Breast Cancer Heterogeneity PDF
Document Details
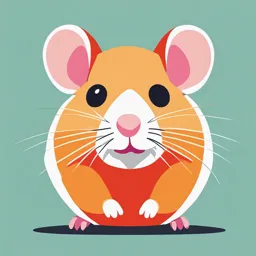
Uploaded by TopNotchSurrealism6085
Cardiff University
Tags
Summary
This document discusses the challenges of treating breast cancer due to its heterogeneous nature. It examines the concept of tumour heterogeneity, distinguishing between inter- and intra-tumour variations. The document explores the role of biomarkers in predicting treatment response and improving prognostication, aiming for more individualized and effective therapies.
Full Transcript
**[1 - MEASURING AND MODELLING]** **[HETEROGENEITY IN BREAST CANCER]** **[WHY DOESN\'T ONE DRUG FIT ALL FOR A PARTICULAR] [DISEASE?]** Oncology Challenges: -Oncology is one of the hardest fields to treat in US and European hospitals. -About 25% of oncology patients experience substantial improv...
**[1 - MEASURING AND MODELLING]** **[HETEROGENEITY IN BREAST CANCER]** **[WHY DOESN\'T ONE DRUG FIT ALL FOR A PARTICULAR] [DISEASE?]** Oncology Challenges: -Oncology is one of the hardest fields to treat in US and European hospitals. -About 25% of oncology patients experience substantial improvement in health and survival. -Approximately 7% of patients die from the treatment itself, -------------------------------------------------------------- -- highlighting a significant problem. Reasons for Poor Response to Drugs: -Cancer patients often respond poorly to existing drugs due to the complexity and variability of cancer. -It's not feasible to use every available drug on a cancer patient due to potential severe side effects and varying effectiveness. Treatment Considerations: -Near end-of-life cancer patients may accept treatments with severe side effects for a chance at extra weeks or months of life. -Different patients require different drugs based on their specific type of cancer and individual circumstances. -Patients vary in what side effects they are willing to tolerate. Quality of Life vs. Treatment: -There is a temptation to use aggressive treatments that can reduce quality of life and contribute to the 7% mortality rate. -This practice is globally accepted but not ideal. Therapy Safety and Effectiveness: -It's crucial to consider the safety and impact of therapy on the patient. -Focus should be on understanding why patients respond poorly to drugs and improving treatment strategies accordingly. **[TUMOUR HETEROGENEITY]** **[FUNDAMENTAL REASON BEHIND CANCER NOT BEING A]** **[SINGLE DISEASE = TUMOUR HETEROGENEITY]** Tumour Heterogeneity: - Differences between one tumour and the next. - Classified as either inter-tumour or intra-tumour heterogeneity. Inter-Tumour Heterogeneity: ------------------------------------------- -- in different patients or types of cancer. ------------------------------------------- -- - Tumour behaviour is influenced by its origin (e.g., breast cancer vs. colorectal cancer). - 95% of solid tumours arise from epithelial cells, but differences depend on the specific epithelial cells/tissues they originate from. - Therapies should target epithelial characteristics, which are similar across different tumour types. - Considering the **tumour MICROENVIRONMENT** (surrounding environment of a tumour) is crucial as it influences tumour growth and response to therapies. Intra-Tumour Heterogeneity: **-Intra-Tumour Heterogeneity** = heterogeneity (diversity) of cell types within a single solid tumour (e.g., breast cancer). - Result of **CLONAL EXPANSION** of different subsets of mutated cells = as these cells continue to mutate (subsequent mutations), some gain a survival advantage, allowing them to outcompete other cells (these mutations lead to pockets of activity) within the tumour - These advantaged cells outcompete others, dominating the **[INTER-TUMOUR HETEROGENEITY = BETWEEN TUMOURS]** **[AND/OR PATIENTS]** **[INTRA-TUMOUR HETEROGENEITY = WITHIN A TUMOUR]** \* Metastasis in cancer refers to the process by which cancer cells spread from the primary (original) site to other parts of the body. This occurs when cancer cells break away from the original tumour, travel through the bloodstream or lymphatic system, and form new tumours (secondary tumours) in other organs or tissues. **[TREATMENTS?]** Tumours Arise from Different Tissues: -Tumours originate from various tissues in the body. -Each tumour has a different "cell-of-origin." Genetic and Phenotypic Heterogeneity: -Tumours are genetically and phenotypically diverse. -This heterogeneity means that cells within a single tumour can ----------------------------------------------------------------- -- vary significantly. Treatment Ineffectiveness: -Treatments don't work on all tumours or all cells within a tumour. -Due to tumour heterogeneity, a single treatment is not --------------------------------------------------------- -- effective for all tumours or all cells within a tumour. Challenges in Predicting Response and Prognosis: -It is difficult to predict how a tumour will respond to therapy. -Accurately forecasting a patient's prognosis is challenging because cancers can become resistant and gain survival advantages through mutations. -Not all cancers respond the same way to treatment. Resistance to Therapy: -Cancer cells can develop resistance to treatments, making ------------------------------------------------------------ -------------------------------- them less effective over time. This resistance can arise from genetic mutations or adaptations within the tumour cells. Complexity of Cancer: -Cancer is not a single disease but a combination of hundreds of ------------------------------------------------------------------ -- -- different diseases. Each type of cancer has different aetiology (causes) and mechanisms. **[IS THERE A SOLUTION TO TREATMENT OF HETEROGENOUS] [DISEASE?]** **1. [Finding a common cause or mechanism...]** Targeting Common Mechanisms: - This approach aims to address the root cause shared by many cancers. - Example: Chemotherapy agents target proliferating cells. - Cancers often have **abnormal proliferation**, meaning they grow uncontrollably. Challenges: - Not all parts of a tumour grow rapidly; some cells lie dormant and resist treatment. - Off-target effects can harm other rapidly proliferating cells in the body, such as those in the gut, bone marrow, and immune system (T/B cells). **2. [Stratification of the Disease into Subtypes...]** Personalised Medicine: - Stratification involves dividing a disease (e.g., breast cancer) into subtypes based on specific characteristics, which allows for more tailored treatments (by targeting therapies to the specific needs of each subtype) Using Biomarkers: **-BIOMARKERS** = these are measurable indicators found in cells that can provide valuable information about the disease. - Cells are labelled or identified by characteristics that serve as biomarkers. - Biomarkers can be **prognostic** (indicating the likely course of the disease) or **predictive** (indicating likely response to treatment). **[PROGNOSTIC = PREDICT HOW AGGRESSIVE CANCER MIGHT BE] [OR LIKELIHOOD OF ITS RECURRENCE]** **[PREDICTIVE = PREDICT HOW LIKELY A PATIENT IS TO RESPOND]** **[TO A PARTICULAR TREATMENT]** By targeting common mechanisms and stratifying diseases into subtypes, treatments can become more effective and personalized, addressing the unique aspects of each patient\'s cancer. **[BIOMARKERS IN CANCER TREATMENT]** 1. **Prognostic Biomarkers:** - Purpose: Identify clinical outcomes. - Example: A factor on the outside of a cancer cell indicating tumour aggressiveness. - Presence: Suggests the tumour is very aggressive, leading to a poorer prognosis. -Absence: Indicates a better clinical outcome for the patient. - Utility for Doctors: Helps decide whether to treat a patient. Often more important to know when not to treat to avoid --------------------------------------------------------- -- harsh treatments that may not be necessary. - Mechanistic Insight: The biomarker may reveal information about the disease mechanism. - For example, gene X might drive aggression ( so therapies can be designed to specifically inhibit its activity), be involved in cell-cell communication, or influence metastasis. -Understanding how gene X contributes to metastasis can help predict which cancers are more likely to spread to other parts of the body. - Understanding these mechanisms helps in predicting outcomes and developing targeted therapies. 2. **Predictive Biomarkers:** - Purpose: Determine if a specific treatment will work for a patient. - Function: Indicates the likelihood of a patient responding to a particular treatment (positive response) **-Companion Diagnostics** = tests used to identify specific predictive biomarkers. - Example: Before administering treatment X, test for the related biomarker. - Presence: Likely indicates the treatment will be effective. - Absence: Suggests the treatment will not be effective. Importance: - The biomarker plays a role in the disease or the patient\'s response to treatment. - Treatment X is designed to target the mechanism involving the biomarker. - Without the biomarker, the treatment cannot perform its intended function effectively. -This means that the treatment's effectiveness relies on the presence of the biomarker to interact with and inhibit the disease process. **[PROGNOSTIC BIOMARKER ABSENT = BETTER CLINICAL]** **[OUTCOME FOR PATIENT]** **[PREDICTIVE BIOMARKER PRESENT = TREATMENT WILL BE]** **[EFFECTIVE]** **[BIOMARKERS CONTINUED...]** Biomarkers are biological measures used to assess the presence or progress of a disease or the effects of treatment. They can be found in blood, other body fluids, or tissues. **Prognostic biomarkers key points:** - They provide information about a patient\'s overall clinical outcome, regardless of the treatment they receive. -They help predict the overall outcome for a patient, such as the likelihood of disease recurrence or survival. -They must be easy to measure. -They have implications for therapy, particularly in deciding not ----------------------------------------- ------------------------------ to treat with certain therapies if they effective. - **Example:** If a patient has a specific subtype of disease with a certain biomarker, it might indicate that a particular treatment (e.g., Treatment X) will not be effective. This helps avoid unnecessary treatments that could be harmful. **Predictive biomarkers key points:** -They identify patients who are likely to respond to a specific treatment. -They help determine which patients will benefit from a particular treatment. - Heterogeneity: They reveal the variability within tumours, indicating that not all tumour cells may respond to the treatment. - Statistical tests are necessary to determine the overall clinical benefit. - **Example:** A patient may test positive for a predictive biomarker, suggesting that a specific treatment will be effective. However, not all tumour cells may have this biomarker. For instance, if 90% of tumour cells have the biomarker and respond to the treatment, the remaining 10% may not, leading to potential relapse as these cells can grow back. **[HETEROGENEITY IN BREAST CANCER]** In the UK: 1 in 9 women will be diagnosed with BC in their lifetime; 44,000 women will be diagnosed each year and 12,500 will die from BC related disease. Fundamental Morphology of the Breast: Epithelial Compartment - Tumour Origin: About 95% of all breast tumours originate from the epithelial compartment. - Structure: The epithelial compartment consists of a ductal system made up of **tubular ducts**. These ducts are composed of small sacs or bubbles, which can be visualized as a \'seaweed\' structure with a single strand branching out to various sacs. - Interconnection: These sacs are interconnected and lead to a central duct. - **Secretory Cells** = epithelial cells are the secretory cells of the breast. During pregnancy, these elongated cells (apical cells) secrete milk into the lumen. Non-Pregnant State: - Cell Presence: In non-pregnant females, many of these epithelial cells are absent because there is no need for them. - Gland Weight: Consequently, the epithelial content is very low in terms of the overall weight of the gland. Changes During Pregnancy: - Cell Proliferation: During pregnancy, there is a significant proliferation of epithelial cells. The gland transforms into a \'milk-making factory\' to nourish the young. - Post-Lactation: Once the child no longer requires milk, these epithelial cells undergo natural apoptosis (programmed cell death) and regression. - Evolutionary Reason: This process occurs to prevent a large number of epithelial cells from remaining without function, which could increase the risk of mutations. Residual Epithelial Compartment: - Not Completely Removed: Although many epithelial cells are removed after lactation, a residual compartment remains. This residual compartment is more prevalent after a single pregnancy. Summary: **-Epithelial Compartment:** Key area where most breast tumours originate, consisting of a ductal system with secretory cells. - **Pregnancy Impact:** Massive proliferation of epithelial cells during pregnancy, followed by apoptosis post-lactation to reduce mutation risk. - **Residual Cells:** Some epithelial cells remain after pregnancy, forming a residual compartment.  **[HOW DO WE CATEGORISE BREAST CANCER? -]** Breast cancer (BC) is categorized based on its pathology and the characteristics of the cancer cells. Classical Traditional Way of Characterizing Breast Cancer: Traditionally, breast cancer is studied by examining tissue sections under a microscope. This method helps identify various types of breast cancer based on their appearance and behavior. Classic Pathology Subtypes: **1. DCIS = Ductal Carcinoma In Situ:** - Common Subtype: One of the most common types of breast cancer. - Cell Mutation: In DCIS, a cell undergoes a proliferative mutation, survives, and continues to divide, filling up the sac (alveoli) with cancer cells. - Location: This can occur in the alveolar sacs at the end of the ducts or within the ducts themselves (lobular areas). - Non-Life Threatening: At this stage, the cancer is not lifethreatening because the mammary gland is not a vital organ. Surgical removal of the lump is often the most effective treatment. **More Aggressive Subtypes?** - Abnormal Cancer Cells: These subtypes involve cancer cells that attempt to form their own ducts or sacs. Although they are abnormal, they are quite differentiated, meaning they try to mimic normal mammary gland structures but fail. - Expansion: These cells continue to expand, potentially leading to more aggressive forms of cancer, such as infiltrating ductal carcinoma (IDC) **2. IDC = Infiltrating Ductal Carcinoma:** - Life-Threatening: At this stage, the epithelial cancer cells take on different characteristics and become life-threatening. - Infiltration: The cancer cells may infiltrate the surrounding stroma, blood vessels, and lymph vessels. This infiltration marks a significant drop in life expectancy and survival rates. **[HETEROGENEITY AND STRATIFICATION OF BREAST CANCER]** -Breast cancer is heterogeneous, meaning it consists of different types of cancer cells with varying characteristics. This heterogeneity affects how the cancer behaves and responds to treatment. Stratification of Breast Cancer: -Stratification involves categorizing breast cancer into subtypes based on specific characteristics, which helps tailor treatment plans. - **Histological Subtypes:** Breast cancer is categorized into subtypes like DCIS, IDC, and others based on the microscopic appearance of the cells. - **Molecular Subtypes:** Advances in molecular biology have led to the identification of molecular subtypes based on genetic and protein expression profiles. Examples include hormone receptor-positive, HER2-positive, and triple-negative breast cancers. - Treatment Implications: Understanding the subtype of breast cancer helps in selecting the most effective treatment. **[STRATIFICATION OF BREAST CANCER]** Classical Classification Systems: -The classical way to classify breast cancer involves grading the ------------------------------------------------------------------- -- tumour based on its appearance under a microscope. grading system helps predict the aggressiveness of the cancer and the patient\'s prognosis. Explanation of Grades: **1. G1 (Well Differentiated, Low Grade):** - Appearance: Tumors still resemble normal alveolar sacs but show increased cell proliferation. - Prognosis: Generally good, with high expected survival rates and positive outcomes. 2. **G2 (Moderately Differentiated, Intermediate Grade):** - Appearance: Tumors show more abnormalities and less resemblance to normal tissue compared to G1. - Prognosis: Intermediate, with moderate survival rates and outcomes. 3. **G3 (Poorly Differentiated, High Grade):** - Appearance: Tumors are significantly abnormal and less like normal tissue. - Prognosis: Poorer than G1 and G2, with lower survival rates and outcomes. **4. G4 (Undifferentiated, High Grade):** - Appearance: Tumors lack any normal structure and integrate into the surrounding stroma. - Prognosis: Very poor, with low survival rates and outcomes. **[What about Heterogeneity?]** - Impact on Treatment: The heterogeneity of breast cancer affects how it responds to treatment. Some subtypes may respond well to certain therapies, while others may not. - Personalized Medicine: Understanding the specific subtype and grade of breast cancer helps in tailoring treatment plans to the individual patient, improving the chances of successful outcomes. **[INTRA-TUMOUR HETEROGENEITY]**  Intra-Tumour Heterogeneity = refers to the diversity of cell types within a single tumour. This complexity can significantly impact treatment strategies and outcomes. Tumour Microenvironment (TME) - consists of various components, including: - **Tumour Epithelium**: The cancerous cells originating from the epithelial tissue. - **Stroma**: The supportive tissue surrounding the tumour, which includes: 1. **Fibroblasts**: Cells that produce collagen and other fibres. 2. **Endothelium**: Cells lining the blood vessels. 3. **Neuro-endocrine Tissue**: Cells that release hormones into the blood. 4. **Immune Cells and Macrophages**: Cells involved in the body\'s immune response. Mitotic Index **-Mitotic index** -------------------- -- death. = a measure of cell proliferation versus cell -In tumours, there is typically an increase in cell division, which can continue even during treatment. -This imbalance between cell proliferation and apoptosis (programmed cell death) is a hallmark of cancer. Cross-Section of a Breast Cancer Tumour A cross-section of a classical breast cancer tumour reveals its heterogeneity: - **Non-Homogeneous Structure**: The tumour is not a uniform layer of epithelial-like cells. Instead, it contains various cell types, including myoepithelial and fibroblast-like cells. This diversity contributes to the tumour's complexity and affects how it grows and responds to treatment. - **Quasi-Normal Cells**: Some cells within the tumour may appear genetically normal but behave abnormally. This abnormal behavior is often due to their proximity to cancerous cells, which send out abnormal signals that influence the quasinormal cells. **[What are the Treatment Challenges?]** - Targeting Different Cell Types: The diversity of cell types within the tumour makes it challenging to target all cells effectively with treatment. - Drug Uptake Barriers: High collagenous areas within the tumour can act as barriers to drug penetration, complicating treatment. Cancer Stem Cells - Small Proportion: Cancer stem cells make up a very small percentage (around 1%) of the tumour mass but are highly malignant. - Resistance: These cells are difficult to identify and treat, as they are resistant to conventional therapies. They are responsible for new tumour growth, similar to seeds in a fruiting body. **[THE PROBLEM WITH CLASSIC HISTOLOGY AS A MEANS OF]** **[PREDICTING OUTCOME]** Classical histology, which includes the histopathological type and grade of tumours, has been a traditional method for assessing breast cancer. However, it has several limitations when it comes to predicting clinical outcomes and treatment responses. Classical Classification Systems: - Histopathological Type and Grade: These systems categorize tumours based on their microscopic appearance and how much the cancer cells resemble normal cells. - Prognosis: They provide an approximate measure of prognosis, indicating how aggressive the cancer might be and the likely survival rates. Limitations of Classical Histology: **1. Lack of Precision:** - Approximate Measures: While these systems give a rough idea of prognosis, they are not precise enough to accurately predict individual patient outcomes. - Inefficiency: They are not efficient in providing detailed information about which patients will respond to specific treatments. **2. Inability to Discriminate:** - Clinical Outcome: Classical histology does not effectively differentiate between patients with different clinical outcomes. For example, two patients with the same histological grade might have very different survival rates and disease progressions. - Treatment Response: These systems do not indicate which treatments will be most effective for individual patients. This is crucial because different patients may respond differently to the same treatment. **[SOLUTION?]** Using Molecular Biomarkers to Improve Breast Cancer Stratification: over the past 20-30 years, the use of molecular biomarkers has emerged as a solution to the limitations of classical histology in predicting breast cancer outcomes and treatment responses. Classical Prognostic Biomarkers: traditionally, breast cancer prognosis has been assessed using several key factors: 1. **Type/Grade:** The histopathological type and grade of the tumour, which provide a rough estimate of prognosis. 2. **Nodal Status:** The presence of cancer cells in the lymph nodes within the breast, indicating potential spread. 3. **Lymphatic/vascular Invasion:** The invasion of cancer cells into the lymphatic or vascular systems, suggesting a more aggressive disease. 4. **Tumour Size:** An important indicator of disease advancement and overall prognosis. 5. **Cofactors:** Age, ethnicity, smoking, drinking, obesity, and other comorbidities, which can influence overall risk and life expectancy. -While these factors provide a combination of risk indicators, they are not always accurate in predicting individual outcomes or treatment responses. Molecular Biomarkers: molecular biomarkers offer a more precise method for stratifying breast cancer into additional subtypes, improving the accuracy of prognosis and treatment planning. **1. Hormone Receptor Status:** - **Oestrogen Receptor (ER) and Progesterone Receptor (PR):** The presence of these receptors indicates that the cancer may respond to hormone therapy. - HER2 Status: Human Epidermal growth factor Receptor 2 (HER2) positive cancers may respond to targeted therapies like trastuzumab. **2. Genetic and Protein Expression Profiles:** - Gene Expression Tests: Tests like Oncotype DX and MammaPrint analyse the expression of specific genes to predict the risk of recurrence and the likely benefit of chemotherapy. - Protein Markers: The presence of certain proteins can indicate how aggressive the cancer is and how it might respond to treatment. **3. Additional Stratification:** - Molecular Subtypes: Breast cancer can be divided into subtypes such as Luminal A, Luminal B, HER2-enriched, and Triple-negative, each with distinct characteristics and treatment responses. **[MOLECULAR BIOMARKERS = BETTER THAN PROGNOSTIC AS]** **[DIVIDES BREAST CANCER INTO ADDITIONAL SUBTYPES]** **[(STRATIFICATION)]**  **[THE FIRST MOLECULAR (AND TARGETABLE) BIOMARKERS FOR] [BREAST CANCER: OESTROGEN RECEPTOR]** [https://www.science.org/doi/epdf/10.1126/science.3753802] George Beatson\'s Study (1896): - Discovery: George Beatson, a clinician, performed an ovariectomy (removal of ovaries) on a breast cancer patient and observed a dramatic effect on disease progression. Significance: This study highlighted the role of oestrogen in breast cancer, as removing the ovaries (the main source of oestrogen) significantly impacted the disease. Geoffrey Greene\'s Study (1986)\*: - Cloning of Oestrogen Receptor: In 1986, Geoffrey Greene and his team cloned the oestrogen receptor from a breast cancer cell line called MCF7. This process involved isolating and replicating the receptor, allowing for detailed study and analysis. - Importance: The study revealed that the oestrogen receptor plays a crucial role in the development and progression of breast cancer. This receptor, when bound by oestrogen, can promote the growth of cancer cells. By understanding the function and structure of the oestrogen receptor, researchers were able to develop targeted therapies. These therapies, such as hormone treatments, specifically inhibit the receptor's activity, effectively slowing down or stopping the growth of cancer cells. This discovery has led to more personalized and effective treatment options for patients with breast cancer. **[SUMMARY:]** **[GEORGE BEATSON 1896 = OVARIECTOMY OF BC PATIENT]** **[GEOFFREY GREENE 1986 = CLONED ER FROM MCF7 CELLS]** What is the Oestrogen Receptor? - Type: The oestrogen receptor is a nuclear receptor and a transcription factor. - Function: Oestrogen enters the cell, travels to the nucleus, and binds to the oestrogen receptor. This complex then binds to DNA, promoting the activation of genes involved in cell proliferation and survival. - Mutations: Mutations or upregulation of the oestrogen receptor can lead to increased activation of these target genes, contributing to cancer progression. **[ER + OESTROGEN = BIND TOGETHER]** **\*GEOFFREY GREENE PAPER explained...** Mechanism of Oestrogen Receptor and Steroid Hormone Receptors in Gene Regulation This paper explores the mechanism by which the oestrogen receptor (ER) and other steroid hormone receptors regulate gene expression in eukaryotic cells. Oestrogen Receptor (ER) Cloning and Expression **1. Cloning:** - Researchers cloned the entire translated portion of the messenger RNA (mRNA) for the oestrogen receptor from MCF-7 human breast cancer cells. - This complementary DNA (cDNA) was then sequenced. **2. Expression in CHO Cells:** - The cDNA was expressed in Chinese hamster ovary (CHO-K1) cells, producing a functional oestrogen receptor protein. - The open reading frame of the cDNA corresponded to a polypeptide of 595 amino acids with a molecular weight of approximately 66,200, consistent with known values for the oestrogen receptor. **[cDNA = OESTROGEN RECEPTOR]** Functional Analysis **1. Binding and Sedimentation:** - The expressed protein **(protein = ER)** in CHO cells bound to \[3H\]estradiol, a radioactive form of oestrogen. **[CHO = Chinese] [hamster ovary cells]** - In salt-containing sucrose gradients, the receptor-estradiol complex sedimented as a 4S complex, and as an 8 to 9S complex in the absence of salt. **2. Antibody Interaction:** \- The receptor-\[3H\]estradiol complex interacted with a monoclonal antibody specific for primate ER, confirming the identity of the expressed cDNA as the human oestrogen receptor. Homology and DNA-Binding Domain **1. Amino Acid Sequence Comparisons:** \- Significant regional homology was found among the human oestrogen receptor, the human glucocorticoid receptor, and the putative v-erbA oncogene product. - This suggests that steroid receptor genes and the avian erythroblastosis viral oncogene are derived from a common primordial gene. **2. Homologous Region:** - The homologous region, rich in cysteine, lysine, and arginine, may represent the DNA-binding domain of these proteins. Mechanism of Action - Nuclear Receptor and Transcription Factor: - The oestrogen receptor is a nuclear receptor that functions as a transcription factor. - Oestrogen enters the cell, travels to the nucleus, and binds to the oestrogen receptor. - The oestrogen-receptor complex then binds to specific DNA sequences, promoting the activation of genes involved in cell proliferation and survival. **[ER = ACTIVATES GENES INVOLVED IN CELL PROLIFERATION]** Gene Activation: - The activated genes are primarily involved in cell proliferation and survival. - Mutations or upregulation of the oestrogen receptor can lead to increased activation of these target genes, contributing to cancer progression. Experiment Summary: 1. Cloning and Expression: The oestrogen receptor was cloned from MCF-7 cells and expressed in CHO cells, producing a functional protein. 2. Functional Analysis: The receptor bound to estradiol and interacted with specific antibodies, confirming its identity. 3. Homology: Significant homology was found with other steroid receptors, suggesting a common evolutionary origin. 4. Mechanism: The oestrogen receptor acts as a nuclear receptor and transcription factor, regulating gene expression involved in cell proliferation and survival. **[THE FIRST MOLECULAR (AND TARGETABLE) BIOMARKERS FOR]** **[BREAST CANCER: HER2 RECEPTOR]** Dennis Slamon\'s Study (1987)\*: Discovery: Dennis Slamon is widely regarded as the pioneer of HER2-targeting therapies. In 1987, he identified the HER2 receptor in breast cancer.   [So, what is the HER2 Receptor?] - Cell Surface Receptor: HER2 is a receptor located on the surface of cells. - Family: It is a member of the Epidermal Growth Factor Receptor (EGFR) family of receptors. - **Activation and Signalling:** - **Dimerization/Trimerization**: HER2 signals through dimerization or trimerization at the cell surface. -Dimerization is the process where two HER2 molecules pair up at the cell surface. -This pairing activates the receptor's kinase activity, leading to a cascade of downstream signalling pathways that promote cell growth, survival, and proliferation. -Trimerization involves the formation of a complex of three HER2 molecules or a combination of HER2 with other HER family members. -Similar to dimerization, trimerization activates multiple signalling pathways, but with potentially greater complexity and strength. - **Proliferation and Survival**: Once activated, HER2 sends signals that promote cell proliferation and survival, making it a critical factor in cancer progression. In many cancers, particularly breast cancer, HER2 is overexpressed, leading to excessive dimerization or trimerization, leading to uncontrolled cell growth and division, contributing to tumour development and progression. HER2 as a molecular biomarker: **HER2 (Human Epidermal growth factor Receptor 2)** = is another critical molecular biomarker in breast cancer - Role: HER2 is a protein that promotes cell growth. In some breast cancers, the HER2 gene is amplified, leading to overexpression of the HER2 protein. - Impact: HER2-positive breast cancers tend to be more aggressive and have a poorer prognosis compared to HER2negative cancers. Targeted Therapy explained: - Trastuzumab (Herceptin): The development of **trastuzumab**, a monoclonal antibody that targets the HER2 receptor, revolutionized the treatment of HER2-positive breast cancer. It binds to the HER2 protein, inhibiting its activity and marking the cancer cells for destruction by the immune system. Effectiveness: Trastuzumab has significantly improved outcomes for patients with HER2-positive breast cancer, making HER2 one of the first and most important targetable ------------------------------------------------------------ -- biomarkers in breast cancer treatment. **\*DENNIS SLAMON PAPER explained...** [https://www.science.org/doi/epdf/10.1126/science.3798106] - HER-2/neu Oncogene in Breast Cancer: The HER-2/neu oncogene, also known as ERBB2, is a member of the erbB-like oncogene family. It is related to, but distinct from, the epidermal growth factor receptor (EGFR). This gene plays a crucial role in the development and progression of certain aggressive types of breast cancer. Key Findings: **1. Gene Amplification:** \- Prevalence: The HER-2/neu gene is often found in higherthan-normal amounts (amplified) in breast cancer cells. In a study of 189 breast cancer cases, 30% showed this gene was amplified between 2 to over 20 times the normal level. - Significance: The amplification of the HER-2/neu gene is a strong indicator of how long a patient might survive and how quickly the cancer might return after treatment. Higher amplification generally means a poorer prognosis. **2. Correlation with Disease Parameters:** - **Prognostic Value** = ability to predict the outcome of a disease. Even when considering other factors that affect breast cancer prognosis, HER-2/neu amplification remains a crucial predictor. It is more reliable than many traditional factors, such as hormone receptor status, especially in patients with cancer that has spread to the lymph nodes. - The data suggest that HER-2/neu amplification influences how aggressively the cancer behaves and develops. This means that tumours with this amplification may grow and spread more rapidly. Clinical Implications: - The identification of HER-2/neu as a **significant prognostic marker** ( = an important indicator of how breast cancer will progress) led to the development of targeted therapies, such as trastuzumab (Herceptin), which specifically target the HER-2 receptor. - These therapies have significantly improved the prognosis for patients with HER-2-positive breast cancer, making HER-2 one of the most important molecular biomarkers in breast cancer treatment. **[\*\*AARONSON PAPER explained...]** **[erbB-2 Is a Potent Oncogene When Overexpressed in NIHI/3T3]** **[Cells]** - [https://www.science.org/doi/epdf/10.1126/science.2885917] erbB-2 as a Potent Oncogene: The erbB-2 gene, also known as HER2, is a significant oncogene when overexpressed -Gene and Protein: The erbB-2 gene encodes a protein similar to growth factor receptors. This protein is involved in cell signalling pathways that regulate cell growth and survival. - Expression in NIH/3T3 Cells: Researchers expressed erbB-2 complementary DNA (cDNA) in NIH/3T3 cells, a type of mouse fibroblast cell line, using the SV40 promoter. Initially, despite detectable levels of the erbB-2 protein, the gene did not exhibit transforming activity, meaning it did not induce cancerous changes in the cells. Key Findings: **1. Increased Expression:** \- When the erbB-2 gene's expression was increased five- to tenfold using a specific viral element (LTR of Moloney murine leukaemia virus), it showed strong cancer-causing (oncogenic) activity. This means that higher levels of this gene can significantly promote cancer development. - Malignant Transformation: High levels of the erbB-2 protein were linked to the transformation of NIH/3T3 cells (a type of mouse cell line) into cancer cells. This indicates that overexpression of the erbB-2 gene can drive the development of cancer. **2. Human Tumors:** - Overexpression in Tumors: Similar high levels of the erbB-2 protein were found in human breast cancer cells that overexpressed this gene. This suggests that the same mechanism observed in the mouse cells also occurs in human cancer cells. - Mechanism of Oncogenesis: These findings suggest a new way that genes encoding growth factor receptor-like proteins, such as erbB-2, can become cancer-causing through overexpression. This means that simply having too much of this protein can turn normal cells into cancer cells. **[SO HER2 IS AN ONCOGENE = CAN TURN NORMAL CELLS INTO]** **[CANCER CELLS WHEN PRESENT IN HIGH AMOUNTS]** **[(OVEREXPRESSION)]** Implications: - Oncogenic Properties: The study demonstrates that the overexpression of erbB-2 can transform normal cells into cancerous cells, highlighting its role as a **potent oncogene**. - Cancer Development: The overexpression of erbB-2 in human tumours underscores its importance in the development and progression of malignancies, particularly in breast cancer. **[HOW DO WE TEST & MEASURE THESE MOLECULAR] [BIOMARKERS IN PATIENTS?]** The best method to measure and test molecular biomarkers in patients is through \*\*immunohistochemistry (IHC)\*\*. This technique uses antibodies to detect specific proteins in tissue samples, which are then visualized using a colorimetric agent. 1. **[Immunohistochemistry (IHC): OESTROGEN]** Oestrogen Receptor (ER) Staining: - Appearance: In IHC staining for the oestrogen receptor, the nuclei of cancer cells appear brown. - Localization: The oestrogen receptor is a nuclear receptor, so the staining is localized to the nuclei, not the surrounding cytoplasm. **[ER STAINING = ER IS A NUCLEAR RECEPTOR]** **2. [Immunohistochemistry: HER2]** HER2 staining: - Appearance: In IHC staining for HER2, there is a halo-like stain around the nuclei of the cells. - Localization: HER2 is a cell surface receptor, so the staining appears around the edge of the cell surface. **[HER2 STAINING = HER2 IS A CELL SURFACE RECEPTOR]**  Pie Chart Explanation: Using IHC markers on histopathological samples allows for the stratification of breast cancer populations based on ER and HER2 staining: 2. **ER/Hormone Receptor Positive**: 60-70% of breast cancer cases are positive for oestrogen or hormone receptors. 3. **HER2 Positive:** About 25% of breast cancer cases are HER2 positive. 4. **Triple-Negative:** 10-15% of breast cancer cases do not overexpress either ER or HER2. This group lacks clear biomarkers driving their disease, making it more challenging to understand and treat. Importance of Molecular Biomarkers: - Both ER and HER2 play crucial roles in the pathology (causes and effects of diseases) and aetiology (origin or cause) of breast cancer. - These receptors are not only important biomarkers but also indicators of the underlying mechanisms driving the cancer in specific tumour cells. **[Oestrogen Receptor (ER) and HER2 continued...]** Treatment Implications: - Targeted Treatments: If a treatment targets either the oestrogen receptor (ER) or HER2, patients generally have better outcomes. This is because these receptors are both prognostic and predictive biomarkers. 5. **Estrogen Receptor (ER)** Prognostic Biomarker: - Good Prognosis: Patients with ER-positive breast cancer typically have a relatively good prognosis compared to those with triple-negative breast cancer (which lacks ER, PR, and HER2 expression). - Triple-Negative Breast Cancer: This type does not express ER or HER2, making it more challenging to treat and generally associated with a poorer prognosis. Predictive Biomarker: - Mechanism of Disease: Understanding that ER drives the disease mechanism allows for targeted treatments. - Targeted Drugs: ER-positive patients are likely to respond to anti-oestrogen therapies such as tamoxifen or faslodex, which block the oestrogen receptor. **2. HER2** Prognostic Biomarker: - Aggressive Disease: HER2-positive breast cancers tend to be more aggressive, but the presence of HER2 also provides a target for specific therapies. Predictive Biomarker: - Targeted Therapy: HER2-positive patients are treated with trastuzumab (Herceptin), an antibody that specifically targets the HER2 receptor, improving outcomes significantly. -slide shows **4 basic subtypes of breast cancer** (in orange) and their prognosis (good/poor) and treatment options (on the right) Breast Cancer Subtypes: -Classification: The subtypes are generally arranged from those with a good prognosis to those with a poor prognosis. -Triple Negative Breast Cancer (TNBC): This subtype represents about 20% of the breast cancer population and typically has a poorer prognosis compared to ER+ (oestrogen receptor-positive) cancers. Targeted Therapies: -Receptor-Specific Drugs: The slide shows drugs that have been specifically designed to target the HER2 and oestrogen receptors. These drugs are effective because they directly target the overexpressed receptors in certain breast cancer subtypes. -Stratification: By identifying the overexpression of these receptors, patients can be stratified into groups that are more likely to respond to these targeted therapies. Success of Therapeutic Targets: -Effective Treatments: Targeted therapies for HER2 and ER+ breast cancers have been very successful, significantly improving outcomes for these patients. -Challenges with TNBC: The triple negative group, which lacks these receptors, has traditionally been considered "untreatable" with targeted therapies. As a result, chemotherapy and other non-receptor-targeted therapies are often used for these patients. **[Summary Breast Cancer Subtypes]** About 40 years ago, breast cancer was categorized into four subtypes based on the expression of two receptors: **1. HER2:** --------------------------------- -- **2. Oestrogen Receptor (ER):** Found on the cell surface. Located within the cell. **- Triple Negative Breast Cancer (TNBC):** This subtype has very low or no expression of these receptors, making it harder to treat. Epigenetics - Epigenetic changes refer to modifications in gene expression that do not involve changes to the DNA sequence itself. 1. Gene Amplification: -Not a Mutation in Sequence: Epigenetic changes do not necessarily mean mutations in the DNA sequence. -Duplication of Gene Locus: Sometimes, the gene locus (specific location of a gene on a chromosome) can be duplicated, creating multiple copies of the gene. -Overexpression of Receptors: This duplication leads to an overexpression of receptors like HER2 and oestrogen receptors, meaning there are too many of these proteins on the cell surface or within the cell. 2. Protein Overexpression: -Increased Protein Levels: The overexpression of these receptors increases the viability and proliferation rate of the cancer cells. -Disease Progression: This abnormal increase in cell growth and survival is a driving factor in the progression of breast cancer. Importance of Biomarkers -Drivers of Disease: In breast cancer, the oestrogen receptor and HER2 receptor can act as drivers of the disease, meaning they play a crucial role in the development and progression of cancer. -Biological and Therapeutic Significance: Understanding these molecular factors is essential for both biological research and developing targeted therapies. **[STRATIFICATION BIOMARKERS: Receptor Status as a]** **[Predictive Biomarker]** **Receptor status** = refers to the presence or absence of specific --------------------------------------------------------------------- -- -- receptors, such as the oestrogen receptor (ER), on breast cancer cells. This status is used as a predictive biomarker to guide treatment decisions. In table, can see a cohort of 75 are either ER+ or ER-... 1\. ER+ vs. ER- Disease: **- ER+ (Oestrogen Receptor Positive):** These patients have breast cancer cells that express the oestrogen receptor. **- ER- (Oestrogen Receptor Negative):** These patients lack the oestrogen receptor on their cancer cells. 2\. Response to Therapy: - ER+ Patients: About 50% of patients in cohort with ER+ breast cancer respond to anti-oestrogen or endocrine therapy. This means that while ER+ status can indicate potential responsiveness to these therapies, it is not a perfect predictor since the other 50% do not respond. - ER- Patients: These patients generally do not respond to endocrine therapy. Therefore, testing for ER status is useful to identify patients who should not receive this type of treatment. 3\. Predictive Value: - ER+ as a Biomarker: While ER+ status is a reasonable biomarker for predicting response to endocrine therapy, it is not highly reliable since only about half of the ER+ patients respond. - ER- as a Biomarker: ER- status is more definitive in predicting non-responsiveness to endocrine therapy, making it useful for ruling out this treatment option. 4\. Clinical Implications: - Treatment Decisions: Typing for ER status helps in deciding whether to use endocrine therapy. For ER- patients, alternative therapies need to be considered since endocrine therapy is unlikely to be effective. - Need for Additional Biomarkers: For ER+ patients, further molecular biomarkers are needed to better predict which individuals will respond to endocrine therapy and which will not. **[ER POSITIVE = 50 % REPSONSIVENESS TO ENDOCRINE]** **[THERAPY ER NEGATIVE = NON-RESPONSIVENESS TO]** **[ENDOCRINE THERAPY]**  Why is Receptor Status a better predictor than prognosticator? - A predictive biomarker helps predict a patient\'s response to a specific treatment, while a prognostic biomarker indicates the overall outcome or progression of the disease regardless of treatment. -The slide suggests that ER status is better as a predictive biomarker than as a prognostic biomarker. This means that ER status more effectively indicates how well a patient might respond to hormone therapies than it does in predicting the general progression of the disease. Cumulative Survival Comparison (Graph): -The graph on the slide shows cumulative survival rates for ER+ versus ER- patients, with ER+ patients generally having better survival outcomes. -This survival advantage underscores the effectiveness of hormone therapies for ER+ patients. **[BUT DOES IT WORK?]** Current Challenges: - Stratification by ER Status: While we can categorize patients into ER+ and ER- groups, this method has limitations. - Response to Therapy: Despite being classified as ER+, just under half of the patients do not respond to anti-oestrogen (endocrine) therapy. This indicates that simply identifying ER status is not sufficient for predicting treatment success. Reasons for Limited Effectiveness: - Heterogeneity: Breast cancer is a heterogeneous disease, meaning there is significant variability within the ER+ group. This variability affects how patients respond to treatment. - Crude Stratification: The current method of stratifying patients into ER+ and ER- groups is too simplistic. It does not account for the underlying differences within the ER+ group that influence treatment response. Need for Better Stratification: - Identify Non-Responders: We need to find ways to identify the subset of ER+ patients who will not respond to anti-endocrine therapy. This requires a deeper understanding of the molecular and genetic factors at play. - Additional Biomarkers: Over the past 20 years, extensive research has been conducted to find additional biomarkers. These biomarkers can help refine the stratification process and ----------------------------------------------------------------- -- improve the prediction of treatment responses. **[ARE THERE BETTER STRATIFICATION BIOMARKERS?]** -Yes, there are more advanced biomarkers that can better stratify breast cancer patients and better predict clinical outcomes and treatment responses, treatments can also be more effectively tailored to individual needs. -These biomarkers help in stratifying patients, meaning they can categorize patients into different groups based on their risk and likely response to treatments. -These include genomic, transcriptomic, and proteomic data. Types of Biomarkers **1. Genomic Biomarkers:** these are specific changes in the DNA - DNA Mutations (changes in the DNA sequence of genes): Specific gene mutations can provide insights into cancer behavior and treatment responses. For example: - **BRCA1/2 mutations** are linked to a higher risk of breast and ovarian cancers and can influence treatment decisions, such as the use of PARP - **PIK3CA mutations** are common in breast cancer and can affect how the cancer grows and responds to certain therapies. - **TP53 mutations** are often associated with more aggressive cancers and can impact prognosis and treatment strategies. \- Copy Number Variations: Changes in the number of copies of a particular gene can influence cancer progression and treatment efficacy. For example, amplification of the HER2 gene is a well-known CNV in breast cancer, leading to aggressive tumour growth. **2. Transcriptomic Biomarkers:** these are related to the study of the transcriptome, which is the complete set of RNA transcripts produced by the genome at any one time - these biomarkers help in understanding which genes are being actively expressed in a cell. - **Gene Expression Profiling**: This technique measures the levels of mRNA in cells. By analysing mRNA levels, scientists can determine which genes are active (expressed) or inactive in a --------------------------------------------------------------- -- particular cell or tissue. Key Oncogenic Drivers: This profiling helps identify important genes involved in cancer development and progression, such as: - **ER (Oestrogen Receptor):** A protein that, when activated by oestrogen, can promote the growth of some breast cancers. - **HER2 (Human Epidermal Growth Factor Receptor 2):** A protein that can promote the growth of cancer cells. In some cancers, high levels of HER2 are present. - **PI3K (Phosphoinositide 3-Kinase):** An enzyme involved in cell growth and survival, often mutated in cancers. - **mTOR (Mechanistic Target of Rapamycin):** A protein that regulates cell growth, proliferation, and survival. **[GENE EXPRESSION PROFILE ANALYSIS (TRANSCRIPTOMICS)]= A** **TECHNIQUE THAT MEASURES THE AMOUNT OF mRNA FOR EVERY GENE IN A CELL OR TISSUE -** by identifying which genes are on or off, researchers can better understand the molecular mechanisms driving cancer and develop more targeted therapies - Seminal Studies: Around the year 2000, studies by Perou et al., Sorlie et al., and Van't Veer et al. were pivotal in advancing the field of transcriptomics in breast cancer. These studies used global gene expression profiling (they analysed the expression of thousands of genes simultaneously) to classify breast cancer into distinct molecular subtypes. Their work led to the identification of different subtypes of breast cancer, such as Luminal A, Luminal B, HER2-enriched, and Basal-like. Each subtype has unique characteristics and responds differently to treatments, which has greatly improved our understanding and management of breast cancer. **3. Proteomic Biomarkers:** these are related to the study of the proteome, which is the entire set of proteins produced or modified by an organism - these biomarkers help in understanding the protein dynamics within cells and tissues, which is crucial for cancer research. - **Protein Expression:** this involves measuring the levels of specific proteins in cells or tissues. By analysing which proteins are present and in what quantities, scientists can gain insights into the biological processes of cancer. For example: - Cancer Biology: Certain proteins may be overexpressed or under expressed in cancer cells compared to normal cells, providing clues about the mechanisms driving cancer growth and progression. - Therapeutic Targets: Identifying proteins that are crucial for cancer cell survival can help in developing targeted therapies. For instance, if a protein is found to be essential for cancer cell growth, drugs can be designed to inhibit that protein. - **Post-Translational Modifications** (PTMs): these are changes that occur to proteins after they have been synthesised. PTMs can significantly alter a protein's function, stability, and interactions. Examples of PTMs include phosphorylation, glycosylation, and ubiquitination. - Function and Role in Cancer: PTMs can affect how proteins behave and interact with other molecules. In cancer, abnormal PTMs can lead to the activation ----------------------------------------------------- --- of oncogenes (genes that promote cancer) or the inactivation of tumour suppressor genes (genes that prevent cancer). **[TYPES OF BIOMARKERS:]** 1. **[GENOMIC]** = DNA sequence changes 2. **[TRANSCRIPTOMIC]** = RNA transcripts 3. **[PROTEOMIC]** = PROTEINS and their modifications **[EXPERIMENT EXPLAINED: (Perou et al. 2000)]** [https://www.nature.com/articles/35021093] What did they do in the experiment? -In the experiment, they performed global gene expression profile analysis/transcriptomics -This study highlights the diversity and complexity of human breast Tumors, focusing on how variations in gene expression contribute to this diversity. Diversity in Tumors: - Breast tumours vary significantly in their development and how they respond to treatments. - Differences in gene expression (transcriptional programs) account for much of the biological diversity seen in human cells and tumours. This means that different tumours can have different sets of active genes, leading to diverse behaviours and responses to treatments. Gene Expression and Cellular Environment: **- Signal Transduction and Regulatory Systems:** These systems are like communication networks within the cell. They transmit information about the cell's identity (what type of cell it is) and its environment (what signals it is receiving from outside the cell). This information helps control which genes are turned on or off. - Gene Expression Control: The expression level of every gene in the genome is regulated by these systems. This regulation influences how cells behave and respond to their surroundings. For example, in response to certain signals, a cell might start producing more of a protein that helps it grow, or it might activate genes that help it repair damage. Study Methodology: - Sample Collection: The study analysed 65 surgical specimens of breast tumours from 42 individuals using complementary DNA microarrays, which can measure the expression of 8,102 human genes. - Each tumour exhibited a unique pattern of gene expression, providing a distinctive molecular portrait. Chemotherapy and Metastasis: - **Pre- and Post-Chemotherapy Samples:** Twenty tumours were sampled before and after a 16-week course of doxorubicin chemotherapy to observe changes in gene expression. The goal was to observe how gene expression in the tumours changed due to the treatment. By comparing the gene expression profiles before and after chemotherapy, scientists can identify which genes are affected by the treatment and how the tumours respond at a molecular level. **- Lymph Node Metastasis:** In addition to the primary tumours, researchers also looked at metastases, which are cancer cells that have spread to the lymph nodes. They paired two primary tumours with their corresponding lymph node metastases from the same patients. By comparing the gene expression patterns between the primary tumours and the metastases, researchers can understand how cancer cells change as they spread to new locations. This comparison can reveal important information about the mechanisms of metastasis and potential targets for therapy. 5\. Findings: - **Gene Expression Patterns:** The study found that when comparing gene expression patterns, two samples taken from the same individual (e.g., from different parts of the same tumour or from different times) were more similar to each other than to samples taken from different individuals. This means that the gene expression profile is consistent within an individual -this indicates a high level of consistency in gene expression within an individual = consistency proves reliability of gene expression profiling **[GENE EXPRESSION PATTERNS]** = **these are the specific levels at which various genes are active in a cell or tissue** - each gene's activity is measured by the amount of mRNA it produces, which can be quantified using techniques like microarrays or RNA sequencing - **Co-Expressed Genes:** Sets of genes that were co-expressed (their expression levels rise and fall together) showed variations in mRNA levels related to specific physiological features. This means that certain groups of genes are regulated together (meaning these genes are likely involved in similar biological processes or pathways or that they are controlled by similar regulatory mechanisms), and their expression levels can vary depending on the physiological state of the tissue. The variations in mRNA levels are related to specific physiological states or conditions of the tissue. For example, the expression levels of these genes might change in response to different stages of cell growth, stress, or disease states like cancer. By studying the patterns of co-expressed genes, researchers can gain insights into how genes work together to influence the physiological state of a tissue, and how these patterns change in different conditions, such as in healthy versus cancerous tissues. - **Tumour Subtypes:** The study identified that breast tumours could be classified into distinct subtypes based on their gene expression patterns. This classification revealed significant differences among the subtypes, which has important implications for understanding the biology of breast cancer and for developing targeted therapies  Explanation of the Figure (Perou et al.) The figure from Perou et al. illustrates the use of gene expression profiling to classify breast tumours based on their molecular characteristics. Overview **-Selection of Genes:** The figure focuses on 100 genes out of the approximately 90,000 genes in the transcriptional genome. These 100 genes were chosen because they are the most informative for clustering tumours based on their gene expression profiles. **-Tumour Representation:** Each column represents an individual tumour sample, and each row represents a single gene. Clustering Algorithm -**Unbiased Grouping**: An unbiased mathematical algorithm was used to group the tumour samples based on similarities in their gene expression profiles. -**Gene Expression Patterns**: Tumors with similar gene expression patterns are clustered together. For example: - **C: Upregulated**: This means that in the cluster labeled "C," certain genes are expressed at higher levels compared to a baseline. - **D: Downregulated**: In the cluster labeled "D," certain genes are expressed at lower levels compared to a baseline. - **E: Downregulated**: Similar to cluster "D," genes in cluster "E" are also expressed at lower levels. - **F: Upregulated**: In cluster "F," certain genes are expressed at higher levels. - **G: Upregulated**: Similarly, in cluster "G," certain genes are expressed at higher levels. Clustering Results **-Mathematical Clustering:** The algorithm clusters the tumours purely based on their global expression profiles, particularly focusing on the 100 most informative genes. **-Subtype Identification:** The clusters correspond closely to known breast cancer subtypes (HER2+, ER+, and triplenegative), although the researchers used different names based on the gene expression profiles rather than receptor status alone. Data Presentation The data is presented in a matrix format where: **o Rows**: Represent individual genes. - **Columns**: Represent experimental samples (tumours). **Colour Coding**: Indicates the ratio of transcript abundance relative to the median: - **Green**: Transcript levels below the median. o **Black**: Transcript levels equal to the median. o **Red**: Transcript levels above the median**.** - **Grey**: Technically inadequate or missing data. **Colour Saturation**: Reflects the magnitude of the ratio relative --------------------------------------------------------------------- -- to the median. Dendrogram and Clusters **-DENDROGRAM** = a tree-like diagram used to illustrate the arrangement of clusters produced by hierarchical clustering. It shows the relationships between different samples based on their similarities or differences in gene expression patterns - Therefore, a dendrogram visually represents the similarities in gene expression patterns between samples. The closer two samples are on the dendrogram, the more similar their gene expression profiles are. Explanation of the Figure Legend - This figure legend describes the hierarchical clustering of breast cancer samples based on --------------------------------------------------------------- -------------------------------------- gene expression profiles , highlighting the identification of distinct subgroups within the disease. Dendrogram Structure: **-Two Large Branches**: The dendrogram shows two main branches, each containing smaller branches that represent groups of tumours with common biological themes. **-Branch Colours**: Different colours are used to indicate specific subtypes: Cluster Identification: **-Matched Pairs**: Small black bars beneath the dendrogram indicate 17 pairs of samples that were matched by the hierarchical clustering. **-Unmatched Pairs**: Larger green bars show the positions of three pairs that were not matched by the clustering. **-Subclusters**: Within larger groups (e.g., luminal A subtype in the ER+ group), there are subclusters that share specific gene expression profiles. These subclusters can reveal additional molecular drivers of the disease or potential therapeutic targets. Significance **-Stratification**: The ability to stratify breast cancer into subgroups based on gene expression profiles allows for more precise classification and treatment. **-Therapeutic Targets**: Identifying genes with different expression levels within subclusters can lead to the discovery of new molecular drivers and therapeutic targets. **-Scientific Impact**: This approach was revolutionary, as it provided a deeper understanding of the molecular basis of breast cancer, leading to more targeted and effective treatments. **[SUBDIVISION OF BREAST CANCER BY GENE EXPRESSION]** Identification of Gene Networks and Pathways: **-Existing and New Networks**: Gene expression profiles help identify both known and novel gene networks and signalling pathways involved in breast cancer. **-Implications**: Understanding these networks and pathways is crucial for developing targeted therapies and improving treatment outcomes. Subclusters within Groups 1. **Hierarchical Tree**: **-Subclusters**: Within larger groups (e.g., luminal A subtype in the ER+ group), there are smaller subclusters. These subclusters share specific gene expression profiles that are distinct from other samples within the same larger group. **-Stratification**: This further stratification into subcompartments helps in understanding the heterogeneity within each subtype. 2\. **Molecular Drivers and Therapeutic Targets**: **-Gene Expression Profiles**: By examining the gene expression profiles within these subclusters, researchers can identify genes with different expression levels. **-Potential Drivers**: Some of these genes may be additional molecular drivers of the disease = meaning they might be key players in the development and progression of the disease. **-Therapeutic Targets**: These genes could also serve as new targets for therapy, providing more personalized treatment options. Scientific Impact: **-Advantage**: The ability to stratify breast cancer into more precise subgroups based on gene expression was a significant advancement. It allowed scientists to better understand the molecular basis of the disease and identify new therapeutic targets. **-Excitement**: This approach generated a lot of excitement in **[PIE CHART EXPLANATION]** 1. **[Classical Histology (Top Pie Chart):]** **-Pink (60% to 70%):** This large portion represents the \"ER+ve group\", meaning these samples are oestrogen receptor-positive (ER+). Classical histology, such as immunohistochemistry, identifies this based on the presence of oestrogen receptors on the protein level in tissue samples. **-Blue (25% to 30%):** This portion represents another subgroup, possibly based on the level of oestrogen receptor expression or another histological feature. **-Yellow (10% to 15%):** This could indicate a smaller subset of the population with lower ER expression or a different histological marker. 2. **[Transcriptomes (Bottom Pie Chart):]** This pie chart represents the classification of the same samples based on molecular analysis (i.e., transcriptomics, which measures RNA expression to classify tissues). **-Blue (44%):** Represents the Luminal A subtype, which is typically ER+ and has a better prognosis. **-Orange (24%):** Represents the Luminal B subtype, also ER+, but usually more aggressive than Luminal A. **-Green (19%):** Represents the Basal-like subtype, often triplenegative breast cancer (lacking ER, PR, and HER2), which tends to be more aggressive. **-Purple (11%):** Represents HER2-enriched, a group characterized by high HER2 expression. **-Red (2%):** Represents Normal-like, a group that resembles normal breast tissue. **[GENE EXPRESSION PROFILING AS A PROGNOSTIC INDICATOR]** **[OF SURVIVAL]** accurate predictions of survival and more tailored clinical ------------------------------------------------------------- -- decisions. **-Molecular Targets**: This approach also helps identify specific molecular targets for therapy, improving treatment strategies. Data and Criticism **Initial Excitement**: **-Small Sample Size**: The initial studies that generated excitement about GEP were based on very small sample sizes (about 11 patients per group). **-Criticism**: Over the next decade, these studies were criticized for being poorly powered and not representative of larger populations. **Subsequent Studies**: **-Larger Datasets**: Later studies with larger patient cohorts showed that the survival curves began to converge, reducing ------------------------------------------------------------- ---------- the apparent differences between groups initially observed. **-Re-evaluation**: This led to a re-evaluation of the initial findings, highlighting the need for more robust and well-powered studies to validate the effectiveness of GEP as a prognostic tool. 1\. MammaPrint **Technology**: 70 gene = ----------------------------------------------------------- -- --- -- researchers to study the expression of many genes at once , it involves a small chip containing DNA sequences that can hybridize with the genes of interest. **Prognostic Value**: Assesses the risk of 10-year **metastasis** = the spread of cancer cells from the primary site to other parts of the body. **-High Risk**: 44% disease-free survival (DFS = the length of time after treatment during which a patient survives without any signs of the disease), means 44% of patients are expected to remain disease-free for 10 years. **-Low Risk**: 87% DFS. **FDA Approval**: Approved for its prognostic value in assessing the progression to metastasis. 2\. Oncotype DX **Technology**: 21 gene **quantitative RT-PCR array** = a laboratory technique that measures the amount of specific RNA, it is used to quantify gene expression levels. **-Genes Included**: 16 cancer-related genes (ER, HER2, proliferation (rapid multiplication of cancer cells), invasion (the ability of cancer cells to spread into surrounding tissues). **Prognostic Value**: Assesses the risk of recurrence. **-Low Risk**: Less than 12%. **-Medium Risk**: 12-21%. **-High Risk**: 21-33%. **Predictive Value**: Predicts the likelihood of response to chemotherapy, helping to avoid unnecessary treatment for those unlikely to benefit. 3. PAM50 **Technology**: 50 gene quantitative RT-PCR. **Prognostic Value**: Predicts recurrence in both ER-negative and ER-positive patients. **Predictive Value**: Helps in deciding on chemotherapy and predicts response to tamoxifen (TAM = a medication used to treat and prevent breast cancer by interfering with the activity of oestrogen in the breast tissue) in ER-positive patients. 4. Theros-Breast Cancer Index **Prognostic Value**: Assesses the risk of recurrence in tamoxifen- treated patients. --------------------- -- **Genes Included**: (homeobox (a group of genes that play a role in regulating the development of anatomical structures), IL17 (Interleukin 17 -- a cytokine that plays a role in inflammation and the immune response), unknown transcript (gene or RNA sequence that has not yet been fully characterized or identified) **Clinical Use**: Better than ER/PR status for predicting recurrence and used for treatment decisions on alternative aromatase inhibitors (AIs). **[QUANTITATIVE RT-PCR ARRAY]** = uses the expression levels of specific genes to assess the risk of breast cancer recurrence. The expression levels of these genes correlate with cancer outcomes How does Quantitative RT-PCR work? 1\. **Sample Collection**: \- A sample of the patient's tumour tissue is collected, usually from a biopsy or surgery. 2. **RNA Extraction**: -RNA is extracted from the tumour cells in the sample. RNA carries the genetic information needed to produce proteins, and its levels can indicate how active certain genes are. 3. **Quantitative RT-PCR**: -The extracted RNA is then analysed using quantitative RT-PCR (Reverse Transcription Polymerase Chain Reaction). This technique converts RNA into complementary DNA (cDNA) and amplifies it to measure the expression levels of specific genes. -In this case, the three genes of interest (homeobox, IL17, and the unknown transcript) are targeted. 4. **Gene Expression Measurement**: -The expression levels of these three genes are quantified. This means determining how much RNA from each gene is present in the sample, which reflects how active these genes are in the tumour cells. 5. **Risk Assessment**: -The measured expression levels are compared to established thresholds or patterns that have been correlated with cancer outcomes in previous studies. -Based on these comparisons, the assay can predict the likelihood of cancer recurrence. For example, certain expression patterns might be associated with a higher risk of the cancer returning, while others might indicate a lower risk. 6. **Clinical Decision-Making**: -The results of the gene expression analysis are used by doctors to make more informed treatment decisions. For instance, if the assay indicates a high risk of recurrence, a doctor might recommend switching from tamoxifen to an alternative treatment. Context and Impact **Research and Commercialization**: The development of these assays marked a significant advancement in breast cancer diagnostics and treatment. They simplified the analysis by focusing on a smaller set of highly informative genes. **Clinical Application**: These assays help stratify patients based on their gene expression profiles, leading to more personalized treatment plans. Summary **- MammaPrint**: Useful for predicting metastasis risk. **-Oncotype DX**: Helps determine the need for chemotherapy. **-PAM50**: Assists in predicting tamoxifen response and recurrence. **-Theros-BC Index**: Focuses on recurrence risk in tamoxifentreated patients. **[BIOMARKERS PANELS: MAIN ADVANTAGES AND]** **[APPLICATIONS]** What are the advantages? **Reducing Overtreatment**: Biomarker panels have been instrumental in reducing overtreatment, particularly in cancer therapy. For instance, in the 2010s, these panels helped identify ER+ breast cancer patients who were unlikely to respond to certain treatments, thereby sparing them from unnecessary therapies. -**Personalized Treatment:** Tools like MammaPrint have shown that they could have altered the treatment plans for about 34% of patients if used before deciding on a treatment. This highlights the potential of biomarker panels in tailoring treatments to individual patients, improving outcomes and reducing side effects. **-Improved Diagnosis and Prognosis**: Biomarker panels provide a more detailed picture of disease states, aiding in more accurate diagnoses and prognoses. This is particularly valuable in complex diseases like cancer, where a single biomarker might not provide sufficient information. What are the challenges? - **Variability in Clinical Practice:** Not all clinics have the same resources or multi-disciplinary teams, leading to inconsistencies in how biomarker panel results are interpreted and used. This can result in different treatment recommendations for similar patients. - **Sample Collection and Processing:** The way samples are collected and processed can vary significantly between clinics, affecting the reliability and comparability of results. **- Complexity in Interpretation:** The presence of multiple therapeutic strategies and the complexity of interpreting biomarker data can make clinical decision-making more challenging. This requires advanced data analysis tools and standardized protocols to ensure consistent and accurate use of biomarker panels. **[MOLECULAR GENETICS DRIVES PERSONALISED MEDICINE: KEY]** **[OBJECTIVES]** **Molecular genetics** plays a crucial role in personalized medicine by tailoring medical treatment to the individual characteristics of each patient. Here are the key objectives: 1. Prevention - **Early Intervention**: Molecular genetics allows for the forecasting of disease outcomes before symptoms develop. For example, identifying mutations in the BRCA1 gene can lead to early interventions such as regular mammography, prophylactic surgery, or chemoprevention to reduce the risk of breast cancer. 2. Prediction - **Avoiding Adverse Effects:** Genetic analysis can determine whether a patient needs chemotherapy, thereby avoiding unnecessary treatments and their associated side effects. - **Instructing Treatment:** It helps in assessing the risk of developing metastasis, guiding the choice of treatment paths. - **Selecting Optimal Response**: Genetic information can be used to select the most effective type of chemotherapy based on which genes are activated. 3. Participation **-Increased Compliance:** Patients are more likely to comply with treatments that are specifically tailored to their genetic makeup, as these treatments are perceived as more effective and personalized. **[NEW TECHNOLOGIES: NEXT GENERATION SEQUENCING (NGS)]** **Next Generation Sequencing (NGS)** is a revolutionary --------------------------------------------------------------- -- technology that has significantly advanced genomic profiling. Key Features of NGS **1. Comprehensive Genomic Profiling:** ------------------------------------------------------------ -- sequencing of the complete genome, including all mutations. This includes spotting single nucleotide changes, small insertions, and deletions, and large deletions. 2. **Detailed Mutation Identification:** Important mutations that can be identified through NGS include those that are crucial for understanding disease mechanisms and developing targeted therapies. 3. **Enhanced Subtype Identification:** When NGS was applied to cancer panels back in 2012, it provided much better separation within Kaplan-Meier survival curves. This allowed for the identification of more than four subtypes of cancer, and current analyses have now split these into at least 10 different subtypes based on molecular characteristics. 4. **Integration with Transcription Profiling:** Combining NGS with transcription profiling enhances the power of prognostic indicators for each subtype. This integrated approach provides a comprehensive view of the disease, aiding in more accurate prognosis and treatment planning.  Applications and Benefits - **Prognostic Power**: For example, patients with a specific subtype of disease **(indicated as burgundy)** have a much poorer prognosis, with more than half the patients expected to die within 50 months. In contrast, other patients **(indicated as pink)** have a much lower risk of death, not reaching 50% risk even after 200 months. This detailed prognostic information helps in tailoring treatment plans more effectively. - **Identifying High-Risk Patients:** NGS helps in identifying heterogeneity within diseases, allowing for the identification of patients who are most at risk. This enables more personalized and effective treatment strategies. - **Improved Treatment Decisions:** By understanding the molecular basis of different subtypes, clinicians can make more informed decisions about the best treatment options for each patient, potentially improving outcomes and reducing unnecessary treatments. **EXPERIMENT EXPLAINED:** https://www.nature.com/articles/nature10983\#MOESM264 - **Kaplan--Meier Plot**: This type of plot estimates survival probabilities over time for distinct groups. In this context, it's showing the probability that individuals in each subgroup remain disease-free (alive without disease progression) over time in months. **-Subgroups (IntClust)**: Each coloured line represents a different \"integrative cluster\" or subgroup labelled with unique identifiers (e.g., IntClust 1, IntClust 2, etc.). These clusters likely represent different patient groups, stratified based on specific molecular or clinical characteristics. **-Survival Differences**: The downward trend of each line shows the proportion of patients surviving disease-free at each time point. Subgroups with lines that drop steeply (e.g., red for IntClust 7) indicate poorer survival outcomes, meaning these patients have higher disease-specific mortality rates. In contrast, subgroups with more gradual declines (e.g., blue for IntClust 4) show better survival outcomes. **-Log-rank Test**: The top of the plot indicates a log-rank P-value (P = 1.2 × 10⁻¹⁴), suggesting a statistically significant difference in survival rates between the subgroups. A very small P-value like this implies that the differences in survival between clusters are highly unlikely to be due to random chance. **-Sample Size and Deaths**: The legend shows, for each cluster, the total number of patients (\"at risk\") in that group and the ------------------------------------------------------------------ ------------------------------ number of deaths in parentheses. This provides context on how large each subgroup is and how many patients had diseasespecific mortality. **[Analysis of these additional genes has helped to identify] [key *oncogenic drivers*, and *disease modifiers*, that reclassify] [disease subtypes and provide a molecular mechanism for] [disease]**  Analysis of Additional Genes in Breast Cancer **- Identification of Oncogenic** **Drivers and Disease Modifiers**: The analysis of additional genes has been crucial in identifying ------------------------------------------------------------------ ------------------- key oncogenic drivers and disease modifiers. These discoveries help reclassify disease subtypes and provide a molecular mechanism for disease progression. **- Reclassification of Disease Subtypes:** For example, focusing on triple-negative breast cancer (TNBC), which constitutes about 20% of the total breast cancer population, molecular profiling has further divided this group into around 4 to 6 definitive subtypes. This detailed classification is essential for tailoring specific treatment strategies for each subtype. **- Targetable Oncogenic Drivers**: In HER2-positive (HER2+) and oestrogen receptor-negative (ER-) breast cancers, additional genes such as the ones below been identified as key drivers. ***BRCA1**: A gene that, when mutated, significantly increases the risk of breast and ovarian cancers.* ***FGFR2 (Fibroblast Growth Factor Receptor 2)**: A gene involved in cell growth and division. Mutations can lead to uncontrolled cell growth.* ***PTEN (Phosphatase and Tensin Homolog)**: A tumour suppressor gene. Loss of PTEN function can lead to increased cell survival and proliferation.* ***EGFR (Epidermal Growth Factor Receptor)**: A gene that, when overexpressed or mutated, can promote the growth of cancer cells.* These genes are targetable, meaning that specific therapies can ----------------------------------------------------------------- -- be developed to inhibit their activity. **- Pharmaceutical Developments:** Companies like AstraZeneca and others are actively designing drugs to target these individual genes. This approach aims to improve treatment efficacy and patient outcomes by focusing on the specific molecular characteristics of each cancer subtype. **[Summary of the main subtypes based on their expression] [profiling and the genes within those subgroups now that are] [re-stratifying and our new molecular targets]** This image shows a summary of the main molecular subtypes of breast cancer, each defined by specific expression profiles and molecular markers. 1. **Luminal A** Wnt/β-catenin: A signalling pathway involved in cell growth and development. Abnormal activation can lead to cancer. Glu Metabolism: Refers to glucose metabolism, which is how cells process sugar for energy. Cancer cells often have altered glucose metabolism. VEGF (Vascular Endothelial Growth Factor): A protein that promotes the growth of new blood vessels, which tumors need to grow. IGF-1 (Insulin-like Growth Factor 1): A hormone that can promote cell growth and survival. High levels can be associated with cancer. 2. **Luminal B** p38 MAPK: A signalling pathway that responds to stress and inflammation, playing a role in cell differentiation and apoptosis (cell death). Sonic Hedgehog: A pathway important in embryonic development that can also contribute to cancer when abnormally activated. Notch: A signalling pathway involved in cell differentiation. Dysregulation can lead to cancer. G1/S Checkpoint: A control mechanism in the cell cycle that ensures cells are ready to replicate DNA. Dysregulation can lead to uncontrolled cell division. Sterol Biosynthesis: The process by which cells produce sterols, important components of cell membranes. Alterations can affect cell growth. TGF-β (Transforming Growth Factor Beta): A protein that regulates cell growth and differentiation. It can suppress or promote cancer depending on the context. FGF (Fibroblast Growth Factor): A protein involved in cell growth, differentiation, and repair. It can contribute to cancer progression. **3. ERBB2 (HER2-enriched)** G1/S Checkpoint: As mentioned above, this checkpoint controls cell cycle progression. T-cell Receptor: Part of the immune system that helps recognize and respond to pathogens. Its role in cancer is complex and involves immune evasion. NF-κB (Nuclear Factor kappa-light-chain-enhancer of activated B cells): A protein complex that controls DNA transcription and cell survival. It is often activated in cancer. B-cell Receptor: Another component of the immune system, involved in recognizing antigens. Its signalling can be altered in cancer. Death Receptor: Receptors that can trigger apoptosis. Cancer cells often evade death receptor signalling to survive. **4. Basal-like** Wnt/β-catenin: As mentioned, this pathway is involved in cell growth and development. G1/S Checkpoint: As mentioned, this checkpoint controls cell cycle progression. Gly, Ser, and Thr Metabolism: Refers to the metabolism of amino acids glycine, serine, and threonine, which are important for cell growth and proliferation. Estrogen Receptor: A protein that binds oestrogen and can promote the growth of some breast cancers. Basal-like cancers typically lack this receptor. PPAR (Peroxisome Proliferator-Activated Receptor): A group of nuclear receptor proteins that regulate gene expression. They play roles in metabolism and inflammation. **5. Normal-like** JAK/Stat (Janus Kinase/Signal Transducer and Activator of Transcription): A pathway involved in transmitting signals from cytokines and growth factors to the cell nucleus, influencing cell growth and survival. PDGF (Platelet-Derived Growth Factor): A protein that regulates cell growth and division. It can contribute to cancer when overexpressed. EGF (Epidermal Growth Factor): A protein that stimulates cell growth and differentiation. Overexpression can lead to cancer. PPAR: As mentioned, these receptors regulate gene expression related to metabolism and inflammation. IGF-1: As mentioned, this hormone promotes cell growth and survival. IL-4 and IL-6 (Interleukins 4 and 6): Cytokines involved in immune responses. They can promote inflammation and have roles in cancer progression. **[SUMMARY OF LECTURE 1 -- REVIEW PAPER]** **[EXTRA READING:] https://www.wjgnet.com/22184333/full/v5/i3/412.htm** **[Biological subtypes of breast cancer: Prognostic and] [therapeutic implications]** Breast Cancer: A Heterogeneous Disease Breast cancer is not a single disease but a heterogeneous complex of diseases, each with distinct biological features. This heterogeneity leads to differences in treatment responses, modalities, and clinical outcomes. Traditional Classification Limitations - **Traditional Markers**: Tumour size, lymph node involvement, histological grade, age, and the status of oestrogen receptor (ER), progesterone receptor (PR), and human epidermal growth factor receptor 2 (HER2) are commonly used to classify breast cancers. - **Limitations:** These traditional markers can be limited in their ability to tailor treatment strategies because tumours with similar clinical and pathological presentations can behave very differently. Molecular Techniques and Their Impact **- Emerging Techniques:** New molecular techniques, such as gene expression profiling and next-generation sequencing, are providing more accurate methods for analysing breast cancer. **- Prediction of Recurrence:** These techniques enable more precise predictions of recurrence and help classify breast cancer into distinct biological classes based on gene expression patterns. Molecular Classification and Treatment **-Gene Expression Patterns:** There is now evidence that classifying breast cancer based on gene expression patterns can provide insights into patient survival and response to therapy. **-Protein Gene Products:** The behavior and biology of cancer cells, including their response to therapy, can be predicted through gene signatures that identify the protein gene products driving the cancer. **Practical Implications** - **Personalized Treatment:** Understanding the molecular drivers of different breast cancer subtypes allows for more personalized treatment plans. For example, specific subtypes like HER2-positive or triple-negative breast cancers can be further divided based on additional genetic markers, leading to more targeted therapies. **-Pharmaceutical Developments**: Pharmaceutical companies are developing drugs that target these specific genetic markers, improving treatment efficacy and patient outcomes4. In summary, the integration of molecular techniques in breast cancer analysis is revolutionizing how we classify and treat this disease, leading to more personalized and effective treatment strategies. Introduction **-Heterogeneous Disease**: Breast cancer is a complex disease with many subtypes, each having distinct biological features. **-Differences in Treatment and Outcomes**: These biological differences lead to varied responses to treatment and different clinical outcomes. **-Traditional Classification Limitations**: Traditional methods (tumor size, lymph node involvement, histological grade, age, ER, PR, HER2 status) may not fully capture the complexity needed for personalized treatment. **-Histological Appearance**: Similar clinical and pathological presentations can mask underlying genetic differences and biological events. **-Need for Detailed Biological Characteristics**: Recent studies emphasize the importance of detailed biological characteristics for better patient risk stratification and treatment optimization. **-Global Gene Expression Profiling (GEP)**: GEP studies classify breast cancer into distinct biological classes based on gene expression patterns, which correlate with patient survival. **-Impact of Screening Programs**: Population-based screening has shifted the focus to early-stage disease and increased interest in biological prognostic and predictive factors. **-Advances in Molecular Studies**: New molecular techniques are improving the accuracy of recurrence predictions and identifying new therapeutic targets. **-Gene Signatures**: Developed as predictors of therapy response, gene signatures help identify protein gene products that drive cancer biology and clinical behavior. **-Focus on Novel Classification and Predictive Factors**: Current research aims to refine breast cancer classification and identify prognostic and predictive factors to enhance treatment strategies. Identification of breast cancer subtypes by GEP studies **Gene Expression Microarray Studies in Breast Cancer -Identification of Molecular Classes**: Gene expression microarray studies analyse thousands of genes simultaneously to identify distinct molecular tumour classes. **-Perou et al. Study**: - Analysed gene expression in 65 tumour samples from 42 breast cancer patients treated with neoadjuvant doxorubicin. o Used microarrays representing 8102 human genes. Selected 496 genes with significant variation between tumours and minimal variation within paired samples, termed the intrinsic gene subset. o Unsupervised clustering revealed two main branches: ER-positive and ER-negative. **-ER-Positive Group**: Characterized by high expression of genes associated with breast luminal cells, termed the luminal group. **-ER-Negative Group**: Further divided into basal-like, ErbB2positive, and normal-like subclasses. o **Basal-like Tumors**: Express characteristics of breast basal epithelial cells, do not express ER, and show staining with basal keratins. - **ErbB2-Positive Tumors**: High levels of HER2 oncogene expression, low ER expression. **-Four Main Groups Identified**: ER-positive luminal-like, basallike, ErbB2-positive, and normal-like. **-Confirmation in Larger Studies**: Subsequent experiments with more cases confirmed these findings. **Clinical Relevance**: o **Sørlie et al. Study**: Investigated gene expression profiles in 78 breast carcinoma patients. o Showed significant differences in overall survival between subtypes. - Basal-like and ErbB2-positive subtypes had the shortest survival times. - Luminal-like breast cancer subclassified into luminalA, luminal-B, and luminal-C. - Luminal-A subgroup associated with the best **Van't Veer et al. Study**: -Investigated node-negative breast cancer patients. -Identified 231 genes associated with disease outcome, defined by distant metastasis at the 5th year. -Each breast tumor has a unique molecular portrait, leading to an improved molecular taxonomy of the disease. -These studies highlight the importance of molecular profiling in understanding breast cancer subtypes and tailoring treatment strategies accordingly. Subclassification of luminal like breast cancer **General Information** **-75% of breast cancers** are positive for estrogen receptor (ER) and/or progesterone receptor (PR). -ER-positive tumors express ER, PR, ER-responsive genes, and other genes typical of luminal epithelial cells, termed the **luminal group**. -Characterization of luminal-like breast cancer varies due to different intrinsic gene sets used in studies. -Hu et al. identified two main luminal-like subclasses: **luminal-A** and **luminal-B**. Luminal-A Subtype **-Most common subtype**: Represents 50%-60% of all breast cancers. **-Characteristics**: - Low histological grade, low nuclear pleomorphism, low mitotic activity. - Includes special histological types (tubular, invasive cribriform, mucinous, lobular). o Higher levels of ER, lower levels of proliferationrelated genes. - Expression of luminal epithelial cytokeratins (CK) 8 and 18, and other markers like ER1, LIV1, FOXA1, XBP1, GATA3, BCL2, erbB3, and erbB4. **-Definition**: ER-positive and/or PR-positive, HER2-negative, low Ki67 index. **-Prognosis**: Good prognosis, lower relapse rate, common recurrence in bone, treatment mainly hormonal therapy. Luminal-B Subtype **-Prevalence**: Comprises 15%-20% of breast cancers. **-Characteristics**: - More aggressive phenotype, higher histological grade, higher proliferative index. - Higher recurrence rate, lower survival rates after relapse. - Increased expression of proliferation-related genes (v-MYB, GGH, LAPTMB4, NSEP1, CCNE1) and growth receptor signalling genes. - 30% of HER2-positive tumours are luminal-B. **-Definition**: ER-positive, HER2-negative, high Ki67 or ER and HER2-positive. **-Prognosis**: Poorer outcomes with hormonotherapy, higher relapse rates in the first 5 years, better response to neoadjuvant chemotherapy. HER2-POSITIVE \- Member of the family of four membrane tyrosine kinases. -Encoded by the HER2 gene, a proto-oncogene on chromosome 17q21. -Activated through heterodimerization or homodimerization, leading to downstream signalling. **Characteristics of HER2-Positive Cancer -Prevalence**: Accounts for 15-20% of breast cancer subtypes. **-Biological and Clinical Behavior**: More aggressive. **-Gene Expression**: High expression of HER2 gene and associated genes in the HER2 pathway. **-Morphology**: Highly proliferative, 75% have high histological and nuclear grade, over 40% have p53 mutations. **-ER Status**: Nearly half are ER-positive but generally express lower ER levels. **Immunohistochemical Profile** **-ER-Negative and HER2-Positive**: Does not perfectly match intrinsic subtype; only 70% of HER2 tumors by microarray show protein overexpression on immunohistochemistry. **-HER2 Amplification**: Not all tumors with HER2 amplification or overexpression are included in the HER2 cluster by microarray analysis. **Prognosis and Treatment** **-Prognosis**: Poor without treatment. **-Sensitivity**: Increased sensitivity to cytotoxic agents like doxorubicin, relative resistance to hormonal agents. **-Metastasis**: Propensity to metastasize to the brain and visceral organs. **-Doxorubicin Sensitivity**: Possibly due to coamplification of the topoisomerase-2 gene near the HER2 locus. **Advances in Treatment** **-HER Directed Therapies**: Development of a large spectrum of therapies targeting HER2. **Subtypes and Survival** **-Subtypes**: Three separate subtypes identified, one with poor prognosis (12% 10-year survival) compared to others (50-55% survival). **-HDPP Gene Analysis**: Prognostic predictor gene analysis related to immune response to tumor invasion and metastasis. BASAL-LIKE **-Prevalence**: Represents 8% to 37% of all breast cancers, depending on the study population. **-Histological Features**: - High histological and nuclear grade. - Poor tubule formation. o Presence of central necrotic or fibrotic zones. - Pushing borders and conspicuous lymphocytic infiltrate. - Medullary features with high mitotic and proliferative indices. **-Tumour Characteristics**: o Mostly infiltrating ductal tumours with a solid growth pattern. - Aggressive clinical behavior with a high rate of metastasis to the brain and lung. **-Marker Expression**: - High levels of basal myoepithelial markers (CK5, CK14, CK17, laminin). - Do not express ER, PR, and HER2 (triple-negative). - Overexpress P-cadherin, fascin, caveolins 1 and 2, alpha-beta crystallin, and EGFR. - Frequent mutations in TP53, genomic instability, and inactivation of the Rb pathway. - Deregulated integrin expression contributing to aggressive behavior. **-Triple-Negative vs. Basal-like**: - Triple-negative refers to lack of ER, PR, and HER2 expression (immunohistochemical classification). - Basal-like is defined via gene expression microarray analysis. - Approximately 20%-30% discordance between triple-negative and basal-like classifications. **-Biomarkers**: - No international consensus on complementary biomarkers for basal-like cancers. - Several genes implicated in proliferation, survival, migration, and invasion. - Commonly deregulated pathways: MAPK, PI3K, Akt, NF-κB. - Beta catenin accumulation observed, potential therapeutic target. **-BRCA1 Related Cancers**: o Basal-like subtype constitutes about three-quarters of BRCA1-related breast cancers. - BRCA1 gene located on chromosome 17, involved in DNA damage sensing and repair. - BRCA1-related cancers often triple-negative, positive for Ki67, basal cytokeratins, TP53, EGFR, Pcadherin, and X chromosome abnormalities. - Similar outcomes for basal-like and BRCA1-related cancers, especially for early relapse and metastatic patterns. - Potential response to PARP inhibitors due to DNA repair defects. **-Therapeutic Targets**: o EGFR overexpression in basal-like cancer, potential target. - Notch pathway identified as a resistance mechanism to EGFR inhibition. - Dual pathway inhibition may be a viable strategy. **-Claudin-Low Subtype**: - Characterized by low expression of tight junction and cell-cell adhesion genes (claudins 3, 4, 7, occludin, E-cadherin).