AI Governance & Ethics PDF
Document Details
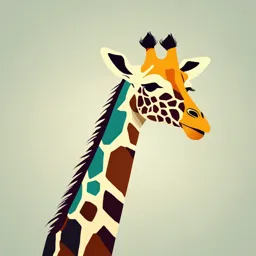
Uploaded by CushyPenguin2486
Tags
Summary
This document discusses AI governance and ethics, covering topics such as ethical considerations, risks, biases, and practical implementation guidelines through the use of AI.
Full Transcript
AI 1 AI Governance - AI and ML help machines make decision faster than humans - Should be designed to support organisation goals - AI must meet ethical standards and not break any rules - Trustworthy AI -> AI works responsibly and ethically - Part of IT but is still e...
AI 1 AI Governance - AI and ML help machines make decision faster than humans - Should be designed to support organisation goals - AI must meet ethical standards and not break any rules - Trustworthy AI -> AI works responsibly and ethically - Part of IT but is still evolving and less defined - Key in IT governance to manage emerging technologies - AI treated as emerging technologies due to its risks and lack of established best practices Need for AI ethics - AI will take over some decision-making from humans - AI systems need to make ethical decisions - AI ethics prevents AI from making unfair decisions and requires humans to maintain control over AI systems, as they start to make more decisions in place of humans - Ensure AI systems do not run rogue or make bad decisions - Keep AI human-centered without limiting its benefits - Ensure AI outcomes stay within set boundaries - Regulate AI in sensitive areas like healthcare, law and engineering AI risks: - Unexplainabilty: hard to understand how AI makes decisions, cant explain how it work o Explainability: ▪ Shows how AI makes decisions ▪ Build trusts in system performance ▪ Help users accept and trust AI ▪ Reduces risk of unexpected behaviour o Mitigations: ▪ Spend more effort in understanding AI system processes ▪ Implement human oversight to check AI decisions - Biasness or discrimination: AI produce results favouring certain groups based on gender, race or other traits o Causes: biased training data leads to biased results o Fix: ▪ Use balanced training data with equal representation of groups ▪ Check and adjust training data to reduce bias ▪ Adjust AI system manually to reduce bias ▪ Use algorithms that reduce known biases - Misclassification: AI make wrong decision due to language, culture or context diff o Often cause by poor training or incomplete data o Mitigation: ▪ Check if ai is suitable for the situation ▪ Allow humans to handle unusual cases AI Ethics BoK (Body of Knowledge) - Developed by SCS to guide SG in using AI - Helps inform, educate and set best practices for AI - Designed for: AI solution providers, businesses and organisation, consumers Guiding principles of AI BOK that promote trust and understand AI: 1. Transparent, fair & explainable o Organisation shld ensure AI is used in a way that aligns with principles o Builds trust and confidence in AI 2. Human-centric o AI shld enhance human abilities while protecting well-being and safety AI Ethics BOK approach to AI 1. Internal governance o Create structures to ensure accountability for AI decisions o Manage risks, values and responsibilities of AI usage 2. Human-centricity o Set acceptable risks lvls for using AI o Decide how much human involvement is needed in AI decisions 3. Operations management o Build, choose and maintain AI models o Manage data, ensuring auditabilty 4. Stakeholder communications o Plan how to communicate with stakeholders o Build and maintain good rs AI 2 AI Model Governance Framework (sg) – provides practical guidance to organisations to address key ethical and governance issues about using AI Features: Not limited to specific algorithms – applicable to all AI methods, focusing on general design and use Not tied to specific technologies – can be used with any system or technology Applicable across industries Works for all organisation sizes and types – fits organisations of any size or business models like B2C or B2B Objective: 1. Build confidence in AI – ensure responsible usage of AI by organisations and gain stakeholder trust 2. Align data protection practices – help organisations show they comply with policies like PDPA and OECD privacy principles thru proper internal policies, structures and processes Guiding principles - Ensure decision-making process is explainable, transparent and fair - AI solutions should be human-centric, protecting interests, wellbeing and safety of humans Internal Governance structures & measures - Define roles and responsibilities – clearly assign roles for managing AI systems - Assign specific responsibilities – set clear duties for those using AI - Manage AI risks – incl AI risks in the organisation risk management system - Align with ethical standards – make sure AI ethics match organisation values Operations management - Minimise bias – ensure AI data and model design reduce unfair bias - Balance needs – ensures AI meets both operational efficiency and stakeholder obligations - Focus on data quality – quality and selection of data crucial for AI systems - Data lineage – understand the journey of data from origin to end-use - Data provenance – keep a record of where data comes from and how it has been transformed Stakeholder Interaction and communication - Create and share policies – develop clear policies and ensure users and stakeholders are aware of them - Encourage feedback – provide two-way communication to gather feedback for improvements - Tailore communication – adjust communication style to suit diff audiences - Allow opt-out options – for consumers, consider offering an opt-out option if possible Human centricity in AI implementations - Focuses on ensuring transparency, trustworthiness and explainability in AI systems - Level of human control to maintain these properties - Balancing control and efficiency – keeping human control over AI ensures human centricity but may conflict with AI’s strength like making faster, more accurate decisions Level of Human Involvement - Potential to replace role of human roles in operations - Organisations may choose to involve humans in key areas to maintain trust, despite potential downsides Human in the loop: human oversee AI, maintaining full control while AI provides recommendations Human out of the loop: no human oversight, AI full control without human override option Human over the loop: Humans monitor AI, can take control if undesirable events occur Human Involvement Model Human in the loop (medical diagnosis, operational technology applications like utilities) - AI cannot be left to make decisions independently due to high impact or unacceptable performance Human out of the loop (personal assistants (siri, alexa), online recommendation engines) - AI operates without human involvements, decisions have low impact Human over the loop (flight, driving, marine simulators, supply chain systems) - AI operates on its own but needs human oversight or intervention Risk Impact Matrix - Assess risk, based on potential harm, reversibility and likelihood - Determines lvl of human involvement in the AI system Green = human out of the loop Easy to implement – can be deploy with little risk, as harm is minor or reversible Eg. Shopping reco system - Low risk as custs can ignore poor suggestions - Main risk -> recommending inappropriate items based on cust gender or race Yellow = human over the loop Fully automating AI too risky, feedback system should be in place to detect abnormal behaviour Eg. New reco system - Most news items don’t need oversight, but a human should review flagged content for harmful or potentially false material Orange = human in the loop Errors can cause moderate to significant harm, not easily reversible Human must always be involved to make final decision AI typically provide recommendations, not final decisions Eg. Medical diagnosis system - AI helps doctors by provide recommendation but doctor makes final diagnosis Dark red / red = unadvisable Errors can have irreversible, severe impacts like loss of life Needs manual human operation Humans may become complacent or fatigue, leading to erros when interacting with AI Eg. Automated large volume trading or missiles launches - Small error in human judgement can lead to catastrophic consequences AI 3 EC Recommendations on AI governance 4 Ethical Principles: - Respect for human autonomy o AI should not limit human freedom o Should enhance human skills and abilities o Human oversight may be needed - Prevention of harm o AI must be safe and protect vulnerable ppl o Harm incl both phy and mental harm o AI shld consider environment and all living beings - Fairness o AI should ensure benefits and costs are fairly distributed o Should improve fairness, even if current situation isn’t fair o Shld not deceive or harm ppl unjustly o Shld be able to challenge unfair decisions - Explicability o AI shld be clear abt its purpose and capabilities o Shld be understandable to those affects 7 requirements for AI system implementations: - Human agency and oversight o respect human rights and allow human control - Technical robustness and safety o Secure, accurate and reliable - Privacy and data governance o Protect privacy and ensure data quality - Transparency o Clear, explainable and easy to unds - Diversity, non-discrimination and fairness o Avoid bias, be accessible and involve everyone - Societal and environmental well-being o Support sustainability, society and democracy - Accountability o Transparent, reduce harm and offer solutions for problem Realising AI Technical methods - Build AI systems with trust and reliability in mind - Incl ethics and legal rules in design - Make AI decisions explainable - Test and check AI systems for accuracy - Track and measure system performance Non-technical methods - Establish rules and regulations for safe AI use - Follow codes of conduct and commitments - Set standards and certify AI systems - Use governance frameworks for accountability - Educate ppl abt AI - Involve diverse teams in AI design - Engage stakeholders in discussions and decisions Data & AI governance - AI systems need large datasets for training and testing - Proper data management ensures quality and security - Good policies help prevent errors like bias or misclassification - Accountability in data use supports effective AI governance Data Accountability Practices Data Lineage - Tracks origin, processing and movement of data - Backward: traces data from its use back to the source - Forward: follows data from source to its use - Endtoend: combines both views for a full pic - Data provenance record: keeps a trace of data origins and quality - Sometimes data sources cant be tracked or controlled, making it hard to manage quality - Organisations can reduce risks by: o Controlling data early on o Monitoring key data metrics to act quickly Data Quality Factors affecting: - Accuracy: How true data is to what it describes - Completeness: How much of needed data is included - Veracity: whether data comes from trusted source - Timeliness: how up-to-date date is - Relevance: how useful data is for its intended purpose - Integrity: how well data been combined and processes - Usability: whether data is in machine-readable format - Human intervention: whether humans have edited or labelled the data Data Bias Selection bias: data doesn’t represent the full pic (only incl one race) Measurement bias: data is influenced by a device that favours certain results Training, testing and validation - Use separate data for training, testing and validation to reduce bias and overfitting - If data is limited, apply techniques to mitigate risk based on the AI system Updating - Regular check datasets for accuracy, quality and relevance - Add new data to improve AI models - New data from AI systems can reinforce existing biases