Data Mining Techniques PDF
Document Details
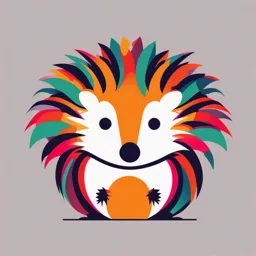
Uploaded by StableBigBen
Brandon University
Tags
Summary
This document provides an overview of data mining techniques across different fields. It explains various concepts and methodologies used in data mining, demonstrating the application of data mining in medicine, agriculture, and business through case studies like in vitro fertilization and dairy farming.
Full Transcript
DM and in vitro Fertilization Human in vitro fertilization involves collecting several eggs from a woman’s ovaries, which, after fertilization with partner or donor sperm, produce several embryos. Some of these are selected and transferred to the woman’s uterus. The challenge is to sel...
DM and in vitro Fertilization Human in vitro fertilization involves collecting several eggs from a woman’s ovaries, which, after fertilization with partner or donor sperm, produce several embryos. Some of these are selected and transferred to the woman’s uterus. The challenge is to select the “best” embryos to use—the ones that are most likely to survive. Selection is based on around 60 recorded features of the embryos DM and in vitro Fertilization The number of features is large enough to make it difficult for an embryologist to assess them all simultaneously and correlate historical data with the crucial outcome of whether that embryo did or did not result in a live child. In a research project in England, data mining has been investigated as a technique for making the selection, using historical records of embryos and their outcome as training data. DM & Dairy Farming Every year, dairy farmers in New Zealand have to make a tough business decision: which cows to retain in their herd and which to sell off to an abattoir. Typically, one-fifth of the cows in a dairy herd are culled each year near the end of the milking season as feed reserves dwindle. Each cow’s breeding and milk production history influences this decision. DM & Dairy Farming Other factors include – age (a cow nears the end of its productive life at eight years), – health problems, – history of difficult calving, – undesirable temperament traits (kicking or jumping fences), – not being pregnant with calf for the following season. About 700 attributes for each of several million cows have been recorded over the years. Data Mining We live in an age where we are overwhelmed with data (data stored in DBs double every 20 months). As the volume of data increases, the proportion of it that we are able to understand and use profitably decreases. Lying hidden in all this data is potentially useful information that is rarely made explicit or taken advantage of. Data mining is about looking (in automated way) for patterns in electronically stored data. Data Mining As the world grows in complexity, overwhelming us with the data it generates, data mining becomes our only hope for elucidating hidden patterns. Intelligently analyzed data is a valuable resource. It can lead to new insights, and, in commercial settings, to competitive advantages. Data Mining Data mining is about solving problems by analyzing data already present in databases. Suppose the problem is fickle customer loyalty in a highly competitive marketplace. A database of customer choices, along with customer profiles, holds the key to this problem. Patterns of behavior of former customers can be analyzed to identify distinguishing characteristics of those likely to switch products and those likely to remain loyal. Data Mining Once such characteristics are found, they can be put to work to identify present customers who are likely to jump ship. This group can be targeted for special treatment, treatment too costly to apply to the customer base as a whole. More positively, the same techniques can be used to identify customers who might be attracted to another service the enterprise provides, one they are not presently enjoying, to target them for special offers that promote this service. Data Mining Data mining is defined as the process of discovering patterns in data. The process must be automatic or (more usually) semiautomatic. The patterns discovered must be meaningful in that they lead to some advantage, usually an economic one. The data is invariably present in substantial quantities. Patterns And how are the patterns expressed? Useful patterns allow us to make nontrivial predictions on new data. There are two extremes for the expression of a pattern: as a black box whose innards are effectively incomprehensible, and as a transparent box whose construction reveals the structure of the pattern. Both, we are assuming, make good predictions. Patterns The difference is whether or not the patterns that are mined are represented in terms of a structure that can be examined, reasoned about, and used to inform future decisions. Such patterns we call structural because they capture the decision structure in an explicit way. In other words, they help to explain something about the data. Thus, we can say that Data Mining is about techniques for finding and describing structural patterns in data. Structural Patterns Consider contact lens data (previous slide). It gives the conditions under which an optician might want to prescribe soft contact lenses, hard contact lenses, or no contact lenses at all. Part of a structural description of this information might be as follows: Structural Patterns Structural descriptions need not necessarily be couched as rules such as these. Decision trees, which specify the sequences of decisions that need to be made along with the resulting recommendation, are another popular means of expression. Contact Lens This example is a very simplistic one. For a start, all combinations of possible values are represented in the table. There are 24 rows, representing three possible values of age and two values each for spectacle prescription, astigmatism, and tear production rate (3 × 2 × 2 × 2 = 24). The rules do not really generalize from the data; they merely summarize it. Contact Lens In most learning situations, the set of examples given as input is far from complete, and part of the job is to generalize to other, new examples. You can imagine omitting some of the rows in the table for which the tear production rate is reduced and still coming up with the rule Contact Lens This would generalize to the missing rows and fill them in correctly. Second, values are specified for all the features in all the examples. Real-life datasets invariably contain examples in which the values of some features, for some reason or other, are unknown—for example, measurements were not taken or were lost. Third, the preceding rules classify the examples correctly, whereas often, because of errors or noise in the data, misclassifications occur even on the data that is used to create the classifier.