203 Lecture 3 Theme 3 Comparing groups 2023.pptx
Document Details
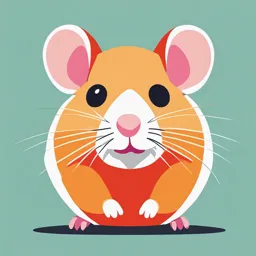
Uploaded by ProlificSynergy
Brighton and Sussex Medical School
Full Transcript
Collecting data about people and comparing the health of groups D R C L I O B E R RY S E N I O R L E C T U R E R I N H E A LT H C A R E E VA LU AT I O N A N D I M P R OV E M E N T C . B E R RY @ BS M S . AC . U K Learning aims To explain why we need to compare health needs of different groups To b...
Collecting data about people and comparing the health of groups D R C L I O B E R RY S E N I O R L E C T U R E R I N H E A LT H C A R E E VA LU AT I O N A N D I M P R OV E M E N T C . B E R RY @ BS M S . AC . U K Learning aims To explain why we need to compare health needs of different groups To be able to explain the different types of data used in health research To understand the principles of case-control studies and RCTs To understand the different uses of the t test and χ 2 (chi square) test To be able to explain the meaning of p value, confidence intervals, and their relationship to sample size To be able to explain Type I and Type II error Core material Introductory/ example material Why compare the health of groups? Research questions such as: o Is this disease increasing in prevalence? o Does it occur with undue frequency in my local community? o Is incidence associated with some suspected risk factor? o Has the outcome changed since control measures were instituted? Differences between groups at a point in time / Differences between groups over time How do we compare the health of groups? o Cross-sectional study one group surveyed to test associations between exposures and outcome/s o Ecological study community/population observed to test associations between exposures and outcome/s o Both studies focus on simultaneous observation of exposure and outcome, but difference is unit of observation o Cross-sectional study – focus on individual level data o Ecological study – focus on population-level data How do we compare the health of groups? o Cohort study disease-free participants followed up to see if they develop a disease (condition/outcome) of interest o Usually with groups who differ at outset on some exposure/s of interest o Case–control study groups who differ at outset on disease (condition/outcome) status o Look back at exposure/s of interest o Randomised controlled trial (RCT) groups who are randomly allocated to receive intervention/s versus comparator/s o Test safety and efficacy/effectiveness of interventions Case-control studies Case–control study groups who differ at outset on disease (condition/outcome) status - Two groups of participants are selected – one with condition (cases) and one without (controls) - Controls selected to be as similar as possible to the cases (e.g. age, gender, occupation, stage of illness, etc.) ◦ Variables not of interest are matched (i.e. potential confounders) at selection ◦ Exposures of interest are not measured or matched at selection - Always retrospective Past exposure/s in both groups E.g. interview/survey, historical records Case-control studies •We cannot calculate risk using case-control data • Because risk = probability of developing the outcome of interest •In case-control studies: • we have determined the outcome • i.e. we have selected participants into the case group or control group • we have decided the size of the groups •Therefore, we cannot calculate relative risk •Instead we calculate the odds of cases and controls in terms of their past exposures • We calculate an odds ratio (OR) which is very similar to the relative risk (RR) • And interpreted in the same way i.e. <1 exposure= protective, >1 exposure = risk factor, =1 no association Case-control studies African American Cancer Epidemiology Study (AACES) This example study shows that for African-American women, development of ovarian cancer was associated with: •Greater odds of 1 year prior to diagnosis, having a BMI of 25 or over •Reduced odds of having completed post-high school education https://bmccancer.biomedcentral.com/articles/10.1186/1471- Strengths and weaknesses of case control studies Can offer some evidence of cause – effect relationship i.e. association between exposure and outcome Can identify multiple exposures (both positive and negative associations) Good when disease/outcome is rare Minimises selection and information bias Retrospective - cheaper and typically shorter in duration Cannot calculate prevalence or incidence Less suitable for rare exposures Can be hard to ensure exposure occurred before onset Retrospective data availability and quality may be poor Suitable control group may be difficult to find Vulnerable to confounding The Randomised Controlled Trial RCT: a study in which participants are allocated randomly between an intervention (e.g. treatment) and a control group (e.g. no treatment or standard treatment) Why are RCTs conducted? Safety • • • Ascertain the safe dose of a new drug. Demonstrate safety and tolerability of a new compound Monitor adverse events profile of a new drug (against an existing drug or placebo) Efficacy/ Effectiveness • • • Demonstrate efficacy of new drug – does it work? Show that treatment T is superior or equivalent to treatment X Demonstrate effectiveness, and cost-effectiveness, of A 12 vs. B RCTs as an experiment RCTs are also a special type of experiment in which randomisation is used Randomisation means that potential confounding variables should be equally distributed between groups This creates two situations which are identical but: One situation in which the supposed cause (intervention of interest) is present One situation in which the supposed cause is absent RCTs can reduce confounding and allow identification of exposures which are causally related to disease of interest 13 ◦ i.e. identification of interventions which cause reduction in disease likelihood or severity Strengths and weaknesses of the RCT Establish the safety and efficacy/ effectiveness of new interventions Minimise selection and information bias Best single-study evidence for casual association between exposure (intervention) and outcome Time-consuming, difficult and expensive Not immune to bias Issues with participant drop-out Can lack generalisability How do we compare the health of groups? https://youtu.be/Jd3gFT0-C4s What data can we collect? Key data properties to consider: Categorical (discrete) Binary variables- e.g. Are you a parent: yes/no; diagnosis of endometriosis (yes/no) Several categories but no order (unordered categorical) – e.g. Sexual orientation; Smoking (never, former, current); Several ordered categories (ordinal) – e.g. Socio-economic status; categorised age; responses on Likert scale Continuous variables (scale) E.g. age; no. of symptoms; score on a questionnaire such as quality of life or satisfaction Error and Power No observed difference Observed Difference No true difference True difference Well Designed Trial Type II Error Type I Error Well Powered Study Protection against Type I error = threshold for determining when effects are significant ◦ Significance level for p value is typically accepted at 5% (or 0.05) i.e. 5% chance of type I error Protection against Type II error = power of the study to detect when significant effects are present ◦ Statistical power is typically accepted at 80 – 90% (or 0.8 – 0.9) i.e. 20% or 10% chance of type II error ◦ The larger the sample, the larger the statistical power Two aspects of assessing certainty of estimates P VALUES CONFIDENCE INTERVALS = probability = precision When you compare groups using a statistical test (t test, χ2), the result has a p value. The confidence interval describes the range of values with a given probability (e.g. 95%) that the true value of a variable is contained within that range. The p value is the probability that the difference observed (or one more extreme) could have occurred by chance if the groups compared were really alike. E.g. P 0.05 = 1/20, P 0.01 = 1/100 P value = type I error protection Larger sample = smaller p value Using data to compare health outcomes of groups Does one group report higher scores (on a continuous scale) than another? ◦E.g. is condition or intervention X associated with reduced symptoms compared to condition/intervention Y? ◦T-test or ANOVA Does one group have a higher proportion of an outcome than another (categories)? ◦E.g. is condition/intervention X associated with increased frequency of diagnosis P? ◦Chi squared test t-tests – what’s being tested? The (null) hypothesis: There is no difference in quality of life between women who have epithelial ovarian cancer (EOC) versus an ovarian germ cell tumour (OGCT) ◦ The mean quality of life in the EOC group is the same as the mean quality of life in the OGCT group The (alternative) hypothesis: There is a difference in mean quality of life between the groups (greater in EOC group) The between-group difference needs to be greater if there is more within-group variability e.g. if women vary greatly in quality of life within each group, we would need to see a bigger between-group difference to be sure it is a between-group difference Test output: t-statistic and accompanying p-value 20 T-test – Hypothesis: Quality of life is higher for women with epithelial ovarian cancer (EOC) versus an ovarian germ cell tumour (OGCT) N Mean Standard deviation EOC 6 5 1.65 OGCT 6 3.75 1.91 Hypothesis: Quality of life is higher for women with epithelial ovarian cancer (EOC) versus an ovarian germ cell tumour (OGCT) Quality of Life P=0.10 Conclusion: There is not enough evidence to suggest quality of life is higher for women with epithelial ovarian cancer (EOC) versus an ovarian germ cell tumour (OGCT) 22 Chi-square tests – what’s being tested? The (null) hypothesis: There is no association between endometriosis and ovarian cancer. ◦ The proportion of women with endometriosis who have ovarian cancer is the same as the proportion of women who do not have endometriosis and have ovarian cancer. The (alternative) hypothesis: There is an association between endometriosis and ovarian cancer. ◦ The proportion of women with endometriosis who have ovarian cancer is not the same as the proportion of women who do not have endometriosis and have ovarian cancer. Test output: chi-squared (χ2) statistic and accompanying p-value 23 Chi-Square- two groups with categorical outcome Hypothesis - Endometriosis increases likelihood of having Ovarian cancer Crosstabulation or Contingency Table Ovarian cancer No ovarian cancer Totals Endometriosis No endometriosis Observed = 136 (20.18%) Expected = 46.26 Observed = 818 (6.18%) Expected = 907.74 Observed = 538 (79.82%) Expected = 627.74 Observed = 12,408 (93.82%) Expected = 12,318.26 954 12946 Totals 674 13226 13900 (Grand Total) Kajiyama et al. (2019). Endometriosis and cancer. Free Radical Biology and Medicine, 133, 186-192. 24 Hypothesis: Endometriosis increases likelihood of having Ovarian cancer: Chi-square test P<0.001 Conclusion: There is strong evidence to reject the null hypothesis of no association between endometriosis and 25 ovarian cancer Two aspects of assessing certainty of estimates P VALUES CONFIDENCE INTERVALS = probability = precision When you compare groups using a statistical test (t test, χ2), the result has a p value. The confidence interval describes the range of values with a given probability (e.g. 95%) that the true value of a variable is contained within that range. The p value is the probability that the difference observed (or one more extreme) could have occurred by chance if the groups compared were really alike. E.g. P 0.05 = 1/20, P 0.01 = 1/100 P value = type I error protection Larger sample = smaller p value Confidence intervals • Confidence intervals (CIs) = range of values that likely contain the true population parameter • Use current sample to imagine how much observed sample values would vary if kept running the study • Create an upper and lower bound (range) which likely contain the true population parameter • 95% CIs would contain the true population value 95% of the time • CIs become narrower as the sample size increases • The larger the sample, the more likely it is that scores will cluster narrowly around the true population mean Confidence intervals • E.g. Odds of exposures for African-American women with ovarian cancer ◦ OR < 1 = protective association ◦ OR > 1 risk factor ◦ OR = 1, no association • Precision of example estimates fairly good, i.e. the 95% CIs are not very wide • But, for most exposures, CIs contain 1 (i.e. true population value could be OR =1, no association) • So can’t be confident about basing clinical recommendations/ predictions on findings Summary To measure existing health needs of populations at one time = prevalence and over time = incidence To compare the health needs/outcomes of different groups = measures of risk, differences in frequency and/or means Comparing groups can allow us to identify casual risk factors (exposures) relevant to the disease or condition of interest - Observational studies (e.g. case control) – describe population, identify potential causal exposure factors - Experimental studies (e.g. RCT) – test safety and efficacy/effectiveness (and best evidence of cause-effect) Selecting appropriate statistical test = consider nature of data - Continuous data e.g. quality of life = t-test or ANOVA - Categorical data e.g. disease presence or absence = chi-squared test In collecting data, we need to consider certainty - How to design a study that is adequately powered - Provide estimates of probability (p value) and estimates of precision (confidence intervals)