Phylogenetics: Building phylogenetic trees PDF
Document Details
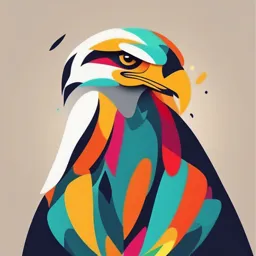
Uploaded by RelaxedPennywhistle7814
Western Connecticut State University
Tags
Summary
This presentation covers the basics of phylogenetics, including how to build phylogenetic trees using various data types. It explores concepts like homology, molecular data analysis, and different methods for phylogenetic inference, such as parsimony, maximum likelihood, and Bayesian inference. Models of substitution and sequence alignments are also discussed.
Full Transcript
Phylogenetics: Building phylogenetic trees Class goals Know the inputs and outputs for a phylogenetic reconstruction Learn to build a phylogeny This Photo by Unknown Author is licensed under CC BY-SA-NC How do we build a phylogeny? To build a phylogeny, we ne...
Phylogenetics: Building phylogenetic trees Class goals Know the inputs and outputs for a phylogenetic reconstruction Learn to build a phylogeny This Photo by Unknown Author is licensed under CC BY-SA-NC How do we build a phylogeny? To build a phylogeny, we need to collect data about the terminals (taxa) we are studying. This data can include: Morphological traits DNA/RNA/Protein sequence Behavioral traits How do we recognize which traits (molecular, morphological, behavioral) we use? We recognize what characters to use through the concept of Homology Owen (1843): “Homologue” as “The same organ in different animals under every variety of form and function” Comparisons: Do we really compare everything? “Nothing in biology makes sense except in the light of evolution” Dobzhansky Pinterest How do we recognize homology? Owen (1849) Taxic homology “Homologue characters are located on the same body position” Santibáñez-López, Sharma (in prep.) Molecular homology Sp1 ATGCTTATTCGATATAAACTG Sp2 TGCTTTTGATATAAACTG Sp3 AGCTATTCATATAACTG Sp1 ATGCTTATTCGATATAAACTG Sp2 TTGTTTTGATATAAACTG Sp3 ATCTATTCATATAACTG Sp1 ATGCTTATTCGATATAAACTG Sp2 - TTGTT- TT- GATATAAACTG Sp3 A- GCT- ATT- CATATAA- CTG Sp1 ATGCTTATTCGATATAAACTG Sp2 TTG- TT- TT- GATATAAACTG Sp3 A- GCT- ATT- CATAT- AACTG Molecular Data DNA ATGCTTATACG RNA AUGCUUAUACG Proteins MASKRLPDH Phylogeny Evolutionary history of organisms Represented as a diagram, network (tree) Molecular Biology and Evolution 35 (cover) OTUs (Operational Taxonomical Units) = Terminals HTUs (Hypothetical Taxonomical Units) = Nodes, speciation events Wheeler (2012) Fully resolved vs Unresolved tree Page & Holmes (2004) Page & Holmes (2004) Parenthetical tree mode ((A,B),C) Page & Holmes (2004) Phylogenetic analysis Maximum Parsimony Maximum likelihood Model based Bayesian inference Parsimony WHAT IS A CLADOGRAM? “Simplest explanation” “The best hypothesis that requires fewest evolutionary changes” Produces a cladogram ML: Principle You want to know if your data supports a hypothesis of how this data was produced Example: (from Baum & Smith, 2013) You have a bag with coins and half of them are fair (50% chance of head/tails), and the other half is biased (75% chance of head). You draw one coin and you want to know if the coin you have is fair or not. The coin is tossed 10 times and each time falls heads ML in phylogenetics COIN EXAMPLE PHYLOGENETICS DATA Bag with coins Matrix Hypothesis ”I picked up a fair coin” Tree Model of evolution, Probability 0.5% for a fair coin to get a head branch lengths, rates, frequency The tree obtained is the one that has the Maximum Likelihood given your data Bayesian inference vs ML ML -> searches for the tree based on how probable evolution produce it BI -> searches for the tree based on their posterior probability that the tree is true given the data, model of evolution, and prior information. Bag full of coins With ML we didn’t consider the information about the probability that the coin was biased or not = prior probability likelihood Prior probability of our hypothesis Pr ( D | H ) x Pr ( H ) Pr ( H | D ) = Pr ( D ) Posterior probability Prior probability of data hypothesis given our data Bayesian phylogenetics Pr ( Data | Tree ) x Pr ( Tree ) Pr ( Tree | Data ) = Pr ( Data ) Pr ( Data | Tree ) = Likelihood (calculated as before, but integrating the prior probability of all parameters) Pr ( Tree ) = The probability that our tree is the correct among all trees Pr ( Data ) = Summation of over all trees Felsenstein (2004) Phylogenetic inference with molecular data Two types of homology: 𝝰 Orthology Paralogy Paralogs Homologs β Orthologs Globulin proteins Molecular evolution Sequence evolution = changes in the nucleotides / amino acids at a particular position in that sequence Substitution = one base change into a different base at the same position. Insertion = one base appeared in the sequence without locally homologous position Deletion = one base disappeared in the sequence Sequence alignment Pairwise alignment Sequence 1 A T G C T T A T C G A Sequence 2 A T C C T A A T C C T Multiple sequence Sequence 1 ATGCTT-ATCGA alignment Sequence 2 ATCCTA -ATCCT Sequence 3 ATGGTTAATGGA Sequence 4 ATGCTT -- TCGA Multiple sequence alignment (MSA) Progressive method Iterative method Katoh et al. 2009 Distance between two sequences Expected number of nucleotide substitutions per site Transitions A G Transversions C T Transitions Most common models of substitution JC69 (Jukes and Cantor 1969) = Assumes every nucleotide has the same rate of changing into another nucleotide. K80 (Kimura 1980) = Accounts different rates for transitions and transversions. A G A G C T C T Most common models of substitution HKY85 (Hasegawa et al. 1985) = unequal base compositions and asymmetrical substitution rates GTR (General reversible model) = each possible substitutions has its own probability A G A G C T C T Most common models of substitution Dayhoff (Dayhoff et al. 1978) = using a parsimony argument to reconstruct ancestral sequences. JTT (Jones et al. 1992) WAG (Whelan and Goldman 2001) Summary Phylogenies are built using different type of data (morphological, molecular, behavioral) We compare only ”homologues” traits (taxic homology, molecular homology) Within molecular data, we recognize two types of homology: Orthology – Paralogy We use Parsimony, or model-based methods (Maximum Likelihood or Bayesian Inference) to construct phylogenies Summary Parsimony yields cladograms only ML searches for the tree based on how probable evolution produced it BI searches for the tree based on their posterior probability that this tree is true given the data, model, and prior information Molecular data must be aligned (using Multiple Sequence Alignments) Models of substitution accounts for the probability that one nucleotide will change for another