Teachers' Generative AI Self-Efficacy and Integration (2024 PDF)
Document Details
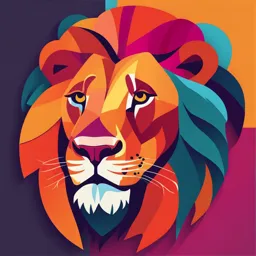
Uploaded by MagnificentHammeredDulcimer6619
Ain Shams University
2024
Rebecca J. Collie, Andrew J. Martin, Dragan Gasevic
Tags
Related
- Education in the Era of Generative AI (2023) PDF
- Generative AI in Education Policy Paper PDF
- Classification Tool for Self-Regulated Learning with ChatGPT PDF
- Generative AI and the Future of Education PDF
- Education Hazards of Generative AI PDF
- Technology for Teaching and Learning P1 (EDUC 015) Learning Module PDF
Summary
This research investigates the factors influencing teachers' motivation and integration of generative AI in the classroom. The study analyzes the effects of job resources, time pressure, and disruptive student behavior on teachers' self-efficacy and valuing related to AI, and their integration into classroom activities. The study, conducted among Australian teachers during the 2024 school year, provides substantial insights into this area.
Full Transcript
Computers and Education: Arti cial Intelligence 7 (2024) 100333 Contents lists available at ScienceDirect Computers and Education: Artificial Intelligence jo...
Computers and Education: Arti cial Intelligence 7 (2024) 100333 Contents lists available at ScienceDirect Computers and Education: Artificial Intelligence journal homepage: www.sciencedirect.com/journal/computers-and-education-artificial-intelligence Teachers’ generative AI self-efficacy, valuing, and integration at work: Examining job resources and demands Rebecca J. Collie a,*, Andrew J. Martin a, Dragan Gasevic b a School of Education, University of New South Wales, Australia b Faculty of Information Technology, Monash University, Australia A R T I C L E I N F O A B S T R A C T Keywords: Generative AI (genAI) tools have involved rapid and broad uptake since their wide release in late 2022, including Generative AI among teachers. We investigated several factors that play a role in teachers’ motivation and engagement to Teachers harness genAI in teaching and learning. We examined contextual factors (in-school support to apply genAI, time Motivation pressure, disruptive student behavior) as predictors of motivation (genAI self-efficacy and genAI valuing) and, in Engagement Integration turn, engagement (i.e., genAI integration in teaching-related work and student learning activities) over the Job demands course of one school term. Among 368 Australian primary and secondary school teachers, our findings revealed Job resources that genAI support was associated with greater genAI self-efficacy and genAI valuing. Time pressure was also linked with greater genAI valuing, whereas disruptive student behavior was not linked with the genAI motivation or engagement variables. In turn, genAI self-efficacy was linked with greater levels of both types of genAI integration. GenAI valuing was associated with greater genAI integration in teaching-related work only. Our results provide knowledge about factors relevant for supporting genAI and its application among teachers in Australia—and also hold relevance to teachers in other countries. 1. Introduction knowledge of predictors linked with greater motivation and engagement for this technology is important to better understand what factors are Generative artificial intelligence (genAI) refers to technology that supportive of, and what factors may be hindering, teachers’ employs transformer architectures of deep machine learning techniques genAI-related motivation and engagement. (Vaswani et al., 2017) to generate new content, including text, images, The aim of this study, therefore, was to advance knowledge of the and audio (e.g., ChatGPT, Microsoft Copilot, Canva; Lim et al., 2023). role of contextual factors in predicting school teachers’ motivation and Although ample research has looked at different factors that predict engagement for applying genAI in their work. For motivation, we teachers’ technology and (gen)AI acceptance (Holden & Rada, 2011; Joo assessed teachers’ self-efficacy and valuing for using genAI at work. For et al., 2018; Kong et al., 2024; Martínez-Moreno & Petko, 2024), there is engagement, we assessed genAI integration in teaching-related work more limited understanding of the factors that predict teachers’ inte and student learning activities. Among a sample of Australian primary gration of genAI in teaching-related work and student-learning activ and secondary school teachers, we examined the contextual factors at ities. Emerging work is now examining such factors (Collie & Martin, the beginning of a school term with particular interest in their relation to 2024). However, given the nascency of this technology, which was teachers’ genAI-related motivation and engagement near the end of the widely released from late 2022, more work is needed. This gap is term. We also investigated the associations between teachers’ genAI- important to address given that many educational bodies are now related motivation and engagement. We examined three contextual developing policies that encourage teachers to employ genAI to assist factors: one factor that can be considered a job resource—in-school with their teaching work (e.g., Australian Government, 2023; BC Min support to apply genAI; and two factors that can be considered job istry of Education and Child Care, 2024; NSW Department of Education, demands—time pressure and disruptive student behavior. Fig. 1 dis 2024; US Office of Educational Technology, 2023), and some are also plays the hypothesized model. Although our study involves a sample of promoting its use in student learning activities (e.g., UK Department for Australian primary and secondary teachers, the factors examined in our Education, 2023; SA Department of Education, 2024). Thus, developing study have been found to be salient experiences for teachers in many * Corresponding author. School of Education, University of New South Wales, NSW, 2052, Australia. E-mail address: [email protected] (R.J. Collie). https://doi.org/10.1016/j.caeai.2024.100333 Received 9 October 2024; Received in revised form 24 November 2024; Accepted 25 November 2024 Available online 26 November 2024 2666-920X/© 2024 The Authors. Published by Elsevier Ltd. This is an open access article under the CC BY license (http://creativecommons.org/licenses/by/4.0/). R.J. Collie et al. Computers and Education: Arti cial Intelligence 7 (2024) 100333 other countries, including in cross-cultural work (e.g., time pressure, theory, teachers who experience greater job demands (again, which disruptive student behavior; Collie, 2021; Skaalvik & Skaalvik, 2018) represent a loss of one or more types of assets or tools) can turn towards and thus we draw on empirical research across numerous countries. genAI as a means of developing additional assets/tools to protect Moreover, findings are expected to hold relevance to teachers across themselves going forward. Together, then, job demands play two different jurisdictions and countries. different roles in these theories—they are hindering for general work motivation and engagement in job demands-resources theory, but can 1.1. Conceptual framing for our study end up being beneficial for motivation and engagement directed to wards asset/tool accumulation in conservation of resources theory. We refer to two frameworks for our study’s conceptual un Below, we provide further details about how these two theories derpinnings. At a general level, we refer to job demands-resources the informed our hypothesizing. ory (Bakker & Demerouti, 2017), which establishes the role of contextual factors in predicting employee functioning at work. Namely, 1.2. GenAI motivation and engagement the theory differentiates between job resources and job demands. Job resources are contextual factors that help workers undertake their work Motivation and engagement are recognized as salient for teachers’ effectively and to meet their professional goals. In contrast, job demands functioning at work (Lazarides & Schiefele, 2024; Mérida-López & are contextual factors that hinder workers’ ability to function effectively Extremera, 2020), and research is now revealing understanding about and meet their goals at work. Research among teachers supports these these constructs in relation to genAI (Al Darayseh, 2023; Collie & propositions looking at teachers’ work motivation generally. For Martin, 2024; Martínez-Moreno & Petko, 2024; Moorhouse, 2024). In example, teachers’ perceptions of job resources, such as feeling sup the current study, we extend knowledge related to genAI-related moti ported at work, are linked with greater levels of work motivation and vation and engagement. For motivation, we assessed self-efficacy and engagement, whereas perceptions of job demands, such as time pressure valuing for using genAI. Self-efficacy is a capability self-belief reflecting and disruptive student behavior, are linked with less adaptive forms of individuals’ confidence that they will be able to effectively undertake an work motivation and engagement (Collie, 2023b; Granziera et al., 2022; upcoming task (Bandura, 2001). Self-efficacy, and other capability Lu, Chen, et al., 2024; Nie et al., 2015). self-beliefs (e.g., perceived competence, expectancies), feature in When specifically considering the domain of genAI—that is, moti numerous motivation theories and are considered a critical facet of vation and engagement related to genAI—job resources are expected to motivation (e.g., Bandura, 2001; Eccles & Wigfield, 2023; Ryan & Deci, play a similar role as that indicated in job demands-resources theory. 2017). Self-efficacy has also been examined as a predictor of technology This is because job resources provide teachers with opportunities to and (gen)AI acceptance among teachers (e.g., Al Darayseh, 2023; Ghi meet their work goals and further develop as a professional. GenAI mire & Edwards, 2024, pp. 129–134; Holden & Rada, 2011; Kong et al., represents a new technology that teachers can explore and apply to 2024). In the present study, genAI self-efficacy was operationalized as develop their professional skills. Prior research supports this showing teachers’ confidence to effectively use genAI to complete core teaching job resources are linked with greater genAI-related motivation (e.g., tasks (e.g., develop lesson plans or feedback, create assessment tasks). Collie & Martin, 2024). As such, job demands-resources theory informed Valuing is another core facet of motivation that features in numerous our hypothesizing related to our job resource (discussed in detail below). theories (e.g., Eccles & Wigfield, 2023; Ryan & Deci, 2017). Valuing When it comes to job demands, however, there are two lines of denotes individuals’ subjective valuing of a particular task or tool theorizing that are potentially relevant. The first is job demands- (Wigfield et al., 2016).Valuing comprises four components: attainment resources theory, which as noted above, states that job demands lead value, which signifies how crucial individuals perceive a task or tool to to lower motivation and engagement. In our study, the domain- be in achieving their objectives; intrinsic value, which relates to how specificity of the motivation and engagement factors means that individuals perceive a task or tool as being enjoyable or interesting to another line of theorizing is also relevant. Conservation of resources perform or utilize; utility value, which reflects individuals’ belief that a theory (Hobfoll, 1989, 2018) states that job demands indicate that an task or tool will be beneficial for achieving future goals; and, relative individual has experienced a loss of resources (e.g., the loss of time due cost, which pertains to individuals’ belief that using a task or tool will to the job demand of time pressure). For clarity, we will refer to these divert time from other goals (Wigfield et al., 2020). In the present study, resources as assets or tools to avoid confusion with job resources above. we examined the four components under an overarching construct, but Individuals must then seek out other assets/tools in order to recover considered relative benefits instead of costs. Relative benefits reflect from those losses and protect from losses in future. That is, individuals individuals’ perceptions that application of the task or tool will provide build a reservoir of assets/tools to manage potential future experiences additional time or opportunities to undertake other activities (Collie & of job demands. Alongside this, when individuals are experiencing high Martin, 2024). Relative benefits are considered particularly appropriate levels of job demands (e.g., high time pressure), it becomes even more for genAI because of the ease with which teachers are able use the important to seek out assets/tools. In the present study, genAI represents technology. This approach also gets around issues with the construct of a technological asset/tool. Thus, according to conservation of resources relative cost, which is negatively-valenced compared with the other Fig. 1. Hypothesized Model Note. GenAI = generative artificial intelligence. 2 R.J. Collie et al. Computers and Education: Arti cial Intelligence 7 (2024) 100333 valuing components, and at times can lead to issues in modelling (Jiang teachers’ integration of technology in student learning activities. et al., 2018). As noted, we examined the four components under an Together, then, various supports at work have been linked with overarching construct of genAI valuing, which reflects teachers’ beliefs teachers’ motivation and engagement in genAI or technology more that genAI will be valuable for their work (attainment value), will mean generally. The present study extends that work by considering support their work is more enjoyable (intrinsic value), will help them undertake specifically for using genAI. Based on this prior work and job demands- their work tasks (utility value) and, will allow them to do tasks they resources theory (Bakker & Demerouti, 2017), we hypothesized that could not ordinarily do (e.g., due to limited knowledge of an area or time having greater access to school-based support for genAI would mean constraints; relative benefits). Valuing by way of perceived usefulness teachers are more likely to be motivated (Hypothesis 2) and engaged to (which is very similar to utility value) has been examined as a predictor harness genAI in the workplace (Hypothesis 3). of technology and (gen)AI acceptance among teachers (e.g., Al Dar ayseh, 2023; Ghimire & Edwards, 2024; Holden & Rada, 2011; Kong 1.3.2. Time pressure and disruptive student behavior as job demands et al., 2024). The two job demands examined in the current study were time For engagement, we investigated teachers’ genAI integration at work pressure and disruptive student behavior. Time pressure denotes teach in their own teaching-related tasks and in student learning activities. ers’ perceptions that their workload is too high to complete within their These two forms of genAI integration can be considered types of available time (Prieto et al., 2008). Disruptive student behavior pertains to behavioral engagement because they reflect teachers’ actions (Martin teachers’ perceptions that their students are off-task and distracting et al., 2024; Skinner & Pitzer, 2012). In our study, genAI integration in others within the classroom (e.g., by calling out; Collie, 2023b). These teaching-related work denotes teachers’ use of the technology to aid them two job demands were selected as they are recognized as common with their work as a teacher (planning, providing feedback, assessing; challenges for teachers (Dicke et al., 2018; Stang-Rabrig et al., 2022), Collie & Martin, 2024). GenAI integration in student learning activities and they may also be implicated in teachers’ genAI motivation and captures the extent to which teachers incorporate genAI in student engagement. More precisely, teachers who are experiencing time pres learning activities (e.g., to develop ideas, check their work, sure may turn to genAI to assist in streamlining their workloads (Collie & self-assessment; Collie & Martin, 2024). Martin, 2024; Goldman et al., 2024). Indeed, there are emerging genAI Together, the motivation and engagement factors denote teachers’ technologies aimed at assisting teachers with their lesson planning (Fan perceptions about genAI as relevant to their professional tasks, and their et al., 2024), improving their questioning quality (Demszky et al., 2023), current application of genAI in teaching and learning. As we explain in and broader professional development (Lu, Zheng, et al., 2024). Reports detail below, we were interested in examining contextual predictors of also indicate that teachers are using genAI to generate ideas for new both genAI motivation and engagement. Alongside that aim, and since activities, plan the scope and sequence of learning, develop individual motivation is typically understood to function as a precursor to education plans, or polish communication to parents (Cambium engagement (e.g., Eccles & Wigfield, 2023; Ryan & Deci, 2017), we Learning Group, 2024; Collie & Martin, 2025; Goldman et al., 2024). anticipated that genAI motivation would be linked with higher levels of GenAI assistance in these areas may help to save teachers time. Addi genAI engagement (Hypothesis 1). tionally, teachers who experience disruptive student behavior may turn to genAI to provide additional ideas for classroom management or to 1.3. Job resources and demands as predictors of GenAI motivation and make content and pedagogical approaches more engaging and relevant engagement for students. For example, reports indicate that teachers are using genAI to design their presentations, reading lists and timetables, provide Job resources and demands are known to be associated with teach real-time feedback, and to develop personalized learning tasks ers’ motivation and engagement at work generally (Granziera et al., (Cambium Learning Group, 2024; Collie & Martin, 2025). Teachers are 2022; Lu, Chen, et al., 2024). In our study, we examined one job also using genAI to develop self-assessment or peer-assessment tasks for resource and two job demands as predictors of genAI motivation and students, and are asking students to generate their own personal orga engagement. nization approaches with genAI (Collie & Martin, 2025). Research that directly examines the link between job demands and 1.3.1. GenAI support as a job resource genAI motivation/engagement is only now emerging. For example, The job resource was in-school support to apply genAI or genAI Collie and Martin (2024) demonstrated that teachers experiencing support, which reflects teachers’ perceptions that their workplace pro autonomy-thwarting leadership (i.e., a job demand related to pressuring vides them with adequate support and guidance to apply genAI in their and inflexible leadership practices) reported greater genAI engage teaching work. Although research examining this job resource has yet to ment—specifically, integration in student learning activities. Consid emerge, work examining support more broadly is informative. For ering technology more broadly, research has examined transactional example, researchers have found that teachers’ perceptions of leadership, which can be perceived as autonomy-thwarting by em autonomy-supportive leadership (i.e., when their principal takes ployees given it focuses on ensuring compliance. Prior research has teachers’ perspective and supports their self-determination) are linked found that transactional leadership is linked with reduced innovation in with greater genAI valuing at work (Collie & Martin, 2024). In other teaching (Pierterse et al., 2010) and lower levels of digital trans work, Lu, Zheng, et al. (2024) examined a professional development formation in schools (Ruloff & Petko, 2021). Clearly, additional research program for pre-service teachers that embedded genAI as an additional is needed to better understand what factors may support or hinder genAI support. Results revealed that participation in the program was linked motivation and engagement. This is important given the changing policy with higher teaching self-efficacy compared with a control group where space that is encouraging teachers, and sometimes students, to use genAI pre-service teachers underwent the same training but without support technology in schools (e.g., NSW Department of Education, 2024; UK from genAI. More generally, research has found that teachers’ percep Department for Education, 2023). tions of transformational leadership at their school are linked with Our study advances knowledge by focusing on two common, but greater technology valuing, but are unassociated with their integration unexamined job demands in relation to genAI motivation and engage of technology in student learning activities (Schmitz et al., 2023; see also ment. As noted earlier, there are two lines of theorizing relevant to job Chiu, 2022; Vermeulen et al., 2015). In another study, Karaca et al. demands. According to job demands-resources theory (Bakker & (2013) investigated a broader technological support variable Demerouti, 2017), the experience of job demands is linked with lower (comprising technological access, technical support, and professional genAI-related motivation and engagement because teachers do not have development for technology integration). Their study showed this sup the time (i.e., time pressure) or are focusing on more immediate issues (i. port variable was linked with greater technology-related valuing and e., disruptive behavior) that prevents them from exploring and applying 3 R.J. Collie et al. Computers and Education: Arti cial Intelligence 7 (2024) 100333 genAI at work. However, according to conservation of resources theory align with the population parameters of Australian teachers. For (Hobfoll, 1989, 2018), individuals who experience job demands seek out example, primary schools comprise 82% female staff and secondary additional assets/tools to assist them to better manage future experi schools comprise 61% female staff (ABS, 2022). Australian teachers ences of those job demands. In that sense, job demands promote greater work in government (63%), systemic Catholic (19%), and independent motivation and engagement directed towards asset/tool accumulation. schools (17%; ABS, 2022). GenAI acts as a technological tool that is potentially relevant to the two Data were collected between April and July of 2024 at two time job demands of time pressure and disruptive student behavior because it points, which corresponded with Term 2 of four terms in the Australian may save teachers time (relevant to time pressure), and it provides op school year. Time 1 was collected in week 2 of the school term, and Time portunities to develop teaching and learning approaches that may assist 2 was collected in week 9 of the same term. Teachers were recruited with classroom management and student engagement (relevant to through Qualtrics and their market research panel partners. Individuals disruptive student behavior). who had previously specified interest in participating in education Given this theorizing, two opposing, alternative hypotheses were surveys were notified of the study via email or app and invited to advanced for the link between the job demands and our motivation/ participate. These individuals opened the online survey, indicated their engagement factors. Based on job demands-resources theory, we antic consent, and answered screening questions that ensured they were ipated that time pressure may be negatively associated with genAI working as a teacher in an Australian school. Individuals who passed motivation (Hypothesis 4.1) and engagement (Hypothesis 5.1). We also screening requirements, but who completed the survey very quickly expected that disruptive student behavior may be negatively associated (80%), or with genAI motivation (Hypothesis 6.1) and engagement (Hypothesis alike to two oppositely formulated item pairs, were removed from the 7.1). In contrast, and based on conservation of resources theory, we final sample (Dewitt et al., 2019). The response rate was 92% at Time 1. anticipated that time pressure may be positively associated with genAI The sample size at Time 2 was 241, reflecting an attrition rate of 35% motivation (Hypothesis 4.2) and engagement (Hypothesis 5.2). We also across time. Two background characteristics were linked with missing expected that disruptive student behavior may be positively associated ness at Time 2 (teacher age, school type), but when these were entered with genAI motivation (Hypothesis 6.2) and engagement (Hypothesis into our final model (described below) all significant paths remained. As 7.2). We also had a research question surrounding the extent to which such, only the original research-supported covariates were retained the contextual factors would be indirectly associated with the engage (Enders, 2022)—also because there was evidence of multicollinearity ment outcomes via motivation (Research Question [RQ] 1). between age and one of our core covariates (teaching experience), which were highly correlated. For completeness, we also reran our analyses 2. Study overview with the fully matched sample only, and all significant paths involving our substantive factors remained. This provides robust evidence that We examined the role of job resources and demands assessed at the there was no bias associated with attrition that impacted findings. The beginning of a school term (Time 1) in relation to teachers’ genAI study received Institutional Review Board ethics approval. motivation (self-efficacy and valuing) and genAI engagement (integra tion of genAI in teaching-related work and student learning activities) assessed near the end of the same school term (Time 2). We also 3.2. Measures investigated the association between teachers’ genAI motivation and genAI engagement. One relevant job resource was examined (i.e., genAI Prior work has yielded evidence of validity for the scales scores by support) along with two job demands (i.e., time pressure, disruptive demonstrating adequate reliability, measurement invariance, and ex student behavior). Although not central to hypotheses, we also pected associations with correlates (Collie, 2023a; Collie & Martin, controlled for background factors comprising teacher and job charac 2024; Donnellan et al., 2006). The current research provides validity teristics (i.e., gender, teaching experience, contract type, class size, evidence for the scores of the new scales (genAI support and school level) and the Big-5 personality factors (which represent dispo self-efficacy; see Data Analysis). Reliability estimates are displayed in sitional traits of openness, agreeableness, conscientiousness, extraver Table 1 and were all at adequate levels (i.e., ω >.70). In the survey, sion, and neuroticism that impact individuals’ perceptions and response teachers were first provided with the following brief description of in life; see Section 3.2.4 for more on these factors). Fig. 1 displays the genAI before they answered relevant items: “Generative artificial intel hypothesized model. ligence (generative AI) refers to advanced technology that uses machine learning to generate new content, such as text, images, music. Some 3. Methods Table 1 3.1. Sample and procedure Reliabilities, descriptive statistics, and factor loadings for substantive factors. Omega M SD Factor Our study comprised a sample of 368 Australian school teachers that (ω) Loadings M (Range) completed two surveys, the first near the start of a 10-week school term and the second near the end of the same term. The teachers worked in T1 Contextual factors primary schools (56%), secondary schools (40%), or at both levels (4%). GenAI support.94 2.62 1.39.89 (.75–.95) Time pressure.90 5.84 1.24.83 (.71–.91) Participants identified as female (82%), male (17%), or as non-binary Disruptive behavior.89 4.58 1.48.81 (.73–.87) (or used other terms) for gender (1%). The sample’s average age was T2 Motivation 39 (SD = 11) years and they had an average of 13 (SD = 10) years’ GenAI self-efficacy.96 4.10 1.69.90 (.84–.93) experience as teachers. Most of the participants had received a bache GenAI valuing.94 4.65 1.43.89 (.84–.92) T2 Engagement Outcomes lor’s degree (63%) or a postgraduate degree (35%; the rest held a cer GenAI integration in teaching- – 2.13 1.05 – tificate or diploma, 2%), and nearly all (93%) spoke only English at related work home. Participants indicated they worked in inner city (10%), suburban GenAI integration in student – 0.33 0.47 – (72%), rural (17%), or remote areas (1%). Teachers also reported the learning activities socio-economic status for their school as low (20%), below average Note. Factor loadings and omegas are from the measurement model involving (17%), average (38%), above average (21%), or high (4%). Participants genAI integration in teaching-related work. Loadings were very similar for the were employed in government (70%), systemic Catholic (15%), or non- CFA involving genAI integration in student learning activities, as expected. T1 = government/independent schools (15%). The sample statistics generally Time 1. T2 = Time 2. 4 R.J. Collie et al. Computers and Education: Arti cial Intelligence 7 (2024) 100333 examples of these online tools include ChatGPT, GPT-4, Dall-E 2, Bing potential tasks (e.g., learning activities in-class, homework, study/ Chat AI, and Bard AI.” (Collie & Martin, 2024). Scales were scored from revision, other). Teachers selected the responses that applied to them 1 (strongly disagree) to 7 (strongly agree) unless stated otherwise below. and then responses were coded 0 (for “none”) or 1 (when teachers chose one or more learning task). Of the Time 2 respondents, 34% indicated 3.2.1. Contextual factors (time 1) they were integrating genAI in student learning activities. GenAI support was measured with four items developed for this study that tap into teachers’ perceptions that their school provides 3.2.4. Background characteristics support for effectively using genAI in their work. The items were: “At Several background characteristics were included as covariates in work, I receive the support I need to integrate generative AI into my our study to ascertain unique links among substantive factors: gender, teaching,” “My school provides me with sufficient resources for using teaching experience, longer-term contract, class size, school level, and generative AI in my work,” “At work, I am encouraged to use generative the Big 5 personality factors (openness, agreeableness, conscientious AI for my teaching,” “I receive the necessary support at work to apply ness, extraversion, and emotional instability). In prior research, these generative AI effectively.” The present study provides evidence of val characteristics have been associated with our substantive factors. For idity for the scale scores via demonstration of measurement invariance, example, male teachers have reported greater genAI valuing (Collie & anticipated associations with correlates, sound factor structure, and Martin, 2024) and time pressure has been linked with genAI integration adequate reliability (see Results for details). Time pressure was assessed (Goldman et al., 2024), and the personality factors are implicated in with the 4-item Time Pressure Scale (“I feel pressed for time in my job,” teachers’ general work motivation in distinct ways (Perera et al., 2018). “I rarely have enough time to get everything done in my work,” “In my Gender was coded 0 (male) or 1 (female). Teaching experience was job, I rarely get to do my planning and preparation to the depth I would continuous (coded in years). Longer-term contract was coded 0 (fixed like,” and “I feel rushed in my job because there is rarely enough time to term for less than 1 year) or 1 (permanent, or fixed term for more than 1 get things done; ” Collie, 2023a). Disruptive student behavior was assessed year). Class size was continuous and coded as total number. School level with the 4-item Disruptive Behavior Scale (“When I’m teaching, there is was coded 0 (elementary school) or 1 (secondary school). Donnellan a lot of noise and disorder among my students,” “It is difficult to ensure et al.’s (2006) 20-item Mini-IPIP (International Personality Item Pool) my students concentrate on their schoolwork,” “My students take a long scale was used to assess the Big-Five personality dimensions of openness time to quieten down when I begin teaching,” and “Managing my stu (e.g., “I have a vivid imagination”), agreeableness (“I sympathize with dents’ behaviour in lessons takes a lot of time and effort; ” Collie, others’ feelings”), conscientiousness (e.g., “I get chores done right 2023a). away”), extraversion (e.g., “I talk to a lot of different people at parties”), and neuroticism (e.g., “I get upset easily”). Items were scored from 1 3.2.2. GenAI motivation (time 2) (very inaccurate) to 5 (very accurate). GenAI self-efficacy was assessed with the following stem: “Assuming I decide or am asked to use generative AI in my teaching next week …” 3.3. Data Analysis This was accompanied by 5 items that capture self-efficacy in relation to major tasks in teaching work: “I am confident I will be able to effectively Using Mplus 8.11 (Muthén & Muthén, 2024), confirmatory factor use generative AI to: “… develop lesson plans,” “… develop teaching analysis (CFA) and structural equation modelling (SEM) were conduct resources and activities,” “… create assessment tasks that are aligned ed. Because outcome factors were operationalized and scored in with the curriculum,” “… develop feedback to share with students,” and different ways (see Measures, above), we ran separate models for each. “… prepare written communication to share with parents and More precisely, because one outcome was ordinal (genAI integration in colleagues.” teaching-related work) and one was binary (genAI integration in student GenAI valuing was assessed with Collie and Martin’s (2024) four activities), these entailed different estimation procedures. By running items and used the following stem: “Thinking ahead, in the next 1–2 the two models separately we were able to estimate appropriately for the years I believe …” The items tapped into the various types of valuing: different outcomes and also retain all controls for shared variance attainment value (“It will be important to use generative AI in my work among the predictors (these controls are critical for identifying unique as a teacher”), intrinsic value (“Using generative AI will make my work predictors).1 We used robust maximum likelihood (MLR) for the esti more interesting”), utility value (“Using generative AI will be useful for mator in models involving genAI integration in teaching-related work helping me do various teaching tasks”), and relative benefit (“Using (the ordinal outcome) with full information maximum likelihood generative AI will enable me to do things in my work that I normally (FIML). We used weighted least squares means and variances (WLSMV) could not do [e.g., save time that I can put towards other work-related for the estimator in models involving genAI integration in student ac tasks]”). Notably, the two motivation factors had different stems. For tivities (the binary outcome) with pairwise present. Missing data were valuing, we wanted to allow all teachers to report on this factor minimal (