Document Details
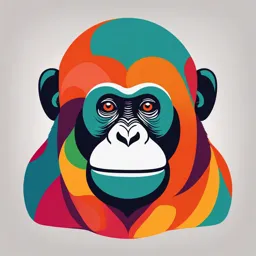
Uploaded by FinerPinkTourmaline7587
null
Full Transcript
9 First analytic steps: familiarisation and data coding...
9 First analytic steps: familiarisation and data coding OVERVIEW Data collection and data analysis: separate stages? Reading and familiarisation: essential beginnings What is coding? Doing complete coding What role do computer programs have in qualitative coding and analysis? Once you have data ready for analysis (transcribed or collated), you can begin. In the following three chapters (9–11) we aim to provide practical ‘how to’ guidance around analysis; as much as we describe what you need to do, we provide illustrated worked examples to show how you do it, using the weight and obesity focus group (FG) dataset we introduced in Chapter 5 (see the companion website). Showing what analysis looks like can take away some of the (anxiety-provoking) uncertainty of qualitative research. Some methods – such as interpretative phenomenological analy- sis (IPA) and thematic analysis (TA) – provide more detailed guidance than others – such as discourse analysis (DA) (see Table 9.1). We primarily demonstrate a basic TA approach, and discuss how IPA, grounded theory (GT) and pattern-based DA do things differently. It’s tempting to view analytic guidelines as recipes that have to be precisely followed, as if adhering to these will ensure a successful outcome. This isn’t the case. Obviously, being systematic and thorough is crucial (see Chapter 12), but good qualitative analysis is primarily a product of an ‘analytic sensibility’, not a product of ‘following the rules’. An analytic sensibility is often viewed as a rather esoteric skill that some rarefied individuals naturally possess, rather than a skill that can be developed. We think it can be developed. An analytic sensibility refers to the skill of reading and interpreting data through the particular theoretical lens of your chosen method. It also refers to being able to produce insights into the meaning of the data that go beyond the obvious or surface-level content 09-Braun & Clarke_Ch-09.indd 201 28/02/2013 7:43:49 PM 204 Successfully analysing qualitative data of the data, to notice patterns or meanings that link to broader psychological, social or theoretical concerns. Essentially, it relates to taking an inquiring and interpretative posi- tion on data (see Chapter 11 for more discussion around interpretative analysis). This is easier if you feel you have a handle on what you’re supposed to be doing. Chapters 9–11 are designed to provide that. The first analytic steps we lay out in this chapter can be done either on hard copy (paper and pen) or electronically, either with one of many different computer programs (discussed later) or in Microsoft Word using the comment feature. We explain the pro- cess as if you were using hard copy data. It’s good to learn to code using a manual, hard-copy process, even if you eventually do it electronically. Quite apart from anything else, being away from a screen allows for a different mode of interaction with data, and moves you into a different conceptual and physical space for conducting analysis (Bringer, Johnston, & Brackenridge, 2006). DATA COLLECTION AND DATA ANALYSIS: SEPARATE STAGES? In quantitative research, analysis generally only begins once all data have been collected. In qualitative research, it isn’t essential to have all your data collected to start your analy- sis. In reality, there’s not always a clean separation between data collection and analysis – GT even prefers that there isn’t – which can result from a drawn-out data collection period, where you begin your data coding while still collecting final data items, or from a staged data collection process, where you collect part of your data, review it with an analytic eye for possible patterns, and then refine or reorient subsequent data collection. This is one of the advantages of the flexibility of qualitative research designs. READING AND FAMILIARISATION: ESSENTIAL BEGINNINGS The analysis of qualitative data essentially begins with a process of ‘immersion’ in the data. The aim of this phase is to become intimately familiar with your dataset’s con- tent, and to begin to notice things that might be relevant to your research question. For textual data, this process involves reading, and re-reading, each data item; for audio (or visual) data, it involves a similar pattern of repeated listening to (and viewing) the material. During this process, you’ll probably start to notice things of interest. These might be loose overall impressions of the data (e.g. food seems to be talked about in two ways – as friend and as enemy), a conceptual idea you have about the data (e.g. participants use an implicit model of the person as naturally ‘gluttonous’ and ‘lazy’), or more concrete and specific issues (e.g. that a participant uses euphemistic language around weight and body size). It’s good to keep a record of these ‘noticings’, and 09-Braun & Clarke_Ch-09.indd 204 28/02/2013 7:43:49 PM First analytic steps: familiarisation and data coding 205 record them in a place you can refer back to. This might be a separate file (e.g. your research journal) or notes directly on the data themselves. This process is observa- tional and casual, rather than systematic and precise. Don’t agonise over the wording of your noticings; you aren’t coding the data yet. Noticings would typically be written down as a stream of consciousness, a messy ‘rush of ideas’, rather than polished prose. Such notes are written only for you, to help you with the process of analysis – think of them as memory aids and triggers for developing your analysis. These noticings often reflect what we bring to the data, and while they can enrich our analysis, we should be wary of using them as the main or sole basis for developing our analysis, as they are not based in a systematic engagement with the data. The things that jump out at you initially are likely either the most obvious aspect of the data, or reflect things that are salient to you as a person. For example, when we were familiaris- ing ourselves with the FG data, one of Virginia’s noticings was that physical activity seemed to be framed incredibly negatively by participants, as a chore with no pleasure attached. This likely reflects the fact that she has always been an enthusiastic participant in a variety of exercise that ranges from school PE to football to hiking, and people’s lack of enthusiasm doesn’t resonate with her experience in any way. The negativity around exercise was not one of Victoria’s noticings, which likely reflects that her own experience of exercise is similar to that which participants’ expressed. The point is not that one of us is ‘right’ and one ‘wrong’, but that our personal experiences shape how we read data; they can be a great resource for analysis, but they can also limit what we see in data. You need to recognise this, and reflect on it during the analytic process (see Box 13.2 in Chapter 13). Familiarisation is not a passive process of just understanding the words (or images); it is about starting to read data as data. Reading data as data means not simply absorb- ing the surface meaning of the words (or images), as you typically absorb a crime novel or a Hollywood blockbuster, but reading the words actively, analytically and critically, starting to think about what the data mean. This involves asking questions like: How does a participant make sense of their experiences? Why might they be making sense of their experiences in this way (and not in another way)? In what different ways do they make sense of the topic discussed? How ‘common-sense’ is their story? How would I feel if I was in that situation? (Is this different from or similar to how the participant feels, and why might that be?) What assumptions do they make in talking about the world? What kind of world is ‘revealed’ through their account? The more you engage with the data, the more they ‘open up’ to you, so don’t worry if you feel that you don’t ‘see’ anything beyond the obvious in your data at first. An analytic sensibility is essential for moving beyond a surface, summative reading of the data, and questions like the above will help in developing an analytic sensibility. You don’t need to be overly concerned at this point about the theoretical coherence of your initial noticings either. 09-Braun & Clarke_Ch-09.indd 205 28/02/2013 7:43:50 PM 206 Successfully analysing qualitative data Different analytic approaches to analysis treat these noticings differently. In approaches like TA they may become the initial blocks in the process of coding then building your final analysis. In IPA, in contrast, where the focus is on capturing and interpreting the participants’ experiences, it is recommended that you note down your ideas and observations and then put them aside (basically forget them for a while), so that your analytic eye remains focused on the participants’ meanings and experiences. You may revisit your noticings later as you move to the more researcher-interpretative stages of IPA, but initially you want to stay with the participants’ meanings (Smith et al., 2009). WHAT IS CODING? Coding is a process of identifying aspects of the data that relate to your research ques- tion. There are two main approaches to coding in pattern-based forms of qualitative analysis (see Table 9.1), which we call selective coding and complete coding. SELECTIVE CODING Selective coding involves identifying a corpus of ‘instances’ of the phenomenon that you’re interested in, and then selecting those out. The purpose here is one of ‘data reduction’. Imagine your dataset was a bowl of multi-coloured M&Ms. The process of selective cod- ing is akin to pulling out only the red or yellow ones, and leaving the rest in the bowl. What you gather is a collection of data of a certain type. This approach to coding is often seen as a pre-analytic process, the pragmatic selection of your data corpus, rather than as part of your analysis (e.g. Potter & Wetherell, 1987). However, it does inevitably have an analytic element, in that you need to work out what counts as an instance of what you’re looking for, and where that instance starts and finishes. It also requires pre-existing theoreti- cal and analytic knowledge that gives you the ability to identify the analytic concepts that you’re looking for. The process of reading and familiarisation may be more involved and take longer with this approach than for a complete coding approach, as you have to come to ‘see’ what it is that you will identify, and then selectively code for, in the data. In complete coding, the process itself develops and refines what it is that you are interested in, analyti- cally. Selective coding is most typically used for narrative, discursive and conversa- tion analytic approaches, as well as pattern-based DA, to build a corpus of instances of the phenomenon you’re interested in (Arribas-Ayllon & Walkerdine, 2008; Potter & Wetherell, 1987) – for instance, from Virginia and colleagues’ DA work, all interview talk that invoked the concept of reciprocity when discussing heterosex (Braun, Gavey, & McPhillips, 2003). COMPLETE CODING Complete coding is a rather different process. Instead of looking for particu- lar instances, you aim to identify anything and everything of interest or relevance to answering your research question, within your entire dataset. This means that rather than selecting out a particular corpus of instances which you then analyse, you code all the data that’s relevant to your research question, and it’s only later in the analytic process that you become more selective. 09-Braun & Clarke_Ch-09.indd 206 28/02/2013 7:43:50 PM First analytic steps: familiarisation and data coding 207 In complete coding, codes identify and provide a label for a feature of the data that is potentially relevant for answering your research question. A code is a word or brief phrase that captures the essence of why you think a particular bit of data may be useful. In qualitative research, coding is not an exclusive process, where an excerpt of data can only be coded in one way. Any data extract can and should be coded in as many ways as fits the purpose. For example, if you look at the extract of coded data in Table 9.2, we coded Judy’s line ‘Yeah if people are working hard they want something quick which tends to be the unhealthy food rather than the healthy food’ in three different ways, each capturing different elements in the data that might be useful in our developing analysis: i) common-sense association: working hard and wanting ‘convenient’ (i.e. quick) food; ii) categorisation of food: healthy/unhealthy; good/bad; iii) unhealthy food = quick/ convenient; healthy food = slow/inconvenient. Codes provide the building blocks of analysis: if you imagine your analysis is a brick-built, tile-roofed house, your themes are the walls and roof; your codes the indi- vidual bricks and tiles. In broad terms, codes can either reflect the semantic content of the data (we call these data-derived or semantic codes) or more conceptual or theo- retical interpretations of the data (we call these researcher-derived or latent codes). Different approaches have different labels for these types of codes (see Table 9.1). DATA-DERIVED AND RESEARCHER-DERIVED CODES Data-derived codes provide a succinct summary of the explicit content of the data; they are semantic codes, because they are based in the semantic meaning in the data. When coding participant-generated data, they mirror participants’ language and concepts. In the example of coding in Table 9.2, the codes modern technology facilitates obesity and kids don’t know how to cook directly map onto the content of what the participant has said. As analysts, we haven’t put an interpretative frame around their words. Researcher-derived codes go beyond the explicit content of the data; they are latent codes which invoke the researcher’s conceptual and theoretical frameworks to identify implicit meanings within the data. By implicit meanings, we mean the assumptions and frameworks that underpin what is said in the data. In the example in Table 9.2, the code humans as naturally lazy is a clear example of a researcher-derived code. The partici- pants never actually express this sentiment, but many of the things they say around exer- cise and modern lifestyles rely on this particular understanding of what humans are like. The theoretical and knowledge frameworks you bring will allow you to ‘see’ particular things in the data, and interpret and code them in certain ways; no two analysts will code in exactly the same way. Going back to the idea of the analyst as a sculptor rather than an archaeologist (Chapter 2), two sculptors with different tools, techniques and experiences would produce (somewhat) different sculptures from the same piece of marble. Likewise, two researchers would code the same dataset somewhat differently (see also Chapter 12). This separation between semantic and latent codes is not pure; in practice codes can and do have both elements. A good example of this is the code Gendered safety: (women) feeling unsafe running alone (which you see in the extended version of Table 9.2 on the companion website). It captures the explicit content of what the participant has said – she doesn’t feel safe to run by herself – but then applies an interpretative lens – gender – to it, derived from 09-Braun & Clarke_Ch-09.indd 207 28/02/2013 7:43:50 PM 210 Successfully analysing qualitative data our theoretical and topic-based knowledge. Here, she doesn’t suggest her safety has any- thing to do with her being a woman. However, we suggest ‘safety’ in relation to exercise is a concern typically experienced differently by women and men, reflecting wider gendered safety concerns commonplace in western societies (e.g. Valentine, 1989). New qualitative researchers tend to initially generate mostly data-derived codes as they are easier to identify, and rely less on having conceptual and theoretical knowledge through which to make sense of the data. The ability to generate researcher-derived codes develops with experience, as they require a deeper level of engagement with the data and with fields of scholarship and theorising. This doesn’t mean that researcher- derived codes are inherently better than data-derived ones, but they do assist in devel- oping an interpretative analysis which goes beyond the obvious (see Chapter 11). In certain forms of pattern-based analysis, particularly DA and more theoretical forms of TA, there is a much stronger focus on researcher-derived codes. DOING COMPLETE CODING With complete coding, you begin with your first data item, and systematically work through the whole item, looking for chunks of data that potentially address your research question. You can code in large chunks (e.g. 20 lines of data), small chunks (e.g. a single line of data), and anything in between, as needed. Data that don’t contain anything rele- vant to the research question don’t need to be coded at all. If you are starting with a very broad research question, which you may refine during the analytic process, you want to code widely and comprehensively; if you already have a very specific research question, you may find that large sections of the data are not relevant and don’t need to be coded. The outcome of a first (but thorough) coding of an excerpt of our FG data is provided in Table 9.2 (see the companion website for an extended version). In coding this extract, we were working with a broad research question informed by a constructionist position: ‘In the context of an “obesity epidemic”, how do people make sense of obesity?’ Basically, every time you identify something potentially relevant, code it. Remember, you can code a chunk of data in as many ways as you need (as Table 9.2 shows; but note this shows very detailed coding and coding doesn’t always need to be that detailed, as it depends on your focus). Coding on hard-copy data, clearly writing down the code name, and marking the text associated with it in some way, is common. Other techniques include using specialised computer software (see below), using the comments feature in Microsoft Word, using some kind of a file card system – keep a card for each code, with data summary and location information listed – or cutting and pasting extracts of text into a new word-processing file, created for this purpose (making sure you record where each extract came from). Some methods allow you to collate coded text as you code, which is helpful, but there is no right or wrong way to manage the mechanics of coding. Work out what suits you best. What is important is that coding is inclusive, thorough and systematic, working through each data item in full, before proceeding to the next, except in IPA (we discuss this more below). What makes a good code? Codes should be as concise as possible – except in IPA, where coding can be more akin to writing brief commentaries on the data (see Table 9.3). A code 09-Braun & Clarke_Ch-09.indd 210 28/02/2013 7:43:50 PM First analytic steps: familiarisation and data coding 211 captures the essence of what it is about that bit of data that interests you. Codes should ‘work’ when separated from the data (imagine, horror of horrors, that you lost your data – good codes would be informative enough to capture what was in the data, and your analytic take on it), because you initially develop candidate themes from your codes, and then your coded data, rather than directly from the full data items. This means developing codes may take some thought. When coding the extract in Table 9.2, in Sally’s first response, we first had a code ‘dif- ferent lifestyles’, but ‘different lifestyles’ doesn’t really tell us anything without the data. After thinking it through, and talking about it, we decided that ‘times have changed’ was a better code: it works without the data, and better captures her point that the organisation of our world has changed, meaning people do things differently now. The process continues in the same way for the rest of the data item, and indeed the whole dataset. For each new bit of text you code, you have to decide whether you can apply a code you have already used, or whether a new code is needed to order to capture what it is you’ve identified. Coding is an organic and evolving process. As your coding progresses and you start to understand the shape and texture of your data a bit more, you will likely modify exist- ing codes to incorporate new material. Once you’ve finished the first coding of the dataset, it’s worth revisiting the whole thing, as your codes will probably have developed during coding. Each code should be distinct in some way, so if you have codes that almost com- pletely overlap, a broader code might usefully be developed to reflect the general issue. For instance, if you had some data coded as ‘hates exercise’ and other data coded as ‘doesn’t like exercise’ you might want to merge them into a single code called ‘dislike of exercise’. However, this isn’t always the case, as you may want to preserve such nuanced differences in your coding. In our coded extract (Table 9.2) two similar codes are ‘exer- cise as negative (chore and burden)’ and ‘exercise as negative (inherently unpleasant)’. These may want to be refined down to a broader ‘exercise is negative’ code, depending on the rest of the dataset, and also on the research question. Subtle distinctions in codes are about staying close to the data during coding. Some overlap is likely and not a problem – such overlaps are partly how patterns are formed (see Chapter 10). Ultimately, you want a comprehensive set of codes that differentiates between different concepts, issues and ideas in the data, which has been applied consistently to the dataset. Your motto should be inclusivity. If you are unsure about whether something in the data may be relevant to addressing your research question, code it. It’s much easier to discard codes than go back to the data and recode it all later – although, as noted above, some recoding is typically part of the analytic process. Depending on your topic, dataset and precision in coding, you will have generated any number of codes – there is no maxi- mum, and no minimum. You want to ensure that you have enough codes to capture both the patterning and the diversity within the data. You also want to ensure that the coding of each data item is not entirely idiosyncratic; most of your codes should be evident in more than one data item, and you want some that are evident in many if not most data items. The main exception to this general approach to complete coding occurs with GT, where you aren’t aiming to identify all instances of a code in the dataset, but rather map all the different facets of the concept you’re coding around (Charmaz, 2006; Pidgeon & Henwood, 1996). This is because GT seeks to understand a phenomenon in its entirety, and selects data on that basis (often through theoretical sampling; see Box 3.3 in Chapter 3). In contrast, other approaches, like TA and IPA, seek to understand a phenomenon as it appears within 09-Braun & Clarke_Ch-09.indd 211 28/02/2013 7:43:50 PM 214 Successfully analysing qualitative data the dataset collected. Approaches like TA and IPA are also interested in diversity, but iden- tify it through a comprehensive coding approach. We discuss some of the specifics of GT coding briefly outlining the IPA approach to coding. CODING IN IPA Coding in IPA is referred to as noting or commenting, and unlike other coding, doesn’t aim to produce succinct codes – a code is more like a brief commentary on the data. This commenting occurs at three main levels: descriptive comments focus on the lived worlds and meanings of participants; linguistic comments focus on the language partic- ipants’ use and how they use it to communicate their experiences; abstract or conceptual comments stay with the participant’s experience but interpret it from the researcher’s perspective. Coding in IPA can involve ‘sweeps’ of (reading through) the data – coding at these different levels: 1) to make descriptive comments; 2) to make linguistic com- ments; 3) to make conceptual comments. IPA coding also includes ‘free associating’ where you note whatever comes to mind when reading the data (Smith et al., 2009). In Table 9.3 we provide an example of IPA initial noting (‘coding’) of two excerpts from our weight and obesity FG where one of the participants, Sally, talked about her experi- ence of fatness and weight gain. The extracts come from different points in the FG (see the companion website for the full transcript) – different segments are separated by horizontal black lines. We provide quite detailed comments on these rich extracts, and separate them by ‘type’ of comment. The broad research question is ‘What is the subjec- tive experience of “obesity”?’ CODING IN GROUNDED THEORY Coding in GT covers the whole analytic process; the early stages are typically known as initial coding (Charmaz, 2006) or open coding (Pidgeon & Henwood, 2004). Throughout coding, codes should be refined as necessary until the best possible fit with the data has been determined. Coding in GT has a number of named features. Key is the constant comparative ana- lytic technique, which aims to ensure that the complexity of the data are represented in the analysis by requiring the analyst to constantly move back and forth, to flip flop (Henwood & Pidgeon, 1994), between their developing codes, categories, concepts and the data (Charmaz, 2006). (Although identified here as a key feature of GT, we recommend a recursive approach like this as essential for rigorous qualitative analysis in general.) Codes are the smallest unit of analytic information in GT. As in TA, they are a label applied to a segment of data. They condense, summarise, and potentially provide some analytic ‘handle’ on the data (Charmaz, 2006). Categories are higher-level concepts derived during analysis through clustering codes (Birks, Chapman, & Francis, 2008), akin to themes in TA. Both codes and categories aim to capture concepts (ideas) in the data. Like other methods, GT distinguishes between data-derived codes (in their language: in vivo or member codes) and researcher-derived codes, and coding happens from the very focused and specific to the broader, more conceptual levels, as in TA. Two useful GT techniques are indexing and memo-writing. Indexing refers to the way GT records 09-Braun & Clarke_Ch-09.indd 214 28/02/2013 7:43:51 PM First analytic steps: familiarisation and data coding 215 concepts derived through coding. The aim of indexing is to include all relevant coded material so as to demonstrate fully the diversity of the concept captured by the code. Indexing can take place using a computer or manually, for instance using index cards or Post-it notes (Birks et al., 2008; Pidgeon & Henwood, 1997). Memo writing is a process of recording analytic insights that provide more depth and complexity than codes. Memo writing starts as soon as you have any analytic ideas that that you may want to pursue, and continues throughout the research process, with early memos giving way to advanced memos as the coding (i.e. analysis) develops. Charmaz (2006: 80) recommends you ‘use early memos to explore and fill out your qualitative codes’. Memo writing offers a process for refining and developing your analytic ideas, as you return to past memos and write additional memos on that topic. Memos are the step between analysis and write-up (Charmaz, 2006): in writing memos, you set up the basis for your analytic write-up through the ideas they capture. There are no rules as to how many memos you need, or how frequently you need to write them; they can also serve many different functions (Birks et al., 2008). You shouldn’t struggle over wording – they aren’t polished prose; they’re analytic notes-to-self that can be more or less devel- oped, and may or may not include relevant data extracts. Box 9.1 provides an example of an early memo from our GT analysis of the focus group data. The research question we were working to was ‘What factors are influential in becoming “obese”?’ BOX 9.1 A GT MEMO The ways participants understand obesity (13 July 2010) In order to understand how participants make sense of the causes of obesity, we have to understand how they view obesity itself. In the opening sequences of the data, obesity is framed in a most ‘extreme’ way, and a consensual view is being built up around this – until one participant ‘comes out’ as formerly obese, and still on the ‘boundaries’ (Carla, L71) of obesity, and another participant reveals a similar ‘obese’ past. But even in revealing this ‘fact’ they question the validity of the medical ‘fact’ of obesity; obesity to them is very ‘overweight’, not just ‘overweight’. So even though they speculate that the media probably influence their views in this, they still implicitly work with a model of obesity that is quite different from, and more extreme than, the medical one. They talk about things like ‘averagely overweight’ (Sally, L432) people who are classed as obese, and dispute the validity of this. They present obesity as a rare and non- normative condition (e.g. ‘Britain’s fattest man’ [Sally, L1141-2]; ‘people that you know can’t fit in their bed or can’t fit on a chair’ [Rebecca, L600]), whereas ‘overweight’ is presented as a normative condition, something common (and thus shouldn’t be considered obesity). In addition, GT analysis can also be distinguished by its particular focus on pro- cesses, rather than topics (Charmaz, 2006), and so coding often focuses on data related to actions and processes. This stems from the social interactionist orientation (of some forms of GT), and a view of ‘human beings as active agents in their lives’ (Charmaz, 09-Braun & Clarke_Ch-09.indd 215 28/02/2013 7:43:51 PM 216 Successfully analysing qualitative data 2006: 7). Charmaz (2006: 55) suggests asking a series of questions, including the fol- lowing, when coding, to stay focused on action and process in the data: What process(es) are at issue here? How can I define it? How do the research participant(s) act while involved in this process? What are the consequences of the process? Charmaz also suggests the use of gerunds to help keep the focus on actions and pro- cesses. In GT, this refers to using verbs which end in ‘ing’, such as ‘describing’ or ‘leading’ (in grammar, gerunds are ‘ing’-ending verbs which function as nouns). Using an ‘ing’ word keeps the focus on practices and actions, rather than states our outcomes. So a code using a gerund could be ’fat shaming’; the code captures the idea that sham- ing is a process fat people experience (a code ‘fat shame’ would, by contrast, emphasise a state). It also contains enough information to be informative without the data present. FINISHING UP COMPLETE CODING The final stage of complete coding is collating the coded data. For each individual code, you need to collate together all instances of text where that code appears in the dataset. If some codes cluster together (e.g. have fine distinctions between them, such as ‘exercise as negative [chore and burden]’ and ‘exercise as negative [inherently unpleasant]’), it would probably make sense to collate all data excerpts for the similar codes in one place, instead of collating them for each code individually. This should be determined by the level of similarity in your codes, and how important fine-grained distinctions are likely to be for answering your research question. Codes should be clearly titled, and excerpts of data should be identified to indicate what data item they came from, and where they can be found in that item (e.g. FG1, lines 99–101). Table 9.4 provides examples of some collated coded data for three codes from the FG. IPA is an exception to this, as you code and analyse each data item sequentially. This means that you don’t collate codes and coded data. Instead, after coding a data item, you develop your analysis of that particular data item (see Table 10.2 and Box 10.3 in Chapter 10), before moving to the next one. A detailed comparison of coding in TA, IPA and GT is provided on the companion website. DOING SELECTIVE CODING IN PATTERN-BASED DISCOURSE ANALYSIS We briefly outline the different process for doing selective coding, particularly in rela- tion to pattern-based DA. To do selective coding, you need to know what you’re looking to code before you begin; data familiarisation is thus particularly vital. The basic ele- ments of selective coding include: Identifying what you’re coding for: this involves a) knowing before you start coding what is that you’re looking for; b) looking for it; and c) marking those instances in some way (e.g. on a hard copy of the data). A novice qualitative researcher may benefit by doing more complete coding of the data first, to help identify the instances that you’ll then selectively code for. 09-Braun & Clarke_Ch-09.indd 216 28/02/2013 7:43:51 PM 218 Successfully analysing qualitative data Determining the boundaries of instances: this involves deciding when an instance begins and ends. In some cases, it may be really obvious; in others, you may have to make a judgement. If so, err on the side of over-inclusivity, and include at least a few lines of data on either side of the instance. Collating instances: this involves compiling all instances into a single file. If you are simultaneously coding for two or more phenomena, keep a separate file for each phenomenon you’re looking at (the same data extracts can appear in more than one place, if relevant). Ideally, code as inclusively as possible (Potter & Wetherell, 1987) – for all instances of a phenomenon and anything that vaguely resembles it. It might involve collating all data in which a particular word or topic appears (e.g. talk about causes of obesity). It might be tempting to see selective coding as data selection, rather than analysis, but it is part of the analysis, and is not one step in a linear analytic process. Often additional coding occurs throughout the development of the analysis, as the shape of the analysis takes form. This means some instances will be rejected as no longer relevant, and other data may need to be collated to fully develop and complete the analysis. Continuing our earlier M&M example, after selecting the red and yellow M&Ms as your data, you may decide that the yellow ones don’t fit – and you put them back in the bowl. But you realise that blue M&Ms should be coded, so you need to go back to the bowl (your data) and select out all blue ones and add them to your already selected data (the red M&Ms). Pattern-based DA coding involves a strong focus on researcher-derived codes: rather than developing an analysis that represents the participants’ words or perspectives, the discourse researcher is interested unpicking the language used, to understand its effects within (and beyond) the data (see Chapter 8). They bring their theoretical understanding of language – as productive – to the analysis, and look ‘beneath the semantic surface’ of the data when coding, in order to identify how language produces or reproduces differ- ent versions of reality or particular effects (Arribas-Ayllon & Walkerdine, 2008; Potter & Wetherell, 1987). Coding can occur from quite micro – a few lines of talk – to a more macro focus. In poststructuralist DA, for example, it’s often oriented at a broader level: if we were interested in discourses around obesity, for instance, much of the extract of data in Table 9.4 might be ‘coded’ as evidencing a discourse of modern life. Pattern-based discourse analytic approaches can combine complete and selective coding styles: very broad complete coding followed by selective coding to extract data excerpts of interest, which would then be coded in more detail. So while the require- ment to be systematic applies, the actual mechanics of coding in pattern-based discourse approaches are less defined than for TA, IPA or GT (hence we provide no recommended further reading on coding in pattern-based DA). WHAT ROLE DO COMPUTER PROGRAMS HAVE IN QUALITATIVE CODING AND ANALYSIS? Discussions about the role of computers in qualitative analysis have been happening for over two decades (e.g. Fielding & Lee, 1991). A range of programs, often collectively termed CAQDAS (computer-assisted qualitative data analysis software), is available (widely used 09-Braun & Clarke_Ch-09.indd 218 28/02/2013 7:43:51 PM First analytic steps: familiarisation and data coding 219 Table 9.5 The strengths and limitations of using computer programs in data coding Strengths Limitations Can increase the organisation of data, coding Cost – if you have to buy a program, it may not be and analysis through functioning as on online affordable; commercialisation has been raised as a ‘filing’ system concern in this area in general Allows quick searching for codes, data, and May not be possible to spend time learning to (often) the generation of visual connections use (well) new software in a time-limited (e.g. Can increase efficiency, making the process of seven-month) project coding and analysis quicker. However, this only For some forms of analysis, such as DA, it can take applies if you’re competent with the program, longer (and be unsatisfactory, MacMillan, 2005) or a quick learner (otherwise it can take longer) Risk of ‘usability frustration, even despair and Can give reassurance of comprehensiveness of hopelessness” (Lu & Shulman, 2008: 108) if not coding (but this does depend on you doing it tech-savvy well in the first place) Risk of technologically mediated ‘distancing’ from Subsequently, may increase the rigour of the data – less ‘immersion’ leading to less insight qualitative coding and analysis Can work as a distraction; the technologies can May facilitate visualisation and (thus) be seductive, and assist (fear-induced) analytic- theoretical/analytic development (Konopásek, avoidance – aka procrastination (Bong, 2002) 2008) Carries the temptation to over-code or use May increase transparency of qualitative features of the program not necessary for your research process, as there are clear ‘audit trails’ analysis (Mangabeira, 1995) (see Chapter 12) Risk of producing a focus on quantity – Can you be very useful for managing a large with frequency being mistaken for meaningfulness dataset Risk that the software can promote certain forms Can be useful for team projects of analysis (e.g. tendency towards GT in many Some have particularly argued for the programs, MacMillan & Koenig, 2004), rather compatibility of CAQDAS and GT (Bringer et al., than facilitating the use of a chosen method – this 2006) risks analysis being determined by techniques and technologies, rather than conceptual or other factors (see Chapter 3), a process referred to as methodolatry (Chamberlain, 2000; Reicher, 2000) Programs can contain embedded methodological and theoretical assumptions (often derived from GT), and these need to be critically considered (MacMillan & Koenig, 2004; Mangabeira, 1995) ones include NVivo and ATLAS.ti). Some qualitative researchers revere CAQDAS; others revile it (Lu & Shulman, 2008). Traditionally, CAQDAS has been separable into programs that just allow you to ‘code’ data and then ‘retrieve’ all coded data, and those that allow some ‘conceptual mapping’ of coded data to explore relationships between codes (Fielding & Lee, 1998). While the sophistication and scope of programs have increased over the years (Mangabeira, Lee, & Fielding, 2004), resulting in user-friendly tools that can assist in the 09-Braun & Clarke_Ch-09.indd 219 28/02/2013 7:43:51 PM 220 Successfully analysing qualitative data production of very complex and nuanced analyses, and may aid interpretation and theorising (Silverman, 2005), none escape the fact that qualitative analysis is an interpretative process driven by what the analyst sees in, and makes of, the data. So before you get excited at the thought that a computer can do your analysis, such programs only offer a tool to assist with coding and analysis. That said, they do offer exciting – if still quite modest (Silver & Fielding, 2008) – pos- sibilities, and, if used in a critical, thoughtful, creative and flexible way that serves the needs of the project, driven by the researcher, research questions and research design, have the potential to enhance the process and the outcome of qualitative analysis. Ultimately, whether or not CAQDAS in general is right for you and for a particular project will depend on a number of factors, such as the scope and scale of the project, the research questions, data type and analytic approach (MacMillan, 2005; MacMillan & Koenig, 2004) and your familiarity and comfort with different technologies (Mangabeira et al., 2004). The CAQDAS site at Surrey University, and the hands-on guide they have pub- lished (Lewins & Silver, 2007), offer a useful resource for CAQDAS-related decisions. Table 9.5 summarises some strengths and limitations noted around CAQDAS programs (Bourdon, 2002; Lu & Shulman, 2008; Mangabeira et al., 2004; Roberts & Wilson, 2002; Silver & Fielding, 2008). Whether or not you use CAQDAS, doing analysis requires understanding of the analytic method you are using, and the frameworks in which it is embedded, rather than knowing how to use a CAQDAS program (MacMillan & Koenig, 2004), so any use of CAQDAS does not replace knowledge of an analytic approach. CHAPTER SUMMARY This chapter: outlined the first stages of analysis: familiarisation with your data; defined different types of coding: selective vs. complete; data-driven vs. researcher-driven; demonstrated the process of complete coding; outlined and illustrated differences in complete coding for IPA and GT; discussed the process of selective coding in pattern-based DA; considered the use of computer software in qualitative analysis. ? QUESTIONS FOR DISCUSSION AND CLASSROOM EXERCISES 1 For the coded data in Table 9.2, determine whether each of the codes is data-derived, researcher-derived, or a mix of both. 2 The following data come from a female respondent to the story completion task discussed in Chapter 6, in which a father tells his children he wants to have a ‘sex change’ (see Material Example 6.1). The data are recorded as written, including 09-Braun & Clarke_Ch-09.indd 220 28/02/2013 7:43:51 PM