UNIT-1 Understanding Basic Concepts of IoT (Internet of Things) PDF
Document Details
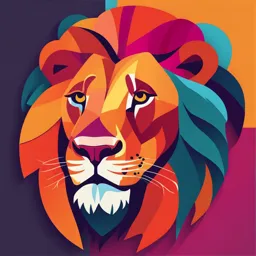
Uploaded by CatchyMars
Tags
Summary
This document provides an overview of the Internet of Things (IoT), covering its basic concepts, components, and applications. It explores the key elements of an IoT ecosystem, including devices, sensors, connectivity, data processing, and user interfaces. The document also delves into various applications like smart homes, healthcare, and industrial IoT, highlighting the challenges and future trends in IoT.
Full Transcript
UNIT-1 Understanding Basic Concepts of IoT (Internet of Things) Introduction to IoT The Internet of Things (IoT) refers to the interconnection of everyday devices to the internet, allowing them to send and receive data. This technological evolution facilitates enhanced communication between devi...
UNIT-1 Understanding Basic Concepts of IoT (Internet of Things) Introduction to IoT The Internet of Things (IoT) refers to the interconnection of everyday devices to the internet, allowing them to send and receive data. This technological evolution facilitates enhanced communication between devices, leading to smarter homes, cities, and industries. The IoT ecosystem includes various components, including devices, sensors, networks, and cloud services. Key Components of IoT 1\. Devices and Sensors Devices are the physical entities that interact with the environment. These can range from simple sensors (temperature, humidity, motion) to complex machines (smart appliances, industrial equipment). Sensors play a critical role in collecting data from the environment, which can then be transmitted to other devices or centralized systems for analysis. 2\. Connectivity Connectivity is vital for IoT devices to communicate. This can be achieved through various technologies, including:     Wi-Fi: Commonly used in home networks for connecting smart devices. Bluetooth: Suitable for short-range communication, often used in wearable technology. Cellular Networks: Enables IoT devices to connect over long distances, suitable for smart vehicles and remote monitoring. LPWAN(LowPowerWideAreaNetwork): Technologies like LoRaWAN and Sigfox allow for low-energy, long-range communication, ideal for battery-powered devices in agriculture and logistics. 3\. Data Processing Once data is collected from devices, it must be processed to derive meaningful insights. This can occur at various levels:   Edge Computing: Processing data near the source (i.e., at the device level) to reduce latency and bandwidth use. Cloud Computing: Centralized processing in the cloud, where vast amounts of data can be analyzed using advanced algorithms and machine learning. 4\. User Interface The user interface is how users interact with IoT systems. This can be through mobile apps, web applications, or voice-activated systems. A well-designed interface is crucial for user engagement and effective operation of IoT systems. HowIoTWorks The functioning of IoT can be broken down into several stages: 1\. Data Collection: Devices with sensors gather data from their environment. 2\. Data Transmission: The collected data is transmitted over a network to processing units, either in the cloud or at the edge. 3\. Data Processing and Analysis: The data is analyzed to extract useful information, identify patterns, and generate insights. 4\. Action: Based on the analysis, automated actions can be taken, or alerts can be sent to users for manual intervention. Applications of IoT The applications of IoT are vast and span multiple domains: 1\. Smart Homes IoT enables home automation, allowing users to control lighting, heating, security systems, and appliances remotely. Smart speakers like Amazon Echo and Google Home integrate with various devices, enhancing convenience and energy efficiency. 2\. Healthcare Wearable devices monitor health metrics such as heart rate and activity levels, sending real-time data to healthcare providers. Remote patient monitoring can lead to early detection of health issues and personalized treatment plans. 3\. Industrial IoT (IIoT) In manufacturing, IoT facilitates predictive maintenance, reducing downtime by analyzing machine performance data. IIoT applications enhance supply chain management, inventory tracking, and overall operational efficiency. 4\. Smart Cities IoT contributes to the development of smart cities by optimizing traffic management, waste management, and energy consumption. Smart streetlights, parking sensors, and environmental monitoring systems are examples of IoT applications in urban environments. 5\. Agriculture IoT in agriculture allows for precision farming, where sensors monitor soil conditions, crop health, and weather patterns. This data helps farmers optimize irrigation, fertilization, and pest control, leading to increased yields and sustainability. Challenges in IoT Despite its potential, IoT faces several challenges: 1\. Security As more devices connect to the internet, the risk of cyber-attacks increases. Ensuring data privacy and security through encryption, authentication, and regular updates is critical. 2\. Interoperability With a myriad of devices and protocols, achieving seamless communication between different IoT systems remains a challenge. Standardization is needed to ensure compatibility. 3\. Data Management The vast amounts of data generated by IoT devices can overwhelm systems. Efficient data storage, processing, and analysis methods are necessary to extract meaningful insights. 4\. Scalability As IoT adoption grows, systems must be scalable to accommodate the increasing number of devices without compromising performance. Future of IoT The future of IoT is promising, with advancements in artificial intelligence, machine learning, and 5G connectivity expected to enhance its capabilities. Key trends include: 1\. AI and Machine Learning Integration AI will enable more sophisticated data analysis, leading to predictive capabilities and improved decision making. 2\. Increased Adoption of 5G The rollout of 5G networks will enhance connectivity, allowing for faster data transmission and supporting a greater number of devices. 3\. Focus on Sustainability IoT solutions will play a significant role in sustainability efforts, optimizing resource use, reducing waste, and monitoring environmental conditions. 4\. Enhanced Security Measures With rising cyber threats, the development of advanced security protocols and frameworks will be essential to protect IoT ecosystems. Consumer IoT vs. Industrial Internet of Things (IIoT) The Internet of Things (IoT) is a transformative technology that connects devices to the internet, enabling data exchange and intelligent decision-making. It can be broadly categorized into two segments: Consumer IoT and Industrial Internet of Things (IIoT). While both share core principles, they differ significantly in applications, technology, and impact. This document delves into the details of both domains, highlighting their differences and implications. Understanding Consumer IoT Definition Consumer IoT refers to the network of everyday devices used by individuals and households. These devices are typically designed to improve convenience, enhance user experiences, and automate daily tasks. Key Characteristics 1\. User-Centric: Designed primarily for personal use, focusing on enhancing comfort and lifestyle. 2\. Ease of Use: Interfaces are intuitive, allowing users of varying technical skills to operate them without extensive training. 3\. Integration: Consumer IoT devices often integrate with smartphones and home automation systems, creating seamless user experiences. Examples    Smart Home Devices: Thermostats (e.g., Nest), lighting systems (e.g., Philips Hue), and security cameras (e.g., Ring). Wearable Technology: Fitness trackers (e.g., Fitbit) and smartwatches (e.g., Apple Watch). Smart Appliances: Refrigerators, ovens, and washing machines that can be controlled via mobile apps. Applications 1\. HomeAutomation: Controlling lights, temperature, and security systems remotely. 2\. Health Monitoring: Tracking physical activity and vital signs to promote wellness. 3\. Energy Management: Optimizing energy consumption through smart meters and connected appliances. Challenges    Security and Privacy: Many consumer IoT devices lack robust security measures, making them susceptible to hacking. Interoperability: A diverse array of devices and platforms can lead to compatibility issues. Data Management: Managing and analyzing the data generated by consumer devices poses challenges, especially concerning privacy. Understanding Industrial IoT (IIoT) Definition Industrial IoT refers to the use of IoT technologies in industrial sectors such as manufacturing, energy, transportation, and logistics. IIoT focuses on optimizing processes, enhancing productivity, and improving operational efficiency. Key Characteristics 1\. Business-Centric: Designed for enterprise applications, focusing on improving operational efficiency and safety. 2\. Complex Systems: Often involves intricate machinery and large-scale networks, requiring specialized knowledge to operate and maintain. 3\. Reliability and Scalability: Systems must be robust and capable of scaling to handle vast amounts of data and devices. Examples    Predictive Maintenance: Sensors on machinery monitor performance, predicting failures before they occur. Supply Chain Management: RFID tags and GPS devices track inventory and shipments in real time. Smart Grids: Electricity grids that utilize IoT technology to manage energy distribution and consumption. Applications 1\. Manufacturing Automation: Streamlining production lines and reducing downtime through real time monitoring. 2\. Asset Tracking: Monitoring the location and condition of equipment and materials. 3\. Energy Management: Optimizing energy use in factories and reducing operational costs. Challenges    Security Risks: Industrial systems are prime targets for cyber-attacks, necessitating robust security measures. Integration with Legacy Systems: Many industries rely on outdated technologies that can be challenging to integrate with new IoT solutions. Data Overload: Managing vast amounts of data generated by IIoT devices requires advanced analytics and storage solutions. Comparative Analysis 1\. Purpose   Consumer IoT: Aims to enhance individual lifestyle and convenience. IIoT: Focuses on improving industrial efficiency, safety, and productivity. 2\. User Base   Consumer IoT: End-users are typically individuals or families. IIoT: Users include businesses, manufacturers, and industries. 3\. Complexity   Consumer IoT: Generally simpler devices with user-friendly interfaces. IIoT: More complex systems that may require specialized training for operation and maintenance. 4\. Data Volume   Consumer IoT: Generates moderate amounts of data, often for personal use. IIoT: Produces vast volumes of data that require sophisticated processing and analytics. 5\. Security Measures   Consumer IoT: Often lacks robust security, leading to potential vulnerabilities. IIoT: Security is critical, with businesses investing heavily in cybersecurity measures. 6\. Return on Investment (ROI)  Consumer IoT: ROI may be less quantifiable, focusing on convenience and quality of life. IIoT: ROI is often measurable through increased efficiency, reduced downtime, and cost savings.  Trends in Both Domains Consumer IoT Trends 1\. Smart Home Integration: Increased adoption of platforms like Amazon Alexa and Google Home to control multiple devices. 2\. Health and Wellness: Growth in wearable devices that monitor health metrics. 3\. Data Privacy Awareness: Consumers becoming more aware of data privacy issues, leading to demand for transparent practices. IIoT Trends 1\. AIandMachine Learning: Utilizing advanced analytics to derive insights from data and enhance predictive maintenance. 2\. 5GConnectivity: Enhancing communication speed and reliability, allowing for real-time monitoring and control. 3\. Sustainability: Increased focus on energy efficiency and reducing carbon footprints through IoT solutions. Fundamental Building Blocks of IoT (Internet of Things) The Internet of Things (IoT) is a rapidly growing network of connected devices that communicate and exchange data. Understanding the fundamental building blocks of IoT is essential for grasping how it functions, its architecture, and the various components that make it operational. This detailed overview will cover the key elements that constitute the IoT ecosystem. 1\. Devices and Sensors Definition Devices and sensors are the core components of IoT. They collect data from the environment or perform specific tasks based on user input or pre-defined conditions. Types of Devices    Sensors: These are devices that detect changes in the environment. Common types include temperature sensors, humidity sensors, motion detectors, and light sensors. Actuators: Actuators are devices that can take action based on data received. Examples include motors, valves, and robotic arms. Smart Devices: These are everyday objects embedded with sensors and connectivity features, such as smart thermostats, wearables, and connected appliances. Functionality Devices and sensors work by capturing data and converting it into a format that can be transmitted over a network. For example, a temperature sensor measures ambient temperature and sends this data to a cloud server for analysis. 2\. Connectivity Definition Connectivity refers to the means through which devices communicate with each other and with centralized systems. It is a critical component of the IoT architecture. Types of Connectivity      Wi-Fi: Widely used in homes and offices, allowing devices to connect to local networks and the internet. Bluetooth: Suitable for short-range communication, often used in wearables and smart home devices. Cellular Networks: 3G, 4G, and 5G networks provide connectivity for devices over long distances, ideal for mobile and remote applications. LPWAN(LowPowerWideAreaNetwork): Technologies like LoRaWAN and Sigfox enable low power, long-range communication, perfect for battery-operated devices in smart agriculture or logistics. Zigbee and Z-Wave: These are specialized protocols designed for low-power, low-bandwidth communication, commonly used in home automation. Importance The choice of connectivity method impacts device performance, battery life, and range. Efficient connectivity ensures reliable data transmission and responsiveness of IoT applications. 3\. Data Processing Definition Data processing involves analyzing the data collected from devices to extract useful information and insights. Levels of Processing   Edge Computing: Data is processed close to where it is generated (at the device level) to reduce latency and bandwidth usage. This is particularly useful for applications requiring real-time responses, such as industrial automation and smart transportation. Cloud Computing: Data is sent to centralized cloud servers for storage and analysis. Cloud computing can handle vast amounts of data, allowing for complex analytics and machine learning applications. Technologies Used  Data Analytics Tools: Software applications that analyze data for trends, patterns, and insights. Tools like Apache Hadoop, Apache Spark, and various machine learning platforms are commonly used.  Database Management Systems: These systems store and manage data collected from IoT devices. Examples include SQL databases, NoSQL databases, and time-series databases. Significance Efficient data processing enables informed decision-making, predictive analytics, and enhanced automation in various applications, from smart homes to industrial settings. 4\. User Interface Definition The user interface (UI) is how users interact with IoT systems. A well-designed UI is crucial for the effective use of IoT devices. Types of User Interfaces    Mobile Applications: Many IoT devices offer companion apps that allow users to control settings, monitor performance, and receive alerts. WebDashboards: Web-based interfaces provide a comprehensive view of data and device status, often used in industrial settings for monitoring and management. Voice Interfaces: Smart assistants (like Amazon Alexa and Google Assistant) allow users to control IoT devices using voice commands, enhancing accessibility. Importance An intuitive UI improves user engagement and satisfaction. It enables users to maximize the benefits of their IoT systems and simplifies complex operations. 5\. Cloud and Edge Infrastructure Definition The cloud and edge infrastructure refers to the frameworks that support data storage, processing, and management for IoT systems. Cloud Infrastructure  Storage: Cloud storage solutions provide scalable storage for large volumes of IoT data, allowing for easy access and management.  Computing Power: Cloud platforms offer computational resources for data analysis and machine learning, enabling advanced analytics without the need for local processing. Edge Infrastructure   Edge Devices: These are localized computing resources that process data closer to the source, reducing latency and bandwidth usage. Gateways: Gateways serve as intermediaries between IoT devices and the cloud, managing data traffic and sometimes providing preliminary data processing. Significance Arobust cloud and edge infrastructure ensures seamless data flow, enhances security, and improves response times for IoT applications. 6\. Security Definition Security in IoT encompasses measures taken to protect devices, networks, and data from unauthorized access and cyber threats. Key Security Measures    Data Encryption: Encrypting data during transmission ensures that sensitive information is protected from interception. Authentication: Ensuring that devices and users are who they claim to be through mechanisms like passwords, biometrics, or two-factor authentication. Regular Updates: Keeping firmware and software up-to-date to protect against vulnerabilities and exploits. Importance As IoT systems expand, security becomes paramount. Protecting data and devices helps maintain user trust and prevents potential financial and reputational damage. 7\. Protocols and Standards Definition Protocols and standards define how devices communicate, ensuring interoperability and seamless integration within the IoT ecosystem. CommonProtocols    MQTT(Message Queuing Telemetry Transport): A lightweight messaging protocol designed for low-bandwidth, high-latency networks, commonly used in IoT applications. HTTP/HTTPS: Widely used for web-based applications and APIs, providing a standard for data exchange. CoAP(Constrained Application Protocol): Designed for low-power devices, CoAP is a web transfer protocol for constrained nodes and networks. Importance Adopting common protocols and standards fosters interoperability, allowing devices from different manufacturers to work together effectively, which is crucial for a cohesive IoT ecosystem. Use Cases of IoT in Various Industry Domains The Internet of Things (IoT) has emerged as a transformative force across multiple industries, revolutionizing processes, enhancing efficiencies, and creating new business models. This detailed overview will explore various use cases of IoT across different industry domains, highlighting how connected devices and data-driven insights are reshaping operations. 1\. Healthcare Remote Patient Monitoring IoT-enabled devices, such as wearables and health monitors, allow healthcare providers to track patients' vital signs in real time. This technology enables early detection of potential health issues, reduces the need for hospital visits, and supports chronic disease management. Smart Hospitals IoT can enhance hospital management through smart beds that monitor patient occupancy and conditions, asset tracking for medical equipment, and environmental controls for optimizing energy use. These applications lead to improved patient care and operational efficiency. Telemedicine IoT facilitates telemedicine services, enabling doctors to consult with patients remotely. Connected devices can transmit health data during virtual visits, ensuring informed decision-making and timely interventions. 2\. Manufacturing Predictive Maintenance IoT sensors can monitor machinery and equipment, predicting failures before they occur. By analyzing performance data, manufacturers can schedule maintenance proactively, minimizing downtime and reducing repair costs. Smart Manufacturing IoT allows for real-time monitoring of production lines, enabling manufacturers to optimize operations. Data-driven insights can enhance supply chain management, inventory control, and quality assurance, leading to increased efficiency and reduced waste. Asset Tracking IoT solutions can track the location and status of tools, machinery, and inventory within manufacturing facilities. This visibility helps in managing assets effectively, reducing losses, and improving productivity. 3\. Agriculture Precision Farming IoT technologies enable precision farming by providing data on soil conditions, weather patterns, and crop health. Farmers can use this information to optimize irrigation, fertilization, and pest management, resulting in increased yields and resource conservation. Livestock Monitoring Connected devices can monitor livestock health, location, and behavior. IoT sensors can track vital signs and alert farmers to potential health issues, enabling timely interventions and improving animal welfare. Smart Irrigation Systems IoT-enabled irrigation systems use sensors to monitor soil moisture levels and weather forecasts, automating watering schedules. This approach conserves water and optimizes crop growth. 4\. Transportation and Logistics Fleet Management IoT devices in vehicles can monitor location, speed, and fuel consumption. Fleet managers can use this data for route optimization, reducing operational costs and improving delivery times. Smart Supply Chains IoT solutions can enhance supply chain visibility by tracking shipments in real time. Sensors can monitor conditions during transit (e.g., temperature, humidity), ensuring that perishable goods are delivered safely. Traffic Management Smart cities utilize IoT to manage traffic flow efficiently. Connected traffic lights, road sensors, and real time data analytics can reduce congestion, improve public transportation, and enhance road safety. 5\. Retail Smart Shelves IoT-enabled smart shelves can monitor inventory levels and product placement. Retailers receive real-time alerts when stock runs low, optimizing inventory management and reducing out-of-stock situations. Personalized Marketing Retailers can leverage IoT data to understand customer preferences and shopping behaviors. This information enables targeted marketing campaigns and personalized shopping experiences, enhancing customer engagement. Supply Chain Optimization IoT technologies help retailers manage their supply chains more effectively by providing real-time visibility into inventory and shipments, allowing for improved demand forecasting and reduced lead times. 6\. Smart Cities Waste Management IoT solutions enable smart waste management systems that monitor waste levels in bins and optimize collection routes. This approach reduces costs and environmental impact while improving service efficiency. Smart Lighting Connected streetlights can adjust brightness based on traffic and pedestrian presence. This not only saves energy but also enhances safety and security in urban areas. Environmental Monitoring IoT sensors can track air quality, noise levels, and other environmental factors in real time. City officials can use this data to implement policies aimed at improving public health and quality of life. 7\. Energy and Utilities Smart Grids IoT technologies enhance the management of electricity distribution networks through smart meters and sensors. These systems provide real-time data on energy consumption and grid health, enabling more efficient energy distribution and reduced outages. DemandResponse Programs IoT allows utilities to implement demand response programs, encouraging consumers to reduce energy use during peak times. This can stabilize the grid and lead to cost savings for both utilities and consumers. Asset Management Utilities can monitor the condition of infrastructure (e.g., pipelines, transformers) using IoT sensors. Predictive maintenance based on this data can prevent failures and extend the life of critical assets. 8\. Financial Services Fraud Detection IoT devices can enhance security in financial transactions by providing real-time data on user behavior. Anomalies can trigger alerts, allowing for immediate action against potential fraud. Smart Banking Banks are utilizing IoT technologies to improve customer experiences through personalized services and location-based offers. Smart ATMs can also monitor usage patterns and optimize cash management. Asset Tracking IoT solutions can track physical assets, such as vehicles or equipment, for leasing companies and insurers. This visibility helps mitigate risks and streamline operations. UNIT-2 IoT ReferenceArchitectures: A Detailed Overview The Internet of Things (IoT) represents a transformative approach to connecting devices, systems, and services to improve efficiency, decision-making, and user experiences. A well-defined reference architecture provides a framework that outlines the components and interactions within an IoT system, serving as a blueprint for designing and implementing IoT solutions. This document will delve into the various layers of IoT reference architectures, their components, and the importance of each layer. What is an IoT Reference Architecture? An IoT reference architecture serves as a standardized framework that guides the design and implementation of IoT systems. It defines the key components, their interactions, and the overall structure of the IoT ecosystem. By providing a common understanding of how various elements work together, reference architectures facilitate interoperability, scalability, and integration among diverse IoT solutions. Key Layers of IoT Reference Architectures IoT reference architectures typically consist of several layers, each playing a crucial role in the overall functionality of the system. These layers can vary depending on the specific architecture model, but the most common layers include: 1\. Perception Layer (Device Layer) Definition The perception layer, often referred to as the device layer, is the foundation of any IoT architecture. It consists of sensors, actuators, and other devices that collect data from the environment and perform actions based on that data. Components  Sensors: Devices that capture data related to physical phenomena, such as temperature, humidity, light, motion, and more.  Actuators: Devices that carry out actions based on commands received from other components. Examples include motors, valves, and robotic arms.  SmartDevices: Consumer and industrial devices equipped with embedded IoT capabilities, such as smart thermostats and connected machinery. Importance The perception layer is essential for gathering real-time data, enabling intelligent responses, and providing insights for decision-making processes. Without this layer, an IoT system would lack the ability to interact with the physical world. 2\. Network Layer Definition The network layer is responsible for transmitting data collected by the devices to other layers for processing and analysis. It establishes communication between devices, gateways, and cloud services. Components  Communication Protocols: Various protocols facilitate communication between devices, such as MQTT, CoAP, HTTP, and WebSocket.  Gateways: Devices that act as intermediaries between IoT devices and cloud services. They can perform data aggregation, filtering, and protocol translation.  Networking Technologies: Includes Wi-Fi, Bluetooth, Zigbee, LPWAN (LoRa, Sigfox), and cellular networks, enabling diverse connectivity options. Importance The network layer ensures reliable and efficient data transmission, enabling real-time communication and interaction between devices and systems. It plays a critical role in ensuring data integrity and security during transmission. 3\. Edge Computing Layer Definition The edge computing layer refers to processing data closer to the source (i.e., at the device level or near the edge of the network) rather than sending all data to the cloud for processing. Components  EdgeDevices: Smart gateways and local servers that perform data processing, analytics, and storage.  DataFiltering and Preprocessing: Techniques that reduce the amount of data sent to the cloud, enhancing efficiency by filtering out irrelevant or redundant data. Importance Edge computing minimizes latency, reduces bandwidth consumption, and enhances response times, making it particularly valuable for applications requiring real-time processing, such as autonomous vehicles and industrial automation. 4\. Data Accumulation Layer Definition The data accumulation layer is responsible for storing and managing the data collected from devices and processed at the edge. It serves as a repository for both real-time and historical data. Components  DatabaseSystems: Includes time-series databases, NoSQL databases, and traditional relational databases designed to store large volumes of structured and unstructured data.  DataLakes:Centralized repositories that allow storage of vast amounts of raw data in its native format until needed for analysis. Importance This layer is crucial for data management, enabling efficient storage, retrieval, and analysis of information. It supports historical analysis, reporting, and decision-making processes across various applications. 5\. Data Processing and Analytics Layer Definition The data processing and analytics layer transforms raw data into meaningful insights through analysis, visualization, and reporting. Components  DataAnalytics Tools: Software solutions that perform data analysis, such as Apache Spark, Apache Flink, and machine learning platforms.  Business Intelligence Tools: Applications that provide dashboards, reports, and visualization tools to present analyzed data in an understandable format. Importance By extracting valuable insights from data, this layer enables organizations to make informed decisions, optimize processes, and enhance user experiences. Predictive analytics, anomaly detection, and trend analysis are common applications in this layer. 6\. Application Layer Definition The application layer consists of software applications and services that utilize the insights derived from the previous layers to deliver specific functionalities to end-users. Components  IoTApplications: Custom applications that cater to specific industry needs, such as smart home applications, industrial automation software, and healthcare monitoring systems.  UserInterfaces: Mobile apps, web applications, and dashboards that allow users to interact with the IoT system, visualize data, and control devices. Importance This layer serves as the point of interaction for users and businesses, enabling them to leverage IoT capabilities to enhance productivity, efficiency, and overall user satisfaction. 7\. Security Layer Definition The security layer encompasses all measures and protocols implemented to protect data, devices, and networks from unauthorized access and cyber threats. Components  DataEncryption: Techniques to secure data in transit and at rest, ensuring confidentiality and integrity.  Authentication and Authorization: Mechanisms that verify user identities and control access to devices and data.  Intrusion Detection Systems: Tools that monitor network traffic for suspicious activities, providing alerts in case of potential security breaches. Importance Security is a critical aspect of any IoT architecture, as connected devices are vulnerable to cyberattacks. Implementing robust security measures helps protect sensitive data and maintain user trust. Benefits of IoT Reference Architectures 1. 2. 3. 4. 5. Standardization: Reference architectures provide a standardized framework, reducing complexity in design and implementation. Interoperability: By defining common protocols and standards, IoT reference architectures enable devices from different manufacturers to communicate seamlessly. Scalability: Well-defined architectures support the easy addition of new devices and functionalities, accommodating growth and technological advancements. Simplified Development: Developers can leverage existing reference architectures to streamline the development process, reducing time-to-market for IoT solutions. Enhanced Security: With a focus on security across all layers, reference architectures help mitigate risks associated with IoT deployments. Industrial Internet Reference Architecture: A Detailed Overview The Industrial Internet of Things (IIoT) is transforming industries by integrating physical machines with digital technologies, enabling real-time data analytics and decision-making. A robust Industrial Internet Reference Architecture (IIRA) provides a framework for understanding the components, layers, and interactions within IIoT systems. This document outlines the key elements of IIoT reference architecture, its layers, and its significance in various industrial applications. What is Industrial Internet Reference Architecture? The Industrial Internet Reference Architecture (IIRA) is a standardized framework developed by the Industrial Internet Consortium (IIC) that guides the design and implementation of IIoT solutions. It outlines the roles of different components, the flow of data, and the necessary interoperability between devices, systems, and applications. The IIRA aims to facilitate the integration of devices, analytics, and services across industrial sectors, ensuring scalability, security, and efficient operation. Key Components of IIoT Reference Architecture The IIRA comprises several key components that can be categorized into four main areas: 1. 2. 3. 4. Devices and Sensors Communication and Connectivity Data Processing and Analytics Applications and Services 1\. Devices and Sensors Definition The devices and sensors layer includes the physical components that gather data from the industrial environment. These elements form the foundation of any IIoT system. Components  Sensors: Devices that measure various physical parameters, such as temperature, pressure, humidity, and vibration. They collect real-time data critical for monitoring equipment and processes.  Actuators: Mechanisms that perform actions based on data received. For example, actuators can control valves, motors, or other equipment in response to analytics or user commands.  EdgeDevices: Devices that process data locally before sending it to the cloud or central systems. This reduces latency and bandwidth usage, enabling real-time decision-making. Importance The effectiveness of an IIoT system hinges on the quality and accuracy of the data collected. Reliable devices and sensors enable real-time monitoring, predictive maintenance, and improved operational efficiencies. 2\. Communication and Connectivity Definition This layer encompasses the technologies and protocols used to facilitate communication between devices, systems, and applications. Components  Communication Protocols: Standards that dictate how devices communicate. Common protocols in IIoT include MQTT, CoAP, OPCUA, and HTTP/HTTPS.  Networking Technologies: Various technologies enable connectivity, such as Ethernet, Wi-Fi, cellular networks (4G, 5G), LPWAN (Low Power Wide Area Networks), and industrial protocols like CAN bus and Modbus.  Gateways: Devices that connect IoT devices to the cloud or enterprise systems. They can perform data aggregation, filtering, and protocol translation, ensuring seamless communication across different systems. Importance Effective communication and connectivity are vital for the success of IIoT systems. They ensure that data flows smoothly between devices and centralized systems, enabling timely decision-making and responsive actions. 3\. Data Processing and Analytics Definition The data processing and analytics layer is responsible for transforming raw data into actionable insights through analysis and visualization. Components  DataProcessing Frameworks: Systems that process and analyze data, such as Apache Spark, Apache Flink, and other big data technologies.  EdgeAnalytics: Processing performed at the edge of the network to reduce latency and bandwidth usage, allowing for immediate insights and actions.  CloudAnalytics: Advanced analytics conducted in the cloud, where large volumes of data can be processed for deeper insights, machine learning, and predictive analytics. Importance Data processing and analytics are at the heart of IIoT. By turning raw data into meaningful insights, organizations can optimize processes, improve maintenance strategies, and enhance overall operational efficiency. 4\. Applications and Services Definition The applications and services layer encompasses software solutions that leverage data from IIoT systems to deliver specific functionalities and value to users. Components  Industry-Specific Applications: Tailored software solutions designed for specific industries, such as predictive maintenance applications, asset management systems, and energy management tools.  UserInterfaces: Dashboards, mobile applications, and web interfaces that allow users to visualize data, monitor systems, and control devices.  CloudServices: Platforms that provide storage, analytics, and other services essential for IIoT applications. Importance This layer provides the end-users with the tools they need to make informed decisions based on data insights. User-friendly interfaces and tailored applications enhance usability and drive adoption across industries. Layers of IIoT Reference Architecture The IIoT reference architecture can also be visualized in terms of distinct layers, each with its own role and responsibilities: 1\. Perception Layer This layer encompasses the devices and sensors that gather data from the physical environment. It includes:  Sensorsand Actuators: For data collection and action execution.  EdgeDevices: For initial data processing. 2\. Network Layer This layer handles communication and connectivity. It includes:  Gateways: Tobridge devices and networks.  Communication Protocols: To ensure seamless data transmission. 3\. Edge Layer The edge layer focuses on processing data close to its source. It includes:  EdgeAnalytics: For immediate insights and actions.  LocalDataStorage: To reduce reliance on cloud storage for real-time applications. 4\. Data Accumulation Layer This layer is responsible for data storage and management. It includes:  DataLakes:For storing vast amounts of raw data.  Databases: For structured and unstructured data management. 5\. Analytics Layer The analytics layer is dedicated to processing and analyzing data to derive insights. It includes:  AdvancedAnalytics Tools: For predictive analytics and machine learning.  Visualization Tools: To present data in a user-friendly format. 6\. Application Layer The application layer provides end-user access to IIoT functionalities. It includes:  Industry-Specific Applications: To address unique business needs.  UserInterfaces: For interaction with the system. 7\. Security Layer This layer encompasses all security measures. It includes:  DataEncryption: To protect data in transit and at rest.  AccessControl Mechanisms: To ensure only authorized users can access data and systems. Benefits of IIoT Reference Architecture 1. 2. 3. 4. 5. Standardization: Provides a common framework for designing IIoT systems, facilitating interoperability and compatibility among devices and systems. Scalability: Supports the addition of new devices, functionalities, and applications, accommodating growth and technological advancements. Enhanced Security: Incorporates security best practices at every layer, helping to mitigate risks associated with cyber threats. Faster Time-to-Market: By utilizing predefined architectures, organizations can accelerate the development process and reduce deployment times. Improved Decision-Making: Enables organizations to leverage real-time data and insights, leading to better-informed decisions and optimized operations. Edge Computing:ADetailed Overview Edge computing is a transformative paradigm in the realm of data processing and networking, addressing the limitations of traditional cloud computing. As the Internet of Things (IoT) continues to expand, the need for efficient data management and real-time processing becomes critical. This document will explore the key concepts, architecture, benefits, challenges, and use cases of edge computing. What is Edge Computing? Edge computing refers to the practice of processing data closer to the source of data generation rather than relying solely on centralized cloud data centers. By moving computation and storage closer to the "edge" of the network---where devices and sensors are located---edge computing enables faster data processing, reduced latency, and improved bandwidth efficiency. Key Characteristics of Edge Computing 1. 2. 3. 4. Proximity to Data Sources: Edge computing operates near the devices generating data, allowing for real-time processing. Reduced Latency: By minimizing the distance data must travel, edge computing significantly reduces response times, which is crucial for applications requiring immediate action. Efficient Bandwidth Utilization: Processing data locally reduces the amount of data transmitted to the cloud, optimizing bandwidth use and lowering operational costs. Enhanced Data Security: Edge computing can enhance security by keeping sensitive data closer to its source, reducing exposure during transmission. Architecture of Edge Computing Edge computing architecture typically comprises several layers, each contributing to the overall functionality and efficiency of the system. The main layers include: 1\. Device Layer This layer consists of the various devices and sensors that generate data. Common devices include:  IoTDevices: Smart sensors, cameras, and actuators that collect data from the environment.  EdgeDevices: Local gateways and servers that perform initial data processing and analysis. 2\. Edge Layer The edge layer is responsible for processing data at or near the source. Key components include:  EdgeGateways: Devices that connect IoT devices to the broader network, performing tasks such as data aggregation, filtering, and preliminary analysis.  EdgeAnalytics: Software applications that analyze data locally to extract actionable insights and make real-time decisions. 3\. Network Layer This layer facilitates communication between edge devices, cloud services, and centralized data centers. Key components include:  Networking Protocols: Standards that enable communication between devices, such as MQTT, CoAP, and HTTP.  Connectivity Options: Various technologies, including Wi-Fi, cellular, LPWAN, and Ethernet, that facilitate data transfer. 4\. Cloud Layer While edge computing emphasizes local processing, the cloud layer remains integral for:  DataStorage: Storing large volumes of historical data for analysis and reporting.  AdvancedAnalytics: Performing more complex data analysis that requires substantial computational resources. 5\. Application Layer The application layer consists of the software solutions that leverage insights from both edge and cloud processing to deliver specific functionalities. This includes:  Industry-Specific Applications: Tailored applications for various sectors, such as smart manufacturing, healthcare, and transportation.  UserInterfaces: Dashboards and mobile apps that allow users to monitor systems and interact with data. Benefits of Edge Computing Edge computing offers several advantages that make it an attractive solution for modern data processing needs:  LowLatency:Byprocessing data close to its source, edge computing significantly reduces latency, enabling real-time applications such as autonomous vehicles and industrial automation.  ImprovedBandwidth Efficiency: By filtering and processing data locally, only essential information is sent to the cloud, reducing bandwidth usage and associated costs.  EnhancedReliability: Edge computing systems can continue to operate even with intermittent connectivity to the cloud, ensuring consistent performance in remote or challenging environments.  Increased Security: Keeping sensitive data closer to its source can enhance security measures, reducing the risk of data breaches during transmission.  Scalability: Edge computing can easily scale to accommodate increasing numbers of devices and data sources without overwhelming centralized systems. Challenges of Edge Computing Despite its numerous benefits, edge computing also presents several challenges:  Complexity in Management: Managing a distributed network of edge devices can be more complex than traditional centralized systems, requiring robust monitoring and management tools.  DataSecurity Risks: While edge computing can enhance security, it also introduces new vulnerabilities, as multiple edge devices can become potential targets for cyberattacks.  Standardization Issues: The lack of universally accepted standards for edge computing can lead to interoperability issues between devices and systems from different vendors.  ResourceConstraints: Edge devices often have limited computational power and storage capacity compared to cloud data centers, which can restrict the complexity of the processing that can be performed locally.  NetworkReliability: While edge computing can function with intermittent cloud connectivity, reliance on stable network connections is still crucial for optimal performance. Use Cases of Edge Computing Edge computing is applied across various industries and use cases, demonstrating its versatility and effectiveness: 1\. Smart Manufacturing In manufacturing, edge computing enables real-time monitoring of equipment and processes. Sensors on machinery can collect data on performance, and edge devices can analyze this data to predict maintenance needs, optimize production schedules, and reduce downtime. 2\. Autonomous Vehicles Autonomous vehicles require real-time data processing for navigation, obstacle detection, and decision making. Edge computing allows vehicles to process data from sensors and cameras locally, ensuring quick responses to dynamic environments. 3\. Healthcare In healthcare, edge computing facilitates remote patient monitoring through wearable devices that track vital signs. Data can be analyzed locally to alert healthcare providers of any anomalies, allowing for timely interventions. 4\. Retail Retailers can utilize edge computing to enhance customer experiences. In-store analytics can process data from sensors and cameras to optimize inventory management, track customer behavior, and personalize marketing efforts. 5\. Smart Cities Edge computing can enhance smart city initiatives by processing data from various sources, such as traffic sensors, surveillance cameras, and environmental monitors. This data can inform traffic management systems, public safety measures, and resource optimization. 6\. Energy Management In the energy sector, edge computing can help monitor and manage energy consumption in real-time. Smart grids can analyze data from distributed energy resources, optimizing energy distribution and enhancing grid reliability. IoT Gateways:ADetailed Overview The Internet of Things (IoT) is revolutionizing how devices connect, communicate, and collaborate. Central to this ecosystem is the IoT gateway, a critical component that facilitates communication between IoT devices and the cloud or other systems. This detailed overview will explore the functions, types, architecture, benefits, challenges, and applications of IoT gateways. What is an IoT Gateway? An IoT gateway is a hardware device or software program that acts as an intermediary between IoT devices and the cloud or local servers. It facilitates the collection, processing, and transmission of data from IoT devices to centralized systems while also enabling commands and data to flow back to the devices. Key Functions of IoT Gateways  DataAggregation: Gateways collect data from multiple IoT devices, reducing the amount of data sent to the cloud and minimizing bandwidth usage.  Protocol Translation: IoT devices often use different communication protocols (e.g., MQTT, CoAP, HTTP). Gateways can translate between these protocols, ensuring seamless communication across heterogeneous devices.  EdgeComputing: Somegateways are equipped with processing capabilities that allow for local data analysis and decision-making. This reduces latency and improves response times for time-sensitive applications.  Security: Gateways can provide an additional layer of security by encrypting data transmitted between devices and the cloud, as well as implementing authentication protocols to ensure only authorized devices communicate with the network.  DeviceManagement: IoT gateways facilitate remote management of connected devices, including monitoring performance, updating firmware, and configuring settings. Types of IoT Gateways IoT gateways can be classified into several types based on their functionality and deployment environment: 1\. Cloud Gateways  Description: These gateways connect IoT devices directly to cloud services, enabling data transmission and command execution.  UseCase:Common inapplications where real-time data access and analysis in the cloud are critical, such as smart home systems. 2\. Local Gateways  Description: Local gateways connect IoT devices to a local network, allowing for processing and analysis without relying on cloud services.  UseCase:Useful in industrial settings where immediate decision-making is necessary, such as manufacturing and process control. 3\. Mobile Gateways  Description: These gateways are embedded in mobile devices or vehicles and facilitate communication while on the move.  UseCase:Applied in applications like fleet management, where real-time data collection and monitoring are needed for vehicles. 4\. Multi-Protocol Gateways  Description: Gateways capable of supporting multiple communication protocols, enabling diverse IoT devices to connect seamlessly.  UseCase:Ideal for smart cities where devices from different manufacturers and technologies need to work together. Architecture of IoT Gateways An IoT gateway typically comprises several layers, each serving a distinct purpose: 1\. Device Layer  Components: This layer includes various IoT devices, such as sensors, actuators, and embedded systems that generate and consume data.  Functionality: The gateway interacts with these devices, collecting data and sending commands. 2\. Communication Layer  Components: Includes the various communication protocols and networking technologies (e.g., Wi-Fi, Bluetooth, Zigbee, cellular).  Functionality: Ensures data transmission between devices and the gateway, as well as between the gateway and cloud services. 3\. Processing Layer  Components: This layer encompasses the processing capabilities of the gateway, including data aggregation, filtering, and edge analytics.  Functionality: Enables local data analysis to reduce latency and bandwidth usage, allowing for immediate insights and actions. 4\. Management Layer  Components: Consists of management tools and software interfaces for configuring and monitoring the gateway and connected devices.  Functionality: Facilitates device management, security configurations, and firmware updates. 5\. Application Layer  Components: Software applications that leverage data from the gateway for specific functionalities.  Functionality: Provides users with interfaces for interacting with the IoT ecosystem, such as dashboards and reporting tools. Benefits of IoT Gateways  ImprovedDataEfficiency: By aggregating and processing data locally, gateways reduce the volume of data sent to the cloud, optimizing bandwidth and storage.  ReducedLatency: Local processing enables real-time decision-making, which is crucial for applications like autonomous vehicles and industrial automation.  EnhancedSecurity: Gateways can implement robust security measures, such as encryption and authentication, safeguarding data in transit and at rest.  Interoperability: Multi-protocol gateways allow devices from different manufacturers and technologies to communicate seamlessly, enhancing system integration.  Cost-Effectiveness: By minimizing cloud storage and processing costs through local data handling, gateways can lead to overall cost savings for IoT deployments. Challenges of IoT Gateways  Complexity in Management: Managing a diverse array of devices and protocols through a single gateway can introduce complexity, requiring sophisticated management tools.  Security Risks: While gateways can enhance security, they can also become targets for cyberattacks, necessitating ongoing security updates and monitoring.  Scalability Issues: As the number of connected devices increases, gateways must be able to scale effectively without performance degradation.  LimitedProcessing Power: Some gateways may have constraints in computational resources, limiting the complexity of analytics that can be performed locally.  Interoperability Challenges: Despite being designed for multi-protocol support, differences in device standards and protocols can still pose challenges in achieving seamless communication. Applications of IoT Gateways IoT gateways are utilized across various industries and applications, demonstrating their versatility and significance: 1\. Smart Homes In smart home applications, gateways connect various IoT devices such as smart thermostats, lights, and security cameras. They enable centralized control and monitoring through mobile apps or voice assistants. 2\. Industrial IoT (IIoT) In industrial settings, gateways facilitate communication between machines, sensors, and monitoring systems. They support predictive maintenance, real-time monitoring, and data-driven decision-making to enhance operational efficiency. 3\. Healthcare In healthcare, gateways enable remote patient monitoring by connecting wearable devices and medical equipment to healthcare providers. They facilitate data transmission for timely interventions and improved patient outcomes. 4\. Smart Cities In smart city initiatives, gateways aggregate data from various sensors, such as traffic lights, waste management systems, and environmental monitors. This data can be analyzed to optimize city services and enhance public safety. 5\. Agriculture IoT gateways are used in precision agriculture to connect sensors and devices monitoring soil moisture, weather conditions, and crop health. This data supports informed decision-making for irrigation, fertilization, and pest management. 6\. Fleet Management In logistics and transportation, gateways in vehicles connect GPS trackers and telematics devices. They enable real-time monitoring of vehicle performance, location tracking, and efficient route planning. Data Ingestion and Data Processing Pipelines:A Detailed Overview In today's data-driven world, organizations are inundated with vast amounts of data generated from various sources. Effectively managing this data requires robust systems for data ingestion and processing. This document provides a comprehensive overview of data ingestion and data processing pipelines, their components, architectures, challenges, and best practices. What is Data Ingestion? Data ingestion is the process of collecting and importing data from various sources into a storage system or data processing platform. This can involve real-time or batch processing, depending on the needs of the organization and the nature of the data. Key Types of Data Ingestion Batch Ingestion: 1. 2. Involves collecting data in batches at scheduled intervals. This method is efficient for processing large volumes of data that do not require immediate analysis. Use Case: Daily sales reports from a retail system are aggregated and analyzed once every 24 hours. Real-time Ingestion: 1. 2. Involves continuously collecting and processing data as it is generated. This approach is crucial for applications requiring immediate insights. Use Case: Streaming data from social media platforms to analyze sentiment in real-time. Streaming Ingestion: 1. 2. Aspecific form of real-time ingestion that handles continuous flows of data from sources like sensors, logs, and event streams. Use Case: Data from IoT devices transmitting sensor readings continuously to a cloud platform. What are Data Processing Pipelines? Data processing pipelines are a series of data processing steps that transform raw data into valuable insights. These pipelines facilitate the systematic handling of data from ingestion through processing, storage, and visualization. Key Stages of Data Processing Pipelines  DataIngestion:The initial stage where data is collected from various sources and ingested into a processing system.  DataCleansing:Involves removing inaccuracies, duplicates, and inconsistencies in the data. This ensures the quality and reliability of the data used for analysis.  DataTransformation:Data is transformed into a suitable format for analysis. This may include normalization, aggregation, and applying business rules.  DataStorage:Processed data is stored in databases or data warehouses for further analysis. Choosing the right storage solution is critical based on the use case (e.g., relational databases, NoSQL, or data lakes).  DataAnalysis:Analytical processes are applied to the transformed data to extract insights. This can include statistical analysis, machine learning, or data mining techniques.  DataVisualization:The final stage involves presenting the processed data through dashboards, reports, or other visualization tools to facilitate decision-making. Architecture of Data Ingestion and Processing Pipelines Data ingestion and processing pipelines can be organized into several architectural models based on the specific needs of the organization: 1\. Lambda Architecture  Description: Combines batch processing and real-time processing to provide a comprehensive view of data. It consists of three layers: o BatchLayer: Processes large volumes of data periodically. o SpeedLayer:Handles real-time data processing. o Serving Layer: Merges results from both the batch and speed layers for analysis.  UseCase:Useful in scenarios where both historical and real-time data insights are necessary, such as fraud detection. 2\. Kappa Architecture  Description: Simplifies the Lambda architecture by using a single processing layer. All data is treated as a stream, enabling real-time processing only.  UseCase:Effective for applications that primarily require real-time data insights without complex batch processing. 3\. Event-Driven Architecture  Description: Focuses on producing and consuming events as they occur. It uses message brokers to facilitate communication between different components of the pipeline.  UseCase:Ideal for applications with high variability and where timely responses to events are crucial, such as IoT applications. Components of Data Ingestion and Processing Pipelines Several key components are essential for building effective data ingestion and processing pipelines:  DataSources:Various origins of data, including databases, APIs, IoT devices, logs, and external data feeds.  Ingestion Tools:Software and frameworks designed for data ingestion, such as Apache Kafka, Apache Nifi, and AWS Kinesis.  DataProcessing Frameworks:Technologies that enable data processing, including Apache Spark, Apache Flink, and Apache Beam.  DataStorage Solutions:Systems for storing processed data, such as data warehouses (e.g., Amazon Redshift), NoSQL databases (e.g., MongoDB), and data lakes (e.g., Hadoop).  Visualization Tools:Applications that allow users to visualize and interact with data, such as Tableau, Power BI, and Grafana. Benefits of Data Ingestion and Processing Pipelines  Efficiency: Streamlined data ingestion and processing allow organizations to handle vast amounts of data quickly and efficiently, enabling timely insights.  DataQuality: Regular cleansing and transformation improve data quality, ensuring that decision making is based on accurate and reliable information.  Scalability: Modern data pipelines can easily scale to accommodate growing data volumes and changing business requirements.  Real-time Insights: With real-time ingestion and processing, organizations can respond rapidly to changing conditions, enhancing their competitiveness.  EnhancedDecision-Making: Effective data processing pipelines provide organizations with actionable insights, leading to informed decision-making. Challenges of Data Ingestion and Processing Pipelines  DataVariety: Handling diverse data types (structured, semi-structured, unstructured) from various sources can complicate ingestion and processing.  Latency: Ensuring low latency in real-time processing can be challenging, particularly when dealing with large data volumes.  DataSecurity: Protecting data during ingestion and processing is critical, requiring robust security measures to prevent breaches.  Complexity: Designing and managing complex data pipelines can be resource-intensive, necessitating specialized skills and tools.  Integration: Integrating data from multiple sources and ensuring compatibility across different systems can pose significant challenges. Best Practices for Data Ingestion and Processing Pipelines  DefineClear Objectives: Establish clear goals for data ingestion and processing to guide the design and implementation of the pipeline.  Prioritize Data Quality: Implement regular data cleansing and validation processes to maintain high data quality throughout the pipeline.  ChoosetheRight Tools: Select appropriate ingestion and processing tools that align with the organization's specific use cases and data requirements.  ImplementSecurity Measures: Ensure robust security protocols are in place to protect data during ingestion and processing, including encryption and access controls.  MonitorPerformance: Continuously monitor the performance of the data pipeline to identify bottlenecks and optimize processes for efficiency.  Scalability Considerations: Design pipelines with scalability in mind, allowing for future growth in data volume and complexity. Data Stream Processing:ADetailed Overview Data stream processing is a vital paradigm in the field of data management, allowing organizations to process continuous flows of data in real-time. As the volume and velocity of data generated by various sources, such as IoT devices, social media, and transactions, continue to increase, the ability to analyze and respond to this data quickly has become crucial. This document explores the fundamental concepts, architectures, technologies, benefits, challenges, and applications of data stream processing. What is Data Stream Processing? Data stream processing refers to the real-time processing and analysis of data streams---continuous sequences of data elements generated by various sources. Unlike traditional batch processing, which handles data in fixed-size chunks at scheduled intervals, stream processing enables the immediate processing of data as it arrives, allowing for timely insights and actions. Key Characteristics of Data Stream Processing  Continuous Data Flow: Data is processed continuously rather than in discrete batches, enabling immediate reaction to new information.  LowLatency:Stream processing systems are designed to minimize processing delay, ensuring that insights are generated in near real-time.  Event-Driven Architecture: Stream processing often employs event-driven architectures, where events (data points) trigger specific actions or processing tasks.  Stateful Processing: Many stream processing applications maintain state information across events, allowing for complex event processing and real-time analytics. Architectures of Data Stream Processing Several architectural approaches can be used to implement data stream processing, each with its advantages and use cases. 1\. Microservices Architecture  Description: In this architecture, applications are built as a collection of loosely coupled services that communicate over a network. Each microservice can handle specific stream processing tasks.  UseCase:Useful for large-scale applications where different services can be developed, deployed, and scaled independently. 2\. Lambda Architecture  Description: This architecture combines batch processing and stream processing. It consists of three layers: o BatchLayer: Processes historical data. o SpeedLayer:Handles real-time data. o Serving Layer: Merges results from both layers for analysis.  UseCase:Suitable for applications that require both real-time and historical analysis, such as fraud detection systems. 3\. Kappa Architecture  Description: Simplifies the lambda architecture by treating all data as a stream. This architecture focuses solely on real time processing without batch components.  UseCase:Ideal for applications that primarily depend on real-time data, such as monitoring and alerting systems. Components of Data Stream Processing Data stream processing systems typically consist of several key components:  DataSources: Various origins of data, including sensors, applications, social media feeds, and logs, continuously generate streams of data.  StreamProcessing Engines: The core component that processes incoming data streams in real-time. Popular engines include Apache Kafka, Apache Flink, Apache Spark Streaming, and Google Cloud Dataflow.  MessageBrokers: These components facilitate the transmission of data between sources and processing systems. Examples include Apache Kafka and RabbitMQ.  StorageSolutions: Processed data needs to be stored for future analysis and reporting. Solutions can include databases, data lakes, or time-series databases.  Monitoring and Management Tools: These tools provide insights into the performance and health of the stream processing system, enabling operators to optimize performance and troubleshoot issues. Technologies for Data Stream Processing Several technologies and frameworks support data stream processing: 1\. Apache Kafka  Description: A distributed event streaming platform that allows for high-throughput, low-latency data streaming. Kafka serves as both a message broker and a storage system for streaming data.  UseCase:Commonly used for building real-time data pipelines and streaming applications, such as log aggregation and real-time analytics. 2\. Apache Flink  Description: A stream processing framework designed for stateful computations over data streams. Flink offers high performance and supports complex event processing.  UseCase:Ideal for applications that require real-time analytics, such as fraud detection and anomaly detection in financial transactions. 3\. Apache Spark Streaming  Description: An extension of Apache Spark that enables processing of live data streams. Spark Streaming allows for batch processing of stream data in micro-batches.  UseCase:Suitable for applications that need real-time processing while leveraging Spark\'s powerful batch processing capabilities. 4\. Google Cloud Dataflow  Description: A fully managed stream and batch processing service that simplifies data processing tasks. It uses the Apache Beam programming model.  UseCase:Useful for organizations looking to run large-scale data processing jobs without managing infrastructure. Benefits of Data Stream Processing  Real-Time Insights: Stream processing enables organizations to gain immediate insights from data as it is generated, facilitating timely decision-making.  Scalability: Stream processing systems can easily scale to accommodate increasing data volumes, making them suitable for large-scale applications.  EnhancedCustomer Experience: By processing data in real time, businesses can respond quickly to customer interactions, leading to improved user experiences and engagement.  Operational Efficiency: Stream processing automates data handling and analysis, reducing the need for manual intervention and enabling teams to focus on higher-level tasks.  Predictive Analytics: Real-time data analysis allows organizations to implement predictive analytics, enabling proactive decision-making and risk management. Challenges of Data Stream Processing  Complexity: Building and maintaining a data stream processing system can be complex, requiring specialized skills and tools.  DataQuality: Ensuring the quality and accuracy of incoming data streams can be challenging, particularly when dealing with diverse data sources.  Latency: While stream processing aims for low latency, achieving it consistently can be difficult, especially under heavy load or with high-volume data streams.  StateManagement: Managing state across different events can introduce challenges, particularly in ensuring data consistency and fault tolerance.  Integration: Integrating stream processing systems with existing infrastructure and applications can pose significant challenges. Applications of Data Stream Processing Data stream processing is applied across various industries and use cases: 1\. Financial Services In finance, stream processing is used for real-time fraud detection, transaction monitoring, and risk assessment. By analyzing transactions as they occur, organizations can identify suspicious behavior and take immediate action. 2\. IoT Applications Stream processing plays a crucial role in IoT applications by processing data from connected devices in real time. This enables immediate responses to events, such as alerting users to anomalies in sensor readings. 3\. Telecommunications Telecom companies use stream processing for network monitoring, call detail record analysis, and customer experience management. By analyzing call data in real-time, they can quickly identify issues and optimize network performance. 4\. E-Commerce In e-commerce, stream processing is employed for real-time inventory management, personalized recommendations, and dynamic pricing. By analyzing customer behavior as it occurs, businesses can tailor experiences to individual preferences. 5\. Social Media Analytics Social media platforms leverage stream processing to analyze user interactions and sentiments in real-time. This enables brands to respond quickly to trends and customer feedback. Best Practices for Data Stream Processing  DefineClear Use Cases: Establish clear objectives for data stream processing to guide design and implementation.  Prioritize Data Quality: Implement data validation and cleansing processes to maintain high data quality throughout the stream.  ChoosetheRight Tools: Select appropriate stream processing technologies that align with organizational goals and infrastructure.  MonitorPerformance: Continuously monitor the performance of the stream processing system to identify bottlenecks and optimize efficiency.  ImplementSecurity Measures: Ensure robust security protocols to protect data in transit and maintain compliance with data privacy regulations. UNIT:-3 Introduction to Sensors and Transducers Sensors and transducers are fundamental components in modern technology, essential for the measurement and conversion of physical parameters into readable data. From monitoring environmental conditions like temperature and pressure to enabling the functionality of devices like smartphones and medical equipment, sensors and transducers have become integral to various fields of science, engineering, and industry. This note provides an in-depth understanding of sensors and transducers, their types, applications, and the principles behind their operation. 1\. What are Sensors and Transducers? Sensors Asensor is a device that detects a physical quantity (e.g., temperature, pressure, humidity, motion) and converts it into an electrical signal that can be read, processed, or recorded. Sensors are designed to respond to a particular stimulus, whether it\'s light, sound, heat, or motion. The output signal can be in various forms, such as voltage, current, or frequency, depending on the type of sensor and its application. For example:    Athermometer is a sensor that measures temperature. Aphotocell is a sensor that detects light intensity. Apressure sensor detects pressure changes. Transducers Atransducer, on the other hand, is a broader term that refers to any device that converts one form of energy into another. While all sensors are transducers (because they convert physical quantities into electrical signals), not all transducers are sensors. Transducers can convert energy from electrical to mechanical, thermal, or optical forms, among others. For example:   Aloudspeaker is a transducer that converts electrical signals into sound (mechanical energy). An electric motor converts electrical energy into mechanical energy. Thus, while a sensor typically refers to a device that detects and measures a physical quantity, a transducer refers to any device that converts energy from one form to another. 2\. Working Principle of Sensors and Transducers Both sensors and transducers operate based on the conversion of energy from one form to another. The working principle of sensors and transducers can generally be broken down into the following steps: 1\. Stimulus: The sensor or transducer is exposed to a physical stimulus, such as light, pressure, temperature, or sound. 2\. Conversion: The device converts the stimulus into an electrical signal or a different form of energy. 3\. Processing: The output signal is processed, amplified, or conditioned to be suitable for further measurement or display. 4\. Output: The processed signal is outputted in a form that can be interpreted, recorded, or acted upon. For example, in a temperature sensor, the temperature variation (stimulus) causes a resistance change in a thermistor (conversion), which is then converted into a voltage signal that can be displayed or recorded (output). 3\. Types of Sensors Sensors are classified based on the type of physical quantity they measure. Some common types of sensors include: 3.1 Temperature Sensors These sensors measure the temperature of an object or environment. Common types include:     Thermistors: These sensors change resistance with temperature. Thermocouples: Two different metals are joined to form a junction, and a voltage is generated that varies with temperature. RTDs (Resistance Temperature Detectors): These sensors use the principle that the resistance of metals increases with temperature. Infrared Sensors: These detect the infrared radiation emitted by an object, which is related to its temperature. 3.2 Pressure Sensors Pressure sensors measure the force exerted by a fluid (liquid or gas) per unit area. Types include:    Strain Gauge Sensors: These measure pressure by detecting the deformation of a material under pressure. Capacitive Pressure Sensors: These sensors detect changes in capacitance caused by pressure induced displacement. Piezoelectric Sensors: These sensors generate an electrical charge in response to mechanical stress or pressure. 3.3 Light Sensors Light sensors detect light intensity and convert it into an electrical signal. Types include:    Photodiodes: These generate current when exposed to light. Phototransistors: Similar to photodiodes but with an amplified output. LDR(Light Dependent Resistor): The resistance of these sensors decreases as light intensity increases. 3.4 Proximity Sensors Proximity sensors detect the presence or absence of an object within a certain range without physical contact. Commontypes include:    Inductive Proximity Sensors: Detect metal objects using electromagnetic fields. Capacitive Proximity Sensors: Detect objects based on changes in capacitance. Ultrasonic Sensors: Measure distance by emitting ultrasonic sound waves and calculating the time taken for them to return after bouncing off an object. 3.5 Motion Sensors These sensors detect movement or changes in position. Examples include:    Passive Infrared Sensors (PIR): Detect infrared radiation changes, typically used in motion detectors. Accelerometers: Measure acceleration and movement in various directions. Gyroscope: Measures the rate of rotation or orientation. 4\. Types of Transducers As mentioned earlier, transducers convert one form of energy to another. Some common types of transducers include: 4.1 Mechanical Transducers These transducers convert mechanical energy into electrical signals. Common examples include:   Microphones: Convert sound (mechanical wave) into electrical signals. Speakers: Convert electrical signals into sound. 4.2 Electrochemical Transducers These transducers convert chemical energy into electrical energy. Examples include:   pHSensors: Convert the concentration of hydrogen ions in a solution into an electrical signal. Gas Sensors: Detect the presence of specific gases by converting chemical reactions into electrical signals. 4.3 Thermoelectric Transducers These transducers convert heat energy into electrical energy. For example:  Thermocouples: As mentioned earlier, they convert temperature differences into voltage. 4.4 Piezoelectric Transducers These transducers convert mechanical stress (pressure) into electrical signals. They are widely used in sensors and actuators, including:  Ultrasonic Transducers: Used in medical imaging and distance measuring devices. 5\. Applications of Sensors and Transducers Sensors and transducers have a wide array of applications across various industries, such as: 5.1 Industrial Automation Sensors are used in manufacturing processes for monitoring and controlling operations. Examples include:    Temperature and pressure sensors to maintain optimal working conditions. Proximity sensors to detect the position of parts in assembly lines. Flow sensors to measure fluid movement in pipes and tanks. 5.2 Automotive Industry In vehicles, sensors and transducers monitor critical parameters, including:    Oxygen sensors for emission control. Accelerometers and gyroscopes for airbag deployment systems. Radar sensors for collision avoidance and parking assistance. 5.3 Medical Field Medical devices heavily rely on sensors for monitoring patients' conditions. Examples include:    ECG(Electrocardiogram) sensors to measure heart activity. Pulse oximeters to measure oxygen saturation in the blood. Temperature sensors for body temperature monitoring. 5.4 Consumer Electronics Sensors play a key role in enhancing the functionality of consumer electronics. Examples include:   Accelerometers in smartphones to detect screen orientation. Proximity sensors in touchless devices like automatic faucets and light switches. 5.5 Environmental Monitoring Environmental sensors are used for the detection of various factors such as:    Air quality sensors for monitoring pollution levels. Humidity sensors for weather forecasting and agricultural purposes. Water quality sensors to monitor contaminants in drinking water. Integrating Sensors into Sensor Processing Boards The integration of sensors into sensor processing boards is a critical aspect of modern embedded systems, enabling the collection, processing, and transmission of data from various physical environments. Sensors are used to measure parameters such as temperature, pressure, humidity, light intensity, motion, and more. The data generated by these sensors must be processed, analyzed, and, in many cases, transmitted to other systems for further actions. This is where sensor processing boards come into play. Asensor processing board typically houses not only the physical sensors but also the associated electronics that manage signal conditioning, processing, communication, and sometimes, actuation. The integration process involves interfacing sensors with microcontrollers, processors, and communication protocols to form a cohesive system that can capture and transmit useful data. This note will discuss the principles and methods for integrating sensors into sensor processing boards, the role of different components in this system, and the design considerations for efficient and effective integration. 1\. Overview of Sensors and Sensor Processing Boards Sensors Sensors are devices that detect physical quantities and convert them into measurable electrical signals. These signals typically need to be conditioned (e.g., amplified, filtered) before they can be interpreted by digital circuits. There are many types of sensors, including:    Analog sensors: Provide a continuous output signal (e.g., temperature sensors, light sensors). Digital sensors: Provide discrete data, often in the form of a serial output or pulse (e.g., digital temperature sensors, motion sensors). Smart sensors: Incorporate signal conditioning and sometimes microcontroller functionalities, providing processed data directly to the system (e.g., MEMS sensors, IoT-enabled sensors). Sensor Processing Boards Asensor processing board is a circuit board designed to interface with multiple sensors, process the data generated by these sensors, and provide the necessary outputs. This typically involves the following functions:     Signal conditioning: Amplifying, filtering, or otherwise modifying sensor outputs to make them usable by the processor. Analog-to-Digital Conversion (ADC): Converting analog signals from sensors into digital data that can be processed by microcontrollers or processors. Processing: Computing and analyzing sensor data using embedded processors or microcontrollers. Communication: Sending data to other devices or networks, often via protocols such as UART, SPI, I2C, or wireless protocols like Wi-Fi, Bluetooth, or Zigbee. 2\. Key Components of Sensor Processing Boards When integrating sensors with sensor processing boards, several components are necessary to ensure proper functioning: 2.1 Microcontroller (MCU) or Microprocessor (MPU) The heart of any sensor processing board is the microcontroller (MCU) or microprocessor (MPU), which manages the overall operation of the board, including interfacing with sensors, processing sensor data, and communicating with other devices.   MCU:Amicrocontroller typically has embedded RAM, flash memory, and several I/O interfaces, and is well-suited for simpler applications that require limited processing power. MPU:Amicroprocessor offers more processing power, more sophisticated interfaces, and the ability to run complex operating systems. MPUs are often used for more advanced sensor applications such as robotics, autonomous vehicles, and machine learning applications. The choice between MCU and MPU depends on the complexity of the task and the processing requirements of the sensor system. 2.2 Signal Conditioning Circuitry Most sensors do not provide data in a form that is directly usable by a processor. For instance, an analog temperature sensor might output a voltage that needs to be amplified or filtered before it can be digitized. Signal conditioning is the process of preparing the raw signal from the sensor for further processing, which may involve:     Amplification: Increasing the strength of weak sensor signals. Filtering: Removing noise from sensor signals, which can be caused by environmental interference or power supply fluctuations. Linearization: Some sensors, such as thermistors or strain gauges, may produce non-linear outputs that require mathematical corrections. Impedance matching: Ensuring that the output of the sensor is compatible with the input requirements of the ADC or processor. Commoncomponents used in signal conditioning include operational amplifiers (op-amps), resistors, capacitors, and analog filters. 2.3 Analog-to-Digital Converter (ADC) Sensors like thermistors, photodiodes, and strain gauges often provide analog output signals that must be converted into digital form for processing by a microcontroller. This is done using an analog-to-digital converter (ADC). The ADC samples the analog signal at regular intervals and converts it into a digital representation, typically in the form of a binary number.   Resolution: The resolution of the ADC determines the accuracy with which the analog signal is represented in digital form. A higher resolution ADC provides finer granularity but requires more processing power and memory. Sampling rate: The rate at which the ADC samples the analog signal affects the ability to capture fast changes in the sensor\'s output. A higher sampling rate is necessary for high-speed measurements. Many microcontrollers come with built-in ADCs, but in cases where precision is critical, external ADCs can be used to achieve better performance. 2.4 Communication Interfaces Sensor processing boards often need to transmit data to external systems for further analysis, storage, or control. Communication interfaces allow the sensor board to send the processed data to other devices, such as computers, cloud platforms, or other embedded systems. Commoncommunication protocols include:     I2C (Inter-Integrated Circuit): A two-wire interface used for connecting sensors and other peripheral devices in a master-slave configuration. SPI (Serial Peripheral Interface): A high-speed, four-wire interface suitable for communicating with multiple devices. UART(Universal Asynchronous Receiver-Transmitter): A simple serial communication protocol often used for short-distance communication with other embedded systems or computers. Wireless protocols: Wi-Fi, Bluetooth, Zigbee, and LoRaWAN are commonly used for wireless communication, particularly in IoT systems. Choosing the appropriate communication protocol depends on factors like speed, distance, and power consumption. 2.5 Power Supply and Management Asensor processing board typically requires a stable and reliable power supply. Sensors themselves may operate at different voltages (e.g., 3.3V, 5V), so the power supply needs to be able to handle the varying requirements of both the sensors and the processing unit.   Voltage regulators: These components ensure that the power supply provides the correct voltage for each part of the sensor board. Power management ICs (PMICs): These are used to manage power distribution and optimize energy usage, especially in battery-powered or energy-harvesting systems. 3\. Integrating Sensors into Sensor Processing Boards The integration process involves several steps: 3.1 Selecting the Sensors The first step is to choose the appropriate sensors based on the desired measurements and the application. This involves considering factors such as:      Measurement range (e.g., temperature range, pressure range). Accuracy and precision. Output type (analog or digital). Response time (e.g., how quickly the sensor reacts to changes in the environment). Power consumption (important for battery-powered systems). 3.2 Interfacing Sensors with the Processing Unit Once the sensors are selected, they need to be interfaced with the processing unit. For analog sensors, the signal must be fed through the signal conditioning circuitry, followed by an ADC. Digital sensors, on the other hand, may communicate directly with the processor via protocols like I2C, SPI, or UART.   For analog sensors, the signal might be conditioned (amplified, filtered), and then sent to an ADC pin on the MCU or external ADC for conversion to a digital signal. For digital sensors, the sensor might directly communicate over a protocol such as I2C or SPI. 3.3 Data Processing and Analysis Once the data from the sensors is digitized, it can be processed by the MCU or MPU. This may include:    Data filtering and noise reduction. Calibration of sensor data. Statistical analysis or algorithms to extract useful information from raw sensor data (e.g., converting voltage measurements into temperature readings). 3.4 Communication and Output Finally, the processed data can be transmitted to external devices or systems via communication protocols. This could involve sending data to a remote server, triggering an alert in case of an anomaly, or storing the data locally for later analysis. 3.5 Testing and Validation After the integration of sensors and processing units, the system needs to be thoroughly tested to ensure the sensors provide accurate and reliable data. This may involve:    Verifying sensor calibration. Testing communication protocols for data integrity. Ensuring that the system works reliably under different environmental conditions. 4\. Design Considerations While integrating sensors into sensor processing boards, several design considerations need to be addressed:     Power efficiency: Particularly for battery-powered applications, it\'s important to minimize power consumption. Techniques such as sleep modes, efficient power supplies, and low-power sensors should be considered. Scalability: If future expansion is needed, the system should support additional sensors without major hardware modifications. Noise immunity: Proper grounding and shielding techniques should be employed to reduce interference and ensure accurate sensor readings. Reliability: Sensors and associated electronics should be chosen to meet the demands of the application environment (e.g., temperature extremes, humidity, vibration). Introduction to Industrial Data Acquisition Systems Industrial Data Acquisition Systems (DAS) are critical components in modern industries, enabling the collection, monitoring, and analysis of data from various machines, sensors, and processes. These systems play a central role in industrial automation, process control, predictive maintenance, and optimization of operations. They provide real-time insights into the operational performance of machinery, processes, and the environment, helping engineers, operators, and decision-makers make informed decisions. This note will delve into the components, working principles, types, applications, and key considerations involved in designing and implementing industrial data acquisition systems. 1\. What is an Industrial Data Acquisition System? An Industrial Data Acquisition System (DAS) is a system that collects, measures, and monitors various physical, electrical, or mechanical parameters from industrial environments. The system converts real-world signals (such as temperature, pressure, humidity, vibration, or flow) into digital data that can be analyzed and acted upon. ADAStypically consists of the following key elements:       Sensors/Transducers: These devices detect physical or environmental variables and convert them into electrical signals. Sensors can measure temperature, pressure, humidity, vibration, and more. Signal Conditioning: Before the data from sensors can be used, it often requires conditioning, which includes amplification, filtering, and sometimes conversion (e.g., analog to digital conversion). Data Acquisition Hardware: This component is responsible for acquiring data from sensors and converting it into a format that can be processed by computers or control systems. Data Processing: Once the data is captured, it is processed and analyzed. This could involve simple operations like filtering and scaling, or more complex tasks such as real-time data analysis, pattern recognition, or predictive modeling. Software Interface: The software interface provides tools for operators and engineers to visualize, interpret, and make decisions based on the acquired data. It may include dashboards, alarms, trends, and analytical tools. Communication Interface: This ensures that the acquired data can be sent to other systems, databases, or cloud platforms for further analysis or storage. Common communication methods include Modbus, Ethernet, OPC, and wireless protocols. 2\. Working Principle of Industrial Data Acquisition Systems An industrial DAS operates through a series of steps to capture and process sensor data: Signal Detection: Sensors are placed at various points in the industrial process to measure different parameters like temperature, pressure, or flow rate. These sensors convert the measured physical quantity into an electrical signal (voltage, current, resistance, etc.). Signal Conditioning: The raw signal from a sensor may need amplification, filtering, or other modifications to be useful. For example, a temperature sensor may produce a small voltage that needs to be amplified to make it detectable by a data acquisition system. Additionally, noise from other electrical equipment may need to be filtered out to ensure accurate measurements. Data Conversion: The signal is converted from analog to digital format using an Analog-to-Digital Converter (ADC). The ADC samples the analog signal at discrete intervals, converting it into a binary number that can be processed by the system. The quality of the ADC (its resolution and sampling rate) directly impacts the accuracy and speed of data acquisition. Data Processing and Storage: After conversion, the digital data is processed, often by a programmable logic controller (PLC) or microcontroller, to perform specific tasks such as calculations, comparisons, and decision-making. The data can be stored locally or transmitted to a central database or cloud storage system for further analysis. Communication: The processed data is transmitted to other systems (e.g., SCADA systems, HMI displays, or cloud platforms) for monitoring, analysis, and decision-making. Communication can occur via various protocols such as Ethernet, Modbus, OPC, Profibus, or wireless networks. Display and Action: Finally, the data is presented in a human-readable format on operator interfaces such as Human-Machine Interfaces (HMI) or SCADA (Supervisory Control and Data Acquisition) systems. Based on the analysis, actions such as system adjustments, alarms, or alerts may be triggered to optimize processes or prevent failures. 3\. Components of an Industrial Data Acquisition System 3.1 Sensors and Transducers Sensors and transducers are the front-end devices of a DAS. They measure physical quantities such as temperature, pressure, flow, humidity, vibration, and more. These sensors output analog or digital signals that the DAS will capture and process.      Temperature Sensors: Thermocouples, RTDs (resistance temperature detectors), thermistors. Pressure Sensors: Strain gauges, capacitive sensors, piezoelectric sensors. Flow Sensors: Electromagnetic, ultrasonic, turbine flow meters. Vibration Sensors: Accelerometers, piezoelectric sensors. Proximity Sensors: Inductive, capacitive, ultrasonic sensors. 3.2 Signal Conditioning Signal conditioning is the process of modifying the sensor's output signal to make it suitable for further processing. It may involve:     Amplification: Increasing the signal strength if it is too weak. Filtering: Removing unwanted noise or high-frequency components. Linearization: Correcting nonlinear sensor outputs (e.g., temperature sensors). Isolation: Providing electrical isolation between the sensor and the acquisition system to prevent ground loops or electrical interference. 3.3 Data Acquisition Hardware This includes the physical hardware components responsible for acquiring and digitizing the signals from sensors. It typically involves:   Data Acquisition Boards (DAQ): These are designed to capture analog signals, convert them to digital, and pass the data to the processing unit. DAQs come in various forms, including PCI, USB, and Ethernet-based devices. Microcontrollers or PLCs: These devices process the digital data and control the system based on the acquired measurements. 3.4 Data Processing and Analysis Once the data is acquired and digitized, it is processed and analyzed to extract meaningful information. This may involve:  Real-Time Analysis: In many industrial settings, real-time processing of sensor data is required for immediate decision-making. For example, in predictive maintenance, data from vibration sensors can be analyzed to detect early signs of equipment failure.  Historical Analysis: Data can also be stored and analyzed over time to identify trends, correlations, and patterns. This helps with long-term optimization and decision-making. 3.5 Software Interface and Visualization Software tools play a key role in visualizing data and providing insights to operators. Commonly used systems include:    SCADASystems: These systems provide real-time monitoring and control capabilities, offering a graphical interface that displays sensor data and alerts. HMI(Human-Machine Interface): These are used to interact with machinery and processes, allowing operators to input commands and receive feedback. Data Logging and Reporting Software: For recording data over time and generating reports for analysis. 3.6 Communication Interfaces Industrial data acquisition systems need to communicate with other devices and systems, which requires a variety of communication protocols:    Ethernet: Common in modern industrial systems for local area networks (LANs) or wide area networks (WANs). Modbus: A widely-used industrial protocol that operates over serial communication (RS-232, RS 485\) or Ethernet (Modbus TCP/IP). OPC(OLEforProcess Control): A standard for industrial communication that allows data exchange between different devices and software applications. 4\. Types of Industrial Data Acquisition Systems Industrial DASs can be categorized based on their architecture, scale, and the specific needs of the application: 4.1 Centralized Data Acquisition Systems In a centralized DAS, all sensors and data acquisition hardware are connected to a single processing unit (e.g., a central computer or PLC). Data from all sensors is sent to this unit for processing and analysis. This system is often used in smaller operations or systems where real-time processing is not as critical. 4.2 Distributed Data Acquisit