GEOG312 Parametric and Non-Parametric Test PDF
Document Details
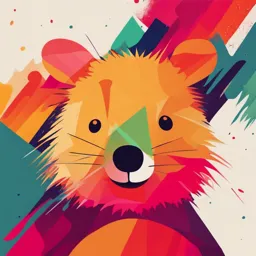
Uploaded by FinestObsidian6088
Tags
Summary
This document appears to be related to GEOG312 and discusses parametric and non-parametric tests. The document is relevant to higher education, specifically at the undergraduate level.
Full Transcript
Today Parametric Tests and Nonparametric Tests Class Activity on Non-Parametric Tests Announcements: Assignment 08 Due next time: Assignment 08 Due next Thursday: Final Project Outline (We will work on these next class and next week as well) Parametric and Non-Parametric...
Today Parametric Tests and Nonparametric Tests Class Activity on Non-Parametric Tests Announcements: Assignment 08 Due next time: Assignment 08 Due next Thursday: Final Project Outline (We will work on these next class and next week as well) Parametric and Non-Parametric Tests Hypothesis Testing Parametric Nonparametric Wilcoxon Rank Kruskal-Wallis Goodness of Fit t-Test ANOVA Sum Test H-Test Chi-squae Many More Tests Exist! Parametric Tests Normality Assumption: Populations are assumed to both follow a normal distribution Data Type Assumption: Interval or ratio data only Variance Assumption: All samples have approximately the same variance Assumptions of Parametric Data All parametric tests have 4 basic assumptions that must be met for the test to be accurate. First assumption: Normally distributed data Normal shape, bell shape, Gaussian shape Transformations can be made to make data suitable for parametric analysis. Assumptions of Parametric Data Frequent departures from normality: Skewness: lack of symmetry of a distribution Skewness < 0 Skewness = 0 Skewness > 0 Kurtosis: measure of the degree of ‘peakedness’ in the distribution The two distributions below have the same variance approximately the same skew, but differ markedly in kurtosis. More peaked distribution: kurtosis > 0 Flatter distribution: kurtosis < 0 Assumptions of Parametric Data Second assumption: Homoscedasticity (Homogeneity in variance) The variance should not change systematically throughout the data Third assumption: Interval data (linearity) The distance between points of the scale should be equal at all parts along the scale. Fourth assumption: Independence Data from different subjects are independent Values corresponding to one subject do not influence the values corresponding to another subject. Important in repeated measures experiments Types of Parametric Tests Examining whether male and female Compares means of two independent t-Test (Independent t-test) students have different average test groups scores Compares means of the same group Measuring weight loss before and after Paired t-Test before and after an intervention a diet plan Compares means across three or more Comparing the average crash time One-way ANOVA groups across different days of the week Examines the effect of two categorical Studying the effect of age and gender Two-way ANOVA independent variables on a continuous on blood pressure levels dependent variable Measures the strength and direction of Checking the relationship between Pearson Correlation the relationship between two continuous study time and exam scores variables Models the relationship between Predicting house prices based on Linear Regression independent and dependent variables square footage What is the t -test A t-test is an inferential statistic used to determine if there is a statistically significant difference between the means of two variables. The t-test is a test used for hypothesis testing in statistics. Calculating a t-test requires the difference between the mean values from each data set, the standard deviation of each group, and the number of data values. T-tests can be dependent or independent. What is the t -test t test is named after its inventor, William Gosset, who published under the pseudonym of student. t test can be used either : 1.to evaluate whether a single group differs from a known value (a one-sample t-test), 2.to compare two independent groups (independent-samples t test) 3.to compare observations from two measurement occasions for the same group (paired-samples t test). Types of t-tests 1 Between subjects 3 t-test One sample t-test Within subjects 2 t-test 1. One Sample t-test A one sample t-test is used if we want to know whether a sample mean is different from a known population mean Before conducting the t-test, we need to first make sure that our data is normally distributed (assumption of normality)... Example In a statistics class of 20 young adults, one student wanted to determine whether they were taller than the national average. They began by wondering: “Is my height significantly different from the average height of young adults in the population?” Are we really taller? This is our degrees of freedom, which is calculated by n-1 (20 people in my class We get a t score of -.639. A negative t score in minus 1 = 19) this case suggests that we are NOT taller than the known population mean, but is this statistically significant? p value is.53. Since this is greater than our alpha value of.5, we say that we fail to reject the null hypothesis. In other words, there is no difference between how tall we are, and the population The output of the t-test will look something like this: Types of t-tests 1 Between subjects 3 t-test One sample t-test Within subjects 2 t-test 2. Between Subjects t-test Also known as independent samples t-test, it is used to compare groups which are not related (i.e., independent) Example A researcher wanted to find out if there is a difference in time spent on social media between males and females. She hypothesised that females spend more time a day on social media, compared to males. The researcher collected data from 25 males and 25 females Do females spend more time in a day on social media compared to males? Assumptions Testing… Before conducting the t-test, we need to first test the assumption of normality This is to evaluate if the variances between 2 groups were t value = 8.12, df = 48, significantly different from each and p value 1 MS B 1 2 3 F= MSW A video for describing the ANOVA procedure: https://www.youtube.com/watch?v=-yQb_ZJnFXw Between group degrees of freedom (k-1) Within group degrees of freedom N-k Critical F-value for result to be significant at 95% confidence level Significant if F>Fcritical Not Significant if F≤Fcritical F-value table for 95% confidence level Assumptions and Limitations Normality Assumption: The populations are assumed to both follow a normal curve Independence Assumption: Independent random samples Data Type Assumption: Interval or ratio data only Sample 1 Sample 2 Sample 2 Homogeneity Variance Assumption: All Is there a difference? samples have approximately the same variance Correlation Correlation: The degree of relationship between the variables under consideration is measure through the correlation analysis. The measure of correlation called the correlation coefficient. The degree of relationship is expressed by coefficient which range from correlation ( -1 ≤ r ≥ +1) The direction of change is indicated by a sign. The correlation analysis enable us to have an idea about the degree & direction of the relationship between the two variables under study. Correlation Correlation is the statistical technique used to determine the degree to which variables are related (“correlation, not causation”) Example: Rectangular coordinates Two quantitative variables: Independent/Explanatory (X) Y Dependent/Response (Y) X Correlation Correlation r indicates strength of correlation and direction of relationship (-/+) r can vary from -1 to 1 r = 0 → no correlation Assumptions and Limitations Normality Assumption: The two datasets are assumed to be normally distributed Independence Assumption: Two independent random samples Data Type Assumption: Interval or ratio data Relationship Assumption: The variables have a linear relationship Correlation & Causation Causation means cause & effect relation. If two variables vary in such a way that movement in one are accompanied by movement in other, these variables are called cause and effect relationship. Causation always implies correlation, but correlation does not necessarily imply causation. Types of Correlation Type I Correlation Positive Correlation Negative Correlation Types of Correlation Type I Positive Correlation: The correlation is said to be positive correlation if the values of two variables changing with same direction. Ex. Pub. Exp. & sales, Height & weight. Negative Correlation: The correlation is said to be negative correlation when the values of variables change with opposite direction. Ex. Price & qty. demanded. Direction of the Correlation Positive relationship – Variables change in the same direction. As X is increasing, Y is increasing Indicated by As X is decreasing, Y is decreasing sign; (+) or (-). E.g., As height increases, so does weight. Negative relationship – Variables change in opposite directions. As X is increasing, Y is decreasing As X is decreasing, Y is increasing E.g., As TV time increases, grades decrease Types of Correlation Type II Correlation Simple Multiple Partial Total Types of Correlation Type II Simple correlation: Under simple correlation problem there are only two variables are studied. Multiple Correlation: Under Multiple Correlation three or more than three variables are studied. Ex. Qd = f ( P,PC, PS, t, y ) Partial correlation: analysis recognizes more than two variables but considers only two variables keeping the other constant. Total correlation: is based on all the relevant variables, which is normally not feasible. Types of Correlation Type III Correlation LINEAR NON LINEAR Types of Correlation Type III Linear correlation: Correlation is said to be linear when the amount of change in one variable tends to bear a constant ratio to the amount of change in the other. The graph of the variables having a linear relationship will form a straight line. Ex X = 1, 2, 3, 4, 5, 6, 7, 8, Y = 5, 7, 9, 11, 13, 15, 17, 19, Y = 3 + 2x Non-Linear correlation: The correlation would be non linear if the amount of change in one variable does not bear a constant ratio to the amount of change in the other variable. Methods of Studying Correlation Scatter Diagram Method Graphic Method Karl Pearson’s Coefficient of Correlation Method of Least Squares Scatter Diagram Method Scatter Diagram is a graph of observed plotted points where each points represents the values of X & Y as a coordinate. It portrays the relationship between these two variables graphically. A perfect positive correlation Weight Weight of B Weight A linear of A relationship Height Height Height of A of B High Degree of positive correlation Positive relationship r = +.80 Weight Height Degree of correlation Moderate Positive Correlation r = + 0.4 Shoe Size Weight Degree of correlation Perfect Negative Correlation r = -1.0 TV watching per week Exam score Degree of correlation Moderate Negative Correlation r = -.80 TV watching per week Exam score Degree of correlation Weak negative Correlation Shoe r = - 0.2 Size Weight Degree of correlation No Correlation (horizontal line) r = 0.0 IQ Height Advantages of Scatter Diagram Simple & Non Mathematical method Not influenced by the size of extreme item First step in investing the relationship between two variables Disadvantage of scatter diagram Can not adopt an exact degree of correlation Karl Pearson's Coefficient of Correlation Pearson’s ‘r’ is the most common correlation coefficient. Karl Pearson’s Coefficient of Correlation denoted by- ‘r’ The coefficient of correlation ‘r’ measure the degree of linear relationship between two variables say x & y. Karl Pearson's Coefficient of Correlation ◼ Karl Pearson’s Coefficient of Correlation denoted by- r -1 ≤ r ≥ +1 ◼ Degree of Correlation is expressed by a value of Coefficient ◼ Direction of change is Indicated by sign ( - ve) or ( + ve) Procedure for computing the correlation coefficient Calculate the mean of the two series ‘x’ &’y’ Calculate the deviations ‘x’ &’y’ in two series from their respective mean. Square each deviation of ‘x’ &’y’ then obtain the sum of the squared deviation i.e.∑x2 &.∑y2 Multiply each deviation under x with each deviation under y & obtain the product of ‘xy’.Then obtain the sum of the product of x , y i.e. ∑xy Substitute the value in the formula. Interpretation of Correlation Coefficient (r) The value of correlation coefficient ‘r’ ranges from -1 to +1 If r = +1, then the correlation between the two variables is said to be perfect and positive If r = -1, then the correlation between the two variables is said to be perfect and negative If r = 0, then there exists no correlation between the variables Assumptions of Pearson’s Correlation Coefficient There is linear relationship between two variables, i.e. when the two variables are plotted on a scatter diagram a straight line will be formed by the points. Correlation does not imply causation—it only indicates association. Advantages of Pearson’s Coefficient It summarizes in one value, the degree of correlation & direction of correlation also. Limitation of Pearson’s Coefficient Always assume linear relationship Interpreting the value of r is difficult. Value of Correlation Coefficient is affected by the extreme values. Time consuming methods Coefficient of Determination Coefficient of determination: The percentage of variance in one variable that is accounted for by the variance in the other variable. = square of coefficient rGPA.Time = 0.70 49% of the variance in GPA can be explained by the variance in studying time r2 GPA.Time = 0.49 Coefficient of Nondetermination The amount of unexplained variance is called the coefficient of undetermination (coefficient of alienation) correlation determination interpretation 0 0 0.5 0.25 0.9 0.81 Advantages of Correlation studies Show the amount (strength) of relationship present Can be used to make predictions about the variables under study. Can be used in many places, including natural settings, libraries, etc. Regression Correlation measures the strength of the linear association between variables Regression analysis refers to the more complete process of studying the relationship between a dependent variable and a set of independent, explanatory variables Linear regression analysis: Assumes a linear relationship exists between the dependent or response variable (y) and the independent or explanatory variables (x) Fits a straight line to the set of observed data Yields an equation that allows us to predict values of y from values of x Regression Analysis Regression Analysis is a very powerful tool in the field of statistical analysis in predicting the value of one variable, given the value of another variable, when those variables are related to each other. Regression Analysis Regression Analysis is mathematical measure of average relationship between two or more variables. Regression analysis is a statistical tool used in prediction of value of unknown variable from known variable. Advantages of Regression Analysis Regression analysis provides estimates of values of the dependent variables from the values of independent variables. Regression analysis also helps to obtain a measure of the error involved in using the regression line as a basis for estimations. Regression analysis helps in obtaining a measure of the degree of association or correlation that exists between the two variable. Regression When there is just one independent variable and we wish to fit a straight line through the set of data points; the equation of this line is: ŷ = a + bx ŷ is the estimated value of y a is the y intercept of the line b is the slope of the line Regression Example: p p = 30,000 + 70*s p: price of house ($) s: area of house (sq ft) s → The price predicted for a house with 2000 square feet is $30,000 + $70 * 2000 = $170,000 Regression Residuals, ei - Each observation (i) of the dependent variable, yi, may be expressed as the sum of a predicted value and a residual term: yi = a + bxi + ei ei = ŷ - yi Fitting a Regression Line Objective: to find the slope ŷ = a + bx and intercept of a best-fitting line that runs through the observed set of data points One approach: “Least ei Squares” Fit the line so that the sum of the minimum distances of the observations to the line was a minimum → minimize the sum of the squared residuals Fitting a Regression Line Equations to find the values of a and b ŷ = a + bx that minimize the sum of the squared residuals: n n n n X iYi − X i Yi i =1 i =1 i =1 b= n n 2 ŷ = a + bx e n X i − X i 2 i i =1 i =1 n n Yi − b X i i =1 i =1 a= n Explained & Unexplained Sums of Squares Regression provides a way to partition the variation of a dependent (y) variable into two parts: 1. A part that is explained by the regression line 2. A part that remains unexplained Expressed by the Variability in y may be measured Coefficient of Determination*, r2 by the sum of squared deviations of the y values from their mean Explained variation in y r2 = total variation in y The total variation in y is the sum of the explained variation and the unexplained variation → Mathematically, this can be expressed as: Total sum of squares (TSS ) = Explained (mean) sum of squares ( MSS ) + Unexplained (residual) sum of squares ( RSS ) n n (Yi − Y ) = ( ) + ( ) 2 2 Yˆi − Y Yi − Yˆi 2 i =1 i =1 n ( ) 2 Yi − Yˆi MSS RSS i =1 r2 = =1− r 2 =1− Defined in terms of r2: TSS TSS n (Yi − Y ) 2 i =1 Explained & Unexplained Sums of Squares Explained variation in y r2 = total variation in y r2 can vary from 0 to 1 r2 = 0.10 → 10% of the variation in y explained by x r2 = 0.50 → 50% r2 = 1.00 → 100% Regression: Testing for Significance Has the regression been successful at explaining a significant portion of the variation in y? Null Hypothesis: the proportion of the variability in y explained by x is equal to zero: H0: r2 = 0 Explained variation in y r2 = total variation in y → Test using the F-statistic: 𝑟 2 (𝑛 − 2) 𝐹= 1 − 𝑟2 Number of variables (V-1) F-statistic Number of paired observations 𝑟 2 (𝑛 − 2) n-2 𝐹= 1 − 𝑟2 Critical value of F for result to be significant at 95% confidence level Significant if F>Fcritical Not Significant if F≤Fcritical Assumptions in Regression Analysis Existence of actual linear relationship. The regression analysis is used to estimate the values within the range for which it is valid. The relationship between the dependent and independent variables remains the same till the regression equation is calculated. The dependent variable takes any random value but the values of the independent variables are fixed. In regression, we have only one dependant variable in our estimating equation. However, we can use more than one independent variable. Regression Equation / Line Simple Regression Equation Regression Equation / Line ▪ Multiple Regression Equation For two or more independent variables, the equation extends to: Here, multiple predictors (X1,X2,...,Xn) influence Y. Correlation analysis vs. Regression analysis. Regression is the average relationship between two variables Correlation need not imply cause & effect relationship between the variables understudy.- R A clearly indicate the cause and effect relation ship between the variables. There may be non-sense correlation between two variables.- There is no such thing like non-sense regression. Next time Introduction to Spatial Analysis Reminders: Project Report Outline Due next time: Assignment 08 on 25th March before class Canvas Quiz: Non-Parametric Tests Access code: idea