Constraint Satisfaction Problems PPT
Document Details
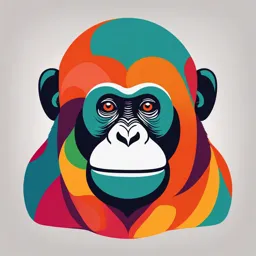
Uploaded by SmarterRococo9687
Tags
Summary
This document is a presentation about Constraint Satisfaction Problems (CSPs). It covers topics such as search, backtracking, constraint propagation, and local research.
Full Transcript
CS 106: Artificial Intelligence Constraint Satisfaction Problems Instructor: Ngoc-Hoang LUONG, PhD University of Information Technology (UIT), VNU-HCM [These slides were adapted from the slides created by Dan Klein and Pieter Abbeel for CS188 Intro to...
CS 106: Artificial Intelligence Constraint Satisfaction Problems Instructor: Ngoc-Hoang LUONG, PhD University of Information Technology (UIT), VNU-HCM [These slides were adapted from the slides created by Dan Klein and Pieter Abbeel for CS188 Intro to AI at UC Berkeley. http://ai.berkeley.edu.] What is Search For? Assumptions about the world: a single agent, deterministic actions, fully observed state, discrete state space Planning: sequences of actions The path to the goal is the important thing Paths have various costs, depths Heuristics give problem-specific guidance Identification: assignments to variables The goal itself is important, not the path All paths at the same depth (for some formulations) CSPs are specialized for identification problems Constraint Satisfaction Problems Constraint Satisfaction Problems Constraint satisfaction problems (CSPs): A special subset of search problems State is defined by variables X with i values from a domain D (sometimes D depends on i) Goal test is a set of constraints specifying allowable combinations of values for subsets of variables Allows useful general-purpose algorithms with more power than standard search algorithms Constraint Satisfaction Problems A constraint satisfaction problem (CSP) is a tuple where: is the set of variables. is the set of domains. is a set of constraints. For example, is a CSP where: Variables are: Domains are: There is a single constraint: https://www.cs.upc.edu/~erodri/webpage/cps/cps.html Constraint Graphs Constraint Graphs Binary CSP: each constraint relates (at most) two variables Binary constraint graph: nodes are variables, arcs show constraints General-purpose CSP algorithms use the graph structure to speed up search. CSP Examples Example: Map Coloring Variables: Domains: Constraints: adjacent regions must have different colors Implicit 𝐻𝐶𝑀 ≠𝑇𝑁 : Explicit: ( 𝐻𝐶𝑀 , 𝑇𝑁 ) ∈ {( 𝑟𝑒𝑑 , 𝑔𝑟𝑒𝑒𝑛 ) , ( 𝑟𝑒𝑑, 𝑏𝑙𝑢𝑒 ) , …} Solutions are assignments satisfying all constraints, e.g.: Example: N-Queens Variables: ♛ Domains: ♛ ♛ Constraints: ♛ Implicit: ♛ Explicit: ♛ ♛ Example: Sudoku Variables: Each (open) square Domains: {1,2,…,9} Constraints: 9-way alldiff for each column 9-way alldiff for each row 9-way alldiff for each region Varieties of CSPs and Constraints Varieties of CSPs Discrete Variables Finite domains Size d means O(dn) complete assignments E.g., Boolean CSPs, including Boolean satisfiability (NP-complete) Infinite domains (integers, strings, etc.) E.g., job scheduling, variables are start/end times for each job Continuous variables E.g., start/end times for Hubble Telescope observations Varieties of Constraints Varieties of Constraints Unary constraints involve a single variable (equivalent to reducing domains), e.g.: Binary constraints involve pairs of variables, e.g.: Higher-order constraints involve 3 or more variables: e.g., Sudoku constraints Preferences (soft constraints): E.g., red is better than green Often representable by a cost for each variable assignment Gives constrained optimization problems Real-World CSPs Assignment problems: e.g., who teaches what class Timetabling problems: e.g., which class is offered when and where? Hardware configuration Transportation scheduling Factory scheduling Circuit layout Fault diagnosis … lots more! Many real-world problems involve real-valued variables… Solving CSPs Standard Search Formulation Standard search formulation of CSPs States defined by the values assigned so far (partial assignments) Initial state: the empty assignment, {} Successor function: assign a value to an unassigned variable Goal test: the current assignment is complete and satisfies all constraints We’ll start with the Search Methods What would BFS do? ♛ ♛ What would DFS do? ♛ ♛ What problems does naïve search have? BFS? ♛ ♛ ♛ ♛ ♛ … ♛ ♛ ♛ ♛ ♛ ♛ … … … … ♛ … ♛ ♛ ♛ ♛ ♛ ♛ ♛ … ♛ ♛ … ♛ ♛ … … … ♛ ♛ ♛ … ♛ ♛ ♛ ♛ ♛ ♛ ♛ ♛ ♛ ♛ ♛ ♛ ♛ … ♛ ♛ ♛ … ♛ ♛ ♛ … ♛ ♛ ♛ …… ♛ ♛ ♛ … ♛ ♛ ♛ …… ♛ ♛ ♛ DFS? ♛ ♛ ♛ ♛ ♛ … ♛ ♛ ♛ ♛ ♛ ♛ ♛ ♛ …… ♛ ♛ … ♛ ♛ ♛ ♛ ♛ ♛ ♛ ♛ ♛ ♛ ♛ … ♛ ♛ ♛ ♛ … ♛ … ♛ ♛ ♛ ♛ ♛ ♛ ♛ ♛ … ♛ ♛♛ ♛ ♛ ♛ ♛ ♛ ♛ ♛ ♛ ♛ … ♛ ♛ ♛ ♛ ♛ ♛ ♛ ♛ Backtracking Search Backtracking Search Backtracking search is the basic uninformed algorithm for solving CSPs Idea 1: One variable at a time Only need to consider assignments to a single variable at each step Idea 2: Check constraints as you go i.e. consider only values which do not conflict previous assignments Might have to do some computation to check the constraints “Incremental goal test” Depth-first search with these two improvements is called backtracking search (not the best name) Can solve n-queens for n 25 Backtracking Example ♛ ♛ ♛ ♛ ♛ ♛ ♛ ♛ ♛ ♛ ♛ ♛ ♛ ♛ ♛ ♛ ♛ ♛ ♛ ♛ Map Coloring with Backtracking Where are we now? https://vi.wikipedia.org/ Map Coloring with Backtracking https://camranhcitygate.info/ Backtracking Example Backtracking Search Backtracking = DFS + variable-ordering + fail-on- violation What are the choice points? https://www.dcc.fc.up.pt/~ines/aulas/1617/IA/cspNew.pdf Improving Backtracking General-purpose ideas give huge gains in speed Filtering: Can we detect inevitable failure early? Ordering: Which variable should be assigned next? In what order should its values be tried? Structure: Can we exploit the problem Filtering Filtering: Forward Checking Filtering: Keep track of domains for unassigned variables and cross off bad options Forward checking: Cross off values that violate a constraint when added to the existing assignment Filtering: Forward Checking Vạn Ninh Ninh Hòa Khánh Vĩnh Diên Khánh Nha Trang Cam Lâm Khánh Sơn Cam Ranh Trường Sa Filtering: Constraint Propagation Forward checking propagates information from assigned to unassigned variables, but doesn't provide early detection for all failures: Vạn Ninh Ninh Hòa Khánh Vĩnh Diên Khánh Nha Trang Cam Lâm Khánh Sơn Cam Ranh Trường Sa Ninh Hòa and Diên Khánh cannot both be green! Why didn’t we detect this yet? Constraint propagation: reason from constraint to constraint Consistency of A Single Arc An arc X Y is consistent iff for every x in the tail there is some y in the head which could be assigned without violating a constraint Vạn Ninh Ninh Hòa Khánh Vĩnh Diên Khánh Nha Trang Cam Lâm Khánh Sơn Cam Ranh Trường Sa Delete from the tail! Forward checking: Enforcing consistency of arcs pointing to each new assignment Consistency of A Single Arc removes values from without support in. https://www.cs.upc.edu/~erodri/webpage/cps/cps.html Arc Consistency of an Entire CSP A simple form of propagation makes sure all arcs are consistent: Vạn Ninh Ninh Hòa Khánh Vĩnh Diên Khánh Nha Trang Cam Lâm Khánh Sơn Cam Ranh Trường Sa Remember: Delete from the tail! Important: If X loses a value, neighbors of X need to be rechecked! Arc consistency detects failure earlier than forward checking Can be run as a preprocessor or after each assignment What’s the downside of enforcing arc consistency? Enforcing Arc Consistency in a CSP https://www.cs.upc.edu/~erodri/webpage/cps/cps.html Limitations of Arc Consistency After enforcing arc consistency: Can have one solution left Can have multiple solutions left Can have no solutions left (and not know it) What went wrong here? Arc consistency still K-Consistency Increasing degrees of consistency 1-Consistency (Node Consistency): Each single node’s domain has a value which meets that node’s unary constraints 2-Consistency (Arc Consistency): For each pair of nodes, any consistent assignment to one can be extended to the other K-Consistency: For each k nodes, any consistent assignment to k-1 can be extended to the kth node. Higher k more expensive to compute (You need to know the k=2 case: arc consistency) Improving Backtracking General-purpose ideas give huge gains in speed Filtering: Can we detect inevitable failure early? Ordering: Which variable should be assigned next? In what order should its values be tried? Structure: Can we exploit the problem Ordering Ordering: Minimum Remaining Values Variable Ordering: Minimum remaining values (MRV): Choose the variable with the fewest legal left values in its domain Vạn Ninh Ninh Hòa Khánh Vĩnh Diên Khánh Nha Trang Cam Lâm Khánh Sơn Cam Ranh Trường Sa Ordering: Minimum Remaining Values Variable Ordering: Minimum remaining values (MRV): Choose the variable with the fewest legal left values in its domain Why min rather than max? Also called “most constrained variable” “Fail-fast” ordering Ordering: Least Constraining Value Value Ordering: Least Constraining Value Given a choice of variable, choose the least constraining value (i.e., the one that rules out the fewest values in the remaining variables). Vạn Ninh Ninh Hòa Khánh Vĩnh Diên Khánh Nha Trang Cam Lâm Khánh Sơn Cam Ranh Trường Sa Ordering: Least Constraining Value Value Ordering: Least Constraining Value Given a choice of variable, choose the least constraining value I.e., the one that rules out the fewest values in the remaining variables Note that it may take some computation to determine this! (E.g., rerunning filtering) Why least rather than most? Combining these ordering ideas makes Improving Backtracking General-purpose ideas give huge gains in speed Filtering: Can we detect inevitable failure early? Ordering: Which variable should be assigned next? In what order should its values be tried? Structure: Can we exploit the problem Structure Problem Structure Extreme case: independent subproblems Example: Tasmania and mainland do not interact Independent subproblems are identifiable as connected components of constraint graph Suppose a graph of n variables can be broken into subproblems of only c variables: Worst-case solution cost is O((n/c)(dc)), linear in n E.g., n = 80, d = 2, c =20 280 = 4 billion years at 10 million nodes/sec (4)(220) = 0.4 seconds at 10 million nodes/sec Tree-Structured CSPs Theorem: if the constraint graph has no loops, the CSP can be solved in O(n d2) time Compare to general CSPs, where worst-case time is O(dn) Tree-Structured CSPs Improving Structure Nearly Tree-Structured CSPs Conditioning: instantiate a variable, prune its neighbors' domains Cutset conditioning: instantiate (in all ways) a set of variables such that the remaining constraint graph is a tree Choose a cutset Cutset Conditioning Instantiate the cutset (all possible Solve the residual ways) CSPs (tree Compute residual structured) CSP for each assignment Cutset Conditioning Vạn Ninh Ninh Hòa Khánh Vĩnh Diên Khánh Nha Trang Cam Lâm Khánh Sơn Cam Ranh Trường Sa Tree-Structured CSP Algorithm for tree-structured CSPs: Order: Choose a root variable, order variables so that parents precede children Vạn Ninh Ninh Hòa Nha Trang Khánh Vĩnh Khánh Sơn Cam Ranh Remove backward: For i = n : 2, apply Remove- Inconsistency(Parent(Xi),Xi) Assign forward: For i = 1 : n, assign Xi consistently with Parent(Xi) Tree-Structured CSP Iterative Improvement Iterative Algorithms for CSPs Local search methods typically work with “complete” states, i.e., all variables assigned To apply to CSPs: Take an assignment with unsatisfied constraints Operators reassign variable values No fringe! Live on the edge. Algorithm: While not solved, Variable selection: randomly select any conflicted variable Value selection: min-conflicts heuristic: Choose a value that violates the fewest constraints I.e., hill climb with h(n) = total number of violated constraints Example: 4-Queens ♛ ♛ ♛ ♛ ♛ ♛ 2 1 0 ♛ ♛ ♛ ♛ ♛ ♛ ♛ ♛ ♛ ♛ 2 0 1 ♛ ♛ 0 3 1 ♛ ♛ ♛ ♛ ♛ ♛ ♛ ♛ ♛ ♛ Performance of Min-Conflicts Given random initial state, can solve n-queens in almost constant time for arbitrary n with high probability (e.g., n = 10,000,000)! The same appears to be true for any randomly-generated CSP except in a narrow range of the ratio Summary: CSPs CSPs are a special kind of search problem: States are partial assignments Goal test defined by constraints Basic solution: backtracking search Speed-ups: Ordering Filtering Structure Iterative min-conflicts is often effective in practice Local Search Local Search Tree search keeps unexplored alternatives on the fringe (ensures completeness) Local search: improve a single option until you can’t make it better New successor function: local changes Generally much faster and more memory efficient (but incomplete and suboptimal) Pretty much unavoidable when the state is “yourself” Hill Climbing Simple, general idea: Start wherever Repeat: move to the best neighboring state If no neighbors better than current, quit Hill Climbing 64 Hill Climbing Diagram Hill Climbing Quiz Starting from X, where do you end up ? Starting from Y, where do you end up ? Starting from Z, where do you end up ? Simulated Annealing Idea: Escape local maxima by allowing downhill moves But make them rarer as time goes on 67 Simulated Annealing Theoretical guarantee: Stationary distribution (Boltzmann): If T decreased slowly enough, will converge to optimal state! Is this an interesting guarantee? Sounds like magic, but reality is reality: The more downhill steps you need to escape a local optimum, the less likely you are to ever make them all in a row “Slowly enough” may mean exponentially slowly Random restart hillclimbing also converges to optimal state… Genetic Algorithms Genetic algorithms use a natural selection metaphor Keep best N hypotheses at each step (selection) based on a fitness function Also have pairwise crossover operators, with optional mutation to give variety Possibly the most misunderstood, misapplied (and even maligned) technique around Example: N-Queens Why does crossover make sense here? When wouldn’t it make sense? What would mutation be? What would a good fitness function be?