7COM2001 Week 02 Lecture A: Law, Standards and Policy PDF
Document Details
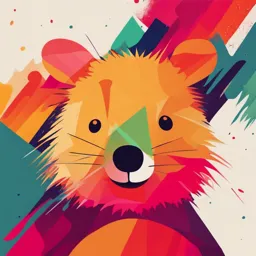
Uploaded by FastestGrowingSwaneeWhistle
University of Hertfordshire
Tags
Summary
This lecture provides an overview of legal areas relevant to computer science, including privacy and data protection, cybercrime, intellectual property, and equality legislation. It also discusses how computer science influences the law and the importance of ethical considerations in technology development.
Full Transcript
1 Law, standards and policy 7COM2001 Responsible Technology Overview 2 In this unit we consider a broad overview of legal areas that are pertinent to computer science Privacy and data protection Cyber...
1 Law, standards and policy 7COM2001 Responsible Technology Overview 2 In this unit we consider a broad overview of legal areas that are pertinent to computer science Privacy and data protection Cybercrime Intellectual property and copyright Equality legislation We also consider the influence of computer science on law Supporting judicial decisions Placing law in context 3 Important to have an understanding of how ethics and law differ Remember the quote about technological innovation outpacing regulation? Another quote that captures our responsibilities: "… law and the rule of law protect what is crucial to consitutional democracy …" "For developers of computational systems, whether based on machine learning, blockchain or other code, knowledge of the law is also crucial because their systems will co-determine law's effectiveness" "If computer systems diminish the substance of human rights or render legal remedies ineffective, they diminish human agency and could even destroy the architecture of constitutional democracy." Hildebrandt (2020), p xi Hildebrandt, M., 2020. Law for computer scientists and other folk. Oxford University Press. Recap – from our introductory discussion 5 Laws are something that we are all bound by within a society: there is no requirement that others are bound by a majority ethical viewpoint (and actually it would be considered unethical to have such a requirement in a democracy) You can dissent or even disobey a law: you may make an ethical decision that you must not comply with a law, for example civil disobedience. In short, a law can be unethical In a democracy we have some control over what laws are introduced Also important to distinguish between policy, legislation, regulations and standard Policy 6 Policy A general statement of intent – what a government (or prospective government) intends to do. Usually enacted through legislation Legislation 7 Legislation (primary legislation) Laws created by some central authority that members of a society are required to follow- in a democracy the central authority is voted in (and out) Regulation 8 Regulation (secondary or delegated legislation) How primary legislation may be implemented. For example, primary legislation may be detailed by domain experts and enforced by a regulator (illegal use of an electromagnetic frequency may be punished by a regulator, likewise the awarding of spectrum bands) Standards 9 Standards Created by an organisation, community. May be de jure (e.g. created through a formal process by experts) or de facto (e.g. emerging from common practice). May inform legislation (e.g. WCAG) 10 Areas of law Of concern to us in our studies on this module Privacy and Data protection 11 You will often come up against privacy and data protection legislation The General Data Protection Regulation – we will provide more details about this to support your independent study. Broadly speaking: Only collect data you need Use it only for the purposes for which you collected the data Ensure data is accurate and timely Users should be able to easily find out what data you hold on them Handle users data securely Example of AWS S3 buckets 12 Leaky buckets were surprisingly common The cloud introduces complexity for operations- seems easy to make configuration errors Exposes data to public Provides information for further attacks (e.g. access credentials have been found, so even properly secured systems may be compromised) Storage of personal data in a leaky bucket tends to breach the data security principle of data protection (and GDPR in practice). Now it is much more difficult to make S3 buckets publicly available AWS shared responsibility model (who is responsible for which aspects of a given system on AWS) relevant here- clients will find it difficult to claim that AWS is liable for accidental disclosure of private information via leaky S3 buckets. Cybercrime 13 Cyber-dependent crimes- require the use of ICT e.g. breaking into systems Cyber-enabled crimes – the use of ICT increases the scale or impact of traditional crimes e.g. fraud, intellectual property crime (counterfeiting, copyright infringement etc.) Source: https://www.cps.gov.uk/legal-guidance/cybercrime-prosecution-guidance Intellectual property and copyright 14 Stock photos – automated search for license infringements Code snippets e.g. from tutorials Use of code libraries and packages – how to keep track? Open source licensing How can a piece of software be used (commercially, only for free software) What are requirements for use e.g. attribution Equality legislation 15 Concerned with people being treated fairly Addressing prohibited behaviours, for example discrimination, based on someone’s characteristics Equality Act (2010) 16 Brings together a range of different pieces of legislation: Sex Discrimination Act 1975 Race Relations Act 1976 Disability Discrimination Act 1995 Protection against discrimination i.e.: direct and indirect discrimination, harassment and victimisation based on protected characteristics Applies to the workplace and society more widely, for example public transport, shops, public events Protected characteristics 17 age disability – we will study this in more detail in the accessibility theme gender reassignment pregnancy and maternity status race religion or belief (including lack of belief) sex sexual orientation marriage and civil partnership status Direct discrimination 18 Discriminating against someone on the basis of a protected characteristic For example: An IT recruitment company only hires people under 25 for junior developer roles Indirect discrimination 19 Creating a circumstance or environment which applies to everyone, but puts an individual or group of people at an unfair disadvantage based on a protected characteristic For example: Requiring a minimum of 5 years experience for a graduate level role will tend to put those aged 21 coming out of University at an unfair disadvantage Requiring all network engineers in your company to adopt flexible shifts without any allowances for those with childcare responsibilities (disproportionately affects women) Harassment 20 Offensive, intimidating, humiliating or degrading behaviour based on a protected characteristic Can be through online media, written word, face to face communication and expressions This is unwanted behaviour and it does not only apply to the person subjected to it. The creation of an environment that is, for example, offensive or intimidating, can mean that others are being harassed. Victimisation 21 Where someone has made a complaint or exercised their rights under the Equality Act or supported someone else to do so and are being treated poorly because of that action Note that this applies to those complaints that are made in good faith. Equality legislation in broader context 22 Example – How to award A-level grades when the exams can not be taken due to Covid-19 Good example of something unethical that is also likely to be illegal. https://www.theguardian.com/education/2020/aug/19/ofqual-exam-results-algorithm-was-unl awful-says-labour?CMP=Share_iOSApp_Other How do you replace public exams for school pupils and still be able to measure their performance reliably (i.e. across the country)? Teachers’ grading and/or algorithm? Breach of education legislation (overseen by a regulator) Potential breach of equal opportunities legislation (schools included in grades estimation algorithm) Case study- Amazon recruitment tool 23 Massive company Many applications for some positions How to pick out the best candidates? Hire more people in Human Resources? Or, build a proof of concept algorithm The algorithm was intended to score applications (1-5) Based on a model based on historic data of the CVs (resumes) sent in Most of these CVs came from men, so most successful applications were from men The algorithm favoured men for new applications… Application of IT to the legal process - COMPAS 24 Correctional Offender Management Profiling for Alternative Sanctions (COMPAS) - A support system for making judicial decisions Not inherently a bad idea: people may make poor, inconsistent decisions. Judicial decisions amongst the most important in society. How can we make better decisions about the likelihood of reoffending But… the algorithm was flawed itself: When it was incorrect in identifying higher risk of re-offending i.e. labelled someone as higher risk for offending who does not actually reoffend, it was twice as likely to identify black people than white people as higher risk for reoffending By contrast, when it was incorrect in identifying lower risk of reoffending i.e. labelling someone as a lower risk for reoffending who then goes onto re-offend, it was much more likely to identify white people than black people as lower risk for reoffending Algorithmic bias- COMPAS 25 Fundamental problem is opacity of algorithm. Laws are debated, legal decisions are subject to review and examination. Can’t do this so easily with proprietary algorithms. Two main issues Algorithmic bias- violates equality legislation The influence of tech on law more generally The influence of tech in law 26 Laws are created with checks and balances and the consent of the people (in democracies). They provide closure, can be enforced Ethics can be created within any organisation (feeds into the organisational culture) i.e. can be created by tech firms for themselves. Now tech firms can come to ethical decisions and force them on users The tech firms can now also bring closure (ethical dilemma is resolved in the firm, the resolution shapes the tech they release, the user uses that tech)- is also force. BUT no other oversight- not like law (at least in democratic systems) Limited (if any) ability to dissent or disobey- you may have a website that includes adverts from a third party. You don’t know how the adverts are chosen for your users, you may be inadvertently supporting decisions that you would not agree with. The ethical decisions of the company have come into force, but you have no room for ethical choice. Did you read about the issue raised by Microsoft, Amazon and others about police use of facial recognition software? They withdrew from market – ethical concerns Who then defines what is ok for facial recognition software? Less scrupulous firms? Motivation is money. It would bring closure to the issue (other firms have to compete) What if the tech is actually involved in the judicial system- as we have seen, this has important implications 27 Any questions? Please ask if you have any questions. herts.ac.uk