Quality Assurance - Clinical Chemistry PDF
Document Details
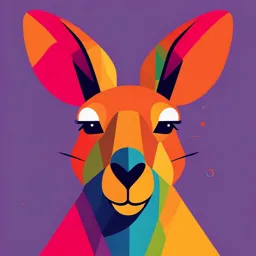
Uploaded by TruthfulMusicalSaw
Conestoga College
Tags
Related
- A Direct Comparison of Patient-Based Real-Time Quality Control Techniques PDF
- MLS1004SEF Clinical Chemistry & Immunonology Lecture 1 PDF
- Module 5 Quality Control PDF
- BNUR 2003 Biomedical Chemistry & Lab Diagnostics Student Notes PDF
- Unit 3.2 Nature of Clinical Laboratory PDF
- MTPLMSP111 Principles Of Medical Laboratory Science PDF
Summary
This document is a lecture presentation on quality assurance in clinical chemistry, covering topics such as descriptive statistics, accuracy/precision, and quality control.
Full Transcript
Quality Assurance - 1 CLINICAL CHEMISTRY: PRINCIPLES, TECHNIQUES, AND CORREL ATIONS (8TH ED.) - CHAPTER 3 Descriptive Statistics - Basic Terms Qualitative - Subjective observations that cannot be quantitated - Ex. Turbidity, color, odor, etc. Quantitative - Measurable characteristics - Ex....
Quality Assurance - 1 CLINICAL CHEMISTRY: PRINCIPLES, TECHNIQUES, AND CORREL ATIONS (8TH ED.) - CHAPTER 3 Descriptive Statistics - Basic Terms Qualitative - Subjective observations that cannot be quantitated - Ex. Turbidity, color, odor, etc. Quantitative - Measurable characteristics - Ex. Glucose (mmol/L), creatinine (μmol/L) Data - Collection of related observations which can be used to arrive at some conclusion about people in a population. - Ex. Reference intervals for healthy people Accuracy and Precision Accuracy - The closeness of a result to the “true value” Precision - The reproducibility of a result In the lab we want results that are both accurate AND precise. Key Terminology Population - All possible observations Sample - Part of a population Random Sample - Sampling so that no part of a population is given preference Parameter - Calculations using population information Calculated Statistical Values Statistics - Values calculated from random samplings of the population Classed into two groups: Measures of Central Tendency Distribution of values around a central value Mean, median, mode Measures of Dispersion How spread out the values are Range, variance, standard deviation Measures of Central Tendency - Mean Mathematical average of a set of values Parametric mean (u) = data based on all of a population Statistical mean = () = data based on a sample of population Mean - Example Example: Find the mean of the following patient glucose results in mmol/L. Patient Result (mmol/L) 1 5 2 3 3 6 4 5 5 10 6 7 Measures of Central Tendency - Median Number in middle when values are arranged in sequential order If an even number of values use average of 2 middle numbers If odd number of values use middle number Median - Example Example: Find the median of the following patient glucose results in mmol/L. Patient Result 1st - Arrange #’s in (mmol/L) sequential order. 1 5 2 3 3 5 5 6 7 10 3 6 5+6 11 = = 5.5 4 5 2 2 5 10 6 7 Measures of Central Tendency - Mode Arrange data in order Most commonly occurring number in a group of numbers Mode - Example Example: Find the mode of the following patient glucose results in mmol/L. Patient Result 1st - Arrange #’s in (mmol/L) sequential order. 1 5 2 3 3 5 5 6 7 10 3 6 4 5 The mode is the most commonly occurring #, which in this case is 5 10 5. 6 7 Measures of Dispersion - Range Measure of Dispersion Difference between highest and lowest value Range - Example Example: Find the range of the following patient glucose results in mmol/L. Patient Result 1st - Arrange #’s in (mmol/L) sequential order. 1 5 2 3 3 5 5 6 7 10 3 6 4 5 The range is the difference between the highest value and 5 10 the lowest value, therefore: 6 7 10 - 3 = 7 Measures of Dispersion - Variance Indicator of the precision of a group of numbers Symbol: s2 Indicates how close together, or how precise, the numbers within a group are A group of numbers with a large variance would be expected to have a wide range of values. A group of numbers with a small variance would be expected to have numbers that are very close in value. The smaller the variance of a group of numbers, the more precise they are. Variance - Formula 𝒔 𝟐 = ∑ ( 𝑥 − 𝑥 )2 𝑛−1 Where: = variance ∑ the sum of the #’s within the brackets x = an individual data point within the group the mean of the group of #’s n = the total amount of #’s in the group Variance - Example Example: Find the variance of the following patient glucose results in mmol/L. Patient Result 1st - Arrange #’s in sequential (mmol/L) order. 1 5 2 3 3 5 5 6 7 10 3 6 Next, subtract the mean from 4 5 each of the #’s 5 10 6 7 Variance - Example Example: Find the variance of the following patient glucose results in mmol/L. Next, subtract the mean Next, square the from each of the #’s difference: Glucose (-) ( - )2 Result 3 - 6 = -3 (-3)2 = 9 3 5 - 6 = -1 (-1)2 = 1 5 5 - 6 = -1 (-1)2 = 1 5 6-6=0 (0)2 = 0 6 7-6=1 (1)2 = 1 7 10 - 6 = 4 (4)2 = 16 10 Variance - Example Example: Find the variance of the following patient glucose results in mmol/L. ( - )2 Next, add all the #’s from the last step: (-3) = 9 2 ∑( - ) 2 = (9 + 1 + 1 + 0 + 1 + 16) = 28 (-1) = 1 2 (-1)2 = 1 Then, divide by (n - 1) to find the variance: (0)2 = 0 (1)2 = 1 = (4) = 16 2 Measure of Dispersion - Standard Deviation The most frequently used measure of precision Symbol: s (or SD) Square root of the variance The formula for the standard deviation (SD) √ ∑ ( 𝑥 − 𝑥) 2 is: 𝑠= 𝑛−1 Standard Deviation - Example Example: Find the standard deviation of the patient glucose results from the previous example. = = = 2.37 Coefficient of Variation Used when comparing two or more groups of data to determine which has the greatest precision (eg. reproducibility or precision of different methods) Expressed as a % The lower the CV, the more precise the data Coefficient of Variation - Example Example: Find the CV of the patient glucose results from the previous example. s= 2.37 =6 = x 100% = 0.395 x 100% = 39.5% Probabilities Associated with Standard Deviation Statistically, if a sample is analyzed 30 times and the mean and SD established for the results obtained: 68.2% of the time, the results will fall within ± 1 SD from the mean. 95.5% of the time, the results will fall within ±2 SD from the mean. 99.7% of the time, the results will fall within ±3 SD from the mean. Normal Distribution Curve Example 1 In a normal distribution curve what percentage of results would be beyond ± 2SD? +2SD = 2.5% -2SD = 2.5% Therefore, 5% of all 34% 34 % results will fall outside of ± 2SD. 13.5 13.5 % % Example 2 In a normal distribution curve what percentage of results would be beyond +1SD? +1SD = 13.5% + 2.5% Therefore, 16% of all results will fall outside of 34% 34 % +1SD. 13.5 13.5 % % Basic Quality Control Concepts Quality Assurance (QA) Process of monitoring any activity that is associated with a lab result This includes any errors that can occur in each of three stages: 1. Pre-analytical Occurs before the sample reaches the lab or is analyzed Ex. Collecting a HbA1c sample in a SST tube 2. Analytical Occurs during or relates to the analysis of a sample Ex. Analyzer malfunction during testing 3. Post-analytical Quality Control (QC) Part of quality assurance (QA) Analyzed along with patient specimens and treated in the same manner Used to detect analytical errors that would cause erroneous patient results. Usually 2 or 3 levels of control are used (low, medium, high) At least 2 levels of QC must be run for every test once every 24 hours Some labs will run their chemistry QC twice a day. Quality Control Material QC should be of the same matrix (i.e same chemical and physical properties) as what you are measuring. If you are analyzing serum samples, use serum-based QC If you are analyzing urine samples, use urine-based QC QC material can be: Lyophilized (freeze-dried) This type requires the material to be diluted prior to use Prediluted, ready-to-use No dilution required. Operation of a QC System A QC system is used in the clinical laboratory to monitor analytical variations. Three stages in this process include: 1. Establishing or verifying allowable statistical limits of variation for each analytic method (means and SDs) 2. Using those limits to evaluate the QC data generated for each test each day. 3. Taking action to fix errors as they occur by: Finding the cause(s) of the error Taking corrective action Reanalyzing control and patient data Establishing Statistical Quality Control Limits When a new lot # of control material is to be put in use, the control material must be first analyzed for 20 days After the 20 days, the mean and standard deviation are determined for each test. Most labs accept a range of the Mean ±2SD as acceptable for QC limits Mean ±2SD = 95% confidence limits QC is plotted on a Levey-Jennings chart and is considered “out of control” if it is outside the confidence limits. Establishing Statistical Quality Control Limits Standard deviation range is the measurement of “dispersion” calculated by: Subtracting the standard deviation value from the mean value to establish the lowest number in the range, then Adding the standard deviation value to the mean value to establish the highest number in the range Established for quality control material by the laboratory as a method of setting acceptable quality control limits. Frequently, 1, 2, and 3 SD ranges are established. Establishing Statistical Quality Control Limits - Example 20 replicates of a single bottle of quality control serum were analyzed for sodium. Mean sodium value = 140 mmol/L. The standard deviation = 3 mmol/L. What would be the 1, 2, and 3 SD ranges, or confidence intervals, for the quality control? Establishing Statistical Quality Control Limits - Example 1 SD range is established as follows: Mean = 140 mmol/L 1 standard deviation = 3 mmol/L 140.0 – 3.0 = 137.0 mmol/L (lower limit) 140.0 + 3.0 = 143.0 mmol/L (upper limit) The 1 SD range is 137−143 mmol/L. All numbers including and between 137 and 143 mmol/L are within 1 SD of the mean. Establishing Statistical Quality Control Limits - Example 2 SD range is established as follows: Mean = 140 mmol/L 1 standard deviation = 3 mmol/L 140.0 – 2(3.0) = 140 - 6 = 134 mmol/L (lower limit) 140.0 + 2(3.0) = 140 + 6 = 146 mmol/L (upper limit) The 2 SD range is 134−146 mmol/L. All numbers including and between 134 and 146 mmol/L are within 2 SD of the mean. Establishing Statistical Quality Control Limits - Example 3 SD range is established as follows: Mean = 140 mmol/L 1 standard deviation = 3 mmol/L 140.0 – 3(3.0) = 140 - 9 = 131 mmol/L (lower limit) 140.0 + 3(3.0) = 140 + 9 = 149 mmol/L (upper limit) The 3 SD range is 131−149 mmol/L. All numbers including and between 131 and 149 mmol/L are within 3 SD of the mean. Quality Control Analysis What is an acceptable limit for my quality control material result?” If the quality control (QC) result is outside of the acceptable limit established by the laboratory, the patient results should not be reported until the problem is solved. No patient results should be reported until the method is “in control.” Types of Errors Out of control: a control result outside of established QC range Two main reasons why a method is out of control: Random error Occurs solely by chance Extreme day-to-day variation in results Can be caused by the instrument, operator, reagent, environmental variation Ex. Power surge, bubble in sample Types of Errors Systematic error All samples affected All values will move in one direction (higher or lower) Two types: Shifts and Trends Ex. Reagents improperly stored Improper calibration Change in lot # of reagent or calibrator Incorrectly prepared reagent or calibrator Deterioration of light source (lamp) Common QC Problems - Shifts Shift Occurs when QC results are all distributed on one side of the mean for 6 or more consecutive days Occurs because of systematic error Cause must be found and corrected because the method is “out of control”. Examples: Incorrectly prepared standard or reagent Change of timing or temperature in a kinetic assay Wrong wavelength Semi-automatic pipette lost calibration Common QC Problems - Shifts Common QC Problems - Trends Trend (or Drift) Occurs when QC results either decrease or increase consistently over a period of 6 or more days Is a type of systematic error Tends to occur more slowly; gradual change Cause must be found and corrected Examples: Deterioration of standard or reagent Gradual decrease in bath temperature Gradual wavelength change Deterioration of the light source (lamp) Common QC Problems - Trends Levey-Jennings Charts In the clinical laboratory, the daily QC is plotted on a Levey- Jennings Chart Shifts and Trends can be easily visualized At least 2 levels of QC material should be analyzed for each test, each day. Levey-Jennings Charts - Example Three levels of control are run daily on a hematology analyzer to measure HGB For the “Normal” control: Mean is 150 g/L SD of 15 g/L Confidence level is mean ± 2 SD 150 - 2(15) = 120 150 + 2(15) = 180 Acceptable control range = 120 - 180 g/L The HGB control results that were obtained on 5 consecutive days are as follows: Day 1 = 130 g/L Day 2 = 125g/L Day 3 = 160 g/L Day 4 = 155 g/L Day 5 = 140 g/L Levey-Jennings Charts - Example Are these control results acceptable? HGB (G/L) Yes - all results fall within ± 2SD of the mean Westgard Multirules Westgard Multirules: set of quality control rules used to interpret quality control results in the clinical laboratory Used to evaluate acceptability of control results If, at any point, a rule, except for the warning rule is violated, the result is rejected and the method may be “out of control.” Westgard 12s Rule The 12s Rule is a warning rule. One point exceeds ±2SD. If no source for the error is found - Indicates acceptable random error (Ex. Air bubble in sample) Westgard 13s Rule The 13s Rule is a rejection rule. One point exceeds ±3SD. Indicates an unacceptable random error or the beginning of a systematic error. Westgard 22s Rule The 22s Rule is a rejection rule. Two points exceeds ±2SD on same side of the mean. Indicates systematic error. Westgard R4s Rule The R4s Rule is a rejection rule. At least a 4SD difference in control values in a single run. Indicates random error. Westgard 41s Rule The 41s Rule is a rejection rule. Four consecutive results are greater than 1SD on the same side of the mean. Indicates systematic error. Westgard 10x Rule The 10x Rule is a rejection rule. 10 control results fall on the same side of the mean. Indicates systematic error.