Statistics: Hypothesis Testing with Nominal & Ordinal Variables (PDF)
Document Details
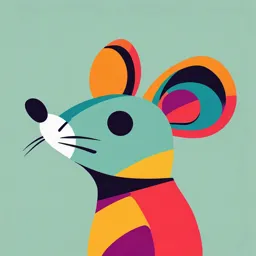
Uploaded by ConscientiousEvergreenForest1127
Toronto Metropolitan University
Tags
Summary
This is a chapter on hypothesis testing with nominal and ordinal variables, focusing on chi-square tests. It explains the five-step process of hypothesis testing, and the key concepts behind this method, such as the null hypothesis, sampling distribution and alpha level.
Full Transcript
Book Title: eTextbook: Statistics: A Tool for Social Research and Data Analysis, Fifth Canadian Edition Chapter 7. Hypothesis Testing with Nominal and Ordinal Variables: Chi Square Chapter 7. Hypothesis Testing with Nominal and Ordinal Variables: Chi Square Learning Objectives By the e...
Book Title: eTextbook: Statistics: A Tool for Social Research and Data Analysis, Fifth Canadian Edition Chapter 7. Hypothesis Testing with Nominal and Ordinal Variables: Chi Square Chapter 7. Hypothesis Testing with Nominal and Ordinal Variables: Chi Square Learning Objectives By the end of this chapter, you will be able to 1. Explain the logic of hypothesis testing 2. Define and explain the conceptual elements involved in hypothesis testing, especially the null hypothesis (and research hypothesis), the sampling distribution, the alpha level, and the test statistic 3. Explain what it means to reject the null hypothesis or fail to reject the null hypothesis 4. List and explain each of the factors (especially sample size) that affect the probability of rejecting the null hypothesis, and explain the differences between statistical significance and importance 5. Identify and cite examples of situations in which the chi square test is appropriate 6. Explain the structure of a bivariate table and the concept of independence as applied to expected and observed frequencies in a bivariate table 7. Explain the logic of hypothesis testing as applied to a bivariate table 8. Perform the chi square test using the five-step model and correctly interpret the results 9. Explain the limitations of the chi square test Book Title: eTextbook: Statistics: A Tool for Social Research and Data Analysis, Fifth Canadian Edition Chapter 7. Hypothesis Testing with Nominal and Ordinal Variables: Chi Square 7.1. Introduction 7.1. Introduction 213 Chapter 6 introduced the techniques for estimating population parameters from sample statistics. Over the next few chapters, we will investigate a second application of inferential statistics called hypothesis testing. We will orient our discussion around level of measurement, as we did when we examined univariate descriptive statistics such as measures of central tendency and dispersion. In this chapter, we will focus on hypothesis-testing procedures when we have two categorical variables—two nominal variables, two ordinal variables, or one nominal and one ordinal variable. We also introduce the basic ideas behind the analysis of association in terms of bivariate tables and column percentages, with a more detailed discussion of bivariate descriptive statistics used to measure association between categorical variables to follow in Chapters 8 and 9. So, our approach is to first test whether a statistically significant relationship exists between our variables, at our chosen level of confidence. If we detect such a relationship, we then use special statistics called measures of association to assess additional characteristics of these relationships, namely their strength and direction. Remember that the use of inferential procedures is only justified when we are using a randomly drawn sample. While it would of course be better if we had access to the full population rather than a sample of it, researchers usually do not have the resources necessary to test everyone in a large group and must instead use random samples. Thanks to the theorems discussed in Chapter 5, we can infer a lot about the population on the basis of the information obtained from our randomly drawn sample. Yet, remember that the EPSEM procedure for drawing random samples does not guarantee representativeness; there will always be a small amount of uncertainty in our conclusions. One of the great advantages of inferential statistics is that we can estimate the probability of error and evaluate our decisions accordingly. Book Title: eTextbook: Statistics: A Tool for Social Research and Data Analysis, Fifth Canadian Edition Chapter 7. Hypothesis Testing with Nominal and Ordinal Variables: Chi Square 7.2. An Overview of Hypothesis Testing 7.2. An Overview of Hypothesis Testing Hypothesis testing, also known as significance testing, is an inferential statistical procedure designed to test for the existence of a relationship between variables, or a difference between groups of cases, at the level of the population. The testing method comprises a distinctive logic based on a comparison of the empirical reality of our actual random sample data to a standard of what we would expect to observe in our sample if there were no relationship between our variables or if there were no difference between groups of cases. Remember that we are interested in the actual situation in the population; however, since we must work with a sample, it is often the case 214 that our sample does not resemble the population perfectly. The relationship or difference that we observe in our sample might therefore be due to random sampling error. So, our attention turns to the question of the size of the observed difference between groups of cases or relationship between variables, for example, the size of the difference in poverty rates of different genders (i.e., the relationship between gender and poverty) in a random sample of 1,000 people. If the size is very small, we would be more inclined to attribute it to random sampling error and to conclude “no difference” or “no relationship”; however, if the size is very large, we would regard a conclusion of “no difference” or “no relationship” as unlikely. Instead, in such a case, we would be more likely to interpret a large discrepancy as pointing toward a real difference between groups or a real relationship between variables in the population. Book Title: eTextbook: Statistics: A Tool for Social Research and Data Analysis, Fifth Canadian Edition Chapter 7. Hypothesis Testing with Nominal and Ordinal Variables: Chi Square 7.3. The Five-Step Model for Hypothesis Testing 7.3. The Five-Step Model for Hypothesis Testing Even though every test of significance is logically unique, all tests of significance follow the same five-step model. The five-step model for hypothesis testing provides a systematic guideline for conducting tests of hypotheses as follows: Step 1. Make assumptions and meet test requirements. Step 2. State the null hypothesis. Step 3. Select the sampling distribution and establish the critical region. Step 4. Compute the test (obtained) statistic. Step 5. Make a decision and interpret the results of the test. We will follow these distinctive steps in every test of significance that we undertake in this and later chapters. Let’s take a closer look at each step. Step 1 Make Assumptions and Meet Test Requirements Any application of statistics requires that certain assumptions be made, and all tests of hypotheses similarly have certain requirements that must be met. First, all tests of hypothesis must be based on a random sample that has been selected according to the rules of EPSEM (see Chapter 5). Most hypothesis tests also have additional requirements pertaining to such considerations as the shape of the population distribution and the level of measurement of the included variables. Step 2 State the Null Hypothesis ( H0 ) The null hypothesis ( H0 ) is the formal name for the statement of “no difference” or “no relationship,” and its exact formulation will vary depending on the specific test being conducted. Usually, however, the researcher believes that a difference between groups, or a relationship between variables, actually does exist and therefore wants to reject the null hypothesis. At this point in the five-step model, the researcher’s belief is stated in the research hypothesis ( H1 ). H1 always directly contradicts H0. Thus, the researcher’s goal in hypothesis testing is often to gather evidence for the research hypothesis by rejecting the null hypothesis. 215 Step 3 Select the Sampling Distribution and Establish the Critical Region The sampling distribution is the probabilistic yardstick against which a particular sample outcome is measured. Specifically, by assuming that the null hypothesis is true (and only by this assumption), we can measure the probability of any specific sample outcome using the sampling distribution. There are several different sampling distributions, and we choose the distribution that is appropriate for our particular hypothesis test. The sampling distributions that we will use for significance testing in this textbook are the standard normal (Z) distribution, the Student’s t distribution, the chi square distribution, and the F distribution. You are already familiar with the Z and t distributions, which we used to construct confidence intervals to estimate population means and proportions, and the chi square distribution is introduced later in this chapter and the F distribution in Chapter 12. The critical region consists of the areas under the sampling distribution that include unlikely sample outcomes. Before conducting the test of hypothesis, we must define what we mean by unlikely. This decision rule will establish the critical region or region of rejection. The word region is used because, essentially, we are describing those areas under the sampling distribution that contain the unlikely sample outcomes. In other words, we must specify in advance those sample outcomes that are so unlikely that they will lead us to reject the null hypothesis, thus lending credibility to our research hypothesis. Conversely, if our sample does not have a value in the critical region, then we will fail to reject the null hypothesis—a decision that makes our research hypothesis seem less likely to be true. By convention, the size of the critical region is reported as the alpha level (α) , the proportion of all of the area included in the critical region. One very commonly used alpha level is 0.05. Others are 0.10, 0.01, and 0.001. Step 4 Compute the Test Statistic To evaluate the probability of any given sample outcome, the sample value must be converted into a test score, such as the Z score (as we will do in Chapter 10) or the chi square score (as we will do later in this chapter). Finding this test score is called computing the test statistic, and the resulting value will be referred to as the obtained score to differentiate it from the score that marks the beginning of the critical region, which is called the critical value. It is also important at this point to distinguish between two closely related concepts, the alpha level, α , and the p value (p stands for probability and should not be confused with a proportion). While p and α are both probabilities, they differ in an important way. As noted in step 3, the alpha level tells us the amount of probability, or the proportion of the area, in the critical region of the sampling distribution. Figure 7.1 illustrates using the normal (Z) sampling distribution, where the critical value marks the beginning of the critical region (green-shaded area). The critical value and region are arbitrarily chosen by the researcher (e.g., α = 0.05 ) before the data analysis. On the other hand, the p value is the amount of probability, or the proportion of the area, in the sampling distribution beyond the obtained score. So, the obtained score and its p value are a product of the actual data, while the critical value and its α level are theoretical and used for comparative purposes —the critical value is the point in the sampling distribution that is compared to the test (obtained) score to decide if the null hypothesis should be rejected. Figure 7.1 Comparison of Alpha Level ( α ) and p Value 216 Step 5 Make a Decision and Interpret the Results of the Test As the last step in the hypothesis-testing process, the test (obtained) statistic is compared with the critical value. If the test statistic (obtained score) is larger than the critical value (i.e., if it falls in the critical region), our decision will be to reject the null hypothesis. If the test statistic is smaller than the critical value (i.e., it does not fall in the critical region), we fail to reject the null hypothesis. Note that there are two parts to step 5. First, we make a decision about the null hypothesis: If the test statistic falls in the critical region, we reject the H0. If the test statistic does not fall in the critical region, we fail to reject the H0. Second, and just as important, we need to interpret the results of the test and say what our decision means. The procedures for making a decision about the null hypothesis and interpreting the results of the test are summarized in Table 7.1. Table 7.1 Making a Decision in Step 5 and Interpreting the Results of the Test Situation Decision Interpretation The test statistic is in Reject the null The difference is the critical region hypothesis (H0) statistically significant The test statistic is Fail to reject the null The difference is not not in the critical hypothesis (H0) statistically region significant 217 Also note that, rather than using this “critical value” method, we can use the “p value” method to come to the same decision—if the p value is less than the alpha level, α , then we reject the null hypothesis. Figure 7.1 provides an example where the p value is below (less than) the alpha level α , so we reject the null hypothesis. Statistical software packages like SPSS calculate both the test (obtained) statistic and its associated p value, and either method can be used to test a hypothesis. This five-step model will serve us as a framework for decision making throughout the hypothesis-testing chapters. The exact nature and method of expression for our decisions will be different for different situations. However, familiarity with the five-step model will help you master this material by providing a common frame of reference for all significance testing. Book Title: eTextbook: Statistics: A Tool for Social Research and Data Analysis, Fifth Canadian Edition Chapter 7. Hypothesis Testing with Nominal and Ordinal Variables: Chi Square 7.4. Selecting an Alpha Level 7.4. Selecting an Alpha Level In order to determine the critical region, the researcher must select an alpha level, as mentioned in Step 3. The alpha level plays a crucial role in hypothesis testing, because it is our selection of alpha that determines exactly what we mean by an unlikely sample outcome. If the probability of the observed sample outcome is lower than the alpha level (i.e., if the test statistic falls in the critical region), we reject the null hypothesis as unlikely to be true. Thus, the alpha level has important consequences for our decision in step 5. How can reasonable decisions be made with respect to the value of alpha? Recall that the alpha level defines what is meant by unlikely. The alpha level also tells us the probability that the decision to reject the null hypothesis, if the test statistic falls into the critical region, is incorrect. In hypothesis testing, the error of incorrectly rejecting the null hypothesis, or rejecting a null hypothesis that is actually true, is called a Type I error, or alpha error. A Type I error can be thought of as a “false positive” outcome of a criminal trial, such that a jury finds the accused guilty of the crime despite their actually being not guilty. The null hypothesis in this example is that the person is not guilty, and the research hypothesis is that the person is guilty. To minimize Type I errors, use very small values for alpha. 218 To elaborate, when an alpha level is specified, the sampling distribution is divided into two sets of possible sample outcomes. The critical region includes all unlikely or rare sample outcomes. Outcomes in this region cause us to reject the null hypothesis. The remainder of the area consists of all sample outcomes that are “non-rare.” The lower the level of alpha, the smaller the critical region and the greater the distance between the mean of the sampling distribution and the beginning of the critical region. Thus, the lower the alpha level, the harder it is to reject the null hypothesis and, because a Type I error can be made only if our decision in Step 5 is to reject the null hypothesis, the lower the probability of a Type I error. However, there is a complication. As the critical region decreases in size (i.e., as alpha levels decrease), the non-critical region must become larger. All other things being equal, the lower the alpha level, the less likely it is that the sample outcome falls in the critical region. This raises the possibility of a second type of incorrect decision, called a Type II error, or beta (β) error: failing to reject a null hypothesis that is, in fact, false. A Type II error is thus analogous to a “false negative” outcome of a criminal trial, such that a jury finds a person not guilty of the crime despite their actually being guilty. In sum, the probability of a Type I error decreases as the alpha level decreases, but the probability of a Type II error increases. The two types of error are inversely related, and it is not possible to minimize both in the same test. In conducting a hypothesis test, we calculate the probability of committing a Type I error (which we control by adjusting the alpha level), but not the probability of committing a Type II error—this remains unknown. Through additional computation, however, we can calculate the probability of not committing a Type II error—in other words, the probability of correctly rejecting the null hypothesis, or making the right decision! This is referred to as the power of a hypothesis test. The routines for computing the power of a hypothesis test will not be considered in this textbook. We must also be aware that there are different consequences associated with making each type of error. For example, in determining guilt during a criminal trial, sending an innocent person to jail (Type I error) has far different consequences than those that result from setting a guilty person free (Type II error). Which of these errors is more serious depends on the social costs related to them. Canadians tend to be distressed when Type II errors occur in the justice system, but outraged by Type I errors. The Canadian justice system, therefore, places great importance on decreasing Type I errors (sending innocent people to jail) at the expense of increasing Type II errors (letting guilty people go free). It may be helpful to clarify the relationships between decision making and errors in table format. Table 7.2 lists the two decisions we can make in step 5 of the five-step model: we either reject or fail to reject the null hypothesis. The other dimension of Table 7.2 lists the two possible conditions of the null 219 hypothesis: it is either actually true or actually false. The table combines these possibilities into a total of four possible combinations, two of which are desirable (“OK”) and two of which are errors. The two desirable outcomes are rejecting null hypotheses that are actually false and failing to reject null hypotheses that are actually true. The goal of any scientific investigation is to verify true statements and reject false statements. Table 7.2 Decision Making and the Null Hypothesis Decision The H0 Is Actually Reject Fail to Reject True Type I or α error OK False OK Type II or β error The remaining two combinations are errors or situations that, naturally, we wish to avoid. If we reject a null hypothesis that is in fact true, we are saying that a true statement is false. Likewise, if we fail to reject a null hypothesis that is in fact false, we are saying that a false statement is true. We want to wind up in one of the boxes labelled “OK” in Table 7.2—to always reject false statements and accept the truth when we find it. Remember, however, that hypothesis testing always carries an element of risk and that it is not possible to minimize the chances of both Type I and Type II errors simultaneously. What all of this means, finally, is that you must think of selecting an alpha level as an attempt to balance the two types of error. Higher alpha levels will minimize the probability of a Type II error (saying that false statements are true), and lower alpha levels will minimize the probability of a Type I error (saying that true statements are false). Normally, in social science research, we want to minimize Type I errors, so lower alpha levels (0.05, 0.01, 0.001, or lower) are used. The 0.05 level in particular has emerged as a generally recognized indicator of a significant result. However, the widespread use of the 0.05 level is simply a convention, and there is no reason that alpha cannot be set at virtually any sensible level (such as 0.04, 0.027, or 0.083). The researcher is responsible for selecting the alpha level that seems most reasonable in terms of the goals of the research project. Book Title: eTextbook: Statistics: A Tool for Social Research and Data Analysis, Fifth Canadian Edition Chapter 7. Hypothesis Testing with Nominal and Ordinal Variables: Chi Square 7.5. Introduction to Chi Square 7.5. Introduction to Chi Square The chi square test is probably the most frequently used test of hypothesis in the social sciences, a popularity that is due largely to the fact that the 220 assumptions and requirements in step 1 of the five-step model are easy to satisfy. Specifically, the test can be conducted with variables measured at any level of measurement, and because it is a non-parametric or “distribution- free” test, it requires no assumptions at all about the shape of the population distribution. While we discuss other non-parametric tests of significance, such as the Mann–Whitney U test and the Wald–Wolfowitz runs test, on the website for this textbook, the chi square test is the focus of this chapter. Why is it an advantage to have assumptions and requirements that are easy to satisfy? The decision to reject the null hypothesis (Step 5) is not specific: it means only that one statement in the model (Step 1) or the null hypothesis (Step 2) is wrong. Usually, of course, we single out the null hypothesis for rejection. The more certain we are of the model, the greater our confidence that the null hypothesis is the faulty assumption. A “weak” or easily satisfied model means that our decision to reject the null hypothesis can be made with even greater certainty. Chi square is also popular for its flexibility. It can be used not only with variables at any level of measurement but also with variables that have many categories or scores. However, while chi square can be used at any level of measurement, it is most appropriate for categorical—i.e., nominal and ordinal —variables. Book Title: eTextbook: Statistics: A Tool for Social Research and Data Analysis, Fifth Canadian Edition Chapter 7. Hypothesis Testing with Nominal and Ordinal Variables: Chi Square 7.6. Bivariate Tables 7.6. Bivariate Tables Chi square is computed from bivariate tables, so called because they display the scores of cases on two different variables at the same time. Bivariate tables are used to ascertain whether there is a significant relationship between the variables and for other purposes that we will investigate in later chapters. In fact, these tables are very commonly used in research, and a detailed examination of them is in order. First of all, bivariate tables have (of course) two dimensions. The horizontal (across) dimension is referred to in terms of rows, and the vertical dimension (up and down) is referred to in terms of columns. Each column or row represents a score on a variable, and the intersections of the rows and columns (cells) represent the various combined scores on both variables. Let’s use an example to clarify. Suppose a researcher is interested in the relationship between place of birth and volunteering. Is there a difference in the level of involvement of Canadians in volunteering depending on place of birth? For the sake of simplicity, let us assume that level of involvement has only two response categories. That is, people have been classified as either born in Canada or not born in Canada and as either high or low in their level of involvement in volunteer associations. 221 By convention, the independent variable (the variable taken to be the cause) is placed in the columns and the dependent variable (the variable taken to be the effect) is placed in the rows. In the example at hand, place of birth is the causal variable (the question was, “Is membership affected by place of birth?”), and each column represents a score on this variable. Each row, on the other hand, represents a score on level of participation (high or low). Table 7.3 displays the outline of the bivariate table for a sample of 100 people. Table 7.3 Level of Participation in Voluntary Associations by Sex for 100 Citizens Note some further details of the table. First, subtotals have been added to each column and row. These are called the row or column marginals, and in this case, they tell us that 50 members of the sample were born in Canada and 50 were not born in Canada (the column marginals) and 50 were rated as high in participation and 50 were rated as low (the row marginals). Second, the total number of cases in the sample (n = 100) is reported at the intersection of the row and column marginals. Finally, take careful note of the labelling of the table: each row and column is identified, and the table has a descriptive title that includes the names of the variables, with the dependent variable listed first. Clear, complete labels and concise titles should be included in all tables, graphs, and charts. As you probably noticed, Table 7.3 lacks one piece of crucial information: the frequency of each place of birth that rated high or low on the dependent variable. To finish the table, we need to classify each member of the sample in terms of both their place of birth and their level of participation, keep count of how often each combination of scores occurs, and record these numbers in the appropriate cell of the table. Because each of our variables (place of birth and participation level) has two scores, there are four possible combinations of scores, each corresponding to a cell in the table. For example, people born in Canada with high levels of participation are counted in the upper left cell, people not born in Canada with low levels of participation are counted in the lower right cell, and so forth. When we are finished counting, each cell will display the number of times each combination of scores occurred. Finally, note how the bivariate table can be expanded to accommodate variables with more than just two scores. For instance, if we have measured participation level with three categories (e.g., high, moderate, and low) rather than two, we simply add an additional row to the table. 222 Book Title: eTextbook: Statistics: A Tool for Social Research and Data Analysis, Fifth Canadian Edition Chapter 7. Hypothesis Testing with Nominal and Ordinal Variables: Chi Square 7.7. The Logic of Chi Square 7.7. The Logic of Chi Square The chi square test has several different uses. This chapter deals with an application called the chi square test for independence. In the context of chi square, the concept of independence refers to the relationship between the variables. Specifically, two variables are independent if the classification of a case into a particular category of one variable has no effect on the probability that the case will fall into any particular category of the second variable. For example, place of birth and participation in volunteer associations are independent of each other if the classification of a case as born in Canada or not has no effect on the classification of the case as high or low on participation. In other words, the variables are independent if level of participation and place of birth are completely unrelated to each other. Consider Table 7.3 again. If these two variables are truly independent, the cell frequencies will be determined solely by random chance and we will find that, just as an honest coin shows heads about 50% of the time when flipped, about half the respondents born in Canada rank high on participation and half rank low. The same pattern holds for the 50 respondents not born in Canada; therefore, each of the four cells will have about 25 cases in it, as illustrated in Table 7.4. This pattern of cell frequencies indicates that the place of birth of the subjects has no effect on the probability that they are either high or low in participation. The probability of being classified as high or low will be 0.50 for Canadians born both in and outside of Canada, and the variables are therefore independent. Table 7.4 The Cell Frequencies That Would Be Expected if Levels of Participation and Sex Were Independent The null hypothesis for chi square is that the variables are independent. Under the assumption that the null hypothesis is true, the cell frequencies we would expect to find if only random chance were operating are computed. These frequencies, called expected frequencies (fe) , are then compared, cell by cell, with the frequencies actually observed (observed frequencies (fo) ) and recorded in a table. If the null hypothesis is true and the variables are independent, then there should be little difference between the expected and observed frequencies. If the null hypothesis is false, however, there should be large differences between the two. The greater the differences between 223 expected (fe) and observed (fo) frequencies, the less likely the variables are independent and the more likely we will be able to reject the null hypothesis. Book Title: eTextbook: Statistics: A Tool for Social Research and Data Analysis, Fifth Canadian Edition Chapter 7. Hypothesis Testing with Nominal and Ordinal Variables: Chi Square 7.8. Computing Chi Square 7.8. Computing Chi Square As with all tests of hypothesis, with chi square we compute a test statistic, χ2 (obtained), from the sample data and then place that value on the sampling distribution of all possible sample outcomes. Specifically, the value of χ2 (obtained) is compared with the value of χ2 (critical), which is determined by consulting a chi square table (Appendix C) for a particular alpha level and number of degrees of freedom, df. Like the t statistic (Chapter 6), chi square (χ2) is a family of sampling distributions based on degrees of freedom—there is a unique distribution for each number of degrees of freedom. However, there is a different formula for calculating degrees of freedom for chi square. As we will see in Section 7.9, it is based on the number of rows and columns in a bivariate table and not on sample size. For example, a table with two rows and columns will always have one degree of freedom. Figure 7.2 shows four chi square distributions, where df = 1 , 5, 10, and 20. The critical value is the beginning of the critical region α (shaded area). For illustration purposes, α is set at 0.05 for each distribution. For example, the distribution for one degree of freedom (df = 1) has a critical value of 3.841, as per Appendix C. This value marks the beginning of the critical region for this distribution. For the other distributions, df = 5 , 10, and 20, the critical values are 11.070, 18.307, and 31.410, respectively. We will learn how to find degrees of freedom and critical values using Appendix C in the next section. Figure 7.2 Chi Square Distributions at Various Degrees of Freedom 224 A few features of the chi square distribution are worth noting. First, the distribution starts at zero. Second, it has a positive skew. Third, it becomes more symmetric and normal in shape as the degrees of freedom increase. This is not a coincidence! The chi square and normal (Z) distributions are mathematically related. As the degrees of freedom increase toward infinity, the chi square distribution, like the t distribution, approaches a normal curve. Before we can use the chi square sampling distribution to conduct a formal hypothesis test, we need to compute the test statistic, (χ2) (obtained), as defined by Formula 7.1: Formula 7.1 2 (fo − fe)2 χ (obtained) = Σ fe where fo = the cell frequencies observed in the bivariate table fe = the cell frequencies that would be expected if the variables were in We must work on a cell-by-cell basis to solve this formula. To compute chi square, subtract the expected frequency from the observed frequency for each cell, square the result, divide by the expected frequency for that cell, and then sum the resultant values for all cells. This formula requires an expected frequency for each cell in the table. In Table 7.4, the marginals are the same value for all rows and columns, and the expected frequencies are obvious by intuition: fe = 25 for all four cells. In the more usual case, the expected frequencies are not obvious because the marginals are unequal, and we must use Formula 7.2 to find the expected frequency for each cell: Formula 7.2 Row marginal × Column marginal fe = n That is, the expected frequency for any cell is equal to the total number of cases in the row (the row marginal) times the total number of cases in the column (the column marginal) divided by the total number of cases in the table (n). An example using Table 7.5 should clarify these procedures. A random sample of 100 social work graduates has been classified in terms of whether the Canadian Association of Schools of Social Work (CASSW) has accredited their undergraduate programs (the column or independent variable), and whether these graduates were hired in social work positions within three months of graduation (the row or dependent variable). 225 Table 7.5 Employment of 100 Social Work Graduates by CASSW Accreditation Status of Undergraduate Program (Fictitious Data) Beginning with the upper left cell (graduates of CASSW-accredited programs who are working as social workers), the expected frequency for this cell, using Formula 7.2, is (40)(55)/100, or 22. For the other cell in this row (graduates of non-accredited programs who are working as social workers), the expected frequency is (40)(45)/100, or 18. For the two cells in the bottom row, the expected frequencies are (60)(55)/100, or 33, and (60)(45)/100, or 27, respectively. The expected frequencies for all four cells are displayed in Table 7.6. Table 7.6 Expected Frequencies for Table 7.5 Note that the row and column marginals as well as the total number of cases in Table 7.6 are exactly the same as those in Table 7.5. The row and column marginals for the expected frequencies must always equal those of the observed frequencies, a relationship that provides a convenient way of checking your arithmetic to this point. The value for chi square for these data can now be found by solving Formula 7.1. It is helpful to use a computing table, such as Table 7.7, to organize the several steps required to compute chi square. The table lists the observed frequencies (fo) in column 1 in order from the upper left cell to the lower right cell, moving left to right across the table and top to bottom. Column 2 lists the expected frequencies (fe) in exactly the same order. Double-check to make sure you have listed the cell frequencies in the same order for both columns. Table 7.7 Computational Table for Table 7.5 The next step is to subtract the expected frequency from the observed frequency for each cell and list these values in column 3. To complete column 4, square the value in column 3, and then, in column 5, divide the column 4 value by the expected frequency for that cell. Finally, add up column 5. The sum of this column is χ2 (obtained): χ2(obtained) = 10.78 226 Note that the totals for columns 1 and 2 ( fo and fe ) are exactly the same. This is always the case, and if the totals do not match, you have probably made a mistake in the calculation of the expected frequencies. Also note that the sum of column 3 is always zero, another convenient way to check your math to this point. This sample value for chi square must still be tested for its significance. (For practice in computing chi square, see any of the end-of-chapter problems.) One Step at a Time Computing Chi Square Begin by preparing a computing table similar to Table 7.7. List the observed frequencies (fo) in column 1. The total for column 1 is the number of cases (n). To Find the Expected Frequencies (fe) Using Formula 7.2 1: Start with the upper left cell, and multiply the row marginal by the column marginal for that cell. 2: Divide the quantity you found in Step 1 by n. The result is the expected frequency (fe) for that cell. Record this value in the second column of the computing table. Make sure you place the value of fe in the same row as the observed frequency for that cell. 3: Repeat Steps 1 and 2 for each cell in the table. Double-check to make sure you are using the correct row and column marginals. Record each fe in the second column of the computational table. 4: Find the total of the expected frequencies column. This total must equal the total of the observed frequencies column (which is the same as n). If the two totals do not match (within rounding error), recompute the expected frequencies. To Find Chi Square Using Formula 7.1 1: For each cell, subtract the expected frequency (fe) from the observed frequency (fo) ; then list these values in the third column of the computational table (fo − fe). Find the total for this column. If this total is not zero, you have made a mistake and need to check your computations. 2: Square each of the values in the third column of the table and record the result in the fourth column, labelled (fo − fe)2. 3: Divide each value in column 4 by the expected frequency for that cell; then record the result in the fifth column, labelled (fo − fe)2/fe. 2: Find the total for the fifth column. This value is (χ2) (obtained). Book Title: eTextbook: Statistics: A Tool for Social Research and Data Analysis, Fifth Canadian Edition Chapter 7. Hypothesis Testing with Nominal and Ordinal Variables: Chi Square 7.9. The Chi Square Test for Independence 7.9. The Chi Square Test for Independence 227 As always, the five-step model for significance testing provides the framework for organizing our decision making. The data presented in Table 7.5 serves as our example. Step 1 Make Assumptions and Meet Test Requirements First, the sample must be selected according to the rules of EPSEM. Second, the variables must be measured at the nominal or ordinal level. Note that we make no assumptions about the shape of the sampling distribution. Model: Random sampling Level of measurement is nominal or ordinal. Step 2 State the Null Hypothesis As stated previously, the null hypothesis in the case of chi square states that the two variables are independent. If the null hypothesis is true, the differences between the observed and expected frequencies will be small. As usual, the research hypothesis directly contradicts the null hypothesis. Thus, if we reject H0 , the research hypothesis is supported: H0 : The two variables are independent. (H1 : The two variables are dependent.) Step 3 Select the Sampling Distribution and Establish the Critical Region The sampling distribution of sample chi squares, unlike the Z and t distributions, is positively skewed, with higher values of sample chi squares in the upper tail of the distribution (to the right). Thus, with the chi square test, the critical region is established in the upper tail of the sampling distribution. Values for χ2 (critical) are given in Appendix C. This table is similar to the t table, with alpha levels arrayed across the top and degrees of freedom down the side. A major difference, however, is that degrees of freedom (df) for chi square are found by the following formula: Formula 7.3 df = (r − 1)(c − 1) where (r − 1)(c − 1) = r − 1(number of rows minus 1) multiplied by c − 1 (number of columns minus 1) A table with two rows and two columns ( 2 × 2 table) has one degree of freedom regardless of the number of cases in the sample. A table with two rows and three columns has (2 − 1)(3 − 1) or two degrees of freedom. Our sample problem involves a 2 × 2 table with df = 1 , so if we set alpha at 0.05, the critical chi square score is 3.841. Summarizing these decisions, we have Sampling distribution = χ2 distribution Alpha = 0.05 Degrees of freedom = 1 χ2 (critical) = 3.841 228 Step 4 Compute the Test Statistic The mechanics of these computations were introduced in Section 7.8. As you recall, we had 2 (fo − fe)2 χ (obtained) = Σ fe = 10.78 Step 5 Make a Decision and Interpret the Results of the Test Comparing the test statistic with the critical region, χ2(obtained) = 10.78 χ2(critical) = 3.841 we see that the test statistic falls in the critical region, and, therefore, we reject the null hypothesis of independence. The pattern of cell frequencies observed in Table 7.5 is unlikely to have occurred by chance alone. The variables are dependent. Specifically, based on these sample data, the probability of securing employment in the field of social work is dependent on the CASSW accreditation status of the program. (For practice in conducting and interpreting the chi square test for independence, see Problems 7.4 to 7.15.) Let’s take a moment to stress exactly what the chi square test does and does not tell us. A significant chi square means that the variables are (probably) dependent on each other in the population: CASSW accreditation status makes a difference in whether or not a person is working as a social worker. Chi square does not give us any detail about the relationship, however. In our example, it does not tell us if it’s the graduates of the accredited programs or the non-accredited programs that are more likely to be working as social workers. To compare these two groups, we must perform some additional calculations. We can figure out how the independent variable (CASSW accreditation status) is affecting the dependent variable (employment as a social worker) by computing column percentages, which is done by calculating percentages within each column of the bivariate table. This procedure is analogous to calculating percentages for frequency distributions (see Chapter 2). 229 To calculate column percentages, divide each cell frequency by the total number of cases in the column (the column marginal). For Table 7.5, starting in the upper left cell, we see that there are 30 cases in this cell and 55 cases in the column. In other words, 30 of the 55 graduates of CASSW-accredited programs are working as social workers. The column percentage for this cell is therefore (30/55) × 100 = 54.55%. For the lower left cell, the column percentage is (25/55) × 100 = 45.45%. For the two cells in the lower right column (graduates of non-accredited programs), the column percentages are (10/45) × 100 = 22.22% and (35/45) × 100 = 77.78%. All column percentages are displayed in Table 7.8. Table 7.8 Column Percentages for Table 7.5 Column percentages help make the relationship between the two variables more obvious. We can see easily from Table 7.8 that students from CASSW- accredited programs are more likely to be working as social workers. Nearly 55% of these students are working as social workers versus less than 25% of the students from non-accredited programs. We already know that this relationship is significant (unlikely to be caused by random chance), and now, with the aid of column percentages, we know how the two variables are related. According to these results, graduating from a CASSW-accredited program is a decided advantage for people seeking to enter the social work profession. One Step at a Time Computing Column Percentages 1: Start with the upper left cell. Divide the cell frequency (the number of cases in the cell) by the total number of cases in that column (or the column marginal). Multiply the result by 100 to convert to a percentage (%). 2: Move down one cell and repeat step 1. Continue moving down the column, cell by cell, until you have converted all cell frequencies to percentages. 3: Move to the next column. Start with the cell in the top row and repeat step 1 (making sure you use the correct column total in the denominator of the fraction). 4: Continue moving down the second column until you have converted all cell frequencies to percentages. 4: Continue these operations, moving from column to column, one at a time, until you have converted all cell frequencies to percentages. 230 Let’s highlight two points in summary: 1. Chi square is a test of statistical significance. It tests the null hypothesis that the variables are independent in the population. If we reject the null hypothesis, we are concluding, with a known probability of error (determined by the alpha level), that the variables are dependent on each other in the population. In the terms of our example, this means that CASSW-accreditation status makes a difference in the likelihood of finding work as a social worker. By itself, however, chi square does not tell us the exact nature of the relationship. 2. Computing column percentages allows us to examine the bivariate relationship in more detail. By comparing the column percentages for the various scores of the independent variable, we can see exactly how the independent variable affects the dependent variable. In this case, the column percentages reveal that graduates of CASSW-accredited programs are more likely to find work as social workers. We will explore column percentages more extensively when we discuss bivariate association in Chapters 8 and 9. Book Title: eTextbook: Statistics: A Tool for Social Research and Data Analysis, Fifth Canadian Edition Chapter 7. Hypothesis Testing with Nominal and Ordinal Variables: Chi Square 7.10. The Limitations of Hypothesis Testing: Significance Versus Importance 7.10. The Limitations of Hypothesis Testing: Significance Versus Importance Given that we are usually interested in rejecting the null hypothesis, we should take a moment to systematically consider the factors that affect our decision in Step 5. Generally speaking, the probability of rejecting the null hypothesis is a function of several independent factors: 1. the size of the observed difference between groups or relationship between variables; 2. the alpha level; 3. the size of the sample. Only the first of these is not under the direct control of the researcher. The relationship between the alpha level and the probability of rejection is straightforward: the higher the alpha level, the larger the critical region, the higher the percentage of all possible sample outcomes that fall in the critical region, and the greater the probability of rejection. Thus, it is easier to reject the H0 at the 0.05 level than at the 0.01 level, and easier still at the 0.10 level. The danger here, of course, is that higher alpha levels will lead to more frequent Type I errors, and we might find ourselves declaring small differences to be statistically significant. 231 The other factor is sample size: with all other factors constant, the probability of rejecting H0 increases with sample size. In other words, the larger the sample, the more likely we are to reject the null hypothesis, and with very large samples (say, samples with thousands of cases), we may declare small differences to be statistically significant. This pattern of higher probabilities for rejecting H0 with larger samples holds for all tests of significance. While larger samples are more accurate and better approximations of the populations they represent, the relationship between sample size and the probability of rejecting the null hypothesis clearly underlines what is perhaps the most significant limitation of hypothesis testing. Simply because a difference is statistically significant does not guarantee that it is important in any other sense. Particularly with very large samples, relatively small differences may be statistically significant. Even with small samples, of course, differences that are otherwise trivial or uninteresting may be statistically significant. In these situations, when it is clear that the research results were not produced by random chance, the researcher must still assess their importance. Do they firmly support a theory or hypothesis? Are they clearly consistent with a prediction or analysis? Do they strongly indicate a line of action in solving some problem? These are the kinds of questions a researcher must ask when assessing the importance of the results of a statistical test. Also, we should note that researchers have access to some very powerful ways of analyzing the importance (versus the statistical significance) of research results. These statistics, including bivariate measures of association and multivariate statistical techniques, will be introduced in later chapters, following the presentation of each test of significance. Furthermore, it can also happen that a finding of no statistical importance may nevertheless be very interesting and theoretically important. An assessment of the importance of the result of a significance test is therefore not limited only to situations of statistical significance. For example, although measures of association are not needed in these circumstances, non-significant results can help us identify weaknesses or limitations in our theoretical models or can show us when programs meant to achieve particular outcomes are not effective and need to be revised. The crucial point is that statistical significance and theoretical or practical importance can be two very different things. In other words, an observed result could therefore be both statistically significant and important; or statistically significant but not important; or not statistically significant and yet important; or neither statistically significant nor important. 232 Applying Statistics 7.1. The Chi Square Test Numerous studies have found that women tend to have poorer health outcomes than men. Is there a gender gap in the health of Indigenous populations in Canada? To examine this question, we use data from the 2017 Aboriginal Peoples Survey, an ongoing national survey of Indigenous people living off reserve, Métis, and Inuit that helps to inform policies and programs aimed at improving the well-being of Indigenous peoples. As a general measure of health, we looked at whether or not a respondent has at least one chronic health condition, defined as an illness that is expected to last or has already lasted six months or more and been diagnosed by a health professional. (To make the number of cases more manageable for computation, yet still representative, we randomly selected about 1% of cases, or 210 respondents, from the full sample of about 21,000 respondents.) The results are as follows: The frequencies we would expect to find if the null hypothesis ( H0 : The variables are independent) were true are as follows: Expected frequencies are found on a cell-by-cell basis by means of the formula Row marginal × Column marginal fe = n The calculation of chi square is organized in a computational table. Step 1 Make Assumptions and Meet Test Requirements Model: Random sampling Level of measurement is nominal or ordinal. Step 2 State the Null Hypothesis H0: The two variables are independent. (H1: The two variables are dependent.) Step 3 Select the Sampling Distribution and Establish the Critical Region Sampling distribution = χ2 distribution Alpha = 0.05 Degrees of freedom = 1 χ2(critical) = 3.841 Step 4 Compute the Test Statistic 2 (fo − fe)2 χ (obtained) = Σ fe = 5.52 Step 5 Make a Decision and Interpret the Results of the Test With an obtained χ2 of 5.52, we reject the null hypothesis of independence. For this sample, there is a statistically significant relationship between gender and health in Indigenous populations in Canada. To complete the analysis, it is useful to know exactly how the two variables are related. We can determine this by computing and analyzing column percentages: 233 The column percentages show that just 41.18% of Indigenous males report a chronic health problem versus 57.41% of females. We have already concluded that the relationship is significant, and now we know the pattern of the relationship. Consistent with other research on gender inequalities in health, Indigenous females tend to have poorer outcomes as measured in this study. Source: Data from Statistics Canada. Aboriginal Peoples Survey, 2017. Reading Statistics 6. Hypothesis Testing Professional researchers use a vocabulary that is much terser than our everyday language when presenting the results of tests of significance. This is partly because space limitations in scientific journals require conciseness and partly because professional researchers can assume a certain level of statistical literacy in their audiences. Thus, they omit many of the elements—such as the null hypothesis or the critical region—that we have been so careful to state. Instead, researchers report only the sample values (e.g., observed frequencies), the value of the test statistic (e.g., chi square), the alpha level, the degrees of freedom (when applicable), and the sample size. The results of a study on region and political party affiliation might be reported as “the relation between these variables was found to be significant, χ2 = 86.14 , p < 0.05.” Note that the alpha level is reported as p < 0.05. This is shorthand for “the probability of a difference of this magnitude occurring by chance alone, if the null hypothesis of independence is true, is less than 0.05” and is a good illustration of how researchers can convey a great deal of information in just a few symbols. In a similar fashion, our somewhat long-winded phrase, “the test statistic falls in the critical region and, therefore, the null hypothesis is rejected,” is rendered tersely and simply: “the relationship … was … found to be significant.” When researchers need to report the results of many tests of significance, they often use a summary table to report the sample information and whether the difference is significant at a certain alpha level. If you read the researcher’s description and analysis of such tables, you should have little difficulty interpreting and understanding them. As a final note, these comments about how significance tests are reported in the literature apply to all of the tests of hypotheses covered in this textbook. Book Title: eTextbook: Statistics: A Tool for Social Research and Data Analysis, Fifth Canadian Edition Chapter 7. Hypothesis Testing with Nominal and Ordinal Variables: Chi Square 7.11. The Chi Square Test: An Example 7.11. The Chi Square Test: An Example To this point, we have confined our attention to 2 × 2 tables, that is, tables with two rows and two columns. For the purposes of illustration, we will work through the computational routines and decision-making process for a larger (3 × 2) table. As you will see, larger tables require more computations (because they have more cells), but in all other essentials they are dealt with in the same way as the 2 × 2 table. 234 Let’s consider the case where a researcher is concerned with the possible effects of working at a paid job on academic progress of university students. Do students who work while studying, with their extra work responsibilities, suffer academically compared to students who do not work while studying? Is academic performance dependent on working while studying? A random sample of 453 students is gathered, and each student is classified as either working while studying (Yes) or not working while studying (No) and—using grade-point average (GPA) as a measure—as a good, average, or poor student. Results are presented in Table 7.9. Table 7.9 Grade-Point Average (GPA) by Working While Studying for 453 University Students For the upper left cell (students who work while studying with good GPAs) the expected frequency is (160 × 175)/453 , or 61.81. For the other cell in this row, the expected frequency is (160 × 278)/453 , or 98.19. In similar fashion, all expected frequencies are computed (being very careful to use the correct row and column marginals) and displayed in Table 7.10. Table 7.10 Expected Frequencies for Table 7.9 The next step is to solve the formula for χ2 (obtained), being very careful to be certain that we are using the proper fo’s and fe’s for each cell. Once again, we will use a computational table (Table 7.11) to organize the calculations and then test the obtained chi square for its statistical significance. Remember that obtained chi square is equal to the total of column 5. Table 7.11 Computational Table for Table 7.9 235 We can now test the value of the obtained chi square (2.78) for its significance. Step 1 Make Assumptions and Meet Test Requirements Model : Random sampling Level of measurement is nominal or ordinal. Step 2 State the Null Hypothesis H0: The two variables are independent. (H1: The two variables are dependent.) Step 3 Select the Sampling Distribution and Establish the Critical Region. Sampling distribution = χ2 distribution Alpha = 0.05 Degrees of freedom = (r − 1)(c − 1) = (3 − 1)(2 − 1) = 2 χ2(critical) = 5.991 Step 4 Compute the Test Statistic 2 (fo − fe)2 χ (obtained) = Σ fe = 2.78 Step 5 Make a Decision and Interpret the Results of the Test The test statistic, χ2(obtained) = 2.78 , does not fall in the critical region, which, for alpha = 0.05 , df = 2 , begins at the (χ2) (critical) of 5.991. Therefore, we fail to reject the null hypothesis. The observed frequencies are not significantly different from the frequencies we would expect to find if the variables were independent and only random chance were operating. Based on these sample results, we can conclude that the academic performance of university students is not dependent on their work status. Since we failed to reject the null hypothesis, we will not examine column percentages as we did for Table 7.5. 236 Applying Statistics 7.2. The Chi Square Test in a Larger (2 × 4) Table There has been a good deal of public debate over privatization of the Canadian health care system. Do attitudes toward privatization of health care vary by region of residence? Is attitude on privatization dependent on region? We will examine this issue with data from the 2019 Canadian Election Study. To make the number of cases in this example more manageable, yet still representative, we randomly selected 500 cases from the full sample of over 40,000 cases. Respondents were classified by their region of residence—Atlantic Canada, Québec, Ontario, and Western Canada—and, as a measure of privatization, by whether they agree or disagree that “people who are willing to pay should be allowed to get medical treatment sooner.” The 2 × 4 table below shows these results: The frequencies we would expect to find if the null hypothesis ( H0 : The variables are independent) were true are Expected frequencies are found on a cell-by-cell basis by the formula Row marginal × Column marginal n The calculation of chi square is organized in a computational table: Step 1 Make Assumptions and Meet Test Requirements Model: Random sampling Level of measurement is nominal or ordinal. Step 2 State the Null Hypothesis H0: The two variables are independent. (H1: The two variables are dependent.) Step 3 Select the Sampling Distribution and Establish the Critical Region Sampling distribution = χ2 distribution Alpha = 0.05 Degrees of freedom = (r − 1)(c − 1) = (2 − 1)(4 − 1) = 3 χ2(critical) = 7.815 Step 4 Compute the Test Statistic 2 (fo − fe)2 χ (obtained) = Σ fe = 14.56 Step 5 Make a Decision and Interpret the Results of the Test With an obtained χ2 of 14.56, we would reject the null hypothesis of independence. For this sample, there is a statistically significant relationship between region of residence and attitude toward health care privatization. To complete the analysis, it would be useful to know exactly how the two variables are related. We can determine this by computing and analyzing column percentages: The column percentages show that just over 30% of people from the Atlantic provinces and Ontario agree with some private health care in Canada. By comparison, about 51% and 46% of people from Western Canada and Québec, respectively, agree with privatization. We have already concluded that the relationship is significant, and now we know the pattern of the relationship. 237 Source: Data from Canadian Election Study. 2019 Canadian Election Study. Book Title: eTextbook: Statistics: A Tool for Social Research and Data Analysis, Fifth Canadian Edition Chapter 7. Hypothesis Testing with Nominal and Ordinal Variables: Chi Square 7.12. The Limitations of the Chi Square Test 7.12. The Limitations of the Chi Square Test Like any other test, chi square has limits, and you should be aware of several potential difficulties. First, even though chi square is very flexible and handles many different types of variables, it becomes difficult to interpret when the variables have many categories. For example, two variables with five categories each generate a 5 × 5 table with 25 cells—far too many combinations of scores to be easily absorbed or understood. As a very general guideline, the chi square test is easiest to interpret and understand when both variables have four or fewer scores. Two further limitations of the test are related to sample size. When the sample size is small, it can no longer be assumed that the sampling distribution of all possible sample outcomes is accurately described by the chi square distribution. For chi square, a small sample is defined as one where a high percentage of the cells have expected frequencies (f e ) of five or less. Various guidelines have been developed to help the researcher decide what constitutes a “high percentage of cells.” Probably the safest course is to take corrective action whenever any of the cells have expected frequencies of five or less. In the case of 2 × 2 tables, the value of χ 2 (obtained) can be adjusted by applying Yates’s correction for continuity, the formula for which is Formula 7.4 (|f o − f e | − 0.50) 2 χ 2c =Σ fe where χ 2c = corrected chi square |f o − f e | = the absolute value of the difference between the observed and expected frequency for each cell The correction factor is applied by reducing the absolute value of the term f o − f e by 0.50 before squaring the difference and dividing by the expected frequency for the cell. For tables larger than 2 × 2 , there is no correction formula for computing χ 2 (obtained) for small samples. It may be possible to combine some of the categories of the variables and thereby increase cell sizes. Obviously, however, this course of action should be taken only when it is sensible to do so. In other words, distinctions that have clear theoretical justifications should not be erased merely to conform to the requirements of a statistical test. When you feel that categories cannot be combined to build up cell frequencies, and the percentage of cells with expected frequencies of five or less is small, it is probably justifiable to continue with the uncorrected chi square test as long as the results are regarded with a suitable amount of caution. A second potential problem related to sample size occurs with large samples. As we noted earlier in the chapter, all tests of hypothesis are sensitive to sample size. That is, the probability of rejecting the null hypothesis increases as the number of cases increases, regardless of any other factor. It turns out that chi square is especially sensitive to sample size and that larger samples may lead to the decision to reject the null hypothesis when the actual relationship is trivial. In fact, chi square is more responsive to changes in sample size than other test statistics, because the value of χ 2 (obtained) increases at the same rate as sample size. That is, if sample size is doubled, the value of χ2 (obtained) is doubled. (For an illustration of this principle, see Problem 7.14.) Our major purpose in stressing the relationship between sample size and the value of chi square is really to point out, once again, the distinction between statistical significance and theoretical importance. On the one hand, tests of significance play a crucial role in research. As long as we are working with random samples, we must know whether our research results could have been produced by mere random chance. On the other hand, like any other statistical technique, tests of hypothesis are limited in the range of questions they can answer. Specifically, these tests tell us whether our results are statistically significant or not. They do not necessarily tell us if the results are important in any other sense. To deal more directly with questions of importance, we must use an additional set of statistical techniques called measures of association. We previewed these techniques in this chapter when we used column percentages. In Chapters 8 and 9, we will take a more in depth look at measures of association for nominal and ordinal variables, respectively. Book Title: eTextbook: Statistics: A Tool for Social Research and Data Analysis, Fifth Canadian Edition Chapter 7. Hypothesis Testing with Nominal and Ordinal Variables: Chi Square Summary Summary 1. All the basic concepts and techniques for testing hypotheses were presented in this chapter. We saw how to test the null hypothesis of the independence of variables. The central question is whether variables are independent in the population represented by the sample. 2. All tests of hypotheses involve finding the probability of the observed sample outcome, given that the null hypothesis is true. If the outcome has a low probability, we reject the null hypothesis. In the usual research situation, we wish to reject the null hypothesis and thereby support the research hypothesis. 3. The five-step model will be our framework for decision making throughout the chapters on hypothesis testing. What we do during each step, however, will vary, depending on the specific test being conducted. 4. There are two kinds of errors in hypothesis testing. A Type I, or alpha, error is rejecting a true null hypothesis; a Type II, or beta, error is failing to reject a false null hypothesis. The probabilities of committing these two types of error are inversely related and cannot be simultaneously minimized in the same test. By selecting an alpha level, we try to balance the probability of these two kinds of errors. 5. The chi square test for independence is appropriate for situations in which the variables of interest are organized in table format. The null hypothesis is that the variables are independent or that the classification of a case into a particular category on one variable has no effect on the probability that the case will be classified into any particular category of the second variable. 6. Because chi square is non-parametric and requires only categorical (i.e., nominal or ordinal) variables, its model assumptions are easily satisfied. Furthermore, because it is computed from bivariate tables, in which the number of rows and columns can be easily expanded, the chi square test can be used in many situations in which other tests are inapplicable. 7. In the chi square test, we first find the frequencies that would appear in the cells if the variables were independent (f e ) and then compare those frequencies, cell by cell, with the frequencies actually observed in the cells (f o ). If the null hypothesis is true, expected and observed frequencies should be quite close in value. The greater the difference between the observed and expected frequencies, the greater the possibility of rejecting the null hypothesis. 8. The chi square test has several important limitations. It is often difficult to interpret for tables that have many (more than four or five) dimensions. Also, as the sample size (n) decreases, the chi square test becomes less trustworthy, and corrective action may be required. Finally, with very large samples, we might declare relatively trivial relationships to be statistically significant. As is the case with all tests of hypothesis, statistical significance is not the same thing as “importance” in any other sense. 9. If you are still confused about the uses of inferential statistics described in this chapter, don’t be alarmed or discouraged. A sizable volume of rather complex material has been presented, and only rarely will a beginning student fully comprehend the unique logic of hypothesis testing on the first exposure. After all, it is not every day that you learn how to test a statement you don’t believe (the null hypothesis) against a distribution that doesn’t exist (the sampling distribution)! 10. In all tests of hypothesis, a number of factors affect the probability of rejecting the null hypothesis: the size of the difference, the alpha level, and the sample size. Statistical significance is not the same thing as theoretical or practical importance. Even after a difference is found to be statistically significant, researchers must still demonstrate the relevance or importance of their findings. The statistics presented in later chapters of this textbook will give us some of the tools we need to deal directly with issues beyond statistical significance. Summary of Formulas Chi square 2 (f o − f e ) 2 χ (obtained) = Σ (obtained) fe Expected fe = Row marginal × Column marginal frequencies n Degrees of freedom, df = (r − 1)(c − 1) bivariate tables Yates’s correction (|f o − f e | − 0.50) 2 χ 2c =Σ for continuity fe Glossary Alpha level ( α ) Bivariate tables Cells χ 2 (critical) χ 2 (obtained) Chi square test Column Column percentages Critical region Critical value Expected frequencies (f e ) Five-step model Hypothesis testing Independence Marginals Non-parametric Null hypothesis (H 0 ) Observed frequencies (f o ) p value Research hypothesis (H 1 ) Rows Test statistic Type I error Type II error Multimedia Resources Visit the companion website for the fifth Canadian edition of Statistics: A Tool for Social Research and Data Analysis to access a wide range of student resources: www.cengage.com/healey5ce. Problems 7.1 For each table below, calculate the obtained chi square. (HINT: Calculate the expected frequency for each cell with Formula 7.2. Double- check to make sure you are using the correct row and column marginals for each cell. It may be helpful to record the expected frequencies in table format as well—see Tables 7.4, 7.6, and 7.10. Next, use a computational table to organize the calculation for Formula 7.1—see Tables 7.7 and 7.11. For each cell, subtract expected frequency from observed frequency and record the result in column 3. Square the value in column 3 and record the result in column 4, and then divide the value in column 4 by the expected frequency for that cell and record the result in column 5. Remember that the sum of column 5 in the computational table is the obtained chi square. As you proceed, check to make sure you are using the correct value for each cell.) a. 20 25 45 25 20 45 45 45 90 SHOW ANSWER b. 10 15 25 20 30 50 30 45 75 SHOW ANSWER c. 25 15 40 30 30 60 55 45 100 SHOW ANSWER d. 20 45 65 15 20 35 35 65 100 SHOW ANSWER 7.2 What is the critical value for a chi square test with five degrees of freedom at the 0.01 level? 7.3 If a researcher found a chi square obtained of 6.75 in a test with three degrees of freedom, would they be able to reject the null hypothesis at the 0.01 level? If not, at what level could they reject the null hypothesis? What is the critical value for a chi square test with five degrees of freedom at the 0.01 level? SHOW ANSWER 7.4 Is there an age gap in support of the Liberal Party of Canada among university faculty? To answer this question, a sample of university faculty has been asked about their political party preference. a. Is there a statistically significant relationship between age and party preference? b. Compute column percentages for the table to determine the pattern of the relationship. Which age range is more likely to prefer the Liberals? 7.5 PA Is there a relationship between salary levels and unionization for public employees? The data below represent this relationship for fire departments in a random sample of 100 cities of roughly the same size. Salary data have been dichotomized (split into two groups) at the median. Summarize your findings. a. Is there a statistically significant relationship between these variables? b. Compute column percentages for the table to determine the pattern of the relationship. Which group is more likely to get high salaries? SHOW ANSWER 7.6 SOCsidents, are reported below. a. Is there a statistically significant relationship between participation and alertness? b. Compute column percentages for the table to determine the pattern of the relationship. Which group is more likely to be alert? 7.7 SOC The provincial Ministry of Education has rated a sample of local school boards for compliance with province-mandated guidelines for quality. Is the quality of a school board significantly related to the affluence of the community as measured by per capita income? a. Is there a statistically significant relationship between these variables? b. Compute column percentages for the table to determine the pattern of the relationship. Which are more likely to have high-quality schools, high- or low-income communities? SHOW ANSWER 7.8 CJ A local judge has been allowing some individuals convicted of impaired driving to work in a hospital emergency room as an alternative to fines, suspensions, and other penalties. A random sample of offenders has been drawn. Do participants in this program have lower rates of recidivism for this offence? a. Is there a statistically significant relationship between these variables? b. Compute column percentages for the table to determine the pattern of the relationship. Which group is more likely to be arrested again for driving under the influence? 7.9 SOC Is there a relationship between length of marriage and satisfaction with marriage? The necessary information has been collected from a random sample of 100 respondents drawn from a local community. a. Is there a statistically significant relationship between these variables? Write a sentence or two explaining your decision. b. Compute column percentages for the table to determine the pattern of the relationship. Which group is more likely to be highly satisfied? SHOW ANSWER 7.10 PS Is there a relationship between political ideology and class standing? Are upper-class individuals significantly different from lower- class on this variable? The table below reports the relationship between these two variables for a random sample of 267 adult Canadians. a. Is there a statistically significant relationship between these variables? b. Compute column percentages for the table to determine the pattern of the relationship. Which group is more likely to be conservative? 7.11 SOC At a large urban university, about half of the students live off campus in various arrangements, and the other half live in residence on campus. Is academic performance dependent on living arrangements? The results, based on a random sample of 300 students, are presented below. a. Is there a statistically significant relationship between these variables? b. Compute column percentages for the table to determine the pattern of the relationship. Which group is more likely to have a high GPA? SHOW ANSWER 7.12 SOC An urban sociologist has built up a database describing a sample of the neighbourhoods in a city and has developed a scale by which each area can be rated for “quality of life” (this includes measures of pollution, noise, open space, services available, etc.). The sociologist also asked samples of residents of these areas about their level of satisfaction with their neighbourhoods. a. Is there significant agreement between the sociologist’s objective ratings of quality of life and the respondents’ self-reports of satisfaction? b. Compute column percentages for the table to determine the pattern of the relationship. Which group is most likely to say that their satisfaction is high? 7.13 SOC Does support for the legalization of all illicit drugs vary by region of Canada? The table displays the relationship between the two variables for a random sample of 1,020 adult Canadians. a. Is there a statistically significant relationship between these variables? b. Compute column percentages for the table to determine the pattern of the relationship. Which region is most likely to favour legalization of all illicit drugs? SHOW ANSWER 7.14 SOC A researcher is concerned with the relationship between attitudes toward violence and violent behaviour. If attitudes “cause” behaviour (a very debatable proposition), then people who have positive attitudes toward violence should have high rates of violent behaviour. A pretest was conducted on 70 respondents. Among other things, the respondents were asked, “Have you been involved in a violent incident of any kind over the past six months?” The researcher established the following relationship: 238 The chi square calculated on these data is 0.23, which is not significant at the 0.05 level (confirm this conclusion with your own calculations). Undeterred by this result, the researcher proceeded with the project and gathered a random sample of 7,000. In terms of percentage distributions, the results for the full sample were exactly the same as for the pretest: However, the chi square obtained is a very healthy 23.40 (confirm with your own calculations). Why is the full-sample chi square significant when the pretest was not? What happened? Do you think the second result is important? 7.15 SOC Some results from a survey given to a random sample of Canadians are presented below. For each table, conduct the chi square test of significance and compute column percentages. Write a sentence or two of interpretation for each test. a. Support for same-sex marriage by age: b. Support for gun control by age: c. Fear of walking alone at night by age: d. Support for legalizing all illicit drugs by age: e. Support for medical assistance in dying when a person has an incurable disease by age: SHOW ANSWER You are the Researcher Using SPSS to Conduct the Chi Square Test with the 2018 GSS The demonstrations and exercises below use the shortened version of the 2018 GSS data set supplied with this textbook. SPSS Demonstration 7.1 Does Volunteering Behaviour Vary by Gender? The Crosstabs procedure in SPSS produces bivariate tables and a wide variety of statistics. This procedure is commonly used in social science research at all levels, and you will see references to Crosstabs in chapters to come. We 239 introduce the command here, and we will return to it in later sessions. In this demonstration, we ask two questions: “Is gender significantly related to volunteering behaviour?” And, if so, “Are females more likely to be volunteers?” We can answer these questions, at least for the 2018 GSS sample, by constructing a bivariate table to display the relationship between fvisvolc (volunteering indicator) and gndr (gender). We will also request a chi square test for the table. Start SPSS, and load the 2018 GSS database (GSS_2018_Shortened.sav). From the main menu bar, click Analyze, Descriptive Statistics, and then Crosstabs. The Crosstabs dialog box will appear with the variables listed in a box on the left. Highlight fvisvolc, click the arrow to move the variable name into the Row(s) box, and then highlight gndr and move it into the Column(s) box. Click the Statistics button at the top of the window, and then click the box next to Chi- square. Click Continue. Then, click the Cells button and check the boxes next to “Expected” in the Counts box and “Column” in the Percentages box. This will generate the expected frequencies and column percentages, respectively, for the table. Click Continue and OK, and the output below will be produced. (Note that the output has been slightly edited for clarity and will not exactly match the output on your screen.) The cross-tabulation table is small ( 2 × 2 ), and the information is straightforward. Let’s begin with the cells. Each cell displays the observed frequency or the number of cases in the cell (“Count”) and the column percentage for that cell (“% within Gender”). For example, 270 males volunteered, or 34.5% of all males. By contrast, 348 females volunteered, or 240 43.9%. Females are generally more likely than males to have volunteered. The table also displays the expected frequency for each cell. The greater the differences between expected frequencies (“Expected Count”) and observed frequencies (“Count”), the less likely the variables are independent and the more likely we will be able to reject the null hypothesis. The results of the chi square test are formally reported in the output block that follows the cross- tabulation table. The value of χ 2 (obtained) is found in the row “Pearson Chi-Square.” The value of the obtained chi square is 14.769, the degrees of freedom is 1, and the exact significance of the chi square is 0.000. The value 0.000 is the p value or the exact probability of getting the pattern observed in the cell frequencies if only chance is operating. It is important to note that we do not interpret 0.000 as a zero probability—to save space, SPSS cuts off the exact probability at the first three decimal places or 0.000. The exact value can be revealed by double- clicking on the value “.000” in the “Asymptotic Significance (2-sided)” column of the Chi-Square Tests table. This will open the Pivot Table Editor window. Double-click the “.000” value one more time to show the exact probability, which is 0.000122. (Make sure to close the Pivot Table Editor window to return to the Output window.) To test whether there is a significant relationship between the variables, we can manually compare the value of χ 2 (obtained) to the value of χ 2 (critical), determined by consulting the chi square table in Appendix C for a particular alpha level and degrees of freedom, as we have practised throughout this chapter. The test statistic, χ 2 (obtained), of 14.769 falls in the critical region (i.e., 14.769 is greater than 3.841). So, we reject the null hypothesis. However, because SPSS also provides the exact probability, there is no need to look up the test statistic in the chi square table. The p value, 0.000 (or more precisely 0.000122), is well below the standard indicator of a significant result (alpha = 0.05), so we reject the null hypothesis that the variables are independent and conclude that there is a statistically significant relationship between gender and volunteering behaviour. Being a volunteer is dependent on gender, with females being more likely to volunteer. In Exercise 7.1, you will have the opportunity to investigate other variables that might be significantly related to volunteering behaviour. As a final note, the number of cells with expected frequencies less than five is provided at the bottom of the “Chi-Square Tests” output box. Yates’s correction for continuity, labelled “Continuity Correction,” should be used in any 2×2 table where one or more cells have expected counts of less than five. When this is the case for tables larger than 2 × 2 , consider collapsing categories of the variables to increase cell sizes. SPSS Demonstration 7.2 Does Religious Participation Vary by Canadian Birth Status? In this demonstration, we will examine the relationship between Canadian birth status and the frequency of religious participation. Specifically, “Is religious participation higher for people born in Canada or people not born in Canada?” Run the Crosstabs procedure again with ree_02 (frequency of religious participation in the past 12 months) as the row variable and brthcan as the column variable. Don’t forget to request chi square, expected frequencies, and column percentages. The output, slightly edited for clarity, is shown below. 241 242 243 244 245 246 247 Chi square is 25.911, degrees of freedom is 4, and the exact probability of getting this pattern of cell frequencies by random chance alone is 0.000. Note that when the exact probability value is less than 0.0005, SPSS cuts it off at three decimal places, or 0.000, to save space. Therefore, there is a significant relationship between the variables. The column percentages show that religious participation increases from people born in Canada to people not born in Canada. Focusing on the top row, for example, we see that 15.7% of people not born in Canada participated in religious services at least once a week in the past 12 months, while 11.7% of people born in Canada did so. Looking at the bottom row, we see that 58.2% of people born in Canada did not participate in religious services at all in the past 12 months, while 44.5% of people not born in Canada followed this pattern. Exercise 7.2 provides an 248 opportunity to look at other variables that might be significantly related to ree_02. Exercises (using GSS_2018_Shortened.sav) 7.1 For a follow-up to Demonstration 7.1, find two more variables that might be related to fvisvolc. Run the Crosstabs procedure with fvisvolc as the row variable and your other variables as the column variables. (If necessary, use the recode procedure shown in Appendix F.5 to reduce the number of categories in the independent (column) variables.) Write a paragraph summarizing the results of these two tests. Which relationships are significant at the 0.05 alpha level? 7.2 As a follow-up to Demonstration 7.2, find two more variables that might be related to ree_02. Use the Crosstabs procedure to see if any of your variables have a significant relationship with ree_02. (If necessary, use the recode procedure shown in Appendix F.5 to reduce the number of categories in the independent (column) variables.) Which of the variables had the most significant relationship? 249 Book Title: eTextbook: Statistics: A Tool for Social Research and Data Analysis, Fifth Canadian Edition Chapter 7. Hypothesis Testing with Nominal and Ordinal Variables: Chi Square Summary of Formulas Summary of Formulas Chi square 2 (f o − f e ) 2 χ (obtained) = Σ (obtained) fe Expected fe = Row marginal × Column marginal frequencies n Degrees of freedom, df = (r − 1)(c − 1) bivariate tables Yates’s correction (|f o − f e | − 0.50) 2 χ 2c =Σ for continuity fe