09-eai.pdf
Document Details
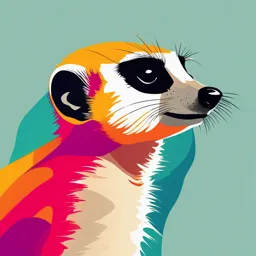
Uploaded by PureToad
Tags
Full Transcript
Prof. Dr. rer. nat. Anne Lauscher Ethics and Modern AI Lecture 10: AI for Social Good Trigger warning: this presentation contains content which might be offensive to some listeners. Organizational Notes Our journey is ending soon! • Start with the exam preparation if you havn’t done so yet! •...
Prof. Dr. rer. nat. Anne Lauscher Ethics and Modern AI Lecture 10: AI for Social Good Trigger warning: this presentation contains content which might be offensive to some listeners. Organizational Notes Our journey is ending soon! • Start with the exam preparation if you havn’t done so yet! • Important: • next session (July 10th) will be online! • we will have a recap and answer questions • For this: Collect questions until Friday 7th! Recap Chris asks Apple’s Siri Issues? • • • • • Fairness and Trust Privacy and Data Protection Dual Use and Misuse Transparency Environmental Aspects Biases in the Training Data The man has a Neural Network The woman has a Prediction family … career Prediction family … career Truth family … career Truth family … career Source: https://www.reuters.com/article/us-amazon-com-jobs-automation-insight/amazon-scraps-secret-ai-recruiting-toolthat-showed-bias-against-women-idUSKCN1MK08G General Data Protection Regulation (GDPR) EU regulation on data protection and privacy https://eur-lex.europa.eu/legal-content/EN/TXT/?uri=CELEX%3A02016R067920160504 “on the protection of natural persons with regard to the processing of personal data and on the free movement of such data” • fundamental rights and freedoms of natural persons • in particular their right to the protection of personal data applies to the processing of personal data wholly or partly by automated means Annotation is just providing labels,… Yes, but • Workers influence the outcome, and thus potential biases you might see in your training data • What are the workers’ conditions? • Payment by mimimum wage? • Potentially Harmful content? Dual-Use Items Dual-use items are goods, software and technology that can be used for both civilian and military applications. (EU Trade Policy) More generally: A good or technology that can be used to to satisfy more than one goal at the same time (e.g., do good or be misused). Military Uses of AI War is inherently controversial Military use of AI is so, too – esp. lethal autonomous weapons systems Capable of autonomously making life and death decisions regarding human targets Examples • Patriot missile system • AEGIS naval weapons system • Phalanx weapons system • Israeli Harpy Anthropomorphism attributing human characteristics or behaviour to non-human entities, e.g. animals or objects parallel with humanness? Rather than a single factor which makes humans human, Scruton (2017, (p. 31)) argues that humanity is emergent: each individual element does not make a human but collectively they make up the language of humanness. Why could that be good? • • • • hedonic motivation – more fun! can increase user engagement (Wagner et al., 2019) can increase reciprocity (Fogg and Nass, 1997) could remedy loneliness (Stupple-Harris, 2021)? Why could that be problematic? Being unfamiliar with the internal states of machines can lead to assuming they have similar internal states of desires and feelings Various ethical risks • • • • • unidirectional emotional bonding leading to misplaced feelings misplaced trust, potential for deception positive feelings can be confused with friendship disappointment, psychological issues people might hold AI for morally accountable Neural Networks are blackboxes • A single neuron is, in the simplest case, just defined by a weight vector (cf. logistic regression) • If I want to understand which features determined the system output, l can look at the weights • However, neural networks are • collection of neurons with non-linear activations • several hidden layers is leading • and thus, difficult to interpret https://fridaysforfuture. org/what-we-do/ourdemands/ GPT4: 170 trillion? https://arxiv.org/pdf /1910.01108.pdf Luccioni, Alexandra Sasha, and Alex Hernandez-Garcia. "Counting carbon: A survey of factors influencing the emissions of machine learning." arXiv preprint arXiv:2302.08476 (2023). Estimating Carbon Emissions • Measurement in CO2 equivalents • The amount of CO2eq (𝐶) emitted during model training can be decomposed into three relevant factors: – the power consumption of the hardware used (𝑃), – the training time (𝑇), – and the carbon intensity of the energy grid (𝐼); • or equivalently, the energy consumed (𝐸) and the carbon intensity 𝐶=𝑃×𝑇×𝐼=𝐸×𝐼 Example Model trained on • a single GPU consuming 300 W (P) • for 100 hours (T) • on a grid that emits 500 gCO2eq/kWh (I) 0.3 kW × 100 h × 500 g/kWh = 15000 g = 15 kg of CO2eq What can we do? • • • Fostering Awareness • Reporting The Environmental Impact • Do we always need big models? Running less experiments • Which ones are necessary? • Sometimes difficult, especially in a research context Developing efficient methods • Researching environmentally sustainable methods • Using those in practise Efficient Methods Parameter Efficiency: Reduce the number of parameters • Adapter Layers (Training Time) • Distillation (Inference Time) • Pruning (Inference Time) Data Efficiency: Use fewer examples or make better use of available examples • • • • Data Filtering Active Learning Curriculum Learning Zero-Shot & Few-Shot LEarning https://mlc o2.github.io /impact/ Questions? After this lecture, you will … • Know about projects and initiatives related to AI for social good • Understand some of the challenges in this area and how to deal with those Learning goals So far, we have looked at many ethical issues AI might be causing and how to mitigate those … But what if … AI could actually be used for something “good”? Definition AI4SG =def. the design, development, and deployment of AI systems in ways that (i) prevent, mitigate or resolve problems adversely affecting human life and/or the wellbeing of the natural world, and/or (ii) enable socially preferable and/or environmentally sustainable developments. https://link.springer.com/article/10.1007/s11948-020-00213-5 Which applications have you heard of/ can you imagine? Example Projects Cassava • supports 75% of all farmers livelihoods in Uganda • important for food security • pests can cause up to 80 percent crop loss • Monitoring of diseases is essential • Problem: limited access to experts https://farmersreviewafrica. com/cassava-diseasehaunts-zambia/ Food Disease Tracking https://www.yo utube.com/watc h?v=ZX5xHzF_Q VI Human Disease Tracking https://www.youtub e.com/watch?v=K8Vh l194ikA Climate Anomalies Tracking https://www.youtube.com/watch?v =LfM8UMkuD20 Cyclone Monitoring https://www.youtube.co m/watch?v=ThNBSNf5Sg o&list=PLXK0SW9W7VG Ao3iFRe8swNTKrY_StQO 9X&index=11 Natural Disasters Management https://www.youtube.com /watch?v=iKn3aRm8DxE&l ist=PLXK0SW9W7VGAo3iF Re8swNTKrY_StQO9X&ind ex=6 Financial Inclusion https://www.youtube.com/wa tch?v=Lhw44jx1xQw&list=PLX K0SW9W7VGAo3iFRe8swNTKr Y_StQO9X&index=14 News Analysis https://www.youtube.com/w atch?v=knjhlrweFk&list=PLXK0SW9W7 VGAo3iFRe8swNTKrY_StQO9X &index=18 Social Media Analysis https://www.youtube.com/ watch?v=DY2fB_fusEM&list =PLXK0SW9W7VGAo3iFRe8 swNTKrY_StQO9X&index=3 Predicting Poverty https://youtu.be /DafZSeIGLNE Language Technology 4 Social Good • News and social media analysis to measure discussions about socially relevant topics • Hate speech detection/ detection of harmful content • Automatic translation • ChatBots for healthcare (patient support, health counseling, disease diagnosis, etc.) • Fact checking • Educational Applications • Automatic text simplification • … Automatic Text Simplification Task: make text “simpler” Why: foster inclusion, e.g., for non-native speakers, individuals with cognitive disabilities, elderly, etc. How: depends on the exact simplification aspect, e.g., lexical simplification (easier words), syntactic simplification (reduce complexity, length) Automatic Text Simplification Example From: Also contributing to the firmness in copper, the analyst noted, was a report by Chicago purchasing agents, which precedes the full purchasing agents report that is due out today and gives an indication of what the full report might hold. (two relative clauses and one conjoined verb phrase, example from Siddharthan (2006)) To: Also contributing to the firmness in copper, the analyst noted, was a report by Chicago purchasing agents. The Chicago report precedes the full purchasing agents report. The Chicago report gives an indication of what the full report might hold. The full report is due out today. Landscape of NLP4SG https://aclanthology.org/2021.nlp4p osimpact-1.3.pdf (Literature overview) Initiatives Charities https://www.d atakind.org/our -story Charities https://ai4good.org/ about-us/ AI for Good’s data catalog Research paper: “Hidden in plain sight”: https://ai4good.org/wpcontent/uploads/2021/04/Hiddenin-Plain-Sight-SDG-DataCatalogue-Paper.pdf Catalog: https://ai4good.org/whatwe-do/sdg-data-catalog/ Academic Programs https://grad.uchicago.ed u/fellowship/datascience-for-social-goodsummer-fellowship/ Research Workshops https://aiforsocialgood. github.io/neurips2019/ Research Workshops https://sites.google.com/view/nlp4positiveimpact2021 Cooperate Funding Programs https://impactchallenge.withgoogle.com/techforsocialgood Meta-initiatives ITU AI Repository https://www.itu.int/en/IT U-T/AI/Pages/airepository.aspx Challenges for AI4SG Challenges Tech culture • moving fast and breaking things while iterating towards solutions • lack of familiarity with the non-technical aspects • homogeneous sociodemographic structure Dual Use Interdisciplinary collaboration … “Good-AI-gone-bad” scenarios Failure of IBM’s oncology-support software: attempts to use machine learning to identify cancerous tumours, but which was rejected by medical practitioners “on the ground” (Ross and Swetlitz 2017). Problem: trained using synthetic data, not sufficiently refined to interpret ambiguous, nuanced, or otherwise “messy” patient health records (Strickland 2019). Reliance on US medical protocols (not applicable worldwide) Result: • misdiagnoses • erroneous treatment suggestions • breaching the trust of doctors Factors for successful AI4SG (1) Falsifiability (e.g., possibility of empirical testing) and incremental deployment; (2) safeguards against the manipulation of predictors (e.g., manipulation of input data); (3) receiver-contextualised intervention (should respect autonomy) (4) receiver-contextualised explanation and transparent purposes; (5) privacy protection and data subject consent; (6) situational fairness; (7) human-friendly semanticisation (how and what meaning we make out of it) https://link.springer.com/article/10.1007/s11948-020-00213-5 Other considerations Alternative approaches could be better! (“Not AI for Social Good”) We should not forget the risks! (”AI for Insufficient Social Good”) AI is no silver bullet! (“Only AI for Social Good”) • We are generally dealing with very complex scenarios • Generally, AI cannot solve complex social issues https://link.springer.com/article/10.1007/s11948-020-00213-5 Guidelines for AI4SG collaborations • Expectations of what is possible with AI need to be well-grounded. • There is value in simple solutions. • Applications of AI need to be inclusive and accessible, and reviewed at every stage for ethics and human rights compliance. • Goals and use cases should be clear and well-defined. • Deep, long-term partnerships are required to solve large problems successfully. • Planning needs to align incentives, and factor in the limitations of both communities. https://www.nature.com/articles/s41467-020-15871-z Guidelines for AI4SG collaborations • • • • Establishing and maintaining trust is key to overcoming organisational barriers. Options for reducing the development cost of AI solutions should be explored. Improving data readiness is key. Data must be processed securely, with utmost respect for human rights and privacy. https://www.nature.com/articles/s41467-020-15871-z Example: Troll Patrol UN SDGs: gender equality (5), strong institutions (6) Overall idea: foster democracy and gender inclusion through providing a safe online space for women to participate in opinion exchange Partners: Amnesty International & Element AI AI Approach: • Quantify online abuse against women • 6500 volunteers analyzed 288,000 tweets sent to 778 women politicians and journalists in the UK and USA in 2017 • Result: • 1.1 million toxic tweets • black women being 84% more likely than white women to experience abuse Questions? Now, you … • Know about projects and initiatives related to AI for social good • Understand some of the challenges in this area and how to deal with those Learning goals Now, you … • Know about projects and initiatives related to AI for social good • Understand some of the challenges in this area and how to deal with those Next: Recap