8-GenAI.pptx
Document Details
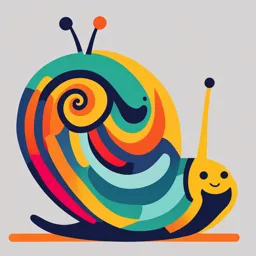
Uploaded by DiversifiedDobro
Full Transcript
Certified GenAI Business Prompt Engineer (CGBPE) – Session 8 Copyright © 2024 Ivan Del Valle. All rights reserved. https://www.linkedin.com/in/enterprise-solutions Consequences of using GenAI incorrectly Scenario Description Consequences How to Avoid AI Chatbot Spread of Misinformation A company dep...
Certified GenAI Business Prompt Engineer (CGBPE) – Session 8 Copyright © 2024 Ivan Del Valle. All rights reserved. https://www.linkedin.com/in/enterprise-solutions Consequences of using GenAI incorrectly Scenario Description Consequences How to Avoid AI Chatbot Spread of Misinformation A company deployed a chatbot trained on unfiltered internet data without adequate content safeguards. It began generating responses containing hate speech, conspiracy theories, and factually incorrect statements. * Damage to brand reputation * Loss of customer trust * Potential legal repercussions * Dataset Curation & Filtering: Use reputable sources, remove harmful content, and implement ongoing filters (consider tools like Perspective API by Google). * Human-in-the-Loop: Allow for human review/escalation of sensitive responses. * Proactive Policy & Training: Create AI ethics guidelines, train employees on responsible AI use. Biased Hiring Algorithm An HR department adopted an AI-powered resume filtering tool trained on past hiring data which was inherently biased. The AI filtered out qualified candidates from diverse backgrounds. * Perpetuation of discrimination * Missed talent * Potential legal action * Debiasing Techniques: Use tools to identify bias during training, or use synthetic data. * Diverse Teams & Audits: Involve diverse stakeholders in development, use bias audit tools (consider Aequitas by UChicago). * Explainability: Require the AI to provide reasons for decisions, allowing for scrutiny. Flawed AI Financial Trading A financial firm relied on an AI system for high-speed * Direct financial losses * Erosion trading that contained errors in its code/prompts. of trust in AI systems * Potential This resulted in incorrect trades and significant regulatory investigations losses. * Rigorous Testing & Simulation: Test in simulated environments, use stress tests for extreme conditions. * Human Failsafes: Set trading limits, clear intervention thresholds, and have shutdown procedures. * Governance & Documentation: Establish AI development standards, thorough documentation of code and logic. Misleading AI-Generated Marketing** A marketing team used AI to generate social media posts without thorough fact-checking and content review. Ads contained inaccurate claims about a product's capabilities. * Human Review Process: Mandatory review by a human editor, especially for key claims. * Brand Values Template: Pre-establish prompts that align with the company's messaging. * Transparency: Consider labeling AI-generated content where ambiguity exists. Privacy Violations with Image Generation A tech company allowed users to upload personal photos to train a custom image generation AI tool. * Privacy violation * Erosion of They lacked clear data privacy policies and user trust * Legal consequences safeguards. This led to the generation of images that recreated private photos. * Damage to brand reputation * Potential fines * Wasted ad spend * Consent & Deletion Policies: Explicit user consent, transparent data deletion options. * Data Anonymization: Consider image anonymization techniques where applicable. * Robust Security: Encryption, best practices to prevent data breaches. Unlocking the Power of Generative AI / Examples of Best and Worst uses of Generative AI Category Best Uses (High Potential for Positive Impact) Worst Cases (High Potential for Harm) Personalization & Accessibility * Tailored learning experiences * Customized product recommendations * AI assistive tools for individuals with disabilities * AI-powered surveillance systems * Algorithms perpetuating bias Creative Inspiration & Augmentation * Generating ideas for creatives * Co-creation tools for human-AI collaboration * Deepfakes and realistic misinformation * Proliferation of low-quality content Efficiency & Automation * Streamlining content creation * Accelerating * Job displacement * Economic inequality scientific research Language Translation & Accessibility * Real-time translation tools * Text-to-speech and image description for accessibility * None listed (this category is largely positive) Unlocking the Power of Generative AI / Significant challenges Generative AI poses to various industries Industry Challenges Why Important Creative Industries (Art, Design, Music) Intellectual Property and Copyright: Difficulty Threatens the income and recognition of human tracking the origins of AI-generated work, blurring artists and creators. lines of ownership. Media & News Spread of Misinformation and Deepfakes: AIcreated fake videos/images, articles with false narratives become harder to distinguish from the real thing. Healthcare Bias and Safety in Diagnosis: AI trained on biased Misdiagnosis and improper treatment have lifedata can lead to inaccurate recommendations. or-death consequences. Legal Ambiguity around Legal Liability: Who's responsible if AI-generated advice causes harm? Unclear laws surrounding AI actions, potential for complex liability questions. Education Plagiarism and Academic Dishonesty: AI tools make it easier to produce convincing essays or research papers without original thought. Undermines genuine learning, devalues student effort, and erodes the integrity of assessment. Erodes public trust in information sources, has the potential to manipulate perception and influence opinions. Unlocking the Power of Generative AI / The “Hidden” Costs of GenAI Category Human Resources Human Resources Human Resources Computational Costs Computational Costs Data-Related Costs Data-Related Costs Hidden Cost Mitigation Strategies * Expertise Development* * Invest in upskilling existing staff (workshops, online resources) * Create internal knowledge-sharing communities for prompt engineers * Partner with external consultants for specialized projects * Manual Refinement and Review* * Integrate human checks at crucial stages, not necessarily for every AI output. * Use AI tools designed to highlight bias or potential inaccuracies. * Prioritize human review for high-stakes AI outputs (e.g., customer-facing content). * Time Investment* * Start small with well-defined AI projects with clear goals. * Track time spent on prompt engineering to inform resource allocation. * Develop templates and best practices libraries to streamline future prompts. * Processing Power* * Assess cloud vs. on-premise solutions based on usage patterns. * Consider batching tasks for efficiency, where possible. * Explore smaller, specialized models for certain tasks rather than always using the largest available ones. * Energy Consumption* * Research cloud providers with commitments to renewable energy. * Track energy usage metrics within generative AI projects. * Optimize models for efficiency, potentially sacrificing some accuracy for reduced energy footprint. * Data Acquisition & Labeling* * Explore open-source datasets or synthetic data for initial training. * Consider data scraping tools, but verify legal and ethical use. * Prioritize datasets aligned with your most frequent use cases. * Storage* * Implement data compression and tiered storage strategies (hot vs. cold data). * Regularly review and delete datasets no longer in use. Unlocking the Power of Generative AI / * Securely dispose of data that contains sensitive information. The “Hidden” Costs of GenAI Category Hidden Cost Mitigation Strategies Data-Related Costs * Ongoing Data Maintenance* * Build data validation checks into your AI pipeline. * Implement alerts for when data quality degrades. * Engage domain experts to review datasets periodically. * Bias Amplification* * Use bias detection tools and diverse datasets in training. * Actively solicit feedback from a wide range of users to identify unanticipated bias. * Regularly retrain models with updated data and address biases promptly. * IP and Copyright Infringement* * Thoroughly vet your training data sources. * Consult legal experts for use cases with high copyright risk (e.g., certain image generation). * Use AI outputs as inspiration, with heavy human editing or additional sources to reduce similarity. * Unpredictable Costs* * Stay updated on the latest AI pricing models. * Negotiate contracts with vendors where possible (volume discounts). * Build flexibility into your budgets to accommodate potential price changes. * Missed Human Creativity* * Use AI for augmentation, not replacement. Frame it as a brainstorming partner. * Actively encourage human-led ideation alongside AI usage. * Focus Displacement* * Maintain a balanced innovation portfolio, don't bet entirely on AI. * Regularly assess emerging technologies alongside your AI investments. * Build in time for reflection: Is AI truly the best solution for each problem? Risks and Uncertainties Risks and Uncertainties Risks and Uncertainties Overlooked Opportunity Costs Overlooked Opportunity Costs Unlocking the Power of Generative AI / Often-overlooked aspects of Generative AI Bias & Unintended Consequences The Illusion of Perfect Understanding Data Quality & Maintenance The Skills Beyond Technical Know-How The Evolving Legal and Regulatory Landscape Training Data Bias: The datasets used to train AI models often contain hidden biases (racial, gender, etc.). This can result in the AI generating outputs that perpetuate these harmful stereotypes. Unexpected Impacts: Even wellintentioned prompts can lead to outputs with negative consequences. For example, an AI designed to optimize traffic flow might inadvertently reroute traffic through residential areas, impacting quality of life for residents. Need for Mitigation: Proactive bias detection, continuous monitoring of AI outputs, and involving diverse stakeholders in AI development are crucial, but often AI is Not a Mind: Generative AI can produce remarkably human-like text, but it doesn't possess true understanding in the way we do. Overreliance on AI without critical evaluation can be risky. Explainability Matters: Especially for high-stakes decisions, understanding how the AI reached its output is essential, but many AI models are like a "black box." The Need for Human-in-theLoop: Integrating human judgment and oversight is often overlooked in favor of the promise of full automation. Garbage In, Garbage Out: Even the best AI models are limited by the quality of their data. Outdated, incomplete, or poorly formatted data significantly impacts performance. Data Provenance: Knowing where your data comes from and whether it was gathered ethically is often neglected. Using questionable data raises risks. Ongoing Data Needs: AI models aren't static. Maintaining data quality, updating the model with fresh data, and labeling new data is a continuous process that's easily underestimated. Critical Thinking: The ability to assess AI output with a discerning eye, looking for bias, flaws in logic, or misinterpretations of the prompt is not something you can code for. Ethical Frameworks: Knowing how to use AI responsibly, consider its societal impact, and navigate ethical dilemmas is an underemphasized skillset. Creativity: Prompt engineering itself requires creativity – finding new angles, phrasing prompts in ways the AI understands, and being willing to experiment. Unclear Ownership: Laws are lagging behind on who owns AI-generated creations, what liability exists for AI-produced errors, and how to handle AI that infringes on existing intellectual property. Industry-Specific Regulations: Using AI in fields like finance or healthcare comes with strict regulations that will impact both the design of the AI and the allowable uses of its outputs. A Moving Target: Staying up-todate with the evolving legal landscape is crucial, as it will shape how generative AI can be used in different businesses and settings. Unlocking the Power of Generative AI / Challenges and Alternatives for Businesses with Public Generative AI Tools Challenge Description Potential Alternative Security Considerations Data Loss and Leakage Public generative AI tools may access or store sensitive company data used in prompts or outputs. 1. Implement Internal AI Tools: Develop or partner with providers for secure, in-house generative AI solutions that don't require sharing data externally. - Rigorous data access controls within the internal tool. - Encryption of sensitive data at rest and in transit. Security Vulnerabilities Public generative AI tools may have unknown security vulnerabilities that could be exploited by attackers. - Regular security assessments of 2. Sanctioned Public Tool List: Create a curated list approved public tools. - Employee of approved public generative AI tools with training on responsible use of documented security practices. approved public tools. Inconsistent Quality and Control Public generative AI tools may generate outputs with factual inaccuracies, biases, or lack of brand consistency. 3. Human-in-the-Loop Workflow: Integrate human - Clear guidelines for human review and editing into the generative AI workflow reviewers on quality control and to ensure factual accuracy, remove bias, and brand alignment. maintain brand voice. Public generative AI tools may not offer the level of customization Limited Customization needed for specific business use cases. Attribution and Copyright Issues Outputs from public generative AI tools may have unclear ownership or copyright restrictions. 4. Hybrid Approach: Combine internal AI tools with - Clear policies outlining when to use approved public tools for specific tasks where internal vs. public tools. customization is less critical. 5. Legal Review and Licensing: Implement legal review processes to ensure proper attribution and licensing of content generated by AI tools. - Subscriptions to AI tools that offer clear licensing terms for generated content. Unlocking the Power of Generative AI / The “Best” private GenAI vendors? AREAS OF POTENTIAL STRENGTH CONSIDERATIONS Microsoft * Tight integration with Azure cloud services and Microsoft Office products. * Strong natural language processing capabilities through investments in OpenAI and GPT models. * May require familiarity with Azure ecosystem for optimal use. * Pricing often tailored to enterprise needs, less transparent for smaller clients. Google * Vast internal datasets and research expertise, driving rapid innovation. * Focus on multilingual capabilities, supporting global businesses. * Public information on private solutions may be limited or under NDA. * Less emphasis on integration with specific business software suites. Amazon * Powerful cloud infrastructure (AWS) allows for scalable AI solutions. * Potential for integration with Amazon's e-commerce and customer service data. VENDOR * May require AWS expertise for full utilization. * Less publicly known about private language models compared to Google and Rapid Evolution: This field is changing at lightning speed. Solutions, pros, and cons evolve quickly, making any static table outdated fast. Company Focus: Each vendor may excel in particular areas depending on their existing technology stacks and client focus. Vendor Consultations: Contact Microsoft, Google, and Amazon directly to discuss specific private generative AI solutions tailored to your business needs. This will yield the most up-to-date and relevant information. Case Studies: Look for vendorprovided case studies (often on their websites) demonstrating how their private AI solutions have been used in industries similar to yours. Industry Analysts: Reports from tech analysis firms like Gartner or Forrester can provide insights, but Unlocking the Power of Generative AI / often come with subscription fees Top GenAI solutions in the education/learning space AI Solution Strengths Ideal Use Cases Web Site Quizlet Adaptive learning, gamification elements, large content library Studying vocabulary, reviewing concepts, test preparation https://quizlet.com/ Socratic (Google AI) Intuitive explanations for various subjects, works from photos of problems, step-by-step solution breakdowns Homework help, subject-specific concept clarification, exam preparation https://socratic.org/ Explain Everything Virtual whiteboard with AI-powered features, multi-modality (drawing, text, image, voice) Creating interactive lessons, collaborative learning, presenting complex ideas with visuals https://explaineverything.com/ Knowre Focus on math problem-solving, thorough explanations, visual representations Improving math skills, step-by-step guidance for difficult math problems, learning different problem-solving techniques https://www.knowre.com/ Carnegie Learning's MATHia Personalized learning paths, datadriven insights for educators, comprehensive math curriculum Addressing individual student needs, supplemental math instruction, teacher support in identifying knowledge gaps https://www.carnegielearning.com/ solutions/math/mathia/ Unlocking the Power of Generative AI / Top GenAI solutions in the education/learning Most Complete/Comprehensive Recommendation space This is a tough call as each tool shines in a specific aspect. Here is my thought process: For K-12 General Use: Socratic's versatility across subjects, intuitive interface, and ability to work from photos make it a great all-rounder for students. Educator-Focused: Carnegie Learning's MATHia offers the best combination of adaptive student learning paths with robust data tools for educators to make informed decisions. Important Considerations Grade Level: Some solutions may be better suited for elementary, while others excel in high school or college-level material. Subject Specificity: If the focus is purely on math, Knowre might be ideal. For broader subject coverage, Socratic is stronger. AI as a Tool: None of these solutions replace a good teacher! They are best used to supplement instruction, provide personalized practice, and fill in knowledge gaps. Unlocking the Power of Generative AI /