Market Size in Innovation: Pharmaceutical Industry PDF
Document Details
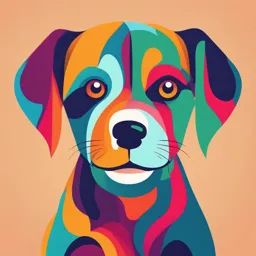
Uploaded by WorldFamousLogic8685
Politecnico di Milano
2003
Daron Acemoglu and Joshua Linn
Tags
Summary
This paper examines the impact of market size on pharmaceutical innovation using data from the U.S. pharmaceutical industry. It finds a strong positive correlation between market size and the number of new drugs, considering both generic and non-generic drugs. The authors use demographic trends as exogenous variation in market size.
Full Transcript
NBER WORKING PAPER SERIES MARKET SIZE IN INNOVATION: THEORY AND EVIDENCE FROM THE PHARMACEUTICAL INDUSTRY Daron Acemoglu...
NBER WORKING PAPER SERIES MARKET SIZE IN INNOVATION: THEORY AND EVIDENCE FROM THE PHARMACEUTICAL INDUSTRY Daron Acemoglu Joshua Linn Working Paper 10038 http://www.nber.org/papers/w10038 NATIONAL BUREAU OF ECONOMIC RESEARCH 1050 Massachusetts Avenue Cambridge, MA 02138 October 2003 We thank David Autor, Ernie Berndt, Olivier Blanchard, Alfonso Gambardella, Amy Finkelstein, Stan Finkelstein, Jerry Hausman, Paul Joskow, Frank Lichtenberg and participants at the NBER Summer Institute and Wharton for useful comments. We are especially grateful to Frank Lichtenberg for generously sharing the Food and Drug Administration drug approval and the Computer Retrieval of Information on Scientific Projects data with us. This research is partially funded by the National Science Foundation and the Russell Sage Foundation. The views expressed herein are those of the authors and not necessarily those of the National Bureau of Economic Research. ©2003 by Daron Acemoglu and Joshua Linn. All rights reserved. Short sections of text, not to exceed two paragraphs, may be quoted without explicit permission provided that full credit, including © notice, is given to the source. Market Size in Innovation: Theory and Evidence From the Pharmaceutical Industry Daron Acemoglu and Joshua Linn NBER Working Paper No. 10038 October 2003 JEL No. O31, O33, L65 ABSTRACT This paper investigates the effect of (potential) market size on entry of new drugs and pharmaceutical innovation. Focusing on exogenous changes driven by U.S. demographic trends, we find that a 1 percent increase in the potential market size for a drug category leads to a 4 to 6 percent increase in the number of new drugs in that category. This response comes from both the entry of generic drugs and new non-generic drugs, and is generally robust to controlling for a variety of non- profit factors, pre-existing trends, and changes in health care coverage. Daron Acemoglu Department of Economics Massachusetts Institute of Technology Cambridge, MA 02142 and NBER daron@mit.edu Joshua Linn Department of Economics Massachusetts Institute of Technology Cambridge, MA 02142 jlinn@mit.edu 1 Introduction This paper constructs a simple model linking innovation rates to current and future market size, and provides evidence from the pharmaceutical industry to support this hypothesis. Our empirical work, which exploits changes in the market size for various drug categories driven by U.S. demographic trends, finds economically significant and relatively robust effects of market size on entry of new drugs. Although many historical accounts of important innovations focus on the autonomous progress of science and on major breakthroughs that take place as scientists build on each other’s work,1 economists typically emphasize profit incentives and the size of the target mar- ket. For example, in his seminal study, Invention and Economic Growth, Schmookler argued that: “...invention is largely an economic activity which, like other economic activities, is pur- sued for gain” (1966, p. 206). To emphasize the role of market size, Schmookler entitled two of his chapters “The amount of invention is governed by the extent of the market.” The role of profit incentives and market size in innovation is also important both for the recent endogenous technological change models, which make profit incentives the central driving force of the pace of aggregate technological progress (e.g., Aghion and Howitt, 1992, Grossman and Helpman, 1991, Romer, 1990), and for the induced innovation and directed technical change literatures, which investigate the influence of profit incentives on the types and biases of new technologies (see, for example, Kennedy, 1964, Drandkis and Phelps, 1965, Samuelson, 1965, Hayami and Ruttan, 1970, and Acemoglu, 1998, 2002, and 2003). A recent series of papers by Kremer, for example (2002), also build on the notion that pharmaceutical research is driven by market size and argue that there is generally insufficient research to develop cures for third-world diseases such as malaria. In this paper, we investigate the effects of market size for different types of drugs on entry of new drugs and innovation. A major difficulty in any investigation of the impact of market size on innovation is the endogeneity of market size–better products will have bigger markets. Our strategy to overcome this problem is to exploit variations in market size driven by demo- graphic changes (or past demographic trends), which should be exogenous to other, for example scientific, determinants of innovation and entry of new drugs.2 We create the potential market 1 See, for example, Ceruzzi (2000), Rosenberg (1974) and Scherer (1984). Ceruzzi emphasizes the importance of a number of notable scientific discoveries and the role played by certain talented individuals in the development of modern computing. He points out that important developments took place despite the belief of many important figures in the development of the computer that there would not be a market greater than a handful of personal computers in the United States (2000, p. 13). 2 For many drugs non-U.S. markets may also be relevant. Nevertheless, the U.S. market is disproportionately important, constituting about 40 percent of the world market (IMS, 2000). Below we also look at the impact of West European and Japanese demographic changes on the entry rates of new drugs. 1 size for various drug categories according to the age distribution of their users at a given point in time, and then trace changes in potential market size driven by changes in demographics (holding the age profile of consumption of various drug categories constant over time).3 We measure entry and innovation using the Food and Drug Administration’s (FDA) approval of new drugs.4 Our results show that there is an economically and statistically significant response of the introduction of new drugs to market size. For example, a 1 percent increase in the size of the potential market for a drug leads to a 4 to 6 percent increase in the number of new drugs. We check the robustness of our results by controlling for lagged FDA approvals, pre-existing trends, differences in the non-economic incentives to innovate, advances in biotechnology and changes in insurance coverage of drug expenditures. New drugs that enter the market comprise both generics and non-generics, and we find that both respond to market size, though the response of generics is larger and somewhat more precisely estimated. We also investigate whether it is the current market size or past or future market sizes that have the largest effect on entry of new drugs. On the one hand, because changes in demographics are known in advance, drug entry may respond to anticipated future market size. On the other hand, because the development process of new drugs can be long, entry may respond to past market size. We find that current market size and 5-10 year leads of market size have the strongest effect on entry rates of new drugs, which is consistent with the predictions of the theoretical model we use to motivate our empirical investigation. Finally, we also look at the response of pharmaceutical patents to market size, and find a positive, but statistically insignificant relationship between future anticipated market size and patents, which might reflect the difficulty of matching patents to drug categories. There are a number of other studies related to our work. First, Schmookler (1966) docu- ments a statistical association between investments and sales, on the one hand, and patents and innovation, on the other, and argues that the causality ran largely from the former to the latter. The classic study by Griliches (1957) on the spread of hybrid seed corn in U.S. agriculture also provides evidence consistent with the view that technological change and technology adoption are closely linked to profitability and market size. Pakes and Schankerman (1984) investigate this issue using a more structural approach, linking R&D intensity at the industry level to factor demands and to growth of output. In more recent research, Scott Morton (1999) and 3 Loosely speaking, “market size” corresponds to the number of users times their marginal willingness to pay. Therefore, market size can increase both because the number of users increases and because of their marginal willingness to pay changes. We focus on changes driven by demographics to isolate exogenous changes in market size. 4 These data were previously used by Lichtenberg and Virahbak (2002), who obtained them under the Freedom of Information Act. We thank Frank Lichtenberg for sharing these data with us. 2 Reiffen and Ward (2002) study the decision of firms to introduce a new generic drug and find a positive relationship between entry into a new market and expected revenues in the target market. None of these studies exploit a potentially exogenous source of variation in market size, however. Second, some recent research has investigated the response of innovation to changes in energy prices. Most notably, Newell, Jaffee and Stavins (1999) show that between 1960 and 1980, the typical air-conditioner sold at Sears became significantly cheaper, but not much more energy-efficient. On the other hand, between 1980 and 1990, there was little change in costs, but air-conditioners became much more energy-efficient, which, they argue, was a response to higher energy prices. In a related study, Popp (2002) finds a strong correlation between aggregate patents and energy prices. These findings are consistent with the hypothesis that the type of innovation responds to profit incentives, though they do not establish causality. Third, there is substantial research focusing on innovation in the pharmaceutical industry. Henderson and Cockburn (1996), Cockburn and Henderson (2001), and Danzon, Nichelson and Sousa Pereira (2003) study the determinants of success in clinical trials, focusing mainly on firm and project size. Galambos and Sturchio (1998), Cockburn, Henderson and Stern (1999), Gambardella (2000), and Malerba and Orsenigo (2000) discuss various aspects of the recent technological developments in the pharmaceutical industry. Ling, Berndt and Frank (2003) investigate the complementarity between new technologies and the skills of physicians in the spread of new drugs. Most closely related to this study are Lichtenberg and Waldfogel (2003) and Finkelstein (2003). Lichtenberg and Waldfogel show that following the Orphan Drug Act there were larger declines in mortality among individuals with rare diseases (compared to other diseases) because of the incentives created by the Act to develop drugs for these rare diseases. Finkelstein exploits three different policy changes affecting the reimbursement of costs of vaccination against 6 infectious diseases: the 1991 policy change that all infants be vaccinated against hepatitis B, the 1993 decision of Medicare to cover the costs of influenza vaccinations, and the 1986 introduction of funds to insure vaccine manufactures against product liability lawsuits for vaccines against polio, diphteria, tetanus, measles, mumps, rubella, or pertussis. She finds that increases in vaccine profitability resulting from these policy changes are associated with a significant increase in the number of clinical trials to develop new vaccines against the relevant diseases.5 The rest of the paper is organized as follows. We outline a simple model linking innovation to market size in the next section. Section 3 briefly explains our empirical strategy, and Section 5 Lichtenberg (2003) also presents evidence suggesting that the types of new drugs changed towards drugs more useful for the elderly after Medicare was established. 3 4 describes the basic data sources and the construction of the key variables. Section 5 presents the empirical results and a variety of robustness checks. Section 6 contains some concluding remarks, while the Appendix gives further data details. 2 Theory We now outline a simple framework to analyze the influence of market size on innovation. Subsection 2.1 discusses the basic model. Subsection 2.2 derives the implications of potential delays in the development and approval processes of new drugs. Subsection 2.3 generalizes the basic model to investigate the response of innovation effort and R&D to anticipated changes in future market size. Finally subsection 2.4 extends the model to introduce an explicit distinction between entry of generic drugs and non-generic drugs. 2.1 Basic Model Consider an economy consisting of a set I individuals, each denoted by i. Time is continuous t ∈ [0, ∞), and all individuals are infinitely lived. There are two types of goods in this economy. First, a basic good, y, which can be consumed or used for the production of other goods, or for research expenditure. Individual i has an exogenously given endowment yi (t) at time t. Second, there is a large number J of drugs, x1 ,...., xJ. Each drug has a potentially time- varying “quality”, q1 (t),...., qJ (t). Each individual demands only one type of drug. Hence, we partition the set I of individuals into J disjoint groups, G1 ,...,GJ with G1 ∪ G2 ∪... ∪ GJ = I, such that if i ∈ Gj , then individual i demands drug j. More specifically, if i ∈ Gj , then his preferences are given by: Z ∞ £ ¤ exp (−rt) ci (t)1−γ (qj (t) xji (t))γ dt, (1) 0 where r is the discount rate of the consumers (also the interest rate in the economy), γ ∈ (0, 1), ci (t) is the consumption of individual i of the basic good at time t, and xji (t) is the consumption of individual i of drug j.6 This Cobb-Douglas functional form, which implies an elasticity of substitution equal to 1 between the basic good and drugs, and the assumption that each 6 Alternatively, suppose the preferences of individual i are: Z ∞ 1−γ exp (−ω (t, qj (t) xji (t))) ci (t) dt, 0 where ω (t, qj (t) xji (t)) is the discount rate, which is influenced by the quality and quantity of drugs that the individual consumes (e.g., because they extend his life or affect its quality). If we assume that ω (t, qj (t) xji (t)) = rt − γ ln (qj (t) xji (t)), we obtain the expression in (1) in the text. 4 individual only consumes one type of drug are for simplicity and do not affect the main results.7 Normalizing the price of the basic good to 1 in all periods, and denoting the price of drug j at time t by pj (t), individual demands for drugs are given by ½ γyi (t) for i ∈ Gj xij (t) = pj (t) (2) 0 for i ∈ / Gj , for all i ∈ I and for all j = 1,..., J. At any point in time, there is one firm with the best-practice technology for producing each type of drug. The best-practice firm in drug line j can produce one unit of drug with quality qj (t) using one unit of the basic good. If there is an innovation for drug line j currently with quality qj (t), this leads to the discovery of a new drug of quality λqj (t) where λ > 1. For the purposes of the model, we think that any new innovation is approved (for example by the FDA) and can be sold to consumers immediately (and is under patent protection indefinitely). We start with a very simple formulation of the R&D technology whereby one unit of the final good devoted to R&D for drug line j leads to a flow rate of δ j > 0 of discovering a new drug of this type. Equivalently, if total R&D effort at time t is zj (t), the flow rate of innovation (the rate of entry of new drugs) for this line of drugs is nj (t) = δ j zj (t). (3) Differences in δ j ’s introduce the possibility that technological progress is scientifically more difficult in some lines than others, which is the effect emphasized by science-driven theories of innovation discussed in the Introduction. The most important feature of this R&D technology for our focus here is that technological progress is directed in the sense that firms can devote their research effort and expenditure to developing particular types of drugs. This contrasts with a different model where firms invest in R&D in an undirected way, and discover new versions of any one of a set of drugs. The pharmaceutical industry, especially in recent past, is a prime example of an industry where companies with fairly sophisticated R&D divisions or specialized R&D firms can undertake research for specific drug lines (e.g., Gambardella, 2000, Malerba and Orsenigo, 2000).8 7 One implication of the Cobb-Douglas functional form is that the share of income that an individual spends on medicine is constant. This implication can be easily relaxed by considering a utility function with an elasticity of substitution different from 1, as in the factor market models with directed technical change (see, for example, Acemoglu, 1998, 2002). It is also straightforward to extend the model so that each individual demands potentially more than one type of drug, though this would require additional notation. 8 Naturally, there exist examples of research directed at a specific drug type leading to the discovery of a different product, such as the well-known example of Viagra, which resulted from research on hypertension and angina, and was partly accidentally discovered from the detection of side effects in a clinical study (see, e.g., Kling, 1998). 5 The demand curves in (2) have an elasticity equal to 1, so an unconstrained monopolist would like to charge an arbitrarily high price. However, the firm with the best drug in line j is competing with the next best drug in that line. Consider such a firm with quality qj (t) charging price pj (t). If this price is arbitrarily high, the next best quality could supply to the market and make positive profits, driving the best technology to zero profits. Therefore, the firm with the best drug has to set a limit price to exclude the next best firm–i.e., to ensure that consumers are happy to buy from it rather than buy from the next best firm even if the next best firm charges the lowest possible price, i.e., equal to its marginal cost, 1. Suppose that a consumer buys from the best firm with quality qj (t) and price pj (t) and chooses her optimal consumption as given by (2), then her instantaneous utility at time t will be: (qj (t))γ (1 − γ)1−γ γ γ (pj (t))−γ yi (t) , and if she purchases from the next best firm, which, by definition, has quality qj (t) /λ and charges price equal to marginal cost, 1, she will have utility: λ−γ (qj (t))γ (1 − γ)1−γ γ γ yi (t). The limit price equalizes these two expressions, hence, equilibrium prices for all j and t satisfy: pj (t) = λ. (4) The profits of the firm with the best product of quality qj (t) in line j at time t are: X πj (qj (t)) = (λ − 1) γ yi (t) (5) i∈Gj = (λ − 1) γYj (t) P where the second line defines Yj (t) ≡ i∈Gj yi (t) as the total income of the group of consumers demanding drug j. Here λγYj (t) corresponds to the market size (total sales) for drug j. Throughout we assume that all Yj (t)’s are known in advance, which is plausible in the context of demographics-driven changes in demand. They can change because the number of consumers demanding this product changes, or because their incomes change, or also because new varieties of drugs steal consumers from this particular drug. Notice that profits of drug companies are independent from quality, qj (t), which is a feature of the Cobb-Douglas utility function. Firms are forward-looking, and discount future profits at the rate r. The discounted value of profits for firms can be written by a standard dynamic programming recursion. Vj (t | qj ), the value of a firm that owns the most advanced drug of quality qj in line j at time t, is:9 rVj (t | qj ) − V̇j (t | qj ) = π j (qj ) − δ j zj (t | qj ) Vj (t | qj ) (6) 9 Throughout, we assume that the relevant transversality conditions hold and discounted values are finite. 6 for each j = 1, 2,..., J, where πj (qj ) is the flow profits in drug line j given by (5), and zj (t | qj ) is equilibrium R&D effort at time t on this line by other firms when current technology is qj (because of the standard replacement effect first emphasized by Arrow, 1963, the firm with the best technology does not undertake any R&D itself, see, for example, Aghion and Howitt, 1992). To simplify notation, we will typically use zj (t) instead of zj (t | qj ). Intuitively, the flow value of owning the best technology in line j, rVj (t | qj ), is equal to the flow profits, π j (qj ) plus the potential appreciation of the value, V̇j (t | qj ), but also takes into account that at the flow rate nj (t) = δ j zj (t) there will be a new innovation, thus the current firm will lose its best-practice status, and make zero profits thereafter. Note that entry of new drugs that are not technologically superior, but steal customers from the incumbent, such as the entry of generics, can also be included in zj (t), especially since equation (5) shows that the profits of a new entrant are independent of the quality of its product, as long as it is sufficient to take over (part of) the market. Thus for now, we think of zj (t) and nj (t) as corresponding to the entry of both generic and non-generic drugs, and in subsection 2.4, we present a model with separate entry rates of generics and non-generics. There is free entry into R&D to develop better quality drugs. Therefore, if there is positive research for some drug line j = 1, 2,..., J at time t, then the free entry condition ensuring zero profits must hold. In other words, if zj (t) > 0, then δ j Vj (t | qj ) = 1. (7) Alternatively, we might have zj (t) = 0, and δ j Vj (t | qj ) ≤ 1, in which case research is not profitable, and in equilibrium, there will be no innovation. An equilibrium in this economy is sequences of prices pj (t)|j=1,..J that satisfy (4), consumer demands for drugs xi (t)|i∈I that satisfy (2) and sequences of R&D levels zj (t)|j=1,..J that satisfy (7) with Vj (·) given by (6). An equilibrium is straightforward to characterize. Differentiating equation (7) with respect to time implies that we must always have V̇j (t | qj ) = 0 for each j = 1, 2,..., J as long as zj (t) > 0. Substituting this equation and (7) into (6) yields the levels of R&D effort in the unique equilibrium: ½ ¾ δ j (λ − 1) γYj (t) − r zj (t) = max ;0 , (8) δj for each j = 1, 2,..., J, and for all t. From now on, unless otherwise stated, we assume that Yj (t)’s are such that all equilibrium research levels are strictly positive, i.e., zj (t) > 0 for all j and t, so that zj (t) = (δ j (λ − 1) γYj (t) − r) /δj , and we will often drop the max operator. 7 The most important feature of (8) is that it highlights the market size effect in innovation, which is the main focus of this paper. The greater is the market size for a particular drug, the more profitable it is to be the supplier of that drug, and consequently, there will be greater research effort to acquire this position. Our empirical work below investigates the strength of this effect in the pharmaceutical industry over recent decades. In addition, naturally, a higher productivity of R&D as captured by δ j also increases R&D, and a higher interest rate reduces R&D since current R&D expenditures are rewarded by future revenues. Another important implication of this equation is that there are no transitional dynamics. At any point in time, the amount of effort devoted to developing a particular drug line is determined by the current market size. Past market sizes and anticipated future market sizes do not affect current research effort. This is an implication of the linear R&D technology, which ensures that whenever there are profit opportunities, there will immediately be sufficient R&D to arbitrage them, thus ensuring V̇j (t | qj ) = 0. The intuition for the lack of response to anticipated changes in future market size here highlights an important effect in quality ladder models of technological progress: with a greater market size in the future, firms would like to own the best-practice product at the time when the market size has actually become larger. Investing in R&D in advance could be useful to the extent that it achieves this objective. However, it is not beneficial to invest in R&D too much in advance, since some other firm would improve over this innovation by the time the new and larger market size materializes. With the linear model here, zj can change discontinuously, so investing even a little bit in advance of the actual increase in the size of the market is not profitable.10 Combining equations (3) and (8) gives entry of new drugs as (ignoring the max operator): nj (t) = δ j (λ − 1) γYj (t) − r. (9) This equation relates innovation or entry of new products, which we will approximate with FDA approval of new drugs, to market size (total expenditure of consumers in this line of drug). In addition, this equation also encapsulates the alternative view of the determinants of innovation, which maintains that cross-drug distribution of R&D is determined largely by technological research opportunities or perhaps by other non-profit related motives. If there are large and potentially time-varying differences in δ j ’s, then these may be the primary factor determining variations in R&D across drug lines, and market size may have only a small effect. Whether or not this is so is an empirical question. 10 In practice, companies may also have an incentive not to market their discoveries before the market size increases in order to prevent competitors from leapfrogging their new product. 8 2.2 Delays in Development and Approval The baseline model ignores the potential delays in the process of development and approval of new drugs (for example, DiMasi et al., 1991, report that the eventual marketing of a drug may be as much as 15 years after the beginning of initial research). To incorporate such delays in the simplest possible way, suppose that it takes an interval of length T after the research decision for the drug to be developed, gain approval, and enter the market. Therefore, we now have nj (t) = δ j zj (t − T ), where zj (t − T ) is the rate of innovation at time t − T. Given this structure, the key value function changes to: rVj (t | qj ) − V̇j (t | qj ) = πj (qj ) − δ j zj (t − T ) Vj (t | qj ). (10) Equation (10) is a delayed differential equation rather than an ordinary differential equation, so a general analysis is more difficult. Nevertheless, the unique equilibrium in this case is still easy to characterize because of the simple structure here. Now the free entry condition is: if zj (t − T ) > 0, then exp (−rT ) δj Vj (t | qj ) = 1, (11) which recognizes that innovation effort at time t − T will lead to revenues at time t, hence the discounting for the interval of length T. Equations (10) and (11) together imply that: ½ ¾ exp (−rT ) δ j (λ − 1) γYj (t) − r zj (t − T ) = max ;0 , (12) δj which is very similar to (8), except for the term exp (−rT ). This term takes into account that because of the development and approval delays, costs of R&D are incurred before the benefits accrue. This equation also makes it clear that longer development and approval delays discourage innovation. Equation (12) may give the impression that there should now be a response of innovation to future market sizes. This is not the case, however, since what we measure in the data is not the actual R&D expenditure, but entry of new drugs. Since nj (t) = δ j zj (t − T ), the key prediction of the model for entry of new drugs changes to: nj (t) = exp (−rT ) δ j (λ − 1) γYj (t) − r, which only differs from (9) by the term exp (−rT ). This analysis therefore shows that delays in development and approval processes do not change the basic predictions relating to entry of new drugs, though the predictions about the timing of R&D and patenting are different (and this is useful to bear in mind when we look at patents below). 9 2.3 Anticipation Effects We now generalize this basic setup to obtain a reaction to (anticipated) future market sizes. We change the baseline model in one dimension: we assume that one unit of final good spent for R&D in line j leads to the discovery of a better drug at the flow rate δ j zj φ (zj ), where zj is the aggregate research effort devoted to the discovery of a new drug in this line. We also assume that φ0 (z) ≤ 0 for all z, which implies that greater research effort runs into decreasing returns within a given period (there are constant returns to scale when φ (z) = 1 for all z), but throughout zφ (z) is strictly increasing in z, so that greater aggregate research effort always leads to faster innovation in the aggregate. Finally, free entry into R&D for all lines implies that any new firm can enter taking zj (t) as given, thus without taking into account the reduction that its entry causes in the innovation rates of other firms.11 Given this specification, the value function changes to: rVj (t | qj ) − V̇j (t | qj ) = π j (qj ) − δ j zj (t) φ (zj (t)) Vj (t | qj ) , (13) for each j = 1, 2,..., J, which only differs from (6) because the flow rate of innovation is now δ j zj (t) φ (zj (t)) rather than δ j zj (t). Since each potential entrant takes the aggregate research effort in each line of drug as given, it anticipates that one unit of the basic good spent for R&D in drug line j will lead to an innovation at the flow rate δ j φ (zj (t)). Thus, the free entry condition is δ j φ (zj (t)) Vj (t | qj ) = 1, (14) for each j = 1, 2,..., J (again as long as zj (t) > 0). An equilibrium is defined similarly to before, except that now the sequence of R&D levels zj (t)|j=1,..J have to satisfy (14) instead of (7) with Vj (·) given by (13). To make further progress in this case, let us assume that Yj (t) = Yj for all t, so that market sizes remain constant over time. Differentiating (14) with respect to time, and substituting into (13) and rearranging, we obtain J equilibrium differential equations in zj (t)’s: żj (t) 1 = [r + δ j zj (t) φ (zj (t)) − δ j φ (zj (t)) (λ − 1) γYj ] , (15) zj (t) εφ (zj (t)) where εφ (zj (t)) = −φ0 (zj (t)) zj (t) /φ (zj (t)) is the elasticity of the φ function. 11 This is the natural assumption given free entry. The alternative would be to assume that there is a consortium of £firms in each line, jointly maximizing profits. ¤ In this case, the free entry condition below would change to: δ j φ (zj (qj (t))) + zj (qj (t)) φ0 (zj (qj (t))) Vj (qj (t)) = 1. This does not affect any of the results of the analysis. 10 Since consumer incomes are now assumed to be constant, the steady-state equilibrium must have żj (t) = 0. This implies that the steady-state R&D level in drug line j = 1, 2,..., J is: ¡ S¢ δ j φ zj (λ − 1) γYj − r zjS = ¡ ¢. (16) δ j φ zjS In the special case where φ (.) = 1, this equation boils down to (8) in the basic model. Moreover, the steady-state R&D levels have the same features as the equilibrium there: a greater market size increases R&D, a greater δj , which corresponds to better research opportunities for this drug line, increases R&D, and higher interest rates reduce R&D. An important feature of this equation is that the equilibrium behavior of zj (t) is inde- pendent of research and profitability in other drug lines. This feature simplifies the dynamics substantially. In addition, the right hand side of (15) is strictly increasing in zj (t) whenever zj (t) = zjS , which implies that there can be at most one intersection of the right hand side with the 0 axis, and at this point of intersection, żj (t) /zj (t) is increasing in zj (t), as drawn in Figure 1. Therefore, (15) defines an unstable differential equation. This implies that starting away from the steady-state, the equilibrium zj (t) has to immediately jump to its steady-state value as given by (16). Hence, there are no transitional dynamics in this extended model either. However, there is now an equilibrium response to anticipated future changes in market size. Consider the following situation: it is suddenly announced at date t0 that Yj will increase to Ŷj in some future date t̂ > t0. How will this fully-anticipated change in market size affect equilibrium R&D? Suppose there is no change in zj (t) until t̂. This implies that zj (t) has to jump up discontinuously at t = t̂. But this implies that anticipating this jump, Vj (t | qj ) will be changing before t̂, in particular, V̇j (t | qj ) < 0. Since zj (t) is constant, this would violate the free entry condition, (14). This reasoning implies that there should be no anticipated jumps in zj (t), in particular no jump at t = t̂, which is only possible if zj (t) jumps by a small amount initially at t = t0 , and then smoothly increases towards the new steady-state equilibrium. Therefore, in this model there are no transitional dynamics starting away from the steady-state, but R&D responds to anticipated future market size changes. Nevertheless, the same considerations as in the baseline model limit this response. In other words, even if a change in market size is anticipated far in advance, increasing R&D investment too far in advance would not be profitable because another firm is likely to innovate further before the actual increase in market size materializes. In terms of our empirical work, even if demographic changes are anticipated at least 20 or 30 years in advance, we may expect significant innovation responses much later, perhaps 5 or 10 years in advance or even contemporaneously.12 12 A previous version of the paper also investigated a number of other generalizations, which we omit because 11 2.4 Generics and Non-Generics The analysis so far did not distinguish between generics and non-generics. In the empirical work, we first look at all entries, and then distinguish between generics and non-generic drugs– which better correspond to “innovation”. We now briefly discuss how the predictions of the model change when we incorporate a distinction between generics and non-generics. We do this in the simplest possible way, and assume that pharmaceutical firms can engage in R&D to discover new drugs as described above, or they can engage in costly development to introduce a generic version of an already-existing drug (without quality improvement).13 Although bringing a generic drug to the market does not involve original research, it still requires substantial resources spent upfront (for the approval process or for marketing).14 Let us suppose that one unit of the final good devoted to prepare a generic for the market in drug line j leads to successful entry at time t at the flow rate θj , so entry of new generics at time t is given by gj (t) = θj hj (t), where hj (t) is total generic development expenditure for drug line j at time t. In practice, in addition to technological factors, length of patents on non-generics influences θj. We assume that θj > δ j , since introducing a generic to the market must be easier than inventing a new drug. If there is entry of a generic into drug line j at time t, we assume that both the incumbent and the generic entrant receive profits of µ (λ − 1) γYj (t) where Yj (t) is defined in (5), and µ ∈ [0, 1/2). Recall that if the generic entrant and the incumbent engage in Bertrand competition, then they will both charge marginal cost, and we would have µ = 0. The formulation here allows some degree of non-Bertrand (e.g., Cournot) competition or collusion, so µ > 0 is possible. If of space constraints. Briefly, we allowed research to be only imperfectly directed, so that research towards the drug line j leads to a flow rate of pδ j of discovering a new drug of this type, and to a flow rate of (1 − p) δ j 0 /J any j 0 = 1,..., J, where 1 ≥ p > 0. When p = 1, we have the model of subsection 2.1, while with p → 0, research becomes undirected. Interestingly, this generalization does not affect the results of the model, and even with p → 0, the innovation rates are given exactly by (9), and thus the results do not depend on the assumption of perfectly directed research. We also generalized to set up by allowing “technological drift”, meaning random innovations arising from non- profit and non-economic motives, which again did not affect the results, and led to an equilibrium relationship similar to (9). Details are available upon request. 13 An alternative approach would be to link the entry of non-generics directly to the delayed entry of generics, since generics can only enter after the patents on previous non-generics expire. Nevertheless, because introducing a generic into the market still involves significant upfront costs, we expect profit incentives to play a major role here. A hybrid model incorporating both such delays and profit incentives is significantly more complicated to analyze. 14 Prior to 1984, the FDA demanded a similar application process for approval of a generic drug as for a non-generic. For example, the generic drug’s safety and efficacy had to be demonstrated through clinical trials. DiMasi et al (1991) suggest that the cost of approval for a generic was as much as 40% of the cost of a non- generic. The Hatch-Waxman Act in 1984 allowed a company to obtain FDA approval for a generic drug by demonstrating that it is molecularly similar to and has the same active ingredients, indications and strength as the non-generic, substantially reducing the costs of introducing a generic. Reiffen and Ward (2002) estimate the cost of introducing a new generic to be around $5 million after the Act, compared to over one hundred million dollars before (DiMasi et al, 1991). 12 µ = 0, there would be no entry of generics, and the results in subsection 2.1 would apply. In addition, we assume that in a market that already includes the incumbent and a generic producer, there is no further room for a third producer, so we can ignore the potential entry from further generic producers. Allowing for further entry of this sort introduces additional notation, but does not affect our qualitative results. Given this structure, the value of innovation (for a non-generic) is now given by rVj (t | qj ) − V̇j (t | qj ) = π j (qj ) − δ j zj (qj ) Vj (t | qj ) − θj hj (t) [Vj (t | qj ) − Wj (t | qj )] , (17) where π j (qj ) = µ (λ − 1) γYj (t) as before and Wj (t | qj ) is the value of being one of two producers supplying drug j at time t. The main difference between this expression and (6) above is the last term, which takes into account that at the flow rate θj hj (t) there will be entry of a generic, in which case the innovator loses its monopoly position, and the associated value Vj (t | qj ), and becomes one of two producers, receiving value Wj (t | qj ). With a similar reasoning to before, this value is given by: rWj (t | qj ) − Ẇj (t | qj ) = µπ j (qj ) − δ j zj (t) Wj (t | qj ). (18) Intuitively, the only reason why the flow of profits captured by Wj (t | qj ) will come to an end is because there is a better drug introduced to the market, which happens at the rate δ j zj (t). Free entry requires that zj (t) ≥ 0, and δ j Vj (t | qj ) ≤ 1 with complementary slackness hj (t) ≥ 0, and θj Wj (t | qj ) ≤ 1 with complementary slackness. These conditions are similar to (7) above, but written out explicitly in the form of a comple- mentary slackness condition to emphasize that there may not be R&D for new drugs or any generic entry under certain conditions. Let us next assume that µθj < δ j (19) for all j. If this assumption does not hold, entry of new generics is so rapid that it ceases to become profitable for pharmaceutical companies to introduce new drugs, and consequently, quality improvements come to an end. As long as Assumption (19) holds, similar arguments to before imply that the unique equilibrium is given by: µθj (λ − 1) γYj (t) − r zj (t) = , (20) δj (δ j − µθj ) (λ − 1) γYj (t) hj (t) =. θj − δ j 13 It can also be verified that if µ = 0, the equilibrium of Proposition 1 applies, and if Assumption (19) does not hold, there will be no R&D, i.e., zj (t) = 0, and thus limt→∞ hj (t) = 0 (or Pr limt→∞ hj (t) = 0) as there will eventually be two producers in the market for drug j. Moreover, the entry rate of non-generics and generics, nj (t) and gj (t), are: nj (t) = µθj (λ − 1) γYj (t) − r, (21) θj (δ j − µθj ) (λ − 1) γYj (t) gj (t) =. θj − δ j There are a number of important points to note about this equilibrium. First, the entry rates of both non-generics and generics respond positively to market size. Therefore, this model generalizes the key prediction of our baseline model in subsection 2.1. Second, other comparative static results are now quite different than in the baseline model. The entry rates of non-generics no longer respond to δ j (and zj (t) is decreasing in δ j ). Instead, they respond positively to µ and θj (two parameters that should intuitively make entry of generics more profitable). This is because the rate of entry of non-generics is determined to ensure 0 profits for generics; once generic drugs enter the market, their producers will continue to make profits until there is a new and better drug. Finally, differentiating the equations in (21) with respect to Yj (t) shows that either generics or non-generics may respond more to changes in market size (it depends on whether µ or (δ j − µθj ) / (θj − δ j ) is larger). Plausibly, we expect µ to be small and θj − δ j to be large, and therefore, generic entry to be more responsive to changes in market size than non-generic entry.15 3 Empirical Strategy 3.1 Empirical Specification and Estimation Issues As r → 0, we can take logs on both sides of equation (9) to obtain: log nj (t) = constant + log δ j + log mj (t) , (22) where mj (t) ≡ λγYj (t) is the market size for drug line j at time t. We measure entry of new drugs (or innovation), nj (t), as new drug approvals by the FDA (Food and Drug Administra- tion) in broad drug categories as described below.16 This measure, denoted by Nct for drug category c at time t, includes entry of generic drugs. Although generic drugs do not correspond to “innovation” according to the standard use of this term, they are still driven by the same 15 In practice, the presence of uncertain delays in the development and approval process for non-generics may also imply a smaller response to the current market size for these drugs. 16 Besides new drug approvals and patents, which we also use below, there is a third proxy for innovation rates: clinical trials. We were unable to obtain data on clinical trials for a sufficient number of drug categories. 14 profit incentives as innovation, and are similar to innovation in the context of our model.17 After presenting results using all drug approvals, we separate generics from non-generics, and investigate whether the relationship between market size and entry differs for the two types of drugs. Instead of actual market size, mj (t), we will use potential market size driven by demographic changes, which we denote by Mct , and discuss its construction below. Adding other potential determinants, time effects and an error term capturing other unob- served influences, and allowing the coefficient of log Mct to differ from 1 as it would with more general preferences than Cobb-Douglas, we arrive at an estimating equation of the form: log Nct = α · log Mct + Xct0 · β + ζ c + µt + εct , (23) where Nct is the number of new drugs in category c in time period t, Mct is potential market size, Xct0 is a vector of controls, including a constant, with β as the corresponding vector of coefficients, ζ c ’s are a full set of category fixed effects that correspond to the log δ j terms above, µt ’s are a full set of time effects capturing any common time component, and finally, εct is a random disturbance term, capturing all omitted influences. The specification with the dependent variable in logarithm is useful, since it ensures that drug category fixed effects and time effects have proportional impacts on entry of new drugs. One problem with equation (23) is that Nct is a count variable (number of new drugs), so it can equal 0. In our data, this is not common, but in most specifications there are typically a few drug category-time cells where Nct is equal to 0. This makes it impossible to estimate (23). We take a number of approaches to this problem. First, we change (23) to log Ñct = α · log Mct + Xct0 · β + γ · dct + ζ c + µt + εct , (24) where Ñct = Nct if Nct ≥ 1 and Ñct = 1 if Nct = 0, and the variable dct is a dummy that equals 1 when there are no approvals, i.e., dct = 1 if Nct = 0 and dct = 0 otherwise. This procedure, which was first used by Pakes and Griliches (1980), has the advantage of simplicity and flexibility (the data determine how Nct = 0 should be treated). The drawkback is that the variable dct is mechanically a function of Nct , so it can introduce various biases. More satisfactory is to consider the following Poisson model (see, for example, Wooldridge, 1999, 2002, or Hausman, Hall, and Griliches, 1984): Nct = exp(α · log Mct + Xct0 · β + ζ c + µt ) + εct , (25) 17 Moreover, if generics and non-generics are imperfect substitutes, generics would correspond to new products, and their entry would increase consumer welfare in a manner similar to technological improvements, and in fact, formally correspond to technological change in the product variety models (e.g., Romer, 1990, Grossman and Helpman, 1991). 15 which is a slight variant on equation (23) above. Estimates from the two models are typically similar when there are only a few empty approval cells. When there are more empty cells, as when we look separately at generics and non-generics, there are larger discrepancies between the two models, and in those cases we favor the Poisson model. The estimation of (25) would lead to biased estimates, however, since the fixed effects, the ζ c ’s, cannot be estimated consistently. To deal with this problem, we follow Hausman, Hall, and Griliches (1984), and transform (25) to obtain: exp(α · log Mct + Xct0 · β + µt ) Sct = PT + εct , (26) 0 τ =1 exp(α · log Mcτ + Xcτ · β + µτ ) PT where Sct = Nct / τ =1 Ncτ is the number of drugs approved in category c at time t, divided by the total number of drugs approved in category c, and T is the total number of time periods in the sample. This transformation removes the drug category dummies, and the coefficient of interest, α, can be estimated consistently. We estimate this equation using nonlinear least squares (NLLS) as well as maximum likelihood (ML). Woodridge (1999) shows that NLLS estimation strategy has good consistency properties, even when the true model is not Poisson. Finally, we also report some estimates from the negative binomial model which relaxes the distributional assumptions of the Poisson model in a maximum likelihood context (see, for example, Wooldridge, 2002, or Hausman, Hall, and Griliches, 1984). In addition to equations (24) and (26), which have the contemporaneous value of log Mct on the right hand side, we also estimate equations with leads and lags of log Mct to determine whether there are significant delays and anticipation effects. Such effects are possible, since, as reported by DiMasi et al. (1991), it can take as long as 15 years for a drug to enter the market from the time of initial research. Furthermore, changes in demographics can be anticipated a long time in advance, so drug approvals may respond to anticipated future market sizes, as highlighted by our analysis in subsection 2.3. 3.2 Potential Market Size and Identification Throughout, we exploit the potentially exogenous component of market size driven by demo- graphic trends, combined with differences in the age distribution of users for different types of drugs. We obtain the age composition of users (and expenditure) from micro drug consumption data, and the changes in U.S. demographics from the CPS (Current Population Survey) data. Our measure of potential market size is: X Mct = uca · pat , (27) a 16 where pat is the U.S. population or income of age group a at time t, and uca is the time-invariant measure of consumption of drug category c by individuals in age group a. We take time periods to be either five-year or ten-year intervals. We compute Mct in two alternative ways: first, we use the U.S. population for age group a at time t for pat and the average number of drugs in category c used per person in age group a for uca ; and second, we use the total income of age group a for pat and the average share of drugs in category c in the total expenditure of those in age group a for uca. The latter, which we refer to be as the income-based measure, corresponds more closely to market size in the theoretical model, which is a combination of the number of consumers and their incomes, and will be our main measure.18 For both measures, the over-time source of variation is not from changes in individual use, but purely from demographic changes captured by pat –i.e., uca ’s are not time-varying. So for example, changes in prices, which potentially result from innovations and affect consumption patterns, will not cause over-time variation in Mct. The major threat to the validity of our empirical strategy is from potentially time-varying omitted variables (any variable that is not time-varying is taken out by the drug category fixed effects). Omitted variables related to market size or profit opportunities may induce a bias in the implied magnitudes, but will not lead to spurious positive estimates of the effect of market size (in other words, the presence of such variables is essentially equivalent to mismeasurement of the appropriate market size). More threatening to our identification strategy would be omitted supply-side variables. If our instrument is valid, it should be orthogonal to variation in supply-side determinants of entry. We attempt to substantiate our identifying assumption further by including lagged dependent variables, and adding controls such as pre-existing trends and proxies for other incentives to undertake research in a particular field.19 4 Data and Descriptive Statistics 4.1 Basic Data Sources The demographic data come from the March CPS, 1964-2000. We construct five age groups, 0-20, 20-30, 30-50, 50-60, and 60+. These divisions are motivated by drug use patterns of these age groups. To construct income shares, we divide household income equally among the 18 If preferences have a Cobb-Douglas form as in (1) and are stable, the expenditure measure of uca will always be constant. With non-Cobb-Douglas preferences, changes in prices will induce changes in uca over time. In this case, by using a time-invariant measure of uca , we remove this potentially endogenous source of variation. 19 Another source of endogeneity may be that innovations in certain drug categories extend the lives of the elderly, thus increasing their Mct. Lichtenberg (2003) provides evidence that new drugs extend lives. This source of endogeneity is not likely to be quantitatively important, however, since the variation resulting from extended lives in response to new drugs is a small fraction of the total variation in Mct. Nevertheless, we will also report estimates that instrument Mct with past demographics, purging it from changes in longevity. 17 members of the household. Figure 2 shows population shares for the five age groups, and Figure 3 shows the corresponding income shares (i.e., income of the corresponding age group divided by total income). To facilitate comparison with Figure 4, this figure starts in 1970. Both figures show a large amount of variation across age groups over time. In particular, it is possible to trace the baby boomers, as the fraction of those in the age bracket 20-30 in the 1970s, and those in the age bracket 30-50 in the 1980s and the 1990s. The FDA classifies all prescription drugs into 20 major drug categories, which are further subdivided into 159 categories. These categories are based on a combination of therapeutic intent and chemical structure.20 We drop 4 of the 20 major categories from this classification: Anesthetics, Antidotes, Radiopharmaceuticals and Miscellaneous.21 We obtain a total of 34 categories by breaking 10 of the 16 remaining categories into finer groups when there are signif- icant heterogeneity in terms of the age distribution of users across subcategories. We separate the major categories of Antimicrobials, Psychopharmacologics, Nutrients, Hormones, Dermato- logics, Neorologics, Ophthalmics, Otologics, Pain Relief and Respiratory because within these categories there are subcategories with significantly different age profiles of users. For example, within Antimicrobials, 0-20 year-olds use Antibiotics (except Tetracyclines) the most, while Antivirals are used most by people 30 and older. Appendix Table A1 lists the 34 categories. Our main data source for drug use is the Medical Expenditure Panel Survey (MEPS), which is a sample of U.S. households over the years 1996-1998. The survey has age and income data for each household member, and covers about 25,000 individuals in each year. There is also a list of prescription drugs used by each person (if any), and the amount spent on drugs. In all, there are about 500,000 medications prescribed. We construct drug use per person and expenditure share for each category and each of our five age groups. Appendix Table A1 reports these numbers, and shows a large amount of variation across drug categories. Many of the categories are used more by older people than by younger, but there are numerous exceptions. For example, Contraceptives are used most by 20-30 and 30-50 year-olds, and Penicillins and Antibacterials are used most by individuals in the youngest group. We construct the measures of potential market size according to equation (27) by combining data from the MEPS and the CPS. We supplement the MEPS data with the National Ambulatory Medical Care Survey (NAMCS), 20 Other authors, for example Lichtenberg (2003), use a different classification system based on diseases. For our purposes, the FDA classification is attractive, both because it enables us to construct a better classification of all approved drugs currently on the market, and because the microdata surveys give the FDA classes for the drugs that the respondents use (see Data Appendix for details). 21 We drop the Anesthetics, Radiopharmaceuticals and Miscellaneous categories because most of the items in these categories were not developed for a distinct market. Radiopharmaceuticals are used for diagnostic purposes, and the Miscellaneous category mainly contains surgical and dental tools. The Antidote category is dropped because there are few drugs approved and there is little use of these drugs in the surveys. See the Data Appendix for further details on the contruction of our categories. 18 which is an annual survey of doctors working in private practices. The survey includes drug use for the years 1980, 1981, 1985, and 1989-2000. Observations are at the doctor-patient-visit level; there are about 40,000 visits per year. Doctors are selected randomly, surveyed for a week, with patient-visits selected randomly from the week. The main use of the NAMCS is that it covers a longer time period, enabling us to check whether the age composition of users across categories has changed over time. Using the NAMCS data, we construct a second drug categorization, with 30 categories.22 Table 1 gives correlations between various measures of drug use. Panel A shows a high degree of correlation between the NAMCS surveys at various dates, indicating that the age profile of users has not changed significantly over the 1980s and the 1990s. The overall correlation row looks at the unconditional correlation. We also report weighted correlations, where observations are weighted by cell size in the MEPS or NAMCS. Weighted correlations are larger than the overall correlation because our estimates of the age distribution of users are less precise when there are fewer individuals using drugs in a particular category. The third row reports mean correlation by drug, which calculates the within category correlation between the two measures and then averages it across all categories. This measure is more informative for the question of whether the age distribution of users for a particular drug has changed over time. Panel B performs the same calculation for the three waves of the MEPS, and similarly shows substantial persistence in the age distribution of drug expenditure. Finally, panel C shows a high degree of correlation between expenditure shares and use per person in the MEPS data. But perhaps surprisingly, there is low correlation between the NAMCS and the MEPS. This is because the two surveys yield very different estimates for total use of each category (but very similar estimates of relative use by age groups within each category, as shown by the high level of mean correlation by drug). We conjecture that this reflects the fact that NAMCS, which samples doctors in private practice rather than individuals, is not as representative as the MEPS. The last major data source is a list of new FDA drug approvals. We exclude over-the-counter drugs, the so-called orphan drugs,23 and drugs that have the same identifying characteristics 22 Appendix Table A2, which is available upon request, compares this classification system with the 34 category system developed with the MEPS. Some of the 159 categories have been dropped from one classification system, but not from the other, because there were not sufficient observations to construct reliable estimates of drug use from one of the surveys. Appendix Table A2 shows that the two systems are closely related. In general the 34 category system is a slightly less aggregated version of the 30 category system. Nevertheless, there are a number of cases where a given FDA category is combined with a second FDA category in one system, but with a third FDA category in the other system (e.g., Misc. Antibacterials are with Sulfonamides in the MEPS system, but with Antiseptics in the NAMCS system). 23 These drugs treat rare conditions, affecting fewer than 200,000 people. An example is botox, first developed to treat adult dystonia, which causes involuntary muscle contractions. We drop these drugs because we have difficulty matching them consistently, and because they receive special inducements under the Orphan Drug 19 (i.e., same name, company, and category, or the same FDA approval number). We focus on the time period 1970-2000. Since we can only match FDA categories for drugs that are still listed by the FDA, the quality of the approvals data deteriorates as we go back in time. In addition, because we are using age composition from the 1990s, the quality of our measures of potential market size also deteriorates as we go back in time. Our approvals dataset for 1970- 2000 comprises 6,595 prescription drugs, including both generics and non-generics (see the Appendix). Since 1970 there have been about half as many approved non-generics as generics. Figure 4 shows the share of drug approvals over time to compare with changes in income shares depicted in Figure 3 (or population shares shown in Figure 2). To construct Figure 4, we compute drug approvals over five-year intervals for the 34 categories. We then combine the 34 categories into five groups, based on the age group that accounts for the largest fraction of use in that category (thus this cut of the data uses only part of information that we will exploit in the regression analysis), and compute the share of drug approvals by dividing the number of approvals in a given category by total approvals in that time period.24 Comparing this figure with Figure 3, a positive association between contemporaneous changes in population share and changes in drug approvals for the corresponding age group can be detected visually. For example, the income share of the 30-50 group increases over the sample, and so does the entry of drugs most used by this group. Both the shares of income and entry of drugs for those 0-20, on the other hand, show a downward trend, while those for the 60+ age group show an increase followed by a decline. Table 2 also shows changes in income shares by age group and in FDA drug approvals (for all drugs as well as separately for generics and non-generics), and confirms the patterns depicted in Figures 3 and 4. These patterns are explored in greater detail in the regression analysis below. 5 Results 5.1 Basic Specifications Table 3A provides the basic results from the estimation of (24) and (26) with non-linear least- squares (NLLS) and ordinary least-squares (OLS), and Table 3B reports maximum likelihood estimates of the Poisson and negative binomial models. We start with the potential market size measure constructed using MEPS data. These basic specifications do not contain any covariates Act. 24 There are large fluctuations in the total number of approvals, presumably because of a number of institu- tional changes. For example, it was discovered in 1989 that some FDA officials were taking bribes to speed up the approval process for generic drugs. As a result, in the early 1990s the approval process for generics was greatly slowed. See, for example, The Washington Post, August 16, 1989. When we separate our approval data into generics and non-generics, we see a large drop in generics approvals in the early 1990s, but only a small decline for non-generics. We thank Ernie Berndt for suggestions on this issue. 20 other than drug category effects and time effects. The results show a large and significant effect of (potential) market size (log Mct ) on entry of new drugs. In column 1 of Table 3A, we start with our basic “income-weighted” measure of log Mct , constructed using expenditure data from the MEPS data set, and income from the CPS, with the time periods corresponding to five-year intervals. Since our estimates of age composition in smaller categories are less precise, observations are weighted by MEPS cell sizes (total expendi- ture for income-based measures in the cell and total drug use for population-based measures). The standard errors throughout are corrected for heteroscedasticity using the Huber-White formula. The NLLS estimate of α in this basic specification is 6.04 with a standard error of 1.95, which is significant at the 1 percent level. The OLS estimate is somewhat smaller, 4.52, with standard error 2.00, thus significant only at the 5 percent level.25 Potential market size appears to explain a sizable fraction of the total variation in the entry of new drugs (for example, the partial R2 of the OLS specification is 0.20), and the empirical relationship is not driven by outliers. Figure 5 shows a plot of the residuals of log Nct against the residuals of log Mct in the OLS regression, in both cases after drug category and period dummies are taken out. Observations are labeled by their drug category codes (see Appendix Table A1), and each code appears more than once, since there are multiple periods. The line in the figure corresponds to the estimated relationship reported in column 1. Although categories 42, 43 and 61 (Anorexiants, Central Nervous System drugs and Vitamins/Minerals) typically fit the pattern less well and are outliers in either direction, excluding these has little effect on our estimate of α. For example, dropping these three categories leads to an NLLS estimate of 7.07 (s.e.= 2.29). The quantitative magnitude of the effect in column 1 is large but plausible, implying that a 1 per cent increase in our market size measure leads to about a 6 percent increase in drug approvals. Since there are a total of 6,595 approvals between 1970 and 2000, thus on average 32 approvals in every five-year interval in each of our 34 categories, the estimate of 6.04 implies that a 1 percent increase in market size leads to the entry of about two new drugs. Total phar- maceutical sales was approximately $130 billion in 1999 (IMS, 2000), which implies an average annual expenditure of $3.8 billion per category. A 1 percent increase therefore corresponds to $38 million, or about $570 million over 15 years, which is the life of a typical drug. Since entry costs for non-generics are around $800 million (in 2000 dollars, DiMasi et al, 2001), while for generics they have varied between $5 million and over $100 million (DiMasi et al, 1991, Reiffen and Ward, 2002), entry of two new drugs in response to an increase of $570 million in revenue 25 Clustering the standard errors at the level of drug categories has little effect because there is no residual serial correlation–see the lagged dependent variable specifications reported in Table 6. 21 is within the range of plausible responses. In column 2, we use ten-year intervals instead of the five-year intervals, both to reduce the potential noise in the entry of new drugs during the five-year intervals and also to check whether ten-year intervals correspond more closely to the relevant match between market size and entry. The estimate of α using NLLS is now 4.95 with standard error 1.90, significant at the 1 percent level. The smaller NLLS estimate indicates that there might be some attenuation with the ten-year invtervals, but the ten-year OLS estimate is slightly larger than the five-year estimate. In the remainder, we mainly focus on five-year intervals. Although the income-weighted measure of market size seems more satisfactory, in columns 3 and 4 we also look at the effects of changes in market size driven purely by population changes. Using this measure leads to estimates that are slightly larger for both NLLS and OLS, and are estimated with about the same level of precision.26 To see the effect of weighting on the estimates, columns 5 and 6 report unweighted regres- sions. Without weights, the NLLS estimates are smaller than in columns 1 and 2, but still significant at the 1 percent level, and the OLS estimates, also smaller than in columns 1 and 2, are significant at the 5 percent level. We conjecture that this somewhat weaker relationship without weights reflects the less precise estimation of age composition in the smaller categories, which is illustrated by the correlations in Table 1.27 The results in Table 3B are broadly similar, and show that the main results are robust to different estimation methods. In panel A of that table, we use maximum likelihood to estimate equation (26). The number below the estimate is the maximum-likelihood standard error, while the number in curly brackets is the robust standard error that does not impose the Poisson structure to calculate the standard errors, and instead uses the Huber-White formula. The estimates are unweighted. In the first two columns, we compute market size using income and expenditure. The estimates are larger than the corresponding estimates in columns 5 and 6 of Table 3A. In panel B we use a weighted maximum likelihood procedure, and obtain similar estimates to those in columns 1-4 of Table 3A. Finally, we also report estimates from the negative binomial model in panel C, which allows for overdispersion of the Poisson parameter. In this case, the estimates of α are slightly smaller than those in columns 1-4 of Table 3A, and 26 We also estimated these models using West European and Japanese demographic information in addition to U.S. information (obtained from the United Nations website, esa.un.org/unpp/). Using the total population- based market size measure combining European, Japanese and U.S. populations gives similar results to those obtained using only U.S. information. For example, the NLLS specification using this total market size and five-year intervals gives an estimate of 5.27 (s.e.=1.50), as compared to the corresponding estimate of 6.16 in column 3. 27 This conjecture also receives support from the fact that when we construct our potential market size measure using the NAMCS, which has a more even distribution of observations across drug categories, the unweighted and weighted results are similar (see Table 7 below). 22 still significant at 1 or 5 percent. 5.2 Delays and Anticipation Effects The theoretical analysis suggested that delays in the development and approval processes are unlikely to create delays in the entry of new drugs in response to changes in market size, but there is room for new drugs to enter before the actual increase in market size because of anticipa- tion effects, especially since demographics-driven changes in market size should be anticipated in advance. We investigate the role of delays and anticipation effects in this subsection by including lags (log Mc,t−1 ) and leads (log Mc,t+1 ) of potential market size on the right hand side of our estimating equations. Columns 1 and 2 of Table 4 replicate the basic five-year and ten-year specifications from Table 3A for comparison. Columns 3 and 4 include the market size from the previous period and show that new drug entry responds mainly to current market size (in fact, the coefficient on lag market size is negative, though insignificant, presumably because current and previous market sizes are highly correlated). For example, in column 3, the coefficient on current market size is larger than our baseline, 13.56 (s.e.=4.45) with NLLS, and 8.62 (s.e.= 3.91) with OLS.28 Columns 5 and 6 show that lag market size is also typically insignificant when entered by itself (with the exception of Panel A in column 5, where it is significant but much smaller than the estimate with current market size in column 1). These results therefore show no evidence of significant delays in the response of new drug entries to changes in market size. In column 7, we include the current and one period ahead market size. Both the contem- poraneous and lead market sizes are individually insignificant, but jointly significant at the 1 percent level. The coefficient on lead market size is much larger. In column 8, when we use ten-year intervals, instead, the coefficients are about the same magnitude, but current market size is significant at the 1 percent level (and similar to the baseline) while the lead market size is insignificant. Columns 9 and 10 show large and significant coefficients when we include only future market size.29 These results therefore suggest that there may be some anticipation effects, perhaps with five- or ten-year leads, which is consistent with the possibility of limited anticipation effects highlighted by the theoretical model.30 28 We construct the lagged market size measures for 1960s using demographic information from the CPS, so the number of observations does not decline. The results are similar if we only use the post-1970 data. 29 We have also extended the sample for the lead specification by combining the population projections of the U.S. Census Bureau for 2010 with the incomes from the 1990s (the results are also similar if we use a linear extrapolation to predict future income). Using this additional period yields similar results. For example, re-estimating the specification in column 4 with NLLS, the estimate on current market size is 3.10 (s.e.=3.75) and the estimate on lead market size is 4.33 (s.e.=4.03), jointly significant at 1 percent. The specification in column 6 gives an estimate on lead market size of 7.10 (s.e.=1.86). 30 Here “limited” does not refer to the strength of the effect, but to the fact that the response to market size 23 5.3 Potential Supply-Side Determinants of Innovation In this subsection, we investigate the robustness of the baseline results to controlling for po- tential non-profit determinants of innovation, such as changes in scientific incentives or oppor- tunities captured by the δ j ’s in the theoretical model. First, recall that the major threat to our identification strategy is changes in the δj ’s (since permanent differences in δ j ’s are already taken out by our drug category fixed effects). If the δ j ’s change over time, they are also likely to be serially correlated. Adding lags of log Nct to our basic specifications is therefore a simple way to check for the importance of these concerns. Columns 1 and 2 of Table 5 report the results of estimating a lagged dependent variable specification, by adding a one-period lag of the dependent variable, log Nct−1 , to our basic specifications. In the OLS version, the basic regression therefore changes to: log Nct = α · log Mct + ψ · log Nct−1 + γ · dct + δ c + µt + εct. (28) Since log Nct−1 is correlated with the error term mechanically, estimates to this equation would be biased, and we deal with this problem by instrumenting log Nct−1 with log Nct−2. This is a valid instrument as long as there is no additional autocorrelation in the error term, εct (see, for example, Blundell and Bond, 1998). This specification is also useful more generally to check for other sources of serial correlation in the entry rate of new drugs (such serial correlation would make the arguments for the exogeneity of log Mct less compelling). The estimates of α from equation (28), reported in columns 1 and 2, are quite similar to the baseline. The coefficient on the lagged dependent variable, log Nct−1 , is essentially 0 and insignificant for five-year intervals; for ten-year intervals, though significant with OLS, it is again insignificant with NLLS. These results therefore show that there is no significant residual serial correlation, due to either changes in scientific opportunities or other sources, and that controlling for such serial correlation has no effect on our estimates. A plausible conjecture is that non-profit incentives to develop drugs would be particularly responsive to opportunities to save lives or cure major illnesses. Motivated by this reasoning, our second strategy looks at variation in the health benefits of new drugs across categories. New drugs in our data set include both drugs that are demanded by the consumers but do not “save lives”, such as Prozac, Paxil, Vioxx, or Viagra, or those that actually save lives such as heart medicines or cancer treatments (see Lichtenberg, 2003, on the effect of pharmaceutical innovations on declines in mortality). To investigate this issue, we measure the number of is 5-10 years before the change in market size, not further in advance. If we include further leads of market size, these are much smaller and insignificant. For example, the 15 years lead when included by itself is highly insignificant; the NLLS estimate is 1.57 (s.e.=2.52), and the OLS estimate is 1.16 (s.e.=6.06). 24 life-years lost corresponding to each drug category using the Mortality Detail Files from the National Center for Health Statistics from 1970-1994. Following Lichtenberg (2003), for each death, we subtract the person’s age from 65, then calculate the total number of life-years lost for all the deaths resulting from diseases related to drugs in each category.31 We add this measure of life-years lost to the right hand side of our baseline regression models as a proxy for this source of non-profit incentive to undertake research. Since we are using mortality data prior to 1995, we drop the last time period from the regression. The baseline regression for the years 1970-1994 is reported in column 3 of Table 5, and leads to an estimate of approximately the same size as the baseline using all years. Column 4 reports the result of using the life-years lost variable, and finds no change in our estimate of α. The coefficient on the life-years lost variable (unreported) is small and insignificant.32 Starting in column 3, in addition to NLLS estimates in panel A and OLS estimates in panel B, which use current market size, we also report NLLS estimates with leads of market size in panel C. These specifications typically yield results very similar to those in Table 4. Third, we investigate the implications of differences in scientific funding for various drug categories. Using the Computer Retrieval of Information on Scientific Projects (CRISP) dataset (details in the Data Appendix), we construct a variable measuring the total amount of federal funding for research projects in all drug categories, and include this variable as a control on the right hand side. To the extent that government funding also responds to potential market size (for example, because drug companies have a greater tendency to apply for funding in areas where they plan to do research), this variable would be correlated with our market size measure. In practice, the correlation is low, and columns 5 and 6 show that the inclusion of this variable, or both its current and lag values, has little effect on our estimates of α. Fourth, to control for potential trends in scientific opportunities across drug categories, we add proxies for pre-existing trends. We construct an estimate for pre-existing trends as ∆c = (log Nc,60 − log Nc,40 )/2, where log Nc,60 is the log approvals for category c in 1960 and log Nc,40 is the log approvals in 1940. We then estimate the equation: X log Nct = α · log Mct + ∆c · σ i + δ c + µt + εct , (29) i=80,90 where σi ’s are dummies for the 1980s and 1990s. This specification allows drug categories that 31 For example, if someone dies at age 32, this counts as 33 life years lost; people dying older than 65 receive no weight in this calculation. Note that the Mortality Detail Files are coded by disease class, so we must convert the classification to our system. Since many of our categories contain diseases or conditions that do not lead to death, we obtain a number of empty cells. 32 We also ran separate regressions using five- and ten-year lags of life years lost (both unreported), and again found no change in our estimates of α. 25 have grown at different rates between 1940 and 1960 to also grow at different rates in the 1980s and the 1990s. Column 7 reports the results of this exercise. The estimate of α is similar to our baseline estimate, 6.23, with standard error 1.88. Column 8 repeats the same exercise with ∆c = (log Nc,70 − log Nc,40 ) /3 as the measure of pre-existing trends. The resulting estimate is again similar to our baseline. These results are perhaps not surprising, since pre-1970 approvals are considerably noisier, thus only an imperfect control for pre-existing trends. An alternative, and substantially more demanding, strategy is to include linear time trends. To do so, we estimate: log Nct = α · log Mct + η C · t + δ c + µt + εct , (30) where c refers to the 34 detailed drug categories, and C refers to the relevant 16 major drug category, i.e., the one which detailed category c belongs to. We expect technological differences to be captured by which of the 16 major drug categories each drug belongs to, since these categories are based on therapeutic intent, while the subcategories are based on use by age group. The estimates, reported in column 9, are close to our baseline. For example, with NLLS, the estimate of α is 5.60 (s.e.=2.10). The OLS estimate is smaller and insignificant. Using lead market size instead of current market size results in a similar pattern: the NLLS estimate, 8.46 (s.e.=2.53), is significant at 1 percent, while the OLS estimate (not reported to save space), 4.99 (s.e.=2.81), is significant at 10 percent. We also investigate the potential effects of advances in biotechnology, such as the use of recombinant DNA, or other technological changes, during the late 1980s and the 1990s. In terms of our model, these developments would correspond to changes in the δ j ’s. In column 10, we drop the categories of Cancer and Cardiovascular, which, according to the FDA approval list, have witnessed the entry of the greatest number of orphan drugs, presumably by biotechnology firms. Although, as noted above, our dependent variable does not include these drugs, we also check whether our results are driven by entry of new drugs in these categories. The estimates in column 10 are close to those in column 1 of Table 3A, demonstrating that the estimates of α are not sensitive to dropping these two categories. In addition, there is anecdotal evidence that biotechnology firms were first active in produc- ing insulin (the Glucose and Thyroid category) and in the Hematologic category.33 In column 11, we drop these two categories, and again find that our results are essentially unchanged. To assess the role of biotechnology firms further, we add the approvals of a group of prod- ucts known as biologics, where biotechnology firms have been active, to our measure of drug 33 Biotechnology firms were also active in producing human growth factor, but since there are only a small number of individuals using these drugs in the MEPS and NAMCS, these drugs are not included in our approvals dataset. 26 approvals. These products include some vaccines, blood and plasma related products, and other products such as interferon and erythroproteins (used for red blood cell production), and are not included in our baseline measure because they go through a separate FDA regulatory process. The results of this regression, reported in column 12, show little change in the estimates of α.34 Finally, to see whether the advent of biotechnology or other technological advances of the past two decades have changed the relationship between market size and entry of new drugs, we estimated our baseline models including an interaction between a post-1985 (or post-1990) dummy and market size. Our estimates showed no evidence of significant interactions. For example, in a specification parallel to the NLLS model of column 1 of Table 3A, the estimate of α is 5.24 (s.e.=1.96), and the interaction with the post-1985 dummy is 0.10 (s.e.=0.07), thus quantitatively very small and insignificant. The results in this subsection therefore show that a number of controls for other (non- market-size related) determinants of the entry of new drugs have little effect on our main findings. Although these results are not conclusive on the effect of scientific or other non-profit considerations in pharmaceutical research, they suggest that the effect of potential market size on entry and innovation is relatively robust. 5.4 Changes in Health Insurance Coverage Our market size measure only exploits changes in potential market size driven by demographic trends. Another source of variation in market size comes from changes in coverage of drug expenditure in private or public health insurance programs. Finkelstein (2003), for example, exploits changes in the coverage of various vaccines to estimate the effect of these policies on the development of new vaccines. During our sample period, there were significant changes in the coverage of drug expenditure in health insurance plans. For example, the percentage of 0-20 year-olds with some form of private health insurance coverage fell from about 73% to 68% between 1974 and 1996, while the percentage of 60+ year-olds with private insurance rose from 62% to 75% (authors’ calculations from the National Health Interview Survey). Furthermore, there have been changes in Medicaid eligibility rules, designed to insure more poor children. These changes in health insurance coverage induce additional changes in market sizes. We now investigate both whether controlling for this source of variation affects the estimates of the impact of the demographics- driven potential market size measure, Mct , and whether we can improve our measure of potential market size by including information on health insurance coverage. 34 We also repeated our basic regressions without the categories where biologics are most common, Antivirals (12), Hematologics (20) and Immunologics (80), with little effect on the estimates of α. 27 We use the National Health Interview Survey (NHIS, 1974-1996) to construct the fraction of each age group covered by a private health insurance plan. Because there is no consistent information on prescription drug coverage, we assign prescription coverage to any individual with both doctor and surgical coverage. Prescription drug coverage is highly correlated with this measure in the years where we can observe it. The NHIS also includes information on Medicaid and Medicare. Because Medicare does not cover prescription drugs, it enables us to perform a simple “falsification test”. First, with direct parallel to our Mct measure, we use the NHIS to construct the following insurance coverage variable: X H̄ct = uca · p̄a · fat , (31) a where fat is the fraction of age group a in period t with private health insurance, uca is the expenditure share as described above, and p̄a is the sample average income of age group a (in this section, we always use income-based market size and weights). We use p̄a rather than pat to isolate changes due to drug insurance coverage rather than demographic changes. We then add log H̄ct to our estimating equations (24) and (26). Column 1 of Table 6 shows that the addition of this variable has no effect on the estimates of α relative to those in Table 3A, and that our baseline results are robust to controlling for separate trends in private health-care coverage. For example, in the NLLS specification the coefficient is now 5.21, as opposed to 6.04 in Table 3A. The coefficient on log H̄ct itself is positive, though insignificant. Next, we construct alternative measures of log H̄ct using Medicaid and Medicare coverage rates for fat (though all individuals above 65 are covered by Medicare, not all of them take up the benefits). Not surprisingly, with Medicaid and Medicare, the estimates of α are unaffected and the effect of log H̄ct is substantially weaker, presumably because these measures exploit much less of the variation in actual market size. Columns 4-6 investigate the implications of adding the H̄ct measures in the specifications with lead market size, and show similar results. Columns 7-9 take an alternative approach and construct a market size measure incorporating both demographic changes and changes in insurance coverage: X e ct = H uca · pat · fat , (32) a where uca , pat and fat are as defined above. Using log H e ct instead of log Mct in column 7 leads to a similar coefficient relative to column 1 of Table 3A (4.27 with standard error of 1.24). The difference in magnitude may be because 28 insurance-driven and demographics-driven market sizes affect different types of drugs, or be- cause the demographics-driven changes are anticipated further in advance, potentially enabling a greater response. The most likely explanation, however, is that H e ct is a worse measure of market size than Mct because (32) effectively assigns 0 use to those without insurance.35 We next construct an alternative measure of H e ct from information on Medicaid coverage. Changes in Medicaid eligibility have made children more likely to be covered. In the 1970s, about 9% of 0-20 year olds were covered by Medicaid; this fraction rose to 17% by the late 1990s. Column 8 shows a very small and marginally significant effect of Medicaid insurance on the rate of entry of new drugs. ect calculated from information on Finally, as a falsification exercise, we use a measure of H Medicare take-up rates. Since Medicare does not cover prescription drugs, this measure should not predict new drug entries (though note that Mct and H ect are correlated by construction). Reassuringly, the estimate in column 9 shows no positive effect of Medicare coverage. 5.5 Reverse Causality Lichtenberg (2003) shows that new drugs have increased the average age at death (and hence, to a lesser extent, life expectancy) by as much as 1 percent per year. This introduces the potential for reverse causality whereby the market size for successful drugs may be endogenously larger, because their users live longer. We think this is not a first-order concern, since drug-induced changes in population are likely to be small relative to the demographic changes that we are exploiting. Nevertheless, we further address this issue by instrumenting for current population using the corresponding population from 10 years before. For example, we use the population fraction of 50+ year-olds in 1970 as an instrument for the population fraction of 60+ year-olds in 1980. The fraction of 50+ year-olds is highly correlated with the fraction of 60+ year-olds 10 years later, but is unaffected by new drugs that are developed in the intervening 10 years. These instrumental-variables (IV) estimates show no evidence of reverse causality. In column 2 of Table 7, we instrument for market size with past market size. With NLLS, the estimate is 5.56 (s.e. =1.69), slightly lower than the non-instrumented estimate for the same time period reported in column 1. Columns 3 and 4 show the corresponding results using ten-year intervals. The IV estimates in column 4 give larger coefficients than the corresponding non-instrumented results, particularly with NLLS, and both are significant at the 1 percent level. 35 Another possibility is that the effect of demographics-driven market size was somewhat overestimated in Table 3A because of the correlation between this measure and insurance coverage. However, the estimates in columns 1-6 in this table suggest that this is not a major concern. 29 5.6 Results from the NAMCS The rest of Table 7 repeats some of our main specifications using data from the NAMCS. This is a useful exercise because the NAMCS has a more even distribution of users across drug categories than the MEPS, and also because it starts in 1980, enabling us to check whether use of drug consumption and expenditure data from the late 1990s introduces any biases. However, as noted above, the NAMCS is less representative, since the data are reported by doctors in private practice, whereas the MEPS is a sample of all U.S. households. Column 5 shows our baseline regression, with the same specification as column 3 of Table 3A (we cannot repeat the specifications of columns 1 and 2, since the NAMCS does not provide drug expenditure information). The estimate of α using NLLS, 2.86, is insignificant and considerably smaller than the corresponding MEPS estimate. The OLS estimate is also smaller than the corresponding MEPS estimate, but is significant at the 1 percent level. Column 6 uses the MEPS data with the NAMCS classification system, and obtains estimates similar to column 3 of Table 3A. This shows that the disparity between the NAMCS results and Table 3A is not due to differences in classification systems, but is probably driven by the non-representative nature of the NAMCS data.36 In column 7, we use ten-year time intervals, and find similar results to column 5; the NLLS is insignificant while the OLS is significant at the 5 percent level. Columns 8 and 9 report unweighted results, which are larger than the weighted estimates. This contrasts with the pattern from the MEPS, and is consistent with our conjecture that the differences between weighted and unweighted results in the MEPS were largely because of the less precise age distribution estimates for the smaller categories in that data set (this is not a problem in the NAMCS, which leads to a more precise estimate of age distribution of users in the smaller cells). Finally, we use the NAMCS to investigate whether our reliance on drug use data from the late 1990s induces any systematic bias.37 We construct an alternative estimate of market size, Mct1980 , with the use per person numbers, u1980 ca , only from the 1980 NAMCS survey. We then