Artificial Intelligence and the Future of Teaching and Learning PDF
Document Details
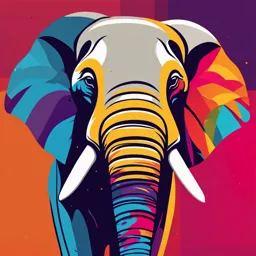
Uploaded by ReadyMalachite3528
2023
Miguel A. Cardona, Ed.D, Roberto J. Rodríguez, Kristina Ishmael
Tags
Related
- Ethical Guidelines on the Use of Artificial intelligence (AI) and Data in Teaching and Learning for Educators PDF
- AI and the Future of Teaching and Learning PDF
- Using Artificial Intelligence in Teaching and Learning PDF
- Introduccion a la Inteligencia Artificial en Educacion PDF
- Transforming Teaching and Learning with AI Tools in Education PDF
- Artificial Intelligence in Education Role (PDF)
Summary
This report from the U.S. Department of Education explores the potential of artificial intelligence in teaching and learning. It examines how AI can enhance various aspects of education, including learning, teaching, formative assessment, and research. It also emphasizes the importance of ethical considerations and equitable implementation.
Full Transcript
Artificial Intelligence and the Future of Teaching and Learning Insights and Recommendations May 2023 1 Artificial Intelligence and the Future of Teaching and Learning Miguel A. Cardona, Ed.D. Secretary, U.S. Department of Education Roberto J. Rodríguez Assistant Sec...
Artificial Intelligence and the Future of Teaching and Learning Insights and Recommendations May 2023 1 Artificial Intelligence and the Future of Teaching and Learning Miguel A. Cardona, Ed.D. Secretary, U.S. Department of Education Roberto J. Rodríguez Assistant Secretary, Office of Planning, Evaluation, and Policy Development Kristina Ishmael Deputy Director, Office of Educational Technology May 2023 Examples Are Not Endorsements This document contains examples and resource materials that are provided for the user’s convenience. The inclusion of any material is not intended to reflect its importance nor is it intended to endorse any views expressed or products or services offered. These materials may contain the views and recommendations of various subject matter experts as well as hypertext links, contact addresses, and websites to information created and maintained by other public and private organizations. The opinions expressed in any of these materials do not necessarily reflect the positions or policies of the U.S. Department of Education. The U.S. Department of Education does not control or guarantee the accuracy, relevance, timeliness, or completeness of any information from other sources that are included in these materials. Other than statutory and regulatory requirements included in the document, the contents of this guidance do not have the force and effect of law and are not meant to bind the public. Contracts and Procurement This document is not intended to provide legal advice or approval of any potential federal contractor’s business decision or strategy in relation to any current or future federal procurement and/or contract. Further, this document is not an invitation for bid, request for proposal, or other solicitation. Licensing and Availability This report is in the public domain and available on the U.S. Department of Education’s (Department’s) website at https://tech.ed.gov. Requests for alternate format documents such as Braille or large print should be submitted to the Alternate Format Center by calling 1-202-260-0852 or by contacting the 504 coordinator via email at [email protected]. Notice to Limited English Proficient Persons If you have difficulty understanding English, you may request language assistance services for Department information that is available to the public. These language assistance services are available free of charge. If you need more information about interpretation or translation services, please call 1-800-USA-LEARN (1-800-872-5327) (TTY: 1-800-437-0833); email us at [email protected]; or write to U.S. Department of Education, Information Resource Center, LBJ Education Building, 400 Maryland Ave. SW, Washington, DC 20202. How to Cite While permission to reprint this publication is not necessary, the suggested citation is as follows: U.S. Department of Education, Office of Educational Technology, Artificial Intelligence and Future of Teaching and Learning: Insights and Recommendations, Washington, DC, 2023. This report is available at https://tech.ed.gov Table of Contents Introduction.......................................................................................................................... 1 Rising Interest in AI in Education......................................................................................................................................................... 1 Three Reasons to Address AI in Education Now...................................................................................................................2 Toward Policies for AI in Education..................................................................................................................................................3 Building Ethical, Equitable Policies Together........................................................ 6 Guiding Questions........................................................................................................................................................................................... 6 Foundation 1: Center People (Parents, Educators, and Students)......................................................................... 6 Foundation 2: Advance Equity...............................................................................................................................................................7 Foundation 3: Ensure Safety, Ethics, and Effectiveness................................................................................................ 8 Foundation 4: Promote Transparency........................................................................................................................................... 9 Overview of Document............................................................................................................................................................................ 10 What is AI?.......................................................................................................................... 11 Perspective: Human-Like Reasoning........................................................................................................................................... 12 Perspective: An Algorithm that Pursues a Goal................................................................................................................... 12 Perspective: Intelligence Augmentation................................................................................................................................... 14 Definition of “Model”.................................................................................................................................................................................... 14 Insight: AI Systems Enable New Forms of Interaction................................................................................................... 15 Key Recommendation: Human in the Loop AI..................................................................................................................... 16 Learning.............................................................................................................................. 18 Insight: AI Enables Adaptivity in Learning................................................................................................................................. 18 Intelligent Tutoring Systems: An Example of AI Models............................................................................................. 19 Important Directions for Expanding AI-Based Adaptivity.......................................................................................... 20 A Duality: Learning With and About AI........................................................................................................................................ 22 A Challenge: Systems Thinking About AI in Education................................................................................................. 22 Open Questions About AI for Learning....................................................................................................................................... 23 Key Recommendation: Seek AI Models Aligned to a Vision for Learning....................................................24 Teaching............................................................................................................................. 25 Always Center Educators in Instructional Loops...............................................................................................................25 Insight: Using AI to Improve Teaching Jobs.......................................................................................................................... 26 Preparing and Supporting Teachers in Planning and Reflecting........................................................................ 29 Designing, Selecting, and Evaluating AI Tools.................................................................................................................... 30 Challenge: Balancing Human and Computer Decision-Making.......................................................................... 30 Challenge: Making Teaching Jobs Easier While Avoiding Surveillance........................................................ 31 Challenge: Responding to Students’ Strengths While Protecting Their Privacy.................................... 32 Questions Worth Asking About AI for Teaching.................................................................................................................34 Key Recommendation: Inspectable, Explainable, Overridable AI.......................................................................34 Formative Assessment................................................................................................. 37 Building on Best Practices.....................................................................................................................................................................37 Implications for Teaching and Learning................................................................................................................................... 38 Insight: AI Can Enhance Feedback Loops............................................................................................................................... 39 An Example: Automated Essay Scoring.................................................................................................................................... 40 Key Opportunities for AI in Formative Assessment......................................................................................................... 41 Key Recommendation: Harness Assessment Expertise to Reduce Bias......................................................42 Related Questions.........................................................................................................................................................................................43 Research and Development....................................................................................... 44 Insight: Research Can Strengthen the Role of Context in AI....................................................................................44 Attention to the Long Tail of Learner Variability................................................................................................................ 46 Partnership in Design-Based Research......................................................................................................................................47 Re-thinking Teacher Professional Development.............................................................................................................. 48 Connecting with Public Policy........................................................................................................................................................... 49 Key Recommendation: Focus R&D on Addressing Context.................................................................................... 50 Ongoing Questions for Researchers............................................................................................................................................ 50 Desired National R&D Objectives.................................................................................................................................................... 51 Recommendations......................................................................................................... 52 Insight: Aligning AI to Policy Objectives.....................................................................................................................................52 Calling Education Leaders to Action............................................................................................................................................53 Recommendation #1: Emphasize Humans in the Loop...............................................................................................53 Recommendation #2: Align AI Models to a Shared Vision for Education.....................................................54 Recommendation #3: Design Using Modern Learning Principles..................................................................... 56 Recommendation #4: Prioritize Strengthening Trust.....................................................................................................57 Recommendation #5: Inform and Involve Educators....................................................................................................57 Recommendation #6: Focus R&D on Addressing Context and Enhancing Trust and Safety.... 59 Recommendation #7: Develop Education-Specific Guidelines and Guardrails..................................... 60 Next Steps.......................................................................................................................................................................................................... 60 Common Acronyms and Abbreviations................................................................. 62 Acknowledgements....................................................................................................... 63 References......................................................................................................................... 64 Introduction The U.S. Department of Education (Department) is committed to supporting the use of technology to improve teaching and learning and to support innovation throughout educational systems. This report addresses the clear need for sharing knowledge and developing policies for “Artificial Intelligence,” a rapidly advancing class of foundational capabilities which are increasingly embedded in all types of educational technology systems and are also available to the public. We will consider “educational technology” (edtech) to include both (a) technologies specifically designed for educational use, as well as (b) general technologies that are widely used in educational settings. Recommendations in this report seek to engage teachers, educational leaders, policy makers, researchers, and educational technology innovators and providers as they work together on pressing policy issues that arise as Artificial Intelligence (AI) is used in education. AI can be defined as “automation based on associations.” When computers automate reasoning based on associations in data (or associations deduced from expert knowledge), two shifts fundamental to AI occur and shift computing beyond conventional edtech: (1) from capturing data to detecting patterns in data and (2) from providing access to instructional resources to automating decisions about instruction and other educational processes. Detecting patterns and automating decisions are leaps in the level of responsibilities that can be delegated to a computer system. The process of developing an AI system may lead to bias in how patterns are detected and unfairness in how decisions are automated. Thus, educational systems must govern their use of AI systems. This report describes opportunities for using AI to improve education, recognizes challenges that will arise, and develops recommendations to guide further policy development. Rising Interest in AI in Education Today, many priorities for improvements to teaching and learning are unmet. Educators seek technology-enhanced approaches addressing these priorities that would be safe, effective, and scalable. Naturally, educators wonder if the rapid advances in technology in everyday lives could help. Like all of us, educators use AI-powered services in their everyday lives, such as voice assistants in their homes; tools that can correct grammar, complete sentences, and write essays; and automated trip planning on their phones. Many educators are actively exploring AI tools as they are newly released to the public 1. Educators see opportunities to use AI-powered capabilities like speech recognition to increase the support available to students with disabilities, multilingual learners, and others who could benefit from greater adaptivity and personalization in digital tools for learning. They are exploring how AI can enable writing or improving lessons, as well as their process for finding, choosing, and adapting material for use in their lessons. Educators are also aware of new risks. Useful, powerful functionality can also be accompanied with new data privacy and security risks. Educators recognize that AI can automatically produce output that is inappropriate or wrong. They are wary that the associations or automations created by AI may amplify unwanted biases. They have noted new ways in which students may 1Walton Family Foundation (March 1, 2023). Teachers and students embrace ChatGPT for education. https://www.waltonfamilyfoundation.org/learning/teachers-and-students-embrace-chatgpt-for-education 1 represent others’ work as their own. They are well-aware of “teachable moments” and pedagogical strategies that a human teacher can address but are undetected or misunderstood by AI models. They worry whether recommendations suggested by an algorithm would be fair. Educators’ concerns are manifold. Everyone in education has a responsibility to harness the good to serve educational priorities while also protecting against the dangers that may arise as a result of AI being integrated in edtech. To develop guidance for edtech, the Department works closely with educational constituents. These constituents include educational leaders—teachers, faculty, support staff, and other educators—researchers; policymakers; advocates and funders; technology developers; community members and organizations; and, above all, learners and their families/caregivers. Recently, through its activities with constituents, the Department noticed a sharp rise in interest and concern about AI. For example, a 2021 field scan found that developers of all kinds of technology systems—for student information, classroom instruction, school logistics, parent- teacher communication, and more—expect to add AI capabilities to their systems. Through a series of four listening sessions conducted in June and August 2022 and attended by more than 700 attendees, it became clear that constituents believe that action is required now in order to get ahead of the expected increase of AI in education technology—and they want to roll up their sleeves and start working together. In late 2022 and early 2023, the public became aware of new generative AI chatbots and began to explore how AI could be used to write essays, create lesson plans, produce images, create personalized assignments for students, and more. From public expression in social media, at conferences, and in news media, the Department learned more about risks and benefits of AI-enabled chatbots. And yet this report will not focus on a specific AI tool, service, or announcement, because AI-enabled systems evolve rapidly. Finally, the Department engaged the educational policy expertise available internally and in its relationships with AI policy experts to shape the findings and recommendations in this report. Three Reasons to Address AI in Education Now “I strongly believe in the need for stakeholders to understand the cyclical effects of AI and education. By understanding how different activities accrue, we have the ability to support virtuous cycles. Otherwise, we will likely allow vicious cycles to perpetuate.” —Lydia Liu During the listening sessions, constituents articulated three reasons to address AI now: First, AI may enable achieving educational priorities in better ways, at scale, and with lower costs. Addressing varied unfinished learning of students due to the pandemic is a policy priority, and AI may improve the adaptivity of learning resources to students’ strengths and needs. Improving teaching jobs is a priority, and via automated assistants or other tools, AI may provide teachers greater support. AI may also enable teachers to extend the support they offer to individual students when they run out of time. Developing resources that are responsive to the knowledge and experiences students bring to their learning—their community and cultural assets—is a priority, and AI may enable greater customizability of curricular resources to meet local needs. 2 As seen in voice assistants, mapping tools, shopping recommendations, essay-writing capabilities, and other familiar applications, AI may enhance educational services. Second, urgency and importance arise through awareness of system-level risks and anxiety about potential future risks. For example, students may become subject to greater surveillance. Some teachers worry that they may be replaced—to the contrary, the Department firmly rejects the idea that AI could replace teachers. Examples of discrimination from algorithmic bias are on the public’s mind, such as a voice recognition system that doesn’t work as well with regional dialects, or an exam monitoring system that may unfairly identify some groups of students for disciplinary action. Some uses of AI may be infrastructural and invisible, which creates concerns about transparency and trust. AI often arrives in new applications with the aura of magic, but educators and procurement policies require that edtech show efficacy. AI may provide information that appears authentic, but actually is inaccurate or lacking a basis in reality. Of the highest importance, AI brings new risks in addition to the well-known data privacy and data security risks, such as the risk of scaling pattern detectors and automations that result in “algorithmic discrimination” (e.g., systematic unfairness in the learning opportunities or resources recommended to some populations of students). Third, urgency arises because of the scale of possible unintended or unexpected consequences. When AI enables instructional decisions to be automated at scale, educators may discover unwanted consequences. In a simple example, if AI adapts by speeding curricular pace for some students and by slowing the pace for other students (based on incomplete data, poor theories, or biased assumptions about learning), achievement gaps could widen. In some cases, the quality of available data may produce unexpected results. For example, an AI-enabled teacher hiring system might be assumed to be more objective than human-based résumé scoring. Yet, if the AI system relies on poor quality historical data, it might de-prioritize candidates who could bring both diversity and talent to a school’s teaching workforce. In summary, it is imperative to address AI in education now to realize key opportunities, prevent and mitigate emergent risks, and tackle unintended consequences. Toward Policies for AI in Education The 2023 AI Index Report from the Stanford Institute for Human-Centered AI has documented notable acceleration of investment in AI as well as an increase of research on ethics, including issues of fairness and transparency. 2 Of course, research on topics like ethics is increasing because problems are observed. Ethical problems will occur in education, too. 3 The report found a striking interest in 25 countries in the number of legislative proposals that specifically include AI. In the United States, multiple executive orders are focused on ensuring AI is trustworthy and equitable, and the White House Office of Science and Technology Policy has introduced a 2Maslej, N., Fattorini, L., Brynjolfsson E., Etchemendy, J., Ligett, K., Lyons, T., Manyika, J., Ngo, H., Niebles, J.C., Parli, V., Shoham, Y., Wald, R., Clark, J. and Perrault, R., (2023). The AI index 2023 annual report. Stanford University: AI Index Steering Committee, Institute for Human-Centered AI. 3Holmes, W. & Porayska-Pomsta, K. (Eds.) (2022). The ethics of artificial intelligence in education. Routledge. ISBN 978- 0367349721 3 Blueprint for an AI Bill of Rights (Blueprint)4 that provides principles and practices that help achieve this goal. These initiatives, along with other AI-related policy activities occurring in both the executive and legislative branches, will guide the use of AI throughout all sectors of society. In Europe, the European Commission recently released Ethical guidelines on the use of artificial intelligence (AI) and data in teaching and learning for educators.5 AI is moving fast and heralding societal changes that require a national policy response. In addition to broad policies for all sectors of society, education-specific policies are needed to address new opportunities and challenges within existing frameworks that take into consideration federal student privacy laws (such as the Family Educational Rights and Privacy Act, or FERPA), as well as similar state related laws. AI also makes recommendations and takes actions automatically in support of student learning, and thus educators will need to consider how such recommendations and actions can comply with laws such as the Individuals with Disabilities Education Act (IDEA). We discuss specific policies in the concluding section. Figure 1: Research about AI is growing rapidly. Other indicators, such as dollars invested and number of people employed, show similar trends. AI is advancing exponentially (see Figure 1), with powerful new AI features for generating images and text becoming available to the public, and leading to changes in how people create text and 4 White House Office of Science and Technology Policy (October 2022), Blueprint for an AI bill of rights: Making automated systems work for the American people. The White House Office of Science and Technology Policy. https://www.whitehouse.gov/ostp/ai-bill-of-rights/ 5European Commission, Directorate-General for Education, Youth, Sport and Culture. (2022). Ethical guidelines on the use of artificial intelligence (AI) and data in teaching and learning for educators, Publications Office of the European Union. https://data.europa.eu/doi/10.2766/153756 4 images6. The advances in AI are not only happening in research labs but also are making news in mainstream media and in educational-specific publications. Researchers have articulated a range of concepts and frameworks for ethical AI 7, as well as for related concepts such as equitable, responsible, and human-centered AI. Listening session participants called for building on these concepts and frameworks but also recognized the need to do more; participants noted a pressing need for guardrails and guidelines that make educational use of AI advances safe, especially given this accelerating pace of incorporation of AI into mainstream technologies. As policy development takes time, policy makers and educational constituents together need to start now to specify the requirements, disclosures, regulations, and other structures that can shape a positive and safe future for all constituents—especially students and teachers. Policies are urgently needed to implement the following: 1. leverage automation to advance learning outcomes while protecting human decision making and judgment; 2. interrogate the underlying data quality in AI models to ensure fair and unbiased pattern recognition and decision making in educational applications, based on accurate information appropriate to the pedagogical situation; 3. enable examination of how particular AI technologies, as part of larger edtech or educational systems, may increase or undermine equity for students; and 4. take steps to safeguard and advance equity, including providing for human checks and balances and limiting any AI systems and tools that undermine equity. 6Sharples, M. & Pérez y Pérez, R. (2022). Story machines: How computers have become creative writers. Routledge. ISBN 9780367751951 7Akgun, S., Greenhow, C. (2022). Artificial intelligence in education: Addressing ethical challenges in K-12 settings. AI Ethics, 2, 431–440. https://doi.org/10.1007/s43681-021-00096-7 5 Building Ethical, Equitable Policies Together In this report, we aim to build on the listening sessions the Department hosted to engage and inform all constituents involved in making educational decisions so they can prepare for and make better decisions about the role of AI in teaching and learning. AI is a complex and broad topic, and we are not able to cover everything nor resolve issues that still require more constituent engagement. This report is intended to be a starting point. The opportunities and issues of AI in education are equally important in K-12, higher education, and workforce learning. Due to scope limitations, the examples in this report will focus on K-12 education. The implications are similar at all levels of education, and the Department intends further activities in 2023 to engage constituents beyond K-12 schools. Guiding Questions Understanding that AI increases automation and allows machines to do some tasks that only people did in the past leads us to a pair of bold, overarching questions: 1. What is our collective vision of a desirable and achievable educational system that leverages automation to advance learning while protecting and centering human agency? 2. How and on what timeline will we be ready with necessary guidelines and guardrails, as well as convincing evidence of positive impacts, so that constituents can ethically and equitably implement this vision widely? In the Learning, Teaching, and Assessment sections of this report, we elaborate on elements of an educational vision grounded in what today’s learners, teachers, and educational systems need, and we describe key insights and next steps required. Below, we articulate four key foundations for framing these themes. These foundations arise from what we know about the effective use of educational technology to improve opportunity, equity, and outcomes for students and also relate to the new Blueprint. Foundation 1: Center People (Parents, Educators, and Students) Education-focused AI policies at the federal, state, and district levels will be needed to guide and empower local and individual decisions about which technologies to adopt and use in schools and classrooms. Consider what is happening in everyday lives. Many of us use AI-enabled products because they are often better and more convenient. For example, few people want to use paper maps anymore; people find that technology helps us plan the best route to a destination more efficiently and conveniently. And yet, people often do not realize how much privacy they are giving up when they accept AI-enabled systems into their lives. AI will bring privacy and other risks that are hard to address only via individual decision making; additional protections will be needed. 6 There should be clear limits on the ability to collect, use, transfer, and maintain our personal data, including limits on targeted advertising. These limits should put the burden on platforms to minimize how much information they collect, rather than burdening Americans with reading fine print.8 As protections are developed, we recommend that policies center people, not machines. To this end, a first recommendation in this document (in the next section) is an emphasis on AI with humans in the loop. Teachers, learners, and others need to retain their agency to decide what patterns mean and to choose courses of action. The idea of humans in the loop builds on the concept of “Human Alternatives, Consideration, and Fallback” in the Blueprint and ethical concepts used more broadly in evaluating AI, such as preserving human dignity. A top policy priority must be establishing human in the loop as a requirement in educational applications, despite contrary pressures to use AI as an alternative to human decision making. Policies should not hinder innovation and improvement, nor should they be burdensome to implement. Society needs an education-focused AI policy that protects civil rights and promotes democratic values in the building, deployment, and governance of automated systems to be used across the many decentralized levels of the American educational system. Foundation 2: Advance Equity “AI brings educational technology to an inflection point. We can either increase disparities or shrink them, depending on what we do now.” —Dr. Russell Shilling A recent Executive Order9 issued by President Biden sought to strengthen the connection among racial equity, education and AI, stating that “members of underserved communities—many of whom have endured generations of discrimination and disinvestment—still confront significant barriers to realizing the full promise of our great Nation, and the Federal Government has a responsibility to remove these barriers” and that the Federal Government shall both “pursue educational equity so that our Nation’s schools put every student on a path to success” and also “root out bias in the design and use of new technologies, such as artificial intelligence.” A specific vision of equity, such as described in the Department’s recent report, Advancing Digital Equity for All10 is essential to policy discussion about AI in education. This report defines digital equity as 8The White House (September 8, 2022). Readout of White House listening session on tech platform accountability. https://www.whitehouse.gov/briefing-room/statements-releases/2022/09/08/readout-of-white-house-listening-session- on-tech-platform-accountability/ 9The White House (February 17, 2023). Executive order on further advancing racial equity and support for underserved communities through the federal government. https://www.whitehouse.gov/briefing-room/presidential- actions/2023/02/16/executive-order-on-further-advancing-racial-equity 10U.S. Department of Education, Office of Educational Technology (2022). Advancing digital equity for all: Community- based recommendations for developing effective digital equity plans to close the digital divide and enable technology- empowered learning. US Department of Education. 7 “the condition in which individuals and communities have the information technology capacity that is needed for full participation in the society and economy of the United States.” Issues related to racial equity and unfair bias were at the heart of every listening session we held. In particular, we heard a conversation that was increasingly attuned to issues of data quality and the consequences of using poor or inappropriate data in AI systems for education. Datasets are used to develop AI, and when they are non-representative or contain undesired associations or patterns, resulting AI models may act unfairly in how they detect patterns or automate decisions. Systematic, unwanted unfairness in how a computer detects patterns or automates decisions is called “algorithmic bias.” Algorithmic bias could diminish equity at scale with unintended discrimination. As this document discussed in the Formative Assessment section, this is not a new conversation. For decades, constituents have rightly probed whether assessments are unbiased and fair. Just as with assessments, whether an AI model exhibits algorithmic bias or is judged to be fair and trustworthy is critical as local school leaders make adoption decisions about using AI to achieve their equity goals. We highlight the concept of “algorithmic discrimination” in the Blueprint. Bias is intrinsic to how AI algorithms are developed using historical data, and it can be difficult to anticipate all impacts of biased data and algorithms during system design. The Department holds that biases in AI algorithms must be addressed when they introduce or sustain unjust discriminatory practices in education. For example, in postsecondary education, algorithms that make enrollment decisions, identify students for early intervention, or flag possible student cheating on exams must be interrogated for evidence of unfair discriminatory bias—and not only when systems are designed, but also later, as systems become widely used. Foundation 3: Ensure Safety, Ethics, and Effectiveness A central safety argument in the Department’s policies is the need for data privacy and security in the systems used by teachers, students, and others in educational institutions. The development and deployment of AI requires access to detailed data. This data goes beyond conventional student records (roster and gradebook information) to detailed information about what students do as they learn with technology and what teachers do as they use technology to teach. AI’s dependence on data requires renewed and strengthened attention to data privacy, security, and governance (as also indicated in the Blueprint). As AI models are not generally developed in consideration of educational usage or student privacy, the educational application of these models may not be aligned with the educational institution’s efforts to comply with federal student privacy laws, such as FERPA, or state privacy laws. 8 Figure 2: The Elementary and Secondary Education Act defines four levels of evidence. Further, educational leaders are committed to basing their decisions about the adoption of educational technology on evidence of effectiveness—a central foundation of the Department’s policy. For example, the requirement to base decisions on evidence also arises in the Elementary and Secondary Education Act (ESEA), as amended, which introduced four tiers of evidence (see Figure 2). Our nation’s research agencies, including the Institute of Education Sciences, are essential to producing the needed evidence. The Blueprint calls for evidence of effectiveness, but the education sector is ahead of that game: we need to insist that AI-enhanced edtech rises to meet ESEA standards as well. Foundation 4: Promote Transparency The central role of complex AI models in a technology’s detection of patterns and implementation of automation is an important way in which AI-enabled applications, products, and services will be different from conventional edtech. The Blueprint introduces the need for transparency about AI models in terms of disclosure (“notice”) and explanation. In education, decision makers will need more than notice—they will need to understand how AI models work in a range of general educational use cases, so they can better anticipate limitations, problems, and risks. AI models in edtech will be approximations of reality and, thus, constituents can always ask these questions: How precise are the AI models? Do they accurately capture what is most important? How well do the recommendations made by an AI model fit educational goals? What are the broader implications of using AI models at scale in educational processes? Building on what was heard from constituents, the sections of this report develop the theme of evaluating the quality of AI systems and tools using multiple dimensions as follows: About AI: AI systems and tools must respect data privacy and security. Humans must be in the loop. Learning: AI systems and tools must align to our collective vision for high-quality learning, including equity. Teaching: AI systems and tools must be inspectable, explainable, and provide human alternatives to AI-based suggestions; educators will need support to exercise professional judgment and override AI models, when necessary. 9 Formative Assessment: AI systems and tools must minimize bias, promote fairness, and avoid additional testing time and burden for students and teachers. Research and Development: AI systems and tools must account for the context of teaching and learning and must work well in educational practice, given variability in students, teachers, and settings. Recommendations: Use of AI systems and tools must be safe and effective for students. They must include algorithmic discrimination protections, protect data privacy, provide notice and explanation, and provide a recourse to humans when problems arise. The people most affected by the use of AI in education must be part of the development of the AI model, system, or tool, even if this slows the pace of adoption. We return to the idea that these considerations fit together in a comprehensive perspective on the quality of AI models in the Recommendations section. Overview of Document We begin in the next section by elaborating a definition of AI, followed by addressing learning, teaching, assessment, and research and development. Organizing key insights by these topics keeps us focused on exploring implications for improving educational opportunity and outcomes for students throughout the report. Within these topics, three important themes are explored: 1. Opportunities and Risks. Policies should focus on the most valuable educational advances while mitigating risks. 2. Trust and Trustworthiness. Trust and safeguarding are particularly important in education because we have an obligation to keep students out of harm’s way and safeguard their learning experiences. 3. Quality of AI Models. The process of developing and then applying a model is at the heart of any AI system. Policies need to support evaluation of the qualities of AI models and their alignment to goals for teaching and learning during the processes of educational adoption and use. “AI in education can only grow at the speed of trust.” —Dr. Dale Allen 10 What is AI? Our preliminary definition of AI as automation based on associations requires elaboration. Below we address three additional perspectives on what constitutes AI. Educators will find these different perspectives arise in the marketing of AI functionality and are important to understand when evaluating edtech systems that incorporate AI. One useful glossary of AI for Education terms is the CIRCLS Glossary of Artificial Intelligence Terms for Educators.11 AI is not one thing but an umbrella term for a growing set of modeling capabilities, as visualized in Figure 3. Figure 3: Components, types, and subfields of AI based on Regona et al (2022).12 11 Search for “AI Glossary Educators” to find other useful definitions. 12 Regona, Massimo & Yigitcanlar, Tan & Xia, Bo & Li, R.Y.M. (2022). Opportunities and adoption challenges of AI in the construction industry: A PRISMA review. Journal of Open Innovation Technology Market and Complexity, 8(45). https://doi.org/10.3390/joitmc8010045 11 Perspective: Human-Like Reasoning “The theory and development of computer systems able to perform tasks normally requiring human intelligence such as, visual perception, speech recognition, learning, decision-making, and natural language processing.” 13 Broad cultural awareness of AI may be traced to the landmark 1968 film “2001: A Space Odyssey”—in which the “Heuristically-programmed ALgorithmic” computer, or “HAL,” converses with astronaut Frank. HAL helps Frank pilot the journey through space, a job that Frank could not do on his own. However, Frank eventually goes outside the spacecraft, HAL takes over control, and this does not end well for Frank. HAL exhibits human-like behaviors, such as reasoning, talking, and acting. Like all applications of AI, HAL can help humans but also introduces unanticipated risks—especially since AI reasons in different ways and with different limitations than people do. The idea of “human-like” is helpful because it can be a shorthand for the idea that computers now have capabilities that are very different from the capabilities of early edtech applications. Educational applications will be able to converse with students and teachers, co-pilot how activities unfold in classrooms, and take actions that impact students and teachers more broadly. There will be both opportunities to do things much better than we do today and risks that must be anticipated and addressed. The “human-like” shorthand is not always useful, however, because AI processes information differently from how people process information. When we gloss over the differences between people and computers, we may frame policies for AI in education that miss the mark. Perspective: An Algorithm that Pursues a Goal “Any computational method that is made to act independently towards a goal based on inferences from theory or patterns in data.” 14 This second definition emphasizes that AI systems and tools identify patterns and choose actions to achieve a given goal. These pattern recognition capabilities and automated recommendations will be used in ways that impact the educational process, including student learning and teacher instructional decision making. For example, today’s personalized learning systems may recognize signs that a student is struggling and may recommend an alternative instructional sequence. The scope of pattern recognition and automated recommendations will expand. 13IEEE-USA Board of Directors. (February 10, 2017). Artificial intelligence research, development and regulation. IEEE http://globalpolicy.ieee.org/wp-content/uploads/2017/10/IEEE17003.pdf 14 Friedman, L., Blair Black, N., Walker, E., & Roschelle, J. (November 8, 2021) Safe AI in education needs you. Association of Computing Machinery blog, https://cacm.acm.org/blogs/blog-cacm/256657-safe-ai-in-education-needs-you/fulltext 12 Correspondingly, humans must determine the types and degree of responsibility we will grant to technology within educational processes, which is not a new dilemma. For decades, the lines between the role of teachers and computers have been discussed in education, for example, in debates using terms such as “’computer-aided instruction,” “blended instruction,” and “personalized learning.” Yet, how are instructional choices made in systems that include both humans and algorithms? Today, AI systems and tools are already enabling the adaptation of instructional sequences to student needs to give students feedback and hints, for example, during mathematics problem solving or foreign language learning. This discussion about the use of AI in classroom pedagogy and student learning will be renewed and intensify as AI-enabled systems and tools advance in capability and become more ubiquitous. Let’s start with another simple example. When a teacher says, “Display a map of ancient Greece on the classroom screen,” an AI system may choose among hundreds of maps by noting the lesson objectives, what has worked well in similar classrooms, or which maps have desirable features for student learning. In this case, when an AI system suggests an instructional resource or provides a choice among a few options, the instructor may save time and may focus on more important goals. However, there are also forms of AI-enabled automation that the classroom instructor may reject, for example, enabling an AI system or tool to select the most appropriate and relevant readings for students associated with a historical event. In this case, an educator may choose not to utilize AI-enabled systems or tools given the risk of AI creating false facts (“hallucinating”) or steering students toward inaccurate depictions of historical events found on the internet. Educators will be weighing benefits and risks like these daily. Computers process theory and data differently than humans. AI’s success depends on associations or relationships found in the data provided to an algorithm during the AI model development process. Although some associations may be useful, others may be biased or inappropriate. Finding bad associations in data is a major risk, possibly leading to algorithmic discrimination. Every guardian is familiar with the problem: A person or computer may say, “Our data suggests your student should be placed in this class,” and the guardian may well argue, “No, you are using the wrong data. I know my child better, and they should instead be placed in another class.” This problem is not limited exclusively to AI systems and tools, but the use of AI models can amplify the problem when a computer uses data to make a recommendation because it may appear to be more objective and authoritative, even if it is not. Although this perspective can be useful, it can be misleading. A human view of agency, pursuing goals, and reasoning includes our human abilities to make sense of multiple contexts. For example, a teacher may see three students each make the same mathematical error but recognize that one student has an Individualized Education Program to address vision issues, another misunderstands a mathematical concept, and a third just experienced a frustrating interaction on the playground; the same instructional decision is therefore not appropriate. However, AI systems often lack data and judgement to appropriately include context as they detect patterns and automate decisions. Further, case studies show that technology has the potential to quickly derail from safe to unsafe or from effective to ineffective when the context shifts even slightly. For this and other reasons, people must be involved in goal setting, pattern analysis, and decision-making.15 15 Russell, S. (2019). Human compatible: Artificial intelligence and the problem of control. Viking. ISBN 978-0-525-55861-3. 13 Perspective: Intelligence Augmentation “Augmented intelligence is a design pattern for a human-centered partnership model of people and artificial intelligence (AI) working together to enhance cognitive performance, including learning, decision making, and new experiences.” 16 Foundation #1 (above) keeps humans in the loop and positions AI systems and tools to support human reasoning. “Intelligence Augmentation” (IA) 17 centers “intelligence” and “decision making” in humans but recognizes that people sometimes are overburdened and benefit from assistive tools. AI may help teachers make better decisions because computers notice patterns that teachers can miss. For example, when a teacher and student agree that the student needs reminders, an AI system may provide reminders in whatever form a student likes without adding to the teacher’s workload. Intelligence Automation (IA) uses the same basic capabilities of AI, employing associations in data to notice patterns, and, through automation, takes actions based on those patterns. However, IA squarely focuses on helping people in human activities of teaching and learning, whereas AI tends to focus attention on what computers can do. Definition of “Model” The above perspectives open a door to making sense of AI. Yet, to assess AI meaningfully, constituents must consider specific models and how they are developed. In everyday usage, the term “model” has multiple definitions. We clarify our intended meaning, which is a meaning similar to “mathematical model,” below. (Conversely, note that “model” as used in “AI model” is unlike the usage in “model school” or “instructional model” as AI model is not a singular case created by experts to serve as an exemplar.) AI models are like financial models: an approximation of reality that is useful for identifying patterns, making predictions, or analyzing alternative decisions. In a typical middle school math curriculum, students use a mathematical model to analyze which of two cell phone plans is better. Financial planners use this type of model to provide guidance on a retirement portfolio. At its heart, AI is a highly advanced mathematical toolkit for building and using models. Indeed, in well-known chatbots, complex essays are written one word at a time. The underlying AI model predicts which next words would likely follow the text written so far; AI chatbots use a very large statistical model to add one likely word at a time, thereby writing surprisingly coherent essays. When we ask about the model at the heart of AI, we begin to get answers about “what aspects of reality does the model approximate well?” and “how appropriate is it to the decision to be made?” One could similarly ask about algorithms—the specific decision-making processes that an AI model uses to go from inputs to outputs. One could also ask about the quality of the data used to build the model—for example, how representative is that data? Switching among three terms— 16Gartner (n.d.) Gartner glossary: Augmented intelligence. Gartner. https://www.gartner.com/en/information- technology/glossary/augmented-intelligence 17Englebart, D.C. (October 1962). Augmenting human intellect: A conceptual framework. SRI Summary Report AFOSR- 3223. https://www.dougengelbart.org/pubs/augment-3906.html 14 models, algorithms, and data—will become confusing. Because the terms are closely related, we’ve chosen to focus on the concept of AI models. We want to bring to the fore the idea that every AI model is incomplete, and it's important to know how well the AI model fits the reality we care about, where the model will break down, and how. Sometimes people avoid talking about the specifics of models to create a mystique. Talking as though AI is unbounded in its potential capabilities and a nearly perfect approximation to reality can convey an excitement about the possibilities of the future. The future, however, can be oversold. Similarly, sometimes people stop calling a model AI when its use becomes commonplace, yet such systems are still AI models with all of the risks discussed here. We need to know exactly when and where AI models fail to align to visions for teaching and learning. Insight: AI Systems Enable New Forms of Interaction AI models allow computational processes to make recommendations or plans and also enable them to support forms of interaction that are more natural, such as speaking to an assistant. AI- enabled educational systems will be desirable in part due to their ability to support more natural interactions during teaching and learning. In classic edtech platforms, the ways in which teachers and students interact with edtech are limited. Teachers and students may choose items from a menu or in a multiple-choice question. They may type short answers. They may drag objects on the screen or use touch gestures. The computer provides outputs to students and teachers through text, graphics, and multimedia. Although these forms of inputs and outputs are versatile, no one would mistake this style of interaction with the way two people interact with one another; it is specific to human-computer interaction. With AI, interactions with computers are likely to become more like human-to-human interactions (see Figure 4). A teacher may speak to an AI assistant, and it may speak back. A student may make a drawing, and the computer may highlight a portion of the drawing. A teacher or student may start to write something, and the computer may finish their sentence—as when today’s email programs can complete thoughts faster than we can type them. Additionally, the possibilities for automated actions that can be executed by AI tools are expanding. Current personalization tools may automatically adjust the sequence, pace, hints, or trajectory through learning experiences. 18 Actions in the future might look like an AI system or tool that helps a student with homework 19 or a teaching assistant that reduces a teacher’s workload by recommending lesson plans that fit a teacher’s needs and are similar to lesson plans a teacher previously liked. 20 Further, an AI-enabled assistant may appear as an additional “partner” in a small group of students who are working together on a collaborative assignment. 21 An AI-enabled tool may also help teachers with complex classroom routines. 22 For example, a 18 Shemshack, A., Spector, J.M. (2020) A systematic literature review of personalized learning terms. Smart Learning Environments, 7(33). https://doi.org/10.1186/s40561-020-00140-9 19 Roschelle, J., Feng, M., Murphy, R. & Mason, C.A. (2016). Online mathematics homework increases student achievement. AERA Open, 2(4), 1-12. DOI: 10.1177/2332858416673968 20Celik, I., Dindar, M., Muukkonen, H. & Järvelä, S. (2022). The promises and challenges of artificial intelligence for teachers: A systematic review of research. TechTrends, 66, 616–630. https://doi.org/10.1007/s11528-022-00715-y 21Chen, C., Park, H.W. & Breazeal, C. (2020). Teaching and learning with children: Impact of reciprocal peer learning with a social robot on children’s learning and emotive engagement. Computers & Education, 150, https://doi.org/10.1016/j.compedu.2020.103836 22Holstein, K., McLaren, B.M., & Aleven, V. (2019). Co-designing a real-time classroom orchestration tool to support teacher–AI complementarity. Journal of Learning Analytics, 6(2). https://doi.org/10.18608/jla.2019.62.3 15 tool may help teachers with orchestrating 23 the movement of students from a full class discussion into small groups and making sure each group has the materials needed to start their work. Figure 4. Differences that teachers and students may experience in future technologies. Key Recommendation: Human in the Loop AI Many have experienced a moment where technology surprised them with an uncanny ability to recommend what feels like a precisely personalized product, song, or even phrase to complete a sentence in a word processor such as the one being used to draft this document. Throughout this supplement, we talk about specific, focused applications where AI systems may bring value (or risks) into education. At no point do we intend to imply that AI can replace a teacher, a guardian, or an educational leader as the custodian of their students’ learning. We talk about the limitations of models in AI and the conversations that educational constituents need to have about what qualities they want AI models to have and how they should be used. “We can use AI to study the diversity, the multiplicity of effective learning approaches and think about the various models to help us get a broader understanding of what effective, meaningful engagement might look like across a variety of different contexts.” —Dr. Marcelo Aaron Bonilla Worsley 23Roschelle, J., Dimitriadis, Y. & Hoppe, U. (2013). Classroom orchestration: Synthesis. Computers & Education, 69, 512-526. https://doi.org/10.1016/j.compedu.2013.04.010 16 These limitations lead to our first recommendation: that we pursue a vision of AI where humans are in the loop. That means that people are part of the process of noticing patterns in an educational system and assigning meaning to those patterns. It also means that teachers remain at the helm of major instructional decisions. It means that formative assessments involve teacher input and decision making, too. One loop is the cycle of recognizing patterns in what students do and selecting next steps or resources that could support their learning. Other loops involve teachers planning and reflecting on lessons. Response to Intervention is another well-known type of loop. The idea of humans in the loop is part of our broader discussions happening about AI and society, not just AI in education. Interested readers could look for more on human-centered AI, responsible AI, value-sensitive AI, AI for social good, and other similar terms that ally with humans in the loop, such as “human-centered AI.” Exercising judgement and control in the use of AI systems and tools is an essential part of providing the best opportunity to learn for all students—especially when educational decisions carry consequence. AI does not have the broad qualities of contextual judgment that people do. Therefore, people must remain responsible for the health and safety of our children, for all students’ educational success and preparation for their futures, and for creating a more equitable and just society. 17 Learning The Department’s long-standing edtech vision sees students as active learners; students participate in discussions that advance their understanding, use visualizations and simulations to explain concepts as they relate to the real world, and leverage helpful scaffolding and timely feedback as they learn. Constituents want technology to align to and build on these and other research-based understandings of how people learn. Educators can draw upon two books titled How People Learn and How People Learn II by the National Academies of Sciences, Engineering, and Medicine for a broad synthesis of what we know about learning. 24 As we shape AI-enhanced edtech around research-based principles, a key goal must be to strengthen and support learning for those who have experienced unfavorable circumstances for learning, such as caused by the COVID-19 pandemic or by broader inequities. And we must keep a firm eye toward the forms of learning that will most benefit learners in their future lives in communities and workplaces. Examples of AI supporting learning principles in this section include the following: AI-based tutoring for students as they solve math problems (based on cognitive learning theories), adapting to learners with special needs (based on the Universal Design for Learning framework and related theories), and AI support for effective student teamwork (based on theories in the field called “Computer Supported Collaborative Learning”). Insight: AI Enables Adaptivity in Learning Adaptivity has been recognized as a key way in which technology can improve learning. 25 AI can be a toolset for improving the adaptivity of edtech. AI may improve a technology’s ability to meet students where they are, build on their strengths, and grow their knowledge and skills. Because of AI’s powers of work with natural forms of input and the foundational strengths of AI models (as discussed in the What is AI? section), AI can be an especially strong toolkit for expanding the adaptivity provided to students. And yet, especially with AI, adaptivity is always more specific and limited than what a broad phrase like “meet students where they are” might suggest. Core limits arise from the nature of the model at the heart of any specific AI-enabled system. Models are approximations of reality. When important parts of human learning are left out of the model or less fully developed, the resulting adaptivity will also be limited, and the resulting supports for learning may be brittle or narrow. Consequently, this section on Learning focuses on one key concept: Work toward AI models that fit the fullness of visions for learning—and avoid limiting learning to what AI can currently model well. AI models are demonstrating greater skills because of advances in what are called “large language models” or sometimes “foundational models.” These very general models still have limits. For example, generative AI models discussed in the mainstream news can quickly generate convincing essays about a wide variety of topics while other models can draw credible images based on just a few prompts. Despite the excitement about foundational models, experts in our 24National Research Council. 2000. How people learn: Brain, mind, experience, and school. The National Academies Press. https://doi.org/10.17226/9853; National Academies of Sciences, Engineering, and Medicine. 2018. How people learn II: Learners, contexts, and cultures. The National Academies Press. https://doi.org/10.17226/24783 25 Aleven, V., McLaughlin, E. A., Glenn, R. A., & Koedinger, K. R. (2016). Instruction based on adaptive learning technologies. In Mayer, R.E. & Alexander, P.A., Handbook of research on learning and instruction, 522-560. ISBN: 113883176X 18 listening sessions warned that AI models are narrower than visions for human learning and that designing learning environments with these limits in mind remains very important. The models are also brittle and can’t perform well when contexts change. In addition, they don’t have the same “common sense” judgment that people have, often responding in ways that are unnatural or incorrect. 26 Given the unexpected ways in which foundational models miss the mark, keeping humans in the loop remains highly important. Intelligent Tutoring Systems: An Example of AI Models One long-standing type of AI-enabled technology is an Intelligent Tutoring System (ITS).27 In an early success, scientists were able to build accurate models of how human experts solve mathematical problems. The resulting model was incorporated into a system that would observe student problem solving as they worked on mathematical problems on a computer. Researchers who studied human tutors found that feedback on specific steps (and not just right or wrong solutions) is a likely key to why tutoring is so effective. 28 For example, when a student diverged from the expert model, the system gave feedback to help the student get back on track. 29 Importantly, this feedback went beyond right or wrong, and instead, the model was able to provide feedback on specific steps of a solution process. A significant advancement of AI, therefore, can be its ability to provide adaptivity at the step-by-step level and its ability to do so at scale with modest cost. As a research and development (R&D) field emerged to advance ITS, the work has gone beyond mathematics problems to additional important issues beyond step-by-step problem solving. In the early work, some limitations can be observed. The kinds of problems that an ITS could support were logical or mathematical, and they were closed tasks, with clear expectations for what a solution and solution process should look like. Also, the “approximation of reality” in early AI models related to cognition and not to other elements of human learning, for example, social or motivational aspects. Over time, these early limitations have been addressed in two ways: by expanding the AI models and by involving humans in the loop, a perspective that is also important now. Today, for example, if an ITS specializes in feedback as a student practices, a human teacher could still be responsible for motivating student engagement and self-regulation along with other aspects of instruction. In other contemporary examples, the computer ITS might focus on problem solving practice, while teachers work with students in small groups. Further, students can be in the loop with AI, as is the case with “open learner models”—a type of AI-enabled system that provides information to support student self-monitoring and reflection.30 26Dieterle, E., Dede, C. & Walker, M. (2022). The cyclical ethical effects of using artificial intelligence in education. AI & Society. https://link.springer.com/article/10.1007/s00146-022-01497-w 27Mousavinasab, E., Zarifsanaiey, N., R. Niakan Kalhori, S., Rakhshan, M., Keikha, L., & Ghazi Saeedi, M. (2021). Intelligent tutoring systems: A systematic review of characteristics, applications, and evaluation methods. Interactive Learning Environments, 29(1), 142–163. https://psycnet.apa.org/doi/10.1080/10494820.2018.1558257 28 Van Lehn, K. (2011) The relative effectiveness of human tutoring, intelligent tutoring systems, and other tutoring systems. Educational Psychologist, 46(4), 197-221. https://doi.org/10.1080/00461520.2011.611369 29Ritter, S., Anderson, J.R., Koedinger, K.R. & Corbett, A. (2007). Cognitive Tutor: Applied research in mathematics education. Psychonomic Bulletin & Review, 14, 249–255/ https://doi.org/10.3758/BF03194060 30Winne, P.H. (2021). Open learner models working in symbiosis with self-regulating learners: A research agenda. International Journal of Artificial Intelligence in Education, 31(3), 446-459. https://doi.org/10.1007/s40593-020-00212-4 19 Although R&D along the lines of an ITS should not limit the view of what’s possible, such an example is useful because so much research and evaluation has been done on the ITS approach. Researchers have looked across all the available high-quality studies in a meta-analysis and concluded that ITS approaches are effective.31 Right now, many school systems are looking at high-intensity human tutoring to help students with unfinished learning. Human tutoring is very expensive, and it is hard to find enough high-quality human tutors. With regard to large-scale needs, if it is possible for an ITS to supplement what human tutors do, it might be possible to extend beyond the amount of tutoring that people can provide to students. Important Directions for Expanding AI-Based Adaptivity Adaptivity is sometimes referred to as “personalization.” Although this is a convenient term, many observers have noted how imprecise it is. 32 For some educators, personalization means giving learners “voice and choice,” and for others it means that a learning management system recommends an individual “playlist” of activities to each student. Hidden in that imprecision is the reality that many edtech products that personalize do so in limited ways. Adjusting the difficulty and the order of lesson materials are among the two most common ways that edtech products adapt. And yet, any teacher knows there is more to supporting learning than adjusting the difficulty and sequence of materials. For example, a good teacher can find ways to engage a student by connecting to their own past experiences and can shape explanations until they really connect in an “aha!” moment for that student. When we say, “meet the learner where they are,” human teachers bring a much more complete picture of each learner than most available edtech. The teacher is also not likely to “over personalize” (by performing like an algorithm that only presents material for which the learner has expressed interest), thereby limiting the student’s exposure to new topics. The nature of “teachable moments” that a human teacher can grasp is broader than the teachable moments today’s AI models grasp. In our listening sessions, we heard many ways in which the core models in an AI system must be expanded. We discuss these below. 1. From deficit-based to asset-oriented. Listening session attendees noted that the rhetoric around adaptivity has often been deficit-based; technology tries to pinpoint what a student is lacking and then provides instruction to fill that specific gap. Teachers also orient to students' strengths; they find competencies or “assets” a student has and use those to build up the students’ knowledge. AI models cannot be fully equitable while failing to recognize or build upon each student’s sources of competency. AI models that are more asset-oriented would be an advance. 2. From individual cognition to including social and other aspects of learning. The existing adaptivity rhetoric has also tended to focus on individualized learning and mostly on cognitive elements of learning, with motivational and other elements only brought in to support the cognitive learning goals. Attendees observe that their vision for learning is broader than cognition. Social learning is important, for example, especially 31Kulik, J.A., & Fletcher, J.D. (2016). Effectiveness of intelligent tutoring systems: A meta-analytic review. Review of Educational Research, 86(1), 42–78; Ma, W., Adescope, O.O, Nesbit, J.C. & Liu, Q. (2014). Intelligent tutoring systems and learning outcomes: A meta-analysis. Journal of Educational Psychology, 106(4), 901–918. http://dx.doi.org/10.1037/a0037123 32Plass, J.L., & Pawar, S. (2020). Toward a taxonomy of adaptivity for learning. Journal of Research on Technology in Education, 52(3), 275–300. https://doi.org/10.1080/15391523.2020.1719943; 20 for students to learn to reason, explain, and justify. For students who are learning English, customized and adaptive support for improving language skills while learning curricular content is clearly important. Developing self-regulation skills is also important. A modern vision of learning is not individualistic; it recognizes that students learn in groups and communities too. 3. From neurotypical to neurodiverse learners. AI models could help in including neurodiverse learners (students who access, process, and interact with the world in less common ways than “neurotypical” students) who could benefit from different learning paths and from forms of display and input that fit their strengths. Constituents want AI models that can support learning for neurodiverse learners and learners with disabilities. Thus, they want AI models that can work with multiple paths to learning and multiple modalities of interaction. Such models should be tested for efficacy, to guard against the possibility that some students could be assigned a “personalized” but inadequate learning resource. In addition, some systems for neurodiverse students are presently underutilized, so designs that support intended use will also be important. 4. From fixed tasks to active, open, and creative tasks. As mentioned above, AI models are historically better at closed tasks like solving a math problem or logical tasks like playing a game. In terms of life-wide and lifelong opportunities, we value learning how to succeed at open-ended and creative tasks that require extended engagement from the learner, and these are often not purely mathematical or logical. We want students to learn to invent and create innovative approaches. We want AI models that enable progress on open, creative tasks. 5. From correct answers to additional goals. At the heart of many adaptivity approaches now on the market, the model inside the technology counts students' wrong answers and decides whether to speed up, slow down, or offer a different type of learning support. Yet, right and wrong answers are not the only learning goals. We want students to learn how to self-regulate when they experience difficulties in learning, for example, such as being able to persist in working on a difficult problem or knowing how and when to ask for help. We want learners to become skilled in teamwork and in leading teams. As students grow, we want them to develop more agency and to be able to act on their own to advance toward their own learning goals. Listing every dimension of expansion that we heard in our listening sessions is beyond the scope of this report. Some additional dimensions are presented in the following sections on Teaching, Assessment, and Research. For example, in Research, we discuss all the ways in which AI systems have trouble with context—context that humans readily grasp and consider. Overall, constituents in the listening sessions realized we need an ambitious outlook on learning to respond to the future today’s learners face. Constituents were concerned about ways in which AI might narrow learning. For example, if the incorporation of AI into education slowed attention to students’ skills on creative, open-ended tasks and their ability to lead and collaborate in teams, then school districts may be less able to realize their students’ progress in relation to a Portrait of a Graduate who excels in communication and other skills valued in communities and careers. 21 Constituents reminded us that as we conceptualize what we want AI in edtech to accomplish, we must start and constantly revisit a human-centered vision of learning. A Duality: Learning With and About AI As AI is brought into schools, two broad perspectives about AI in education arise: (1) AI in support of student learning; and (2) support for learning about AI and related technologies. So far, we’ve discussed AI systems and tools to support student learning and mastery of subjects like mathematics and writing. Yet, it is also important that students learn about AI, critically examine its presence in education and society, and determine its role and value in their own lives and careers. We discuss risks across each section in this report. Here, it is important for students to become more aware of and savvy to the risks of AI—including risks of bias and surveillance—as they appear in all elements of their lives. In the recent past, schools have supported students’ understanding of cybersecurity, for example. AI will bring new risks, and students need to learn about them. We are encouraged by efforts we’ve seen underway that would give students opportunities to learn about how AI works while also giving them opportunities to discuss relevant topics like privacy and security.33 Other learning goals are noted in the K-12 Computer Science Framework. We’ve seen that students can begin learning about AI in elementary, middle, and high school. They can use AI to design simulations and products that they find exciting. And we’ve seen that students want to talk about the ethics of products they experience in their everyday lives and have much to say about the kinds of products they’d like to see or not see in school. (And later, in the Research section, we note the desire for co-design processes that involve students in creating the next generation of AI-enabled edtech). Overall, it’s important to balance attention to using AI to support learning and giving students opportunities to learn about AI. A Challenge: Systems Thinking About AI in Education As AI expands into the educational system, our listening session attendees reminded us that it will be entering parts or locations of the system that are presently dysfunctional. AI is certainly not a fix for broken systems, and instead, must be used with even more care when the systems’ context is unstable or uncertain. 33Forsyth, S., Dalton, B., Foster, E.H., Walsh, B., Smilack, J., & Yeh, T. (2021, May). Imagine a more ethical AI: Using stories to develop teens' awareness and understanding of artificial intelligence and its societal impacts. In 2021 Conference on Research in Equitable and Sustained Participation in Engineering, Computing, and Technology (RESPECT). IEEE. https://doi.org/10.1109/RESPECT51740.2021.9620549; Zhang, H., Lee, I., Ali, S., DiPaola, D., Cheng, Y., & Breazeal, C. (2022). Integrating ethics and career futures with technical learning to promote AI literacy for middle school students: An exploratory study. International Journal of Artificial Intelligence in Education, 1–35. https://doi.org/10.1007/s40593-022- 00293-3 22 “First and foremost, they are getting deployed in educational contexts that are already fragmented and broken and unequal. Technology doesn't discriminate—we do. So, as we think about the application of these new systems, we have to really think about the contextual application of AI.” —Dr. Nicole Turner As discussed previously, because AI systems and tools do not fully align with goals for learning, we have to design educational settings to situate AI in the right place, where educators and other adults can make effective use of these tools for teaching and learning. Within the ITS example, we saw that AI could make learning by practicing math problems more effective, and a whole curricular approach might include roles for teachers that emphasize mathematical practices like argumentation and modeling. Further, small-group work is likely to remain important: Students might work in small groups to use mathematics to predict or justify as they work on responding to a realistic challenge. At the present, one “right place” for people, and not AI, is understanding how learning can be culturally responsive and culturally sustaining, as AI is not even close to being ready to connect learning to the unique strengths in a student’s community and family. Open Questions About AI for Learning With advances occurring in the foundations for AI, opportunities to use AI in support of learning are rapidly expanding. As we explore these opportunities, the open questions below deserve ongoing attention: To what extent is AI enabling adaptation to students’ strengths and not just deficits? Is AI enabling improved support for learners with disabilities and English language learners? How are youth voices involved in choosing and using AI for learning? Is AI leading to narrower student activities (e.g., procedural math problems), or the fuller range of activities highlighted in the National Educational Technology Plan (NETP), which emphasizes features such as personalized learning, project-based learning, learning from visualizations, simulations, and virtual reality, as well as learning across school, community, and familial settings? Is AI supporting the whole learner, including social dimensions of learning such as enabling students to be active participants in small group and collaborative learning? For example, does AI contribute to aspects of student collaboration we value like shared attention, mutual engagement, peer help, self-regulation, and building on each other’s contributions? When AI is used, are students’ privacy and data protected? Are students and their guardians informed about what happens with their data? How strong are the processes or systems for monitoring student use of AI for barriers, bias, or other undesirable consequences of AI use by learners? How are emergent issues addressed? Is high-quality research or evaluations about the impacts of using the AI system for student learning available? Do we know not only whether the system works but for whom and under what conditions? 23 Key Recommendation: Seek AI Models Aligned to a Vision for Learning We’ve called attention to how advances in AI are important to adaptivity but also to ways in which adaptivity is limited by the model’s inherent quality. We noted that a prior wave of edtech used the term “personalized” in differing ways, and it was often important to clarify what personalization meant for a particular product or service. Thus, our key recommendation is to tease out the strengths and limitations of AI models inside forthcoming edtech products and to focus on AI models that align closely to desired visions of learning. AI is now advancing rapidly, and we should differentiate between products that have simple AI-like features inside and products that have more sophisticated AI models. Looking at what’s happening in research and development, we can see significant effort and push toward overcoming these limitations. We noted that decision makers need to be careful about selecting AI models that might narrow their vision for learning, as general artificial intelligence does not exist. And because AI models will always be narrower than real world experience, we need to proceed with systems thinking in which humans are in the loop, with the strengths and weaknesses of the specific educational system considered. We hold that the full system for learning is broader than its AI component. 24 Teaching Teachers have long envisioned many things that technology could make possible for teachers, their classrooms, and their students but not the changes wrought by the recent pandemic. Today, nearly all teachers have experienced uses of technologies for instruction that no one anticipated. Some of those experiences were positive, and others were not. All of the experiences provide an important context as we think further about teaching and technology. There is a critical need to focus on addressing the challenges teachers experience. It must become easier for teachers to do the amazing work they always do. We must also remember why people choose the teaching profession and ensure they can do the work that matters. This section discusses examples of AI supporting teachers and teaching including these concepts: AI assistants to reduce routine teaching burdens; AI that provides teachers with recommendations for their students’ needs and extends their work with students; and AI that helps teachers to reflect, plan, and improve their practice. “One opportunity I see with AI is being able to reduce the amount of attention I have to give to administrative things and increase the amount of attention I can give to my students with their learning needs in the classroom. So that's the first one that I'd say that I'm super excited about the possibility of AI to support me as a teacher." —Vidula Plante Always Center Educators in Instructional Loops To succeed with AI as an enhancement to learning and teaching, we need to always center educators (ACE). Practically speaking, practicing “ACE in AI” means keeping a humanistic view of teaching front and center. ACE leads the Department to confidently respond “no” when asked “will AI replace teachers?” ACE is not just about making teachers’ jobs easier but also making it possible to do what most teachers want to do. That includes, for example, understanding their students more deeply and having more time to respond in creative ways to teachable moments. To bring more precision to how and where we should center educators, we return to our advocacy for human in the loop AI and ask, what are the loops in which teachers should be centered? Figure 5 suggests three key loops (inspired by research on adaptivity loops 34): 34Aleven, V., McLaughlin, E.A., Glenn, R.A., & Koedinger, K.R. (2016). Instruction based on adaptive learning technologies. In Mayer, R.E. & Alexander, P.A., Handbook of research on learning and instruction, 522-560. ISBN: 113883176X 25 1. The loop in which teachers make moment-to-moment decisions as they do the immediate work of teaching. 2. The loop in which teachers prepare for, plan, and reflect on teaching, which includes professional development. 3. The loop in which teachers participate in decisions about the design of AI-enabled technologies, participate in selecting the technologies, and shape the evaluation of technologies—thus setting a context for not only their own classroom but those of fellow teachers as well. Figure 5: Three ways to center educators as we conceptualize human in the loop AI Please note that in the next section, on Formative Assessment, we also discuss teachers’ important role in feedback loops that support students and enable school improvement. That section also includes a discussion of the concepts of “bias” and “fairness,” which are important to teachers. Insight: Using AI to Improve Teaching Jobs The job of teaching is notoriously complex, with teachers making thousands of decisions each day. Teachers participate in classroom processes, in interactions with students beyond classrooms, in work with fellow teachers, and in administrative functions. They also are part of their communities and thus are expected to interact with families and caregivers. 26 If the teacher is able to efficiently predict and understand the range of other answers given by students in the class, it becomes possible to think creatively about the novel answer and figure how and why the student might have generated it.35 We think about how much easier some everyday tasks have become. We can request and receive alerts and notifications about events. Selecting music that we want to hear used to be a multistep process (even with digital music), and now we can speak the name of a song we want to hear, and it plays. Likewise, mapping a journey used to require a cumbersome study of maps, but now cell phones let us choose among several transportation options to reach a destination. Why can’t teachers be supported to notice changing student needs and provided with supports to enact a technology-rich lesson plan? Why can’t they more easily plan their students’ learning journeys? When things change in a classroom, as they always do, why don’t the tools of the classroom make it easier for teachers to adapt to student strengths and needs on the fly? Figure 6: Teachers work about 50 hours a week, spending less than half the time in direct interaction with students. A report by McKinsey 36 first suggested that AI’s initial benefit could be to improve teaching jobs by reducing low-level burdens in administrative or clerical work (Figure 6). The report also suggests that recovered time from AI-enabled technology should be rededicated toward more 35 Hammerness, K., Darling-Hammond, L., & Bransford, J. (2005). Preparing teachers for a changing world: What teachers should learn and be able to do. Jossey-Bass. ISBN: 0787996343 36Bryant, J., Heitz,C., Sanghvi, S., & Wagle, D. (2020, January 14). How artificial intelligence will impact K-12 teachers. McKinsey. https://www.mckinsey.com/industries/education/our-insights/how-artificial-intelligence-will-impact-k-12- teachers 27 effective instruction—particularly, outcomes such as reducing the average 11 hours of weekly preparation down to only six. We highlight these opportunities and two others below. 1. Handling low-level details to ease teaching burdens and increase focus on students. A good teacher must master all levels of details, big and small. When working with a particular student, the teacher may wish to later send that student a helpful learning resource. How will they remember to send it? A voice assistant or other forms of an AI assistant could make it easier to stay organized by categorizing simple voice notes for teachers to follow up on after a classroom session ends. We are beginning to see AI- enabled voice assistants in the market, and they could do many simple tasks so that the teachers can stay focused on students. These tasks can include record-keeping, starting and stopping activities, controlling displays, speakers, and other technologies in the classroom, and providing reminders. Many workers may eventually use assistants to make their jobs easier, and teachers are the most deserving of efforts to ease their jobs now. 2. Extending beyond the teacher's availability with their students but continuing to deliver on the teacher’s intent. Teachers almost always want to do more with each student than they can, given the limited number of hours before the next school day. A teacher may wish to sit with the student as they practice 10 more math problems, giving them ongoing support and feedback. If the teacher can sit with the student for only three problems, perhaps they could delegate to an AI-enabled learning system to help with the rest. Teachers cannot be at their best if on call at all hours to help with homework, but perhaps they can indicate what types of supports, hints, and feedback they want students to receive while studying after school hours. An AI assistant can ensure that students have that support wherever and whenever they do homework or practice skills on their own. Teachers may wish to provide more extensive personal notes to families/caregivers, and perhaps an AI assistant could help with drafts based on students’ recent classroom work. Then, the teacher could review the AI-generated comments and quickly edit where needed before returning it to the student for another draft. AI tools might also help teachers with language translation so they can work with all parents and caregivers of their students. AI tools might also help teachers with awareness. For example, in the next section, Formative Assessment, we note that teachers can’t always know what’s going on for each student and in each small group of students; emerging products might signal to the teacher when a student or teacher may need some more personal attention. 3. Making teacher professional development more productive and fruitful. Emerging products already enable a teacher to record her classroom and allow an AI algorithm to suggest highlights of the classroom discussion worth reviewing with a professional development coach.37 AI can compute metrics, such as whether students have been talking more or less, which are difficult for a teacher to calculate during a lesson. 38 For 37Chen, G., Clarke, S., & Resnick, L.B. (2015). Classroom Discourse Analyzer (CDA): A discourse analytic tool for teachers. Technology, Instruction, Cognition and Learning, 10(2), 85-105 38Jensen, E., Dale, M., Donnelly, P.J., Stone, C., Kelly, S., Godley, A. & D'Mello, S.K. (2020). Toward automated feedback on teacher discourse to enhance teacher learning. In Proceedings of the 2020 CHI Conference on Human Factors in Computing Systems (CHI '20). https://doi.org/10.1145/3313831.3376418 28 teachers who want to increase student engagement, these metrics can be a valuable tool. Classroom simulation tools are also emerging and can enable teachers to practice their skills in realistic situations. 39 Simulators can include examples of teaching from a real classroom while changing the faces and voices of the participants so that teaching situations can be shared and discussed among teachers without revealing identities. Note the emphasis above on what listening-session panelist Sarah Hampton said about the human touch. Teachers will feel that AI is helping them teach with a focus on their human connection to their students when the necessary (but less meaningful) burdens of teaching are lessened. In Figure 7, below, see concerns that teachers raised about AI during listening sessions. Figure 7: Concerns raised during the listening session about teaching with AI Preparing and Supporting Teachers in Planning and Reflecting ACE also means preparing teachers to take advantage of possibilities like those listed above and more. In the Research section, we highlight how pre-service education still tends to compartmentalize and inadequately address the topic of technology. That section suggests a need to invest in research about how to deeply integrate technology in pre-service teacher training programs. In-service teachers, too, will need professional development to take advantage of opportunities that AI can provide, like those presented in the Teaching section. Professional development will need to be balanced not only to discuss opportunities but also to inform teachers of new risks, while providing them with tools to avoid the pitfalls of AI. 39Ersozlu, Z., Ledger, S., Ersozlu, A., Mayne, F., & Wildy, H. (2021). Mixed-reality learning environments in teacher education: An analysis of TeachLivETM Research. SAGE Open, 11(3). https://doi.org/10.1177/21582440211032155. 29 “Humans are well suited to discern the outcomes…because we are the ones that have the capacity for moral reflection and empathy. So, in other words, I want the AI to help me really quickly and easily see what my student needs in their learning journey.” —Sarah Hampton By nature, teaching requires significant time in planning as well to account for the breadth of needs across their rosters—especially for inclusive learning environments and students with IEPs and 504 plans. AI could help teachers with recommendations that are tuned to their situation and their ways of practicing teaching and support with adapting found materials to fit their exact