IMG_3105.jpeg
Document Details
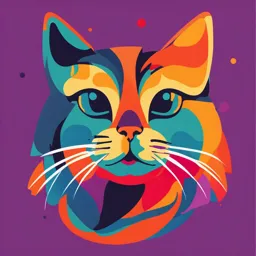
Uploaded by QuieterEarthArt25
University of Surrey
Full Transcript
## Chapter 9: ANOVA - Analysis of Variance ### 9.1 Introduction ANOVA (Analysis of Variance) is a statistical method used to compare the means of two or more groups. It is particularly useful when you want to determine if there are any statistically significant differences between the means of sev...
## Chapter 9: ANOVA - Analysis of Variance ### 9.1 Introduction ANOVA (Analysis of Variance) is a statistical method used to compare the means of two or more groups. It is particularly useful when you want to determine if there are any statistically significant differences between the means of several groups. **Key Concepts:** * **Variance:** A measure of how spread out a set of data is. * **F-statistic:** A test statistic used in ANOVA to determine if the variance between groups is significantly different from the variance within groups. **Purpose of ANOVA:** * To test the null hypothesis that the means of all groups are equal.  $H\_0: \mu\_1 = \mu\_2 =... = \mu\_k$ * To determine if there is a statistically significant difference between at least two of the group means. ### 9.2 One-Way ANOVA One-way ANOVA is used when you have one independent variable (factor) with two or more levels (groups) and one dependent variable. **Assumptions of One-Way ANOVA:** 1. The data is normally distributed for each group. 2. The variances of the groups are equal (homogeneity of variances). 3. The observations are independent. **Steps for Performing One-Way ANOVA:** 1. State the null and alternative hypotheses. 2. Calculate the F-statistic. 3. Determine the degrees of freedom. 4. Find the p-value. 5. Make a decision based on the p-value. **Example:** Suppose you want to compare the test scores of students from three different schools. You would use one-way ANOVA to determine if there is a significant difference in the mean test scores between the schools. ### 9.3 Two-Way ANOVA Two-way ANOVA is used when you have two independent variables (factors) and one dependent variable. It allows you to examine the main effects of each independent variable as well as the interaction effect between them. **Key Terms:** * **Main Effect:** The effect of one independent variable on the dependent variable, ignoring the other independent variable. * **Interaction Effect:** The effect of one independent variable on the dependent variable depends on the level of the other independent variable. **Example:** Suppose you want to study the effects of both diet and exercise on weight loss. You would use two-way ANOVA to determine if there are significant main effects of diet and exercise, as well as an interaction effect between them. ### 9.4 ANOVA Table The ANOVA table summarizes the results of the ANOVA test. It includes the following information: | Source of Variation | Degrees of Freedom (df) | Sum of Squares (SS) | Mean Square (MS) | F-statistic | p-value | | :------------------ | :---------------------- | :------------------ | :--------------- | :---------- | :-------- | | Between Groups | k - 1 | SSB | MSB | F | p | | Within Groups | N - k | SSW | MSW | | | | Total | N - 1 | SST | | | | Where: * k = number of groups * N = total number of observations * SSB = Sum of Squares Between Groups * SSW = Sum of Squares Within Groups * SST = Total Sum of Squares * MSB = Mean Square Between Groups * MSW = Mean Square Within Groups * F = F-statistic * p = p-value ### 9.5 Post-Hoc Tests Post-hoc tests are used after ANOVA to determine which specific groups are significantly different from each other. Common post-hoc tests include: * Tukey's HSD (Honestly Significant Difference) * Bonferroni correction * Scheffe's method **Purpose of Post-Hoc Tests:** * To control for the familywise error rate, which is the probability of making at least one Type I error (false positive) when performing multiple comparisons. ### 9.6 Conclusion ANOVA is a powerful statistical method for comparing the means of two or more groups. It is widely used in various fields, including psychology, education, and medicine. By understanding the principles and assumptions of ANOVA, you can effectively analyze data and draw meaningful conclusions.