Unit 2 Presentation of Data PDF
Document Details
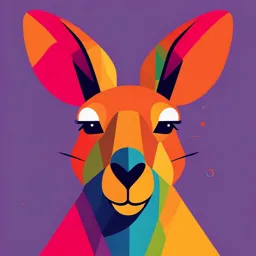
Uploaded by HalcyonMarimba5064
Tags
Related
- Estadistica para administracion y economia PDF
- Data Analytics for Business Optimization - Les Roches 2024.1 BBA6 PDF
- Marketing Data Collection & Analysis PDF
- Marketing Data Collection & Analysis PDF
- QTTM 409 Class Presentaiton of Dr P James Daniel Paul MSB LPU PDF
- Statistics for Business Analytics & Data Science PDF
Summary
This document covers the presentation of data, including classification methods, frequency distributions, and charting techniques such as bar diagrams, histograms, frequency polygons, and ogives. It provides examples to illustrate the concepts and offers exercises for practice.
Full Transcript
Data Collection and Analysis UNIT 2 PRESENTATION OF DATA Objectives After studying this unit, you should be able to : understand the need and significance of presentation of data know the necessit...
Data Collection and Analysis UNIT 2 PRESENTATION OF DATA Objectives After studying this unit, you should be able to : understand the need and significance of presentation of data know the necessity of classifying data and various types of classification construct a frequency distribution of discrete and continuous data present a frequency distribution in the form of bar diagram, histogram, frequency polygon, and ogives. Structure 2.1 Introduction 2.2 Classification of Data 2.3 Objectives of Classification 2.4 Types of Classification 2.5 Construction of a Discrete Frequency Distribution 2.6 Construction of a Continuous Frequency Distribution 2.7 Guidelines for Choosing the Classes 2.8 Cumulative and Relative Frequencies 2.9 Charting of Data 2.10 Summary 2.11 Key Words 2.12 Self-assessment Exercises 2.13 Further Readings 2.1 INTRODUCTION In the previous unit, we discussed the various ways of collecting data. The successful use of the data collected depends to a great extent upon the manner in which it is arranged, displayed and summarised. In this unit, we shall be mainly interested in the presentation of data. Presentation of data can be displayed either in tabular form or through charts. In the tabular form, it is necessary to classify the data before the data is tabulated. Therefore, this unit is divided into two section, viz., (a) classification of data and (b) charting of data. 2.2 CLASSIFICATION OF DATA After the data has been systematically collected and edited, the first step in presentation of data is classification. Classification is the process of arranging the data according to the points of similarities and dissimilarities. It is like the process of sorting the mail in a post office where the mail for different destinations is placed in different compartments after it has been carefully 18 sorted cut from the huge heap. Presentation of 2.3 OBJECTIVES OF CLASSIFICATION Data The principal objectives of classifying data are: to condense the mass of data in such a way that salient features can be readily noticed to facilitate comparisons between attributes of variables to prepare data which can be presented in tabular form to highlight the significant features of the data at a glance 2.4 TYPES OF CLASSIFICATION Some common types of classification are: 1) Geographical i.e., according to area or region. 2) Chronological, i.e., according to occurrence of an event with respect to time. 3) Qualitative, i.e., according to attributes. 4) Quantitative, i.e., according to magnitudes. Geographical Classification. In this type of classification, data is classified according to area or region. For example, when we consider production of wheat statewise, this would be called geographical classification. The listing of individual entries are generally done in an alphabetical order or according to size to emphasise the importance of a particular area or region. Chronological Classification. When the data is classified according to the time of its occurrence, it is known as chronological classification. For example, sales figure of a company for last six years are given below: Year Sales Year Sales (Rs. lakhs) (Rs. Iakhs) 1982-83 175 1985-86 485 1983-84 220 1986-87 565 1984-85 350 1987-88 620 Qualitative Classification. When the data is classified according to some attributes (distinct categories) which are not capable of measurement is known as qualitative classification. In a simple (or dichotomous) classification, an attribute is divided into two classes, one possessing the attribute and the other not possessing it. For example, we may classify population on the basis of employment, i.e., the employed and the unemployed. Similarly, we can have manifold classification when an attribute is divided so as to form several classes. For example, the attribute education can have different classes such as primary, middle, higher secondary, university, etc. Quantitative Classification. When the data is classified according to some characteristics that can be measured, it is called quantitative classification. For example, the employees of a company may be classified according to 19 Data Collection their monthly salaries. Since quantitative data is characterised by different and Analysis numerical values, the data represents the values of a variable. Quantitative data may be further classified into one or two types: discrete or continuous. The term discrete data refers to quantitative data that is limited to integer numerical values of a variable. For example, the number of employees in an organisation or the number of machines in a factory are examples of discrete data. Continuous data can take integer as well as fraction values of the variable. For example, the data relating to weight, distance, and volume are examples of continuous data. The quantitative classification becomes the basis for frequency distribution. When the data is arranged into groups or categories according to conveniently established divisions of the range of the observations, such an arrangement in tabular form is called a frequency distribution. In a frequency distribution, raw data is represented by distinct groups which are known as classes. The number of observations that fall into each of the classes is known as frequency. Thus, a frequency distribution has two parts, on its left there are classes and on its right there are frequencies. When data is described by a continuous variable it is called continuous data and when it is described by a discrete variable, it is called discrete data. The following are the two examples of discrete and continuous frequency distributions. Discrete frequency distribution Continuous frequency distribution No. of employees No. of companies Age (Years) No. of workers 110 25 20-25 15 120 35 25-30 22 130 70 30-35 38 140 100 35-40 47 150 18 40-45 18 160 12 45-50 10 Activity A What do you understand by classification of data? Why classification is necessary? ………………………………………………………………………………. ………………………………………………………………………………. ………………………………………………………………………………. ………………………………………………………………………………. ………………………………………………………………………………. ………………………………………………………………………………. 20 Activity B Presentation of Data With the help of a suitable example, illustrate the difference between qualitative and quantitative data. ………………………………………………………………………………. ………………………………………………………………………………. ………………………………………………………………………………. ………………………………………………………………………………. 2.5 CONSTRUCTION OF A DISCRETE FREQUENCY DISTRIBUTION The process of preparing a frequency distribution is very simple. In the case discrete data, place all possible values of the variable in ascending order in column, and then prepare another column of 'Tally' marks to count the number of times a particular value of the variable is repeated. To facilitate counting, block of five 'Tally' marks are prepared and some space is left in between the blocks. The frequency column refers to the number of 'Tally' marks, a particular class will contain. To illustrate the construction of a discrete frequency distribution, consider a sample study in which 50 families were surveyed to find the number of children per family. The data obtained are: 3 2 2 1 3 4 2 1 3 4 5 0 2 1 2 3 3 2 1 1 2 3 0 3 2 1 4 3 5 5 4 3 6 5 4 3 1 0 6 5 4 3 1 2 0 1 2 3 4 5 To condense this data into a discrete frequency distribution, we shall take the help of 'Tally' marks as shown below: No. of Children No. of families Frequency 0 IIII 4 1 IIII IIII 9 2 IIII IIII 10 3 IIII IIII II 12 4 IIII II 7 5 IIII I 6 6 II 2 Total 50 2.6 CONSTRUCTION OF A CONTINUOUS FREQUENCY DISTRIBUTION In constructing the frequency distribution for continuous data, it is necessary to clarify some of the important terms that are frequently used. 21 Data Collection Class Limits. Class limits denote the lowest and highest value that can be and Analysis included in the class. The two boundaries (i.e., lowest and highest) of a class are known as the lower limit and the upper limit of the class. For example, in the class 60-69, 60 is the lower limit and 69 is the upper limit or we can say that there can be no value if that class which is less than 60 and more than 69. Class Intervals. The class interval represents the width (span or size) of a class. The width may be determined by subtracting the lower limit of one class from the lower limit of the following class (alternatively successive upper limits may be used). For example, if the two classes are 10-20 and 20- 30, the width of the class interval would be the difference between the two successive lower limits, i.e., 20-10 = 10 or the difference between the upper limit and lower limit of the same class, i.e., 20-10 = 10. Class Frequency. The number of observations falling within a particular class is called its class frequency or simply frequency. Total frequency (sum of all the frequencies) indicate the total number of observations considered in a given frequency distribution. Class Mid-point. Mid-point of a class is defined as the sum of class limits divided by 2. Therefore, it is the value lying halfway between the lower and upper class limits. In the example taken above the mid-point would be (10+20)/2 = 15 corresponding to the class 10-20 and 25 corresponding to the class 20-30. Type of Class Interval. There are different ways in which limits of class intervals can be shown such as: i) Exclusive and Inclusive method, and ii) Open-end Exclusive Method. The class intervals are so arranged that the upper limit of one class is the lower limit of the next class. The following example illustrates this point. Sales No. of Sales No. of (Rs. thousands) firms (Rs. thousands) firms 20-25 20 35-40 27 25-30 28 40-45 12 30-35 35 45-50 8 In the above example there are 20 firms whose sales are between Rs.20,000 and Rs. 24,999. A firm with sales of exactly Rs. 25 thousand would be included in the next class viz. 25-30. Therefore in the exclusive method, it is always presumed that upper limit is excluded. Inclusive Method. In this method, the upper limit of one class is included in that class itself. The following example illustrates this point. Sales No. of Sales No. of (Rs. thousands) firms (Rs. thousands) firms 20-24.999 20 35-39.999 27 25-29.999 28 40-44.999 12 22 30-34.999 35 45-49.999 8 In this example, there are 20 firms whose sales are between Rs. 20,000 and Presentation of Data Rs. 24,999. A firm whose sales are exactly Rs. 25,000 would be included in the next class. Therefore in the inclusive method, it is presumed that upper limit is included. It may be observed that both the methods give the same class frequencies, although the class intervals look different. Whenever inclusive method is used for equal class intervals, the width of class intervals can be obtained by taking the difference between the two lower limits (or upper limits). Open-End. In an open-end distribution, the lower limit of the very first class and upper limit of the last class is not given. In distribution where there is a big gap between minimum and maximum values, the open-end distribution can be used such as in income distributions. The income disparities, of residents of a region may vary between Rs. 800 to Rs. 50,000 per month. In such a case, we can form classes like: Less than Rs. 1,000 1,000-2,000 2,000-5,000 5,000-10,000 10,000-25,000 25,000 and above Remark. To ensure continuity and to get correct class intervals, we shall adopt exclusive method. However, if inclusive method is suggested then it is necessary to make an adjustment to determine the class interval. This can be done by taking the average value of the difference between the lower limit of the succeeding class and the upper limit of the class. In terms of formula: Correction factor = This value so obtained is deducted from all lower limits and added to all upper limits. For instance, the example discussed for inclusive method can easily be converted into exclusive case. Take the difference between 25 and 24,999 and divide it by 2. Thus correction factor becomes (25-24,999)/2 = 0.0005. Deduct this value from lower limits and add it to upper limits. The new frequency distribution will take the following form: Presentation of Data Sales No. of Sales No. of (Rs thousand) firms (Rs thousand) firms 19.9995-24.9995 20 34.9995-39.9995 27 24 9995-29 9995 28 39 9995-44 9995 12 29.9995-34.9995 35 44.9995-49.9995 8 23 Data Collection and Analysis 2.7 GUIDELINES FOR CHOOSING THE CLASSES The following guidelines are useful in choosing the class intervals. 1) The number of classes should not be too small or too large. Preferably, the number of classes should be between 5 and 15. However, there is no hard and fast rule about it. If the number of observations is smaller, the number of classes formed should be towards the lower side of this limit and when the number of observations increase, the number of classes formed should be towards the upper side of the limit. 2) If possible, the widths of the intervals should be numerically simple like 5, 10, 25 etc. Values like 3, 7, 19 etc. should be avoided. 3) It is desirable to have classes of equal width. However, in case of distributions having wide gap between the minimum and maximum values, classes with unequal class interval can be formed like income distribution. 4) The starting point of a class should begin with 0, 5, 10 or multiples thereof. For example, if the minimum value is 3 and we are taking a class interval of 10, the first class should be 0-10 and not 3-13. 5) The class interval should be determined after taking into consideration the minimum and maximum values and the number of classes to be formed. For example, if the income of 20 employees in a company varies between Rs. 1100 and Rs. 5900 and we want to form 5 classes, the class interval should be 1000 5900 − 1100 = 4.8 5 1000 All the above points can be explained with the help of the following example wherein the ages of 50 employees are given: 22 21 37 33 28 42 56 33 32 59 40 47 29 65 45 48 55 43 42 40 37 39 56 54 38 49 60 37 28 27 32 33 47 36 35 42 43 55 53 48 29 30 32 37 43 54 55 47 38 62 In order to form the frequency distribution of this data, we take the difference between 60 and 21 and divide it by 10 to form 5 classes as follows: Age (Years) Tally Marks Frequency 20-30 IIII II 7 30-40 IIII IIII IIII I 16 40-50 IIII IIII IIII 15 50-60 IIII IIII 9 60-70 IIII 3 Total 50 24 Activity C Presentation of Data Distinguish between the following: i) Discrete and continuous frequency distributions. ii) Class limits and class intervals. iii) Inclusive and Exclusive method. ………………………………………………………………………………… ………………………………………………………………………………… ………………………………………………………………………………… ………………………………………………………………………………… ………………………………………………………………………………… ………………………………………………………………………………… ………………………………………………………………………………… ………………………………………………………………………………… ………………………………………………………………………………… ………………………………………………………………………………… 2.8 CUMULATIVE AND RELATIVE FREQUENCIES It is often useful to express class frequencies in different ways. Rather than listing the actual frequency opposite each class, it may be appropriate to list either cumulative, frequencies or relative frequencies or both. Cumulative Frequencies. As its name indicates, it cumulates the, frequencies, starting at either the lowest or highest value. The cumulative frequency of a given class interval thus represents the total of all the previous class frequencies including the class against which it is written. To illustrate the concept of cumulative frequencies, consider the following example Monthly salary No. of Monthly salary No. of (Rs.) employees (Rs.) employees 1000-1200 5 2000-2200 25 1200-1400 14 2200-2400 22 1400-1600 23 2400-2600 7 1600-1800 50 2600-2800 2 1800-2000 52 If we keep on adding the successive frequency of each class starting from the frequency of the very first class, we shall get cumulative frequencies as shown below: 25 Data Collection Monthly salary (Rs.) No. of employees Cumulative and Analysis 1000-1200 5 5 1200-1400 14 19 1400-1600 23 42 1600-1800 50 92 1800-2000 52 144 2000-2200 25 169 2200-2400 22 191 2400-2600 7 198 2600-2800 2 200 Total 200 Relative Frequencies. Very often, the frequencies in a frequency distribution are converted to relative frequencies to show the percentage for each class. If the frequency of each class is divided by the total number of observations (total frequency), then this proportion is referred to as relative frequency. To get the percentage for each class, multiply the relative frequency by 100; For the above example, the values computed for relative frequency and percentage are shown below: Monthly salary No. of Relative Percentage (Rs.) employees frequency 1000-1200 5 0.025 2.5 1200-1400 14 0.070 7.0 1400-1600 23 0.115 11.5 1600-1800 50 0.250 25.0 1800-2000 52 0.260 26.0 2000-2200 25 0.125 12.5 2200-2400 22 0.110 11.0 2400-2600 7 0.035 3.5 2600-2800 2 0.010 1.0 200 1.000 100% There are two important advantages in looking at relative frequencies (percentages) instead of absolute frequencies in a frequency distribution. 1) Relative frequencies facilitate the comparisons of two or more than two sets of data. 2) Relative frequencies constitute the basis of understanding the concept of probability. Activity D With the help of an example, explain the concept of relative frequency. 26 ………………………………………………………………………………… Presentation of Data ………………………………………………………………………………… ………………………………………………………………………………… ………………………………………………………………………………… 2.9 CHARTING OF DATA Charts of frequency distributions which cover both diagrams and graphs are useful because they enable a quick interpretation of the data. A frequency distribution can be presented by a variety of methods. In this section, the following four popular methods of charting frequency distribution are discussed in detail. i) Bar Diagram ii) Histogram iii) Frequency Polygon iv) Ogive or Cumulative Frequency Curve Bar Diagram. Bar diagrams are most popular. One can see numerous such diagrams in newspapers, journals, exhibitions, and even on television to depict different characteristics of data. For example, population, per capita income, sales and profits of a company can be shown easily through bar diagrams. It may be noted that a bar is a thick line whose width is shown to attract the viewer. A bar diagram may be either vertical or horizontal. In order to draw a bar diagram, we take the characteristic (or attribute) under consideration on the X-axis and the corresponding value on the Y-axis. It is desirable to mention the value depicted by the bar on the top of the bar. To explain the procedure of drawing a bar diagram, we have taken the population figures (in millions) of India which are given below: Bar Diagram 27 Data Collection Take the years on the X-axis and the population figure on the Y-axis and and Analysis draw a bar to show the population figure for the particular year. As can be seen from the diagram, the gap between one bar and the other bar is kept equal. Also the width of different bars is same. The only difference is in the length of the bars and that is why this type of diagram is also known as one dimensional. Histogram. One of the most commonly used and easily understood methods for graphic presentation of frequency distribution is histogram. A histogram is a series of rectangles having areas that are in the same proportion as the frequencies of a frequency distribution. To construct a histogram, on the horizontal axis or X-axis, we take the class limits of the variable and on the vertical axis or Y-axis, we take the frequencies of the class intervals shown on the horizontal axis. If the class intervals are of equal width, then the vertical bars in the histogram are also of equal width. On the other hand, if the class intervals are unequal, then the frequencies have to be adjusted according to the width of the class interval. To illustrate a histogram when class intervals are equal, let us consider the following example. Daily sales No. of Daily sales No. of (Rs. thousand) companies (Rs. thousand) companies 10-20 15 50-60 25 20-30 22 60-70 20 30-40 35 70-80 16 40-50 30 80-90 7 In this example, we may observe that class intervals are of equal width. Let us take class intervals on the X-axis and their corresponding frequencies on the Y-axis. On each class interval (as base), erect a rectangle with height equal to the frequency of that class. In this manner we get a series of rectangles each having a class interval as its width and the frequency as its height as shown below: Histogram with Equal Class Intervals 28 It should be noted that the area of the histogram represents the total Presentation of Data frequency as distributed throughout the different classes. When the width of the class intervals are not equal, then the frequencies must be adjusted before constructing the histogram. The following example will illustrate the procedure: Income (Rs.) No. of Income (Rs.) No. of employees 1000-1500 5 3500-5000 12 1500-2000 12 5000-7000 8 2000-2500 15 7000-8000 2 2500-3500 18 As can be seen, in the above example, the class intervals are of unequal width and hence we have to find out the adjusted frequency of each class by taking the class with the lowest class interval as the basis of adjustment. For example, in the class 2500-3500, the class interval is 1000 which is twice the size of the lowest class interval, i.e., 500 and therefore the frequency of this class would be divided by two, i.e., it would be 18/2 = 9. In a similar manner, the other frequencies would be obtained. The adjusted frequencies for various classes are given below: Income (Rs.) No. of Income (Rs.). No. of employees employees 1000-1500 5 4000-4500 4 1500-2000 12 4500-5000 4 2000-2500 15 5000-5500 2 2500-3000 9 5500-6000 2 3000-3500 9 6000-6500 2 3500-4000 4 6500-7000 2 7000-7500 1 7500-8000 1 The histogram of the above distribution is shown below: Histogram with Unequal Class Intervals 15 15 12 Number of Employees 10 9 5 5 4 2 1 1000 2000 3000 4000 5000 6000 7000 8000 Income (In Rupees) 29 Data Collection It may be noted that a histogram and a bar diagram look very much alike but and Analysis have distinct features. For example, in a histogram, the rectangles are adjoining and can be of different width whereas in bar diagram it is not possible. Activity E Draw a sketch of a histogram and a bar diagram and explain the difference between the two. ………………………………………………………………………………… ………………………………………………………………………………… ………………………………………………………………………………… ………………………………………………………………………………… ………………………………………………………………………………… Frequency Polygon. The frequency polygon is a graphical presentation of frequency distribution. A polygon is a many sided closed figure. Frequency Polygon 35 35 30 30 Number of Companies 25 25 22 20 20 15 16 15 10 7 5 0 10 20 30 40 50 60 70 80 90 100 Daily Sales (In Rupees) A frequency polygon is constructed by taking the mid-points of the upper horizontal side of each rectangle on the histogram and connecting these mid- points by straight lines. In order to close the polygon, an additional class is assumed at each end, having a zero frequency. If we draw a smooth curve over these points in such a way that the area included under the curve is approximately the same as that of the polygon, then such a curve is known as frequency curve. The following figure shows the same data smoothed out to form a frequency curve, which is another form of presenting the same data. 30 Frequency Curve Presentation of Data 35 30 Number of Companies 25 20 15 10 5 0 10 20 30 40 50 60 70 80 90 100 Daily Sales (In Rupees) Remark. The histogram is usually associated with discrete data and a frequency polygon is appropriate for continuous data. But this distinction is not always followed in practice and many factors may influence the choice of graph. The frequency polygon and frequency curve have a special advantage over the histogram particularly when we want to compare two or more frequency distributions. Activity F What is the procedure of making a frequency polygon? Illustrate with the help of suitable data. ………………………………………………………………………………… ………………………………………………………………………………… ………………………………………………………………………………… Ogives or Cumulative Frequency Curve. An ogive is the graphical presentation of a cumulative frequency distribution and therefore when the graph of such a distribution is drawn, it is called cumulative frequency curve or ogive. There are two methods of constructing ogive; viz., i) Less than ogive ii) More than ogive Less than Ogive. In this method, the upper limit of the various classes are taken on the X-axis and the frequencies obtained by the process of cumulating the preceding frequencies on the Y-axis. By joining these points we get less than ogive. Consider the example relating to daily sales discussed earlier. 31 Data Collection Daily sales No. of Daily sales No. of and Analysis (Rs. thousand) companies (Rs. thousand) companies 10-20 15 Less than 20 15 20-30 22 Less than 30 37 30-40 35 Less than 40 72 40-50 30 Less than 50 102 50-60 25 Less than 60 127 60-70 20 Less than 70 147 70-80 16 Less than 80 163 80-90 7 Less than 90 170 The less than Ogive Curve is shown below: Less than Ogive More than Ogive.Similarly more than ogive or cumulative frequency curve can be drawn by taking the lower limits on X-axis and cumulative frequencies on the Y-axis. By joining these points, we get more than ogive. The table and the curve for this case is shown below: Daily sales No. of Daily sales Cumulative (Rs, thousand) companies (Rs. thousand) frequency 10-20 15 More than 10 170 20-30 22 More than 20 155 30-40 35 More than 30 133 40-50 30 More than 40 98 50-60 25 More than 50 68 60-70 20 More than 60 43 70-80 16 More than 70 23 80-90 7 More than 80 7 The more than ogive curve is shown below: More than Ogive 32 Presentation of Data The shape of less than ogive curve would be a rising one whereas the shape of more than ogive curve should be falling one. The concept of ogive is useful in answering questions such as: How many companies are having sales less than Rs. 52,000 per day or more than Rs. 24,000 per day or between Rs. 24,000 and Rs. 52,000? Activity G With the help of an example, explain the concept of less than ogive and more than ogive. ………………………………………………………………………………… ………………………………………………………………………………… ………………………………………………………………………………… ………………………………………………………………………………… 2.10 SUMMARY Presentation of data is provided through tables and charts. A frequency distribution is the principal tabular summary of either discrete or continuous data. The frequency distribution may show actual, relative or cumulative frequencies. Actual and relative frequencies may be charted as either histogram (a bar chart) or a frequency polygon. Two graphs of cumulative frequencies are: less than ogive or more than ogive. 2.11 KEY WORDS Bar Chart is a thick line where the length of the bars should be proportional to the magnitude of the variable they present. Class Interval represents the width of a class. 33 Data Collection Class Limits denote the lowest and highest value that can be included in the and Analysis class. Continuous Data can take all values of the variable. Discrete Data refers to quantitative data that are limited to non-negative integral numerical values of a variable. Frequency Distribution is a tabular presentation where a number of observations with similar or closely related values are put in groups. Qualitative Data is characterised by exhaustive and distinct categories that do not possess magnitude. Quantitative Data possess the characteristic of numerical magnitude. 2.12 SELF-ASSESSMENT EXERCISES 1) Explain the purpose and methods of classification of data giving suitable examples. 2) What are the general guidelines of forming a frequency distribution with particular reference to the choice of class intervals and number of classes? 3) Explain the various diagrams and graphs that can be used for charting a frequency distribution. 4) What are ogives? Point out the role. Discuss the method of constructing ogives with the help of an example. 5) The following data relate to the number of family members in 30 families of a village. 4 3 2 3 4 5 5 7 3 2 3 4 2 1 1 6 3 4 5 4 2 7 3 4 5 6 2 1 5 3 Classify the above data in the form of a discrete frequency distribution. 6) The profits (Rs. lakhs) of 50 companies are given below: 20 12 15 27 28 40 42 35 37 43 55 65 53 62 29 64 69 36 25 18 56 55 43 35 26 21 48 43 50 67 14 23 34 59 68 22. 41 42 43 52 60 26 26 37 49 53 40 20 18 17 Classify the above data taking first class as 10-20 and form a frequency distribution. 7) The income (Rs.) of 24 employees of a company are given below: 1800 1250 1760 3500 6000 2500 2700 3600 3850 6600 3000 1500 4500 4400 3700 1900 1850 3750 6500 6800. 5300 2700 4370 3300 34 Form a continuous frequency distribution after selecting a suitable class Presentation of Data interval. 8) Draw a histogram and a frequency polygon from the following data: Marks No. of students Marks No. of students 0-20 8 60- 80 12 20-40 12 80-100 3 40-60 15 9) Go through the following data carefully and then construct a histogram. Income (Rs.) No. of Income (Rs.) No. of Persons persons. 500 1000 18 3000-4500 1000-1500 20 4500-5000 12 1500-2500 30 5000-7000 5 2500-3000 25 10 The following data relating to sales of 100 companies is given below: Sales No. of Sales No. of (Rs. lakhs) companies (Rs. lakhs) companies 5-10 5 25-30 18 10-15 12 30-35 15 15-20 13 35-40 10 20-25 20 40-45 7 Draw less than and more than 0 gives. Determine the number of companies whose sales are (i) less than Rs.13 lakhs (ii) more than 36 lakhs and (iii) between Rs. 13 lakhs and Rs. 36 lakhs. 2.13 FURTHER READINGS Clark, T.C. and E.W. Jordan. Introduction to Business and Economic Statistics, South-Western Publishing Co. Ohio, U.S.A. Enns, P.G., Business Statistics, Richard D. Irwin Inc.: Homewood. Gupta, S.P. and M.P. Gupta, Business Statistics, Sultan Chand & Sons.: New Delhi. Levin, R.I., Statistics for Management, Prentice-Hall of India: New Delhi. Moskowitz., H. and G.P. Wright. Statistics for Management and Economics, Charles. E. Merin Publishing Company: Ohio, U.S.A. Edward Tufte The Visual Display of Quantitative Information, Graphic Press. 35