Hypothesize PDF
Document Details
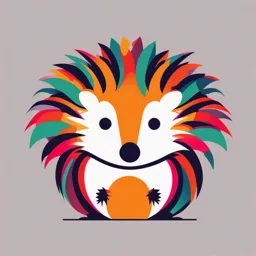
Uploaded by FearlessLoyalty
Tags
Related
- Field Experiments - Digital and Social Media Strategies - PDF
- Hypothesis Testing - CS2MATH211 - University of Science and Technology of Southern Philippines PDF
- CS2MATH211 Hypothesis Testing PDF
- Data Analysis for Marketing Decisions - Lecture Notes PDF
- Case 2.1: A/B Testing PDF
- Applied Econometrics (5AEC) Specimen Test PDF - The London Institute of Banking & Finance
Summary
This document provides an introduction to hypothesis testing, focusing on different approaches like cross-channel reach reporting, attribution modeling, marketing mix modeling, and randomized controlled trials (RCT). It also features examples of hypothesis formulation and testing within business contexts.
Full Transcript
Hypothesize Introduction To run an effective experiment, you should first choose a hypothesis, or question, that you want your experiment to answer. A strong, well-structured hypothesis can be confirmed or rejected through testing and can lead to valuable insights. Formulate a hypothesis. What is...
Hypothesize Introduction To run an effective experiment, you should first choose a hypothesis, or question, that you want your experiment to answer. A strong, well-structured hypothesis can be confirmed or rejected through testing and can lead to valuable insights. Formulate a hypothesis. What is a hypothesis? A hypothesis is an idea or explanation that you can test through study and experimentation. A strong hypothesis includes: ● ● ● ● ● Who: audience What: behavior of that audience Where: location When: ad schedule Why: the rationale for the anticipated audience behavior or perspective In order to develop a hypothesis, you need to first determine a variable and then apply research and industry context. Examples of variables ● ● ● Audience Placement Creative: format, message, quantity of ads Delivery optimization HYPOTHESIZE ● ● ● ● Campaign objective Account structure Budget Frequency 12 Example hypothesis 1 An ecommerce clothing company’s goal is to increase online purchases. In May, they launch their summer line and hypothesize that lifestyle-focused creative will achieve more online purchases than value-focused creative among the 18-34 age group. ● ● ● ● Who: people ages 18-34 What: purchase Where: online When: May ● ● ● ● Who: women over the age of 25 What: brand awareness Where: Facebook and Instagram When: June through August Example hypothesis 2 A CPG/FMCG advertiser’s goal is to increase brand awareness for a new hair-care product among a broad global audience of women over the age of 25 between June and August. The advertiser seeks to test the hypothesis that a 10-second video format on Facebook and Instagram would increase brand awareness more effectively than their current 30-second videos. Formulate a test hypothesis. What is a test hypothesis? A test hypothesis is a proposed explanation for an observation you can test, usually in the form of an if/then statement. While a hypothesis could be a subjective assumption, a test hypothesis includes a null and an alternative hypothesis. The objective is to test if the null hypothesis can be rejected and the alternative can be accepted. How to create a test hypothesis ● ● ● ● Identify the independent variables and dependent variables. Ensure variables are specific, measurable and aligned with your business goal. You can test multiple variables at once, but advertisers should test one variable at a time if the goal is to understand and isolate the effect of the strategy being tested. Identify a null and an alternative hypothesis to understand exactly what is being tested. HYPOTHESIZE 13 Example test hypothesis If an advertiser increases the number of ad placements from 1 to 4 or more, their average cost per acquisition (CPA) will decrease. ● ● Independent variable = the number of ad placements Dependent variable = CPA Null hypothesis: ● CPA (1 placement) = CPA (4 placement) Alternative hypothesis: ● CPA (1 placement) < CPA (4 placement) Determine a measurement approach. Once you have a hypothesis, prove or disprove it by gathering existing insights or research or conducting a test. Choose from the following measurement approaches: ● ● ● ● ● Cross-channel reach reporting Attribution Marketing mix modeling Randomized control trial (RCT) A/B test HYPOTHESIZE 14 Cross-channel reach reporting Measures how channels work together to generate business outcomes. Channels include but are not limited to email, TV, direct mail, Facebook and paid search. Limitations ● ● ● ● Metrics vary by channel. Cross-channel reach reporting can be challenging because not all channels share touchpoint/reach data. Limitations vary by specific solution. In general, limitations arise when metrics are not comparable or like-for-like data is not available. Reach does not always correlate with brand and conversion business outcomes. Example of cross-channel reach reporting Context A CPG advertiser runs ads on multiple platforms, including TV and digital channels, with the goal of reaching a broad audience at an efficient cost per person. Hypothesis The advertiser seeks to test the hypothesis that investment in TV delivers more efficient reach relative to their digital channels. Measurement approach The advertiser uses cross-channel reach reporting to test this hypothesis. HYPOTHESIZE 15 Attribution The process of determining credit for each touchpoint on a consumer’s path to conversion. Multi-touch attribution models, or MTAs, allocate value to more than one touchpoint in a consumer’s path to conversion. Data-driven attribution is the process of determining credit for touchpoints on a conversion path often based on historical data and statistical modeling Limitations ● ● ● ● Statistical attribution models can be costly to access in some cases, while others are limited to specific channels. Cross-device limitations on cookie- or web-based systems. Many tools don’t have access to the full path to conversion. For example, in-app impression data is often not shared across publishers. Often only includes digital channels. Example of Attribution Context An advertiser running prospecting and retargeting campaigns on Facebook and Instagram sought to increase return on ad spend (ROAS) from their Facebook and Instagram advertising during their highest sales period. They do not want to have a holdout on their media during this time. Hypothesis They seek to test the hypothesis that their prospecting campaigns deliver less incremental value than their retargeting campaigns but cannot run an experiment at this time. Measurement approach They use our data-driven attribution model to assess the estimated incremental value of these campaigns in the interim. HYPOTHESIZE 16 Marketing mix modeling A data-driven statistical analysis that quantifies the incremental sales impact and return on investment of marketing and nonmarketing activities, measuring both offline and online sales across channels. Limitations ● ● ● ● ● Can struggle to capture incremental sales where an increase is minimal. Requires collaboration between modelers and an econometric model. Doesn’t help with in-channel optimization. Does not infer causality, only correlation. Can be time-intensive to implement. Example of Marketing mix modeling Context An agency wants to optimize spend across channels for a soda brand. The soda brand client delivers media on TV, email, direct mail, Facebook and other digital channels. Hypothesis The agency hypothesizes that TV achieves a higher ROI than direct mail, and therefore more budget should be allocated to TV next year. Measurement approach Instead of looking at the impact of each channel in a silo, a marketing mix model helps to understand the effect of each channel on sales outcomes in the previous year. HYPOTHESIZE 17 Randomized control trial (RCT) An experiment designed to measure causality. It includes randomization of participants into mutually exclusive groups. One or more of these groups receive the treatment called the test group, while one or more do not receive the treatment, called the control groups. The control provides a standard of comparison and can represent a standard practice, a placebo or no treatment. Limitations ● ● ● ● ● ● ● Tests may not be set up with sufficient statistical power. For example, budget is too low, test duration is too short or not enough conversions. Accurate power calculations can be challenging to carry out where there is a lack of historical data from similar activity on which to base the calculation. Control groups may become contaminated by media running outside the experiment that targets the audience being measured. Treatment variable may not always be isolated. Tests may not run long enough to capture the full purchase cycle or longer-term impact of the strategy being measured. Experiments may not always capture the full impact of a strategy (for example, offline data may not be available to upload). Not all experimental approaches manage outliers and variance equally. Example of Randomized control trial (RCT) Context An advertiser running ads on multiple channels, including Facebook, wants to measure the sales caused by their Facebook advertising in order to assess the accuracy of their current attribution model. Hypothesis The advertiser hypothesizes that their Facebook advertising achieves $500,000 in incremental sales and a $50 cost per incremental purchase in the month of June. Measurement approach They run a randomized control trial experiment to measure the causal impact of their Facebook advertising. HYPOTHESIZE 18 A/B test Testing multiple campaigns against one another to see which tactical approach produces the best results based on your KPIs. Limitations ● ● Some A/B tests don’t include control groups, only randomized test groups. In these cases, they don’t measure causality or the incremental value of a strategy. Therefore, they are not recommended when strategy A has a different baseline conversion rate than strategy B, such as audience. Not all tests are appropriately powered with adequate conversion numbers in each group. Example of A/B test Context An advertiser running ads in Feed on Facebook only wants to reduce their average CPA. Hypothesis They seek to test the hypothesis that incorporating Instagram Feed as an additional placement along with Feed on Facebook would reduce their average CPA. Measurement approach They run an A/B test to measure the cost per result from both strategies. Identify next steps. Once you’ve validated or invalidated your hypothesis, determine the possible actions for the outcomes and recommend appropriate next steps. For example, if your hypothesis was validated, you might test a new hypothesis. You might find that your insights will lead to new questions and new hypotheses that will be the foundation for new tests. It’s always a good idea to think about testing beyond what you have already tested. HYPOTHESIZE 19