as O (N log N) – Linearithmic Time O(N^2) – Quadratic Time O(2^N) – Exponential Time O(N!) – Factorial Time Please spend some time understanding these time complexity measurements.