Introduction to Asset Pricing PDF - Lancaster University
Document Details
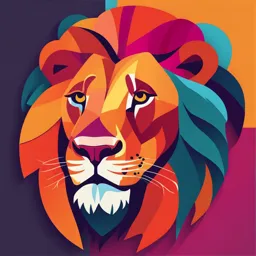
Uploaded by ImmenseLogarithm6297
Lancaster University
2021
Ally Zhang
Tags
Related
Summary
This document contains lecture notes on Introduction to Asset Pricing, presented at Lancaster University in 2021. The topics cover mean-variance portfolio theory, including expected returns, variance, diversification, and the efficient frontier. Relevant formulas and graphical representations are included.
Full Transcript
Introduction to Asset Pricing Part 2: Mean-Variance Portfolio Theory Lecture 1 Ally Zhang Lancaster University Management School Outline The mean-variance concept has provided the dominant framework for understanding security markets and investment...
Introduction to Asset Pricing Part 2: Mean-Variance Portfolio Theory Lecture 1 Ally Zhang Lancaster University Management School Outline The mean-variance concept has provided the dominant framework for understanding security markets and investment management since Markowitz (1952). It introduced the notion of mean-variance efficiency for a portfolio having the highest mean return for all portfolios with the same return variance. Refined by Tobin (1958), to include a risk-free security, also to prove several separation theorems and to add the equilibrium analysis leading to the CAPM. 2021 2/∞ Outline Definitions of expected returns, variances and covariances, How the variance of portfolio returns can be calculated, Benefits of forming diversified portfolios, How efficient portfolios can be formed, Shape of the efficient frontier, Discuss the difficulties of implementing mean-variance analysis. 2021 3/∞ Portfolios Denote by xi the proportion of invested wealth an individual has placed in asset i (stocks, bonds or the risk-free security (a bank account)) Fully invested portfolios; – Are investments where the sum of weights must be one Pn → i=1 xi = 1 Zero investment portfolios; – Are those where long positions in some assets are funded by Pn short-sales of others → i=1 xi = 0 In general, xi will be allowed to take any value, although in practice many investors face constraints on their investment choices (e.g. no short sales). 2021 4/∞ Portfolios Portfolio Returns Assume our individual has invested in N securities. Denote the (ex-ante uncertain) return on asset i by R̃i. Then the portfolio return is N X R̃x = xi R̃i = R̃0 x i=1 where R̃ is the (N × 1) column vector of portfolio returns and x is the (N × 1) column vector of portfolio weights. Ex-ante, the expected return of the portfolio will be given by; N X E[R̃x ] = xi E[R̃i ] = e0 x i=1 where e is the vector of expected returns. 2021 5/∞ Portfolios Using the same notation, variance of the portfolio return is "N # N X X X 2 V[R̃x ] = V xi R̃i = xi V[R̃i ] + xi xj COV(R̃i , R̃j ) i=1 i=1 i6=j In matrix notation, if Σ is the variance-covariance matrix of security returns we have; V[R̃x ] = x0 Σx where Σ has COV(R̃i , R̃j ) as its [i, j] element (and thus V[R̃j ] as its [j, j] element). → combining several securities into a portfolio will reduce return variance unless the securities in question have perfectly (positively) correlated returns. 2021 6/∞ Portfolios Portfolio return covariances If we introduce a second portfolio with weights yi , then the covariance between its return (R̃y ) and that of our original portfolio is given by XN N X N X X N COV(R̃x , R̃y ) = COV xi R̃i , yj R̃j = xi yj COV R̃i , R̃j i=1 j=1 i=1 j=1 If the vector of weights for our second portfolio is denoted by y, then the covariance can be written in matrix form as COV(R̃x , R̃y ) = x0 Σy = y 0 Σx 2021 7/∞ Portfolios Portfolios and variance reduction We concentrate only on the portfolio return variance. Let’s start with the analysis of a 2 asset case. The standard deviation of the return on a 2 asset portfolio is q σx = x21 σ12 + 2ρx1 x2 σ1 σ2 + x22 σ22 where σi is the return standard deviation of security i and COV(X, Y ) = ρσx σy with ρ the correlation between X and Y. Assume we take 2 securities, each with return standard deviation 10%. For a given level of ρ, let’s see how the portfolio return variance changes as we vary x1 , and ρ. 2021 8/∞ Portfolios Diversification: 2 asset case Note: in all cases except that in which the securities are positively perfectly correlated, portfolio return standard deviation is lower than 10%. 2021 9/∞ Portfolios When we have many assets we can also show that portfolio return variance drops: this is called diversification. Assume: An N asset portfolio where the assets are equally weighted Portfolio return variance is XN X 2 V[R̃x ] = xi V[R̃i ] + xi xj COV(R̃i , R̃j ) i=1 i6=j N 1 X 1 X = V[R̃i ] + 2 COV(R̃i , R̃j ) N 2 i=1 N i6=j If V̄ is the average variance of the individual security returns and C̄ is their average covariance then we can rewrite the above as 1 (N − 1) 1 V[R̃x ] = V̄ + C̄ = C̄ + (V̄ − C̄) N N N 2021 10/∞ Portfolios As the number of securities in the portfolio increases, the portfolio return variance tends to the mean security covariance. We call this component of portfolio return variance, un-diversifiable risk. lim V[R̃x ] = C̄ N →∞ The other component of portfolio return variance is called diversifiable risk. It is diversifiable as it can be eliminated by increasing the number of assets in the portfolio. You do not need many assets to largely eliminate diversifiable risk. 2021 11/∞ Portfolios Equally-weighted portfolio return variance versus number of assets You do not need many assets to largely eliminate diversifiable risk 2021 12/∞ Mean-variance expected utility From now on, we work with preferences for our investors such that expected utility is assumed to Increase the expected portfolio return Decrease with portfolio return variance These are commonly called mean-variance preferences. What are the foundations of such preferences? The mean-variance representation is appropriate if EITHER utility is quadratic OR individual security returns are normally distributed. It is probably safest not to presume that either of the preceding assumptions are valid and to view quadratic utility as an approximation to investors true preferences, which we use for tractability 2021 13/∞ Mean-variance expected utility Quadratic utility Denote the investors’ end of period (random) wealth by Ỹ. The Taylor expansion for U (Ỹ ) around E[Ỹ ] is: 1 00 U (Ỹ ) = U (E[Ỹ ]) + U 0 (E[Ỹ ])(Ỹ − E[Ỹ ]) + U (E[Ỹ ])(Ỹ − E[Ỹ ])2 + R3 2 because E[Ỹ ] is certain. Taking expected values on both sides: 1 00 E[U (Ỹ )] = U (E[Ỹ ]) + U 0 (E[Ỹ ])(E[Ỹ ] − E[Ỹ ]) + U (E[Ỹ ])E[(Ỹ − E[Ỹ ])2 ] + E[R3 ] 2 1 00 = U (E[Ỹ ]) + U (E[Ỹ ])σỸ2 + E[R3 ] 2 2021 14/∞ Mean-variance expected utility If U (Y ) is quadratic then U 00 is a constant and E[R3 ] = 0. Hence, E[U (Ỹ )] is a function of E[Ỹ ] and σỸ2 If U (Y ) is not a quadratic function we can still assume that E[R3 ] ' 0 and use the Taylor expansion of second order as an approximation for E[Ỹ ] P∞ (j) Note: R3 = 1 j=3 j! U (Ỹ − E[Ỹ ])j 2021 15/∞ Mean-variance expected utility If individual security returns are Gaussian then: P R̃x = i xi R̃i is also Gaussian If the mean return on the portfolio is µx and its standard deviation is σx. R̃x = µx + σx Z where Z ∼ N (0, 1) Finally, if f (z) is the density of our standard normal we have +∞ Z E[U (R̃x )] = U (µx + σx Z)f (Z)dZ −∞ ,→ Expected utility depends only on mean portfolio returns and portfolio return standard deviation. ,→ Is the assumption of Gaussianity tenable? Empirical evidence suggests that security returns are often non-Gaussian They tend to be negatively skewed and to have fatter tails than a normal distribution. 2021 16/∞ Mean-variance portfolio selection How can frontier portfolios be constructed? Definition: a portfolio is on the mean-variance frontier if it has the smallest return variance of all portfolios with a specified level of expected return. Any investor with mean-variance preferences will clearly choose a portfolio which is a member of this set. Method: Pick a level of portfolio expected return µ̄ Minimize return variance subject to the constraint that mean return is µ̄ 2021 17/∞ Mean-variance portfolio selection Optimisation The problem we wish to solve is: 1 min x0 Σx subject to x0 e = µ̄, x0 1 = 1 x 2 where µ̄ is the level of expected return we have chosen to focus on. This is a simple constrained optimization problem which we solve using the Lagrangian method. The Lagrangian function is 1 0 L = x Σx + λ(µ̄ − x0 e) + θ(1 − x0 1) 2 We have added a factor of 0.5 to the variance term. 2021 18/∞ Mean-variance portfolio selection The first-order conditions of this optimisation problem are dL = Σx − λe − θ1 = 0 (1) dx dL = µ̄ − x0 e = 0 (2) dλ dL = 1 − x0 1 = 0 (3) dθ where 0 denotes a column vector of zeroes. Rearranging (1) in terms of the portfolio weight vector gives x = Σ−1 λe + Σ−1 θ1 (4) We need to get rid of the Lagrange multipliers (λ and θ) from this equation. We do this as follows. First pre-multiply by e0 and use (2) to give µ̄ = λe0 Σ−1 e + θe0 Σ−1 1 2021 19/∞ Mean-variance portfolio selection Then pre-multiply (4) by 10 and use (3) to give 1 = λ10 Σ−1 e + θ10 Σ−1 1 We now have 2 equations which we can solve for the two Lagrange multipliers. We get the following solutions C µ̄ − A B − Aµ̄ λ= θ= D D where A = 10 Σ−1 e B = e0 Σ−1 e C1 = 10 Σ−1 1 D = BC − A2 2021 20/∞ Mean-variance portfolio selection Σ is a variance-covariance matrix, both B and C are guaranteed to be positive and its easy to show that D is positive also. Finally, substituting for λ and θ in (4) we get x∗ = α0 + α1 µ̄ (5) where 1 1 α0 = [BΣ−1 1 − AΣ−1 e] α1 = [CΣ−1 e − AΣ−1 1] D D This expression for the optimal portfolio weights has a particularly simple form. It implies that The optimal portfolio weights are linear in the desired expected return. If you want an expected return of zero, portfolio weights should be α0 If you want an expected return of unity, your weights should be α0 + α1 2021 21/∞ The Portfolio Frontier To obtain the frontier Vary the desired expected return and compute the implied return standard deviation The frontier is the locus traced out in mean-variance space Using equation (5), it is possible to derive the following expression for the variance of the return on a frontier portfolio: 2 C A 1 V̄ = µ̄ − + D C C Features of the frontier: The curve traced out is a parabola in mean/variance space and thus a hyperbola in mean/standard deviation space. The minimum variance portfolio (M ) has: Return variance of C1 and Expected return C A 2021 22/∞ The Portfolio Frontier 3-stock example 2021 23/∞ The Portfolio Frontier Diversification and the efficient set Key fact: Most of the individual securities will lie to the right of the frontier. By combining securities into portfolios we reduce risk → diversification The efficient set: Any investor with mean-variance preferences will hold a portfolio that lies on the frontier. Clearly, our investor will never choose a point on the frontier that lies below (and to the right of) the minimum variance portfolio, M. This is because our investor can always find a portfolio on the frontier but above M which has the same return standard deviation but higher expected return. Investors will only ever hold portfolios on the frontier that lie above the point M - this subset of the frontier is called the efficient set. 2021 24/∞ The Portfolio Frontier Two-fund separation The entire set of frontier portfolios can be derived from any two frontier portfolios. This is easy to see from equation (5) due to its linearity in e. Proof: Assume that we know the frontier portfolios with expected returns e1 and e2. Call the weight vectors corresponding to these expected returns x1 and x2 respectively. We want to derive the weights for a portfolio with expected return e3. As long as e1 is not equal to e2 , there is a λ such that we can express e3 as a linear combination of e1 and e2 : e3 = λe1 + (1 − λ)e2 Via equation (5) and the above we can write x3 = α0 + α1 e3 = α0 + α1 (λe1 + (1 − λ)e2 ) 2021 25/∞ The Portfolio Frontier Obviously, we can write α0 = λα0 + (1 − λ)α0 and so: x3 = λα0 + (1 − λ)α0 + α1 (λe1 + (1 − λ)e2 ) = λ(α0 + α1 e1 ) + (1 − λ)(α0 + α1 e2 ) = λx1 + (1 − λ)x2 Thus, to get the portfolio weights for an expected return of e3 , all we have to do is form a linear combination of x1 and x2 where the coefficient in the combination is that which allows us to derive e3 from e1 and e2. This result is known as two-fund separation. Via this result, if an investor wishes to invest in a specific frontier portfolio, as long as he knows two points on the frontier, he can get to the point he cares about by combining the two portfolios (or funds) with the appropriate coefficient. 2021 26/∞ The Portfolio Frontier M-V analysis: introducing a risk-free asset Finally, we evaluate how the introduction of a risk-free security alters our analysis. Features of the risk-free asset: It delivers a guaranteed return of Rf Its return variance is thus zero. The covariance of its return with any other security return is also zero. 2021 27/∞ The Portfolio Frontier Optimization with the risk-free asset When including a risk-free asset our optimization becomes: 1 0 min x Σx subject to x0 e + (1 − x0 1)Rf = µ̄ x 2 Changes: x is the vector of risky asset weights, while the weight placed on the risk-free security is (1 − x0 1) We have enforced the condition that, when looking across risky and risk-free assets, portfolio weights sum to one. Hence, we lose the explicit constraint that forces the sum of weights to be unity. The expression for portfolio expected return now contains a component attributable to the risk-free asset. 2021 28/∞ The Portfolio Frontier The Lagrangian corresponding to this problem is: 1 0 L= x Σx + λ(µ̄ − x0 e − (1 − x0 1)Rf ) 2 The first-order conditions are dL = Σx − λe + λ1Rf = 0 (6) dx dL = µ̄ − x0 e − (1 − x0 1)Rf = 0 (7) dλ Again, rearrange equation (6) in terms of portfolio weights to yield: x = Σ−1 λ(e − 1Rf ) (8) 2021 29/∞ The Portfolio Frontier Last of all, we need to solve for λ. We substitute equation (8) into the left-hand side of equation (7). This gives an equation in λ and the model parameters e, Σ and Rf. Solving we get: µ̄ − Rf µ̄ − Rf λ= = B − Rf (2A − Rf C) Z where A, B, and C are as defined in the previous problem. Note that Z is a scalar such that our solution for λ is just a multiple of the excess return on the risky portfolio. Substituting for λ in the expression for the optimal portfolio weight we get: µ̄ − Rf x∗ = Σ−1 (e − 1Rf ) (9) Z This gives the vector of optimal investments in the risky securities and implies the optimal investment in the risk-free asset. 2021 30/∞ The Portfolio Frontier Interpretation Consider equation (9) for the optimal weight vector: The term Σ−1 (e − 1Rf ) is a vector µ̄−Rf The term Z is a scalar The expression implies that if we wish to earn a larger expected return, we invest proportionately the same amount more in each risky asset so that their relative proportions are unchanged. As we vary µ̄, then, all we are doing is to invest more or less in a certain portfolio of risky assets: we call this portfolio the tangency portfolio You can see why it is so named from the following slide, which graphs the efficient set with a risk-free asset alongside that in the absence of a risk-free asset. Two-fund separation: the same result holds now, except the most obvious two funds are the tangency portfolio and the risk-free asset. 2021 31/∞ The Portfolio Frontier The efficient set with a risk-free security 2021 32/∞ The Portfolio Frontier Understanding the efficient set Combining the risk-free asset with a risky portfolio: consider an arbitrary portfolio of risky assets, Q. What happens if an investor takes Q and starts to mix it with the risk-free asset? Denote the expected return and return standard deviation of Q with µQ (µQ > Rf ) and σQ respectively. A mixture with weight λ on the risk-free asset has the following properties: µ̃ = λRf + (1 − λ)µQ σ̃ = (1 − λ)σQ Take λ equals unity (i.e. we start with an investment solely in the risk-free asset) and gradually decrease it: Both expected return and standard deviation increase In expected return / standard deviation space, the curve traced out is a straight line (starting at Rf and passing through Q). 2021 33/∞ The Portfolio Frontier Proof: dµ̃ dµ̃/dλ µQ − Rf = = dσ̃ dσ̃/dλ σQ The slope is constant, thus the curve that is traced out must be a straight line. Now: An investor with mean-variance preferences will always choose a portfolio in the efficient set. If we introduce a risk-free security, the efficient set is exactly the straight line that joins the point representing the risk-free rate with the tangency portfolio, T. Any investor can get to any point on this line by forming the appropriate combination of the risk-free security with the tangency portfolio, T : this is two-fund separation again 2021 34/∞ Conclusion In this lecture we have used some simple mathematics, combined with weak restrictions on investors preferences to narrow down the set of portfolios an investor might choose when faced with N risky assets and, later, with a single riskless asset. In both cases; We defined the efficient set of portfolios Showed that the result known as two-fund separation holds. In the next lecture, we will use the foundations we have built here to derive an equilibrium asset pricing model: the famous Capital Asset Pricing Model. 2021 35/∞ Readings T. E. Copeland, J. F. Weston, K. Shastri, Financial Theory and Corporate Policy, 2005/2013 Chapter 5 Bodie, Kane and Markus, Investments and Portfolio Management, McGraw Hill, 9th Edition, 2011 Chapters 6 and 7 Lintner, J. (1965), The Valuation of Risk Assets and the Selection of Risky Investments in Stock Portfolios and Capital Budgets, Review of Economics and Statistics 47, 13-39. Markowitz, H.M. (1952), Portfolio Selection, Journal of Finance 7, 77-91. 2021 36/∞