Chapter 9: Multi Criteria Decision Making 09.pdf
Document Details
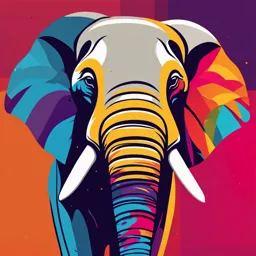
Uploaded by ResoluteConnemara508
Tags
Summary
This chapter discusses techniques for solving problems with multiple objectives, including goal programming, the analytical hierarchy process, and scoring models. It utilizes the Beaver Creek Pottery Company example to illustrate the application of these techniques. The chapter demonstrates how these models are formulated and solved graphically.
Full Transcript
CHAPTER 9: MULTI CRITERIA DECISION MAKING Overview In all the linear programming models presented in Chapters 2 through 8, a single objective was either maximized or minimized. However, a company or an organization often has more than one objective, which may relate to something...
CHAPTER 9: MULTI CRITERIA DECISION MAKING Overview In all the linear programming models presented in Chapters 2 through 8, a single objective was either maximized or minimized. However, a company or an organization often has more than one objective, which may relate to something other than profit or cost. In fact, a company may have several criteria, that is, multiple criteria, that it will consider in making a decision instead of just a single objective. In this chapter, we discuss three techniques that can be used to solve problems when they have multiple objectives: goal programming, the analytical hierarchy process, and scoring models. Goal programming is a variation of linear programming in that it considers more than one objective (called goals) in the objective function. Goal programming models are set up in the same general format as linear programming models, with an objective function and linear constraints. The model solutions are very much like the solutions to linear programming models. The format for the analytical hierarchy process and scoring models, however, is quite different from that of linear programming. These methods are based on a comparison of decision alternatives for different criteria that reflects the decision maker’s preferences. The result is a mathematical ―score‖ for each alternative that helps the decision maker rank the alternatives in terms of preferability. Learning Outcome At the end of this chapter, students must be able to: 1. Discuss the techniques that can be used to solve problems with multiple objectives such as goal programming, the analytical hierarchy process and scoring models. COURSE MATERIALS Goal Programming A goal programming model is very similar to a linear programming model, with an objective function, decision variables, and constraints. Like linear programming, goal programming models with two decision variables can be solved graphically and by using QM for Windows and Excel. Model Formulation We will use the Beaver Creek Pottery Company example again to illustrate the way a goal programming model is formulated and the differences between a linear programming model and a goal programming model. Recall that this model was originally formulated in Chapter 2 as follows: 143 The objective function, Z, represents the total profit to be made from bowls and mugs, given that $40 is the profit per bowl and $50 is the profit per mug. The first constraint is for available labor. It shows that a bowl requires 1 hour of labor, a mug requires 2 hours, and 40 hours of labor are available daily. The second constraint is for clay, and it shows that each bowl requires 4 pounds of clay, each mug requires 3 pounds, and the daily limit of clay is 120 pounds. This is a standard linear programming model; as such, it has a single objective function for profit. However, let us suppose that instead of having one objective, the pottery company has several objectives, listed here in order of importance: 1. To avoid layoffs, the company does not want to use fewer than 40 hours of labor per day. 2. The company would like to achieve a satisfactory profit level of $1,600 per day. 3. Because the clay must be stored in a special place so that it does not dry out, the company prefers not to keep more than 120 pounds on hand each day. 4. Because high overhead costs result when the plant is kept open past normal hours, the company would like to minimize the amount of overtime. These different objectives are referred to as goals in the context of the goal programming technique. The company would, naturally, like to come as close as possible to achieving each of these goals. Our first step in formulating a goal programming model is to transform the linear programming model constraints into goals. Labor Goal The first goal of the pottery company is to avoid underutilization of labor—that is, using fewer than 40 hours of labor each day. To represent the possibility of underutilizing labor, the linear programming constraint for labor, x1 + 2x2 ≤ 40 hours of labor, is reformulated as This reformulated equation is referred to as a goal constraint. The two new variables, d- 1, and d+ 1 are called deviational variables. They represent the number of labor hours less than 40 (d1+). More specifically, d- 1 represents labor underutilization, and d+ 1 represents overtime. For example, of x1 = bowls and x2 = 10 mugs then a total of 25 hours of labor have been expended. Substituting these values into our goal constraint gives 144 Because only 25 hours were used in production, labor was underutilized by 15 hours (40 – 25 = 15). Thus, if we let d1+ = 15 hours amd d1+ = 0 (because there is obviously no overtime), we have Now consider the case where x1 = 10 bowls and x2 = 20 mugs. This means that a total of 50 hours have been used for production, or 10 hours above the goal level of 40 hours. This extra 10 hours is overtime. Thus, d1- = (because there is no underutilization) and d1+ = 10 hours. In each of these two brief examples, at least one of the deviational variables equaled zero. In the first example, d1+ = 0, and in the second example, d2- = 0. This is because it is impossible to use fewer than 40 hours of labor and more than 40 hours of labor at the same time. Of course, both deviational variables, d1+ and d1+ could have equaled zero if exactly 40 hours were used in production. These examples illustrate one of the fundamental characteristics of goal programming: At least one or both of the deviational variables in a goal constraint must equal zero. The next step in formulating our goal programming model is to represent the goal of not using fewer than 40 hours of labor. We do this by creating a new form of objective function: minimize P1d1- The objective function in all goal programming models is to minimize deviation from the goal constraint levels. In this objective function, the goal is to minimize d 1-, the underutilization of labor. If d1- equaled zero, then we would not be using fewer than 40 hours of labor. Thus, it is our objective to make equal zero or the minimum amount possible. The symbol P1 in the objective function designates the minimization of d1- as the first-priority goal. This means that when this model is solved, the first step will be to minimize the value of d1- before any other goal is addressed. The fourth-priority goal in this problem is also associated with the labor constraint. The fourth goal, P4 reflects a desire to minimize overtime. Recall that hours of overtime are represented by d1+ the objective function, therefore, becomes As before, the objective is to minimize the deviational variable d1+. In other words, if d1+ equaled zero, there would be no overtime at all. In solving this model, the achievement of this fourth-ranked goal will not be attempted until goals one, two, and three have been considered. Profit Goal The second goal in our goal programming model is to achieve a daily profit of $1,600. Recall that the original linear programming objective function was Z = 40x1 + 50x2 Now we reformulate this objective function as a goal constraint with the following goal level: 145 The deviational variables d2- and d2+ represent the amount of profit less than $1,600 (d2-) and the amount of profit exceeding $1,600 (d2+). The pottery company’s goal of achieving $1,600 in profit is represented in the objective function as Notice that only d2- is being minimized, not d2+, because it is logical to assume that the pottery company would be willing to accept all profits in excess of $1,600 (i.e., it does not desire to minimize d2+, excess profit). By minimizing d2- at the second-priority level, the pottery company hope that d2- will equal zero, which will result in at least $1,600 in profit. Material Goal The third goal of the company is to avoid keeping more than 120 pounds of clay on hand each day. The goal constraint is Because the deviational variable d3-, represents the amount of clay less than 120 pounds, and d3+ represents the amount in excess of 120 pounds, this goal can be reflected in the objective function as The term P3d3+ represents the company’s desire to minimize excess of 120 pounds. The P3 designation indicates that it is the pottery company’s third most important goal. The complete goal programming model can now be summarized as follows: The one basic difference between this model and the standard linear programming model is that the objective function terms are not summed to equal a total value, Z. This is because the deviational variables in the objective function represent different units of measure. For example, d1- and d2+, represent hours of labor, d2- represents dollars, and d3+ represents pounds of clay. It would be illogical to sum hours, dollars, and pounds. The objective function in a goal programming model specifies only that the deviations from the goals represented in the objective function be minimized individually, in order of their priority. 146 Alternative Forms of Goal Constraints Let us now alter the preceding goal programming model so that our fourth-priority goal limits overtime to 10 hours instead of minimizing overtime. Recall that the goal constraint for labor is In this goal constraint, d1+ represents overtime. Because the new fourth-priority goal is to limit overtime to 10 hours, the following goal constraint is developed: Although this goal constraint looks unusual, it is acceptable in goal programming to have an equation with all deviational variables. In this equation, d4-, less than 10 hours, and d4+ represents the amount of overtime greater than 10 hours. Because the company desires to limit overtime to 10 hours, d4+ is minimized in the objective function: Now let us consider the addition of a fifth-priority goal to this example. Assume that the pottery company has limited warehouse space, so it can produce no more than 30 bowls and 20 mugs daily. If possible, the company would like to produce these numbers. However, because the profit for mugs is greater than the profit for bowls (i.e., $50 rather than $40), it is more important to achieve the goal for mugs. This fifth goal requires that two new goal constraints be formulated, as follows: Notice that the positive deviational variables d+ 5 and d+ 6 have been deleted from these goal constraints. This is because the statement of the fifth goal specifies that ―no more than 30 bowls and 20 mugs‖ can be produced. In other words, positive deviation, or overproduction, is not possible. Because the actual goal of the company is to achieve the levels of production shown in these two goal constraints, the negative deviational variables d5- and d6- are minimized in the objective function. However, recall that it is more important to the company to achieve the goal for mugs because mugs generate greater profit. This condition is reflected in the objective function, as follows: Because the goal for mugs is more important than the goal for bowls, the degree of importance should be in proportion to the amount of profit (i.e., $50 for each mug and $40 for each bowl). Thus, the goal for mugs is more important than the goal for bowls by a ratio of 5 to 4. The coefficients of 5 for P5d6- and 4 for P5d5- are referred to as weights. In other words, the minimization of d6- is ―weighted‖ higher than the minimization of d5- at the fifth priority level. When this model is solved, the achievement of the goal for minimizing (bowls) is more important, even though both goals are at the same priority level. 147 Notice, however, that these two weighted goals have been summed because they are at the same priority level. Their sum represents achievement of the desired goal at this particular priority level. The complete goal programming model, with the new goals for both overtime and production, is Graphical Interpretation of Goal Programming In Chapter 2, we analyzed the solution of linear programming models by using graphical analysis. Because goal programming models are linear, they can also be analyzed graphically. The original goal programming model for Beaver Creek Pottery Company, formulated at the beginning of this chapter, will be used as an example: To graph this model, the deviational variables in each goal constraint are set equal to zero, and we graph each subsequent equation on a set of coordinates, just as in Chapter 2. Figure 9.1 is a graph of the three goal constraints for this model. Notice that in Figure 9.1 there is no feasible solution space indicated, as in a regular linear programming model. This is because all three goal constraints are equations; thus, all solution points are on the constraint lines. Figure 9.1. Goal Constraints The solution logic in a goal programming model is to attempt to achieve the goals in the objective function, in order of their priorities. As a goal is achieved, the next highest-ranked 148 goal is then considered. However, a higher-ranked goal that has been achieved is never given up in order to achieve a lower-ranked goal. In this example, we first consider the first-priority goal, minimizing d1-. The relationship of d1- and d1+ to the goal constraint is shown in Figure 9.2. The area below the goal constraint line x1 + 2x2 = 40 represents possible values for d1-, and the area above the line represents values Figure 9.2. The first-priority goal: minimize d1- for d1+. In order to achieve the goal of minimizing d1- the area below the constraint line corresponding to d1- is eliminated, leaving the shaded area as a possible solution area. Next, we consider the second-priority goal, minimizing d2-. In Figure 9.3, the area below the constraint line 40x1 + 50x2 = 1,600 represents the values for d2- and the area above the line represents the values for d2+. To minimize d2-, the area below the constraint line corresponding d2- is eliminated. Notice that by eliminating the area for d2-, we do not affect the first-priority goal of minimizing d1-. Figure 9.3. The second priority goal: minimize d2-. Next, the third-priority goal, minimizing d3+, is considered. Figure 9.4 shows the areas corresponding to d3-and d3+. To minimize d3+, the area above the constraint line 4x1 + 3x2 = 120 149 Figure 9.4. The third-priority goal: minimize d3+ is eliminated. After considering the first three goals,we are left with the area between the line segments AC and BC, which contains possible solution points that satisfy the first three goals. Finally,we must consider the fourth-priority goal, minimizing d1+. To achieve this final goal, the area above the constraint line x1 + 2x2 = 40 must be eliminated. However, if we eliminate this area, then both d2– and d3- must take on values. In other words, we cannot minimize d1+ totally. without violating the first- and second-priority goals. Therefore, we want to find a solution pointthat satisfies the first three goals but achieves as much of the fourth-priority goal as possible. Point C in Figure 9.5 is a solution that satisfies these conditions. Notice that if we move down the goal constraint line 4x1 + 3x2 = 120 toward point D, d1+ is further minimized; however d2- takes on a value as we move past point C. Thus, the minimization of d1+ would be accomplished only at the expense of a higher-ranked goal. Figure 9.5. The fourth-priority goal (minimize d1+) and the solution The solution at point C is determined by simultaneously solving the two equations that intersect at this point. Doing so results in the following solution: 150 Because the deviational variables d1–, d2-, d3+ all equal zero, they have been minimized, and the first three goals have been achieved. Because d1+ = 15 hours of overtime, the fourth priority goal has not been achieved. The solution to a goal programming model such as this one is referred to as the most satisfactory solution rather than the optimal solution because it satisfies the specified goals as well as possible. Computer Solution of Goal Programming Problems with QM for Windows and Excel Goal programming problems can be solved using QM for Windows and Excel spreadsheets. In this section, we will demonstrate how to use both computer alternatives to solve the Beaver Creek Pottery Company example we solved graphically in the previous section. QM for Windows We will demonstrate how to solve a goal programming model by using our Beaver Creek Pottery Company example, which was formulated as follows: QM for Windows includes a goal programming module that can be accessed by clicking on the ―Module‖ button at the top of the screen. The model parameters are entered onto the data input screen as shown in Exhibit 9.1. Notice that each prioritized deviational variable must be assigned a weight, which for this problem is always one. The solution is obtained by clicking on the ―Solve‖ button at the top of the screen. The solution summary for our model is shown in Exhibit 9.2. Exhibit 9.1 151 Exhibit 9.2 QM for Windows will also provide a graphical analysis of a goal programming model. We click on the ―Windows‖ button and then select ―Graph‖ from the menu. The graph for our example is shown in Exhibit 9.3. Exhibit 9.3 Excel Spreadsheets Solving a goal programming problem by using Excel is similar to solving a linear programming model, although not quite as straightforward. Exhibit 9.4 shows the spreadsheet format for our Beaver Creek Pottery Company example. Cells G5, G6, and G7, under the heading ―Constraint Total,‖ contain the formulas for our goal constraints, including deviational variables. The formula for the labor constraint is shown on the formula bar at the top of the screen in Exhibit 9.4. The model decision variables are in cells B10 and B11, and the deviational variables are in cells E5:F7. The goals are established by setting the constraint formulas in G5 to G7 equal to the goal levels in cells I5 to I7. 152 Exhibit 9.4 When using a spreadsheet (or any regular linear programming program) to solve a goal programming problem, it must be solved sequentially. In this procedure, a new problem is formulated and solved for each priority goal in the objective function, beginning with the highest priority. Once a solution for this formulation is achieved, the value of the deviational variable that is the objective is added to the model as a constraint, and the second-priority deviational variable becomes the new objective. A new solution is achieved for each new objective sequentially until all the priorities are exhausted or it is clear that a better solution cannot be reached. For our purposes, this means editing Excel’s Solver for each new solution. Exhibit 9.5 shows the Solver Parameters window for our spreadsheet example. Recall that the first-priority goal for our model is the minimization of the negative deviational variable (d1-) for our labor goal. This deviational variable is located in cell E5; thus, we start Solver by minimizing cell E5. We identify B10:B11 (the decision variables) as well as E5:F7 (the deviational variables) as variables in the model. The model constraints are for our goals (i.e., G5:G7=I5:I7). Exhibit 9.5 The spreadsheet with the solution to our example problem is shown in Exhibit 9.6. We know this is the most satisfactory solution we can achieve from the QM for Windows solution, so it will not be necessary to perform any additional sequential steps. From the spreadsheet we can see that we have achieved all the goals except the fourth-priority goal, to minimize d1+. 153 Exhibit 9.6 However, if we had not achieved all our top goals so readily, the next step would be to include E5=0 as a constraint in Solver and then minimize our next-priority goal, which would be E6 (i.e. d2-) Next, we will use Excel to solve a slightly more complicated goal programming model— that is, the altered Beaver Creek Pottery Company example we developed at the beginning of this chapter, with goals for overtime and maximum storage levels for bowls and mugs: The spreadsheet for this modified version of our example is shown in Exhibit 9.7. The spreadsheet is set up much the same as the original version of this example, with the exception of the goal constraint for overtime. The formula for this goal constraint is included in cell G8 as =F5+E8-F8. In addition, the positive deviational variables for the last two goal constraints are now included in the formulas embedded in cells G9 and G10. For example, in cell G9 the constraint formula is C9*B13+E9. 154 Exhibit 7 The Solver Parameters window for this spreadsheet is shown in Exhibit 9.8, and the resulting solution is shown in Exhibit 9.9. Exhibit 9.8 Exhibit 9.9 You will notice that this solution achieves the first two priority goals for minimizing d1– and d2- which are in cells E5 and E6. However, the third-priority goal to minimize d3 + is not achieved because its cell (F7) has a value of 24 in it. Thus, we must follow the sequential approach to attempt to obtain a better solution. We accomplish this by including E=50 (the achievement of our first goal) as a constraint in Solver, as well as our second-priority goal that this solution achieved, E6=0 and then minimizing F7. This Solver Parameters screen is shown in Exhibit 155 9.10. Exhibit 9.10 The solution is shown in Exhibit 9.11. This is the same solution that we obtained for our original version of the model, without the alterations for overtime and production levels. This solution achieves the first three priority goals. We could continue to attempt to achieve the fourth- priority goal by including F70 as a constraint in Solver and minimizing cell F5 (i.e., d1+) ; however, doing so will not result in a better solution without sacrificing the goal achievement at the higher-priority levels. Thus, this is the best solution we can achieve. 156 Exhibit 9.11 The Analytical Hierarchy Process Goal programming is a method that provides us with a mathematical ―quantity‖ for the decision variables that best achieves a set of goals. It answers the question ―How much?‖ The analytical hierarchy process (AHP), developed by Thomas Saaty, is a method for ranking decision alternatives and selecting the best one when the decision maker has multiple objectives, or criteria, on which to base the decision. Thus, it answers the question ―Which one?‖ For example, someone buying a house might have several houses for sale from which to choose, the buyer might consider the cost, proximity of schools, trees, neighborhood, and public transportation when comparing several houses. AHP is aprocess for developing a numeric score to rank each decision alternative, based on how well each alternative meets the decision maker’s criteria. We will demonstrate AHP by using an example. Southcorp Development builds and operates shopping malls around the country. The company has identified three potential sites for its latest project, near Atlanta, Birmingham, and Charlotte. The company has identified four primary criteria on which it will compare the sites—(1) the customer market base (including overall market size and population at different age levels); (2) income level; (3) infrastructure (including utilities and roads); and (4) transportation (i.e., proximity to interstate highways for supplier deliveries and customer access). The overall objective of the company is to select the best site. This goal is at the top of the hierarchy of the problem. At the next (second) level of the hierarchy, we determine how the four criteria contribute to achieving the objective. At the level of the problem hierarchy, we determine how each of the three alternatives (Atlanta, Birmingham, and Charlotte) contributes to each of the four criteria. The general mathematical process involved in AHP is to establish preferences at each of these hierarchical levels. First, we mathematically determine our preferences for each site for each criterion. Then we determine our site preferences for income level, and so on. Next, we mathematically determine our preferences for the criteria—that is, which of the four criteria is most important, which is the next most important, and so on. Finally, we combine these two sets of preferences—for sites within each criterion and for the four criteria—to mathematically derive a score for each site, with the highest score being the best. 157 Pairwise Comparisons In AHP the decision maker determines how well each alternative ―scores‖ on a criterion by using pairwise comparisons. In a pairwise comparison, the decision maker compares two alternatives (i.e., a pair) according to one criterion and indicates a preference. These comparisons are made by using a preference scale, which assigns numeric values to different levels of preference. The standard preference scale used for AHP is shown in Table 9.1. This scale has been determined by experienced researchers in AHP to be a reasonable basis for comparing two items or alternatives. Each rating on the scale is based on a comparison of two items. For example, if the Atlanta site is ―moderately preferred‖ to the Birmingham site, then a value of 3 is assigned to this particular comparison. The rating of 3 is a measure of the decision maker’s preference for one of the alternatives over the other. If Southcorp compares Atlanta to Birmingham and moderately prefers Atlanta, resulting in a comparison value of 3 for the customer market criterion, it is not necessary for Southcorp to compare Birmingham to Atlanta to determine a separate preference value for this Table 9.1. Preference scale for pairwise comparisons ―opposite‖ comparison. The preference value of B for A is simply thereciprocal or inverseof the preference of A for B. Thus,in our example if the preference value of Atlanta forBirmingham is 3,the preference value of Birmingham for Atlanta is 1/3.Southcorp’s pairwise comparison ratings for each of the three sites for the customer market criterion are summarized in a matrix, a rectangular array of numbers. This pairwise comparison matrix will have a number of rows and columns equal to the decision alternatives: This matrix shows that the customer market in Atlanta (A) is equally to moderately pre-ferred (2) over the Charlotte (C) customer market, but Charlotte (C) is strongly preferred (5) over Birmingham (B). Notice that any site compared against itself, such as A compared to A, must be ―equally preferred,‖ with a preference value of 1. Thus, the values along the diagonal of our matrix must be 1s. 158 The remaining pairwise comparison matrices for the other three criteria—income level, infrastructure, and transportation—have been developed by Southcorp as follows: Developing Preferences Within Criteria The next step in AHP is to prioritize the decision alternatives within each criterion. For our site selection example, this means that we want to determine which site is the most preferred, the second most preferred, and the third most preferred for each of the four criteria. This step in AHP is referred to as synthesization. The mathematical procedure for synthesization is very complex and beyond the scope of this text. Instead, we will use an approximation method for synthesization that provides a reasonably good estimate of preference scores for each decision in each criterion. The first step in developing preference scores is to sum the values in each column of the pairwise comparison matrices. The column sums for our customer market matrix are shown as follows: Next, we divide each value in a column by its corresponding column sum. This results in a normalized matrix, as follows: Notice that the values in each column sum to 1. The next step is to average the values ineachrow. At this point,we convert the fractional values in the matrix to decimals,as shown inTable 9.2. The row average for each site is also shown in Table 9.2. Table 9.2 The row averages in Table 9.2 provide us with Southcorp’s preferences for the three sites for the customer market criterion. The most preferred site is Atlanta, followed by Charlotte; the least preferred site (for this criterion) is Birmingham. We can write these preferences as a matrix with 159 one column, which we will refer to as a vector: The preference vectors for the other decision criteria are computed similarly: These four preference vectors for the four criteria are summarized in a single preference matrix, shown in Table 9.3. Table 9.3. Criteria preference matrix Ranking the Criteria The next step in AHP is to determine the relative importance, or weight, of the criteria—that is, to rank the criteria from most important to least important. This is accomplished the same way we ranked the sites within each criterion: using pairwise comparisons. The following pairwise comparison matrix for the four criteria in our example was developed by using the preference scale in Table 9.1: The normalized matrix converted to decimals, with the row averages for each criterion, is shown in Table 9.4. Table 9.4. Normalized matrix for criteria, with row averages The preference vector, computed from the normalized matrix by computing the row averages in Table 9.4, is as follows: Clearly, income level is the highest-priority criterion, and customer market is second. Infrastructure and transportation appear to be relatively unimportant third- and fourth-ranked priorities in terms of the overall objective of determining the best site for the new shopping mall. The next step in AHP is to combine the preference matrices we developed for the sites for each criterion in Table 9.3 with the preceding preference vector for the four criteria. 160 Developing an Overall Ranking Recall that earlier we summarized Southcorp’s preferences for each site for each criterion in apreference matrix shown in Table 9.3and repeated as follows: In the previous section, we used pairwise comparisons to develop a preference vector forthe four criteria in our example: An overall score for each site is computed by multiplying the values in the criteria preference vector by the preceding criteria matrix and summing the products, as follows: The three sites, in order of the magnitude of their scores, result in the following AHP ranking: Based on these scores developed by AHP, Charlotte should be selected as the site for the new shopping mall, with Atlanta ranked second and Birmingham third. To rely on this result to make its site selection decision, Southcorp must have confidence in the judgments it made in the pairwise comparisons, and it must also have confidence in AHP. However, whether the AHP- recommended decision is the one made by Southcorp or not, following this process can help identify and prioritize criteria and enlighten the company about how it makes decisions. Following is a summary of the mathematical steps used to arrive at the AHP-recommended decision: 1. Develop a pairwise comparison matrix for each decision alternative (site) for each criterion. 2. Synthesization: a. Sum the values in each column of the pairwise comparison matrices. b. Divide each value in each column of the pairwise comparison matrices by the correspon-ding column sum—these are the normalized matrices. c. Average the values in each row of the normalized matrices—these are the preference vectors. d. Combine the vectors of preferences for each criterion (from step 2c) into one preference matrix that shows the preference for each site for each criterion. 3. Develop a pairwise comparison matrix for the criteria. 161 4. Compute the normalized matrix by dividing each value in each column of the matrix by the corresponding column sum. 5. Develop the preference vector by computing the row averages for the normalized matrix. 6. Compute an overall score for each decision alternative by multiplying the criteriapreference vector (from step 5) by the criteria matrix (from step 2d). 7. Rank the decision alternatives, based on the magnitude of their scores computed in step 6. AHP Consistency AHP is based primarily on the pairwise comparisons a decision maker uses to establish preferences between decision alternatives for different criteria. The normal procedure in AHP for developing these pairwise comparisons is for an interviewer to elicit verbal preferences from the decision maker, using the preference scale in Table 9.1. However, when a decision maker has to make a lot of comparisons (i.e.,three or more), he or she can lose track of previous responses. Because AHP is based on these responses,it is important that they be in some sense valid and especially that the responses be consistent. That is,a preference indicated for one set of pairwise comparisons needsto be consistent with another set of comparisons. Using our site selection example, suppose for the income level criterion Southcorp indicates that A is ―very strongly preferred‖ to B and that A is ―moderately preferred‖ to C. That’s fine, but then suppose Southcorp says that C is ―equally preferred‖ to B for the same criterion. That comparison is not entirely consistent with the previous two pairwise comparisons. To say that A is strongly preferred over B and moderately preferred over C and then turn around and say C is equally preferred to B does not reflect a consistent preference rating for the three sets of pairwise comparisons. A more logical comparison would be that C is preferred to B to some degree. This kind of inconsistency can creep into AHP when the decision maker is asked to make verbal responses for a lot of pairwise comparisons. In general, they are usually not a serious problem; some degree of slight inconsistency is expected. However, a consistency index can be computed that measures the degree of inconsistency in the pairwise comparisons. To demonstrate how to compute the consistency index (CI), we will check the consistency of the pairwise comparisons for the four site selection criteria. This matrix, shown as follows, is multiplied by the preference vector for the criteria: The product of the multiplication of this matrix and vector is computed as follows: Next, we divide each of these values by the corresponding weights from the criteria preference vector: If the decision maker,Southcorp,were a perfectly consistent decision maker,then each ofthese ratios would be exactly 4,the number of items we are comparing—in this case,four 162 criteria.Next,we average these values by summing them and dividing by 4: The consistency index, CI, is computed using the following formula: If CI = 0 then Southcorp would be a perfectly consistent decision maker. Because Southcorp is not perfectly consistent, the next question is the degree of inconsistency that is acceptable. An acceptable level of consistency is determined by comparing the CI to a random index, RI, which is the consistency index of a randomly generated pairwise comparison matrix. The RI has the values shown in Table 9.5, depending on the number of items, n, being compared. In our example, n = 4 because we are comparing four criteria. The degree of consistency for the pairwise comparisons in the decision criteria matrix is determined by computing the ratio of CI to RI: In general, the degree of consistency is satisfactory if CI/RI < 0.10, and in this case, it is. If CI/RI > 0.10, then there are probably serious inconsistencies, and the AHP results may not be meaningful. We would still have to evaluate the pairwise comparisons for each of the four individual criterion matrices before we could be sure the entire AHP for this problem was consistent. AHP with Excel Spreadsheets The spreadsheet includes the pairwise comparison matrix for our customer market criterion. Notice that the pairwise comparisons have been entered as fractions. We can enter fractions in Excel by dragging the cursor over the object cells, in this case B5:D7, and then clicking on ―Format‖ at the top of the screen. When the ―Format‖ menu comes down, we click on ―Cells‖ and then select ―Fraction‖ from the menu. 163 Exhibit 9.12 The sum for column A in the pairwise comparison matrix is obtained by inserting the formula =SUM(B5:B7) in cell B8. The sums for columns B and C can then be obtained by putting the cursor on cell B8, clicking on the right mouse button, and clicking on ―Copy.‖ Then we drag the cursor over cells B8:D8 and press the ―Enter‖ key. This will compute the sum for the other two columns. The cell values for the normalized matrix at the bottom of the spreadsheet in Exhibit 9.12 can be computed as follows. First, make sure you convert the cell values to decimal numbers. To start, divide the value in cell B5 by the column sum in cell B8 by typing =B5/B8 in cell B14. This results in the value 0.5455 in cell B14. Next, with the cursor on B14, click the right mouse button and then click on ―Copy.‖ Drag the cursor across cells B14:D14 and press the ―Enter‖ key. This will compute the values for cells C14 and D14. Repeat this process for each row to complete the normalized matrix. To compute the row averages from the normalized matrix, first type the formula =SUM(B14:D14)/3 in cell F14. This formula is displayed on the formula bar at the top of the spreadsheet. This will compute the value 0.5013 in cell F14. Next, with the cursor on cell F14, click the right mouse button and copy the formula in cell F14 to F15 and F16 by dragging the cursor across cells F14:F16 The next step in AHP is to develop the pairwise comparison matrix and normalized matrix for our four criteria. The spreadsheet to accomplish this step is shown in Exhibit 9.13. These two matrices are constructed in the same way as the two similar matrices in Exhibit 9.12. Exhibit 9.13 164 The final step in AHP is to develop an overall ranking. This requires Southcorp’s preference matrix for each site for all four criteria (Table 9.3) and the preference vector for the criteria, both of which are included in the spreadsheet shown in Exhibit 9.14. Exhibit 9.14 All the values in the two matrices in Exhibit 9.14 were entered as input from previous AHP steps (i.e., Exhibits 9.12 and 9.13). The score for Atlanta was computed using the formula embedded in cell C12 and shown on the formula bar. Similar formulas were used for the other two site scores in cells C13 and C14. Exhibit 9.15 shows the spreadsheet for evaluating the degree of consistency for the pairwise comparisons in the preference matrix for the four criteria. The spreadsheet is broken down into steps that are self-explanatory. Exhibit 9.15 Scoring Models For selecting among several alternatives according to various criteria, a scoring model is a method similar to AHP, but it is mathematically simpler. There are several versions of scoring models. In the scoring model that we will use, the decision criteria are weighted in terms of their relative importance, and each decision alternative is graded in terms of how well it satisfies the 165 criteria, according to the following formula: where wj = a weight between 0 and 1.00 assigned to criterion j, indicating its relative importance, where 1.00 is extremely important and 0 is not important at all. The sum of the total weights equals 1.00. gij = a grade between 0 and 100 indicating how well the decision alternative i satisfies criterion j, where 100 indicates extremely high satisfaction and 0 indicates virtually no satisfaction. Si = the total ―score‖ for decision alternative i, where the higher the score, the better. To demonstrate the scoring model, we will use an example. Sweats and Sweaters is a chain of stores specializing in cotton apparel. The company wants to open a new store in one of four malls around the Atlanta metropolitan area. The company has indicated five criteria that are important in its decision about where to locate: proximity of schools and colleges, area median income, mall vehicle traffic flow and parking, quality and size (in terms of number of stores in the mall), and proximity of other malls or shopping areas. The company has weighted each of these criteria in terms of its relative importance in the decision-making process, and it has analyzed each potential mall location and graded them according to each criterion as shown in the following table: The scores, SI, for the decision alternative are computed as follows: Because mall 4 has the highest score, it would be the recommended decision, followed by mall 3, mall 2, and finally mall 1. Scoring Model with Excel Solution Exhibit 9.16 shows a spreadsheet set up for the Sweats and Sweaters store location example. The formula that computes the score for mall 1 in cell D10, =SUMPRODUCT (C5:C9, D5:D9) is shown on the formula bar at the top of the spreadsheet. This formula is copied and pasted using the right mouse button into cells E10, F10, and G10. 166