Computer Vision Lecture Notes PDF
Document Details
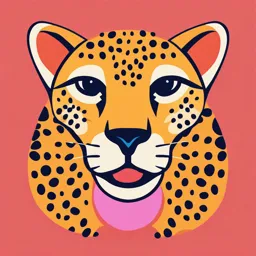
Uploaded by ReadableSanity6589
Benha University
Dr. Ahmed Taha
Tags
Summary
These lecture notes from Benha University provide a comprehensive overview of computer vision, including low-level, mid-level, and high-level aspects. The document also delves into human vision and related topics. It's intended as a learning resource.
Full Transcript
Computer Vision By Dr. Ahmed Taha Lecturer, Computer Science Department, Faculty of Computers & Artificial Intelligence, Benha University 1 Human Vision Lecture Two 2 3 4 Low-Level Visio...
Computer Vision By Dr. Ahmed Taha Lecturer, Computer Science Department, Faculty of Computers & Artificial Intelligence, Benha University 1 Human Vision Lecture Two 2 3 4 Low-Level Vision Photo manipulation - Size - Color - Exposure - X-Pro II Feature extraction - Edges - Oriented gradients - Segments 5 Mid-Level Vision Image Image - Panoramas Image World - Multi-view stereo - Structure from motion - Structured light - LIDAR (Light Detection and Ranging) Image Time - Optical flow - Time lapse 6 High-Level Vision Image Semantics! - Image classification - Object detection - Segmentation Applications - Retrieval - Robots? - and…???? 7 Is vision easy or hard for humans? - Discuss with your neighbors - 2 minutes - Report back 8 Human Vision 9 Human Vision 10 11 Why do things have eyes? - To see other things! - Visual stimulus is an important signal - Started as photoreceptive protein (eyespots) 12 Eyespots - the beginning of vision Simple eyes, named eyespots, are photosensitive proteins with no other surrounding structure 13 Eyespots - the beginning of vision Simple eyes, named eyespots, are photosensitive proteins with no other surrounding structure 14 Eyespots - the beginning of vision - Eyespots are sensitive to ambient light - Just rough direction: - Euglena swim towards light for better photosynthesis - Snails move away from light - No nerves, brain, or processing - Very low acuity (light from many directions all hits same sensitive area) - Started EVOLVING… 15 Pit eyes - the first eyes? - Photosensitive cells in pits - Block some light - More information about where light direction - Very common - Evolved 40-65 times - 28 of 33 animal phyla have them - Very simple, low acuity 16 Simple -> complex eyes 17 Eyespots - no acuity 18 Pit eyes - some acuity 19 Complex eyes - high acuity 20 Refraction: more light + acuity! 21 Focussing: changing refraction 22 Complex eyes - a huge advantage - Many different styles, mechanisms - >= 10, accounting for many of the ways our cameras work now - Same goal: better visual acuity (resolution) - Rare: 6 of 33 animal phyla - Beneficial: 96% of known species - Is it all because of the eyes?? - Image forming - high enough acuity to perceive shapes, objects, etc. 23 So how do human eyes work? - Complex! - Light passes through - Cornea, humours, lens refract light to focus - Hit the retina - Absorbed by photosensitive cells - Info transmitted through optic nerve, processed by visual cortex 24 Human Vision 25 How do we process this light? - Hit photoreceptive cells (rods and cones) - ~120 million of them in retina - Not all the same, not evenly distributed 26 27 Humans Octopus Retina Retina Nerves Nerves Blind spot Optic Optic Nerve Nerve 28 Rods - low light, monochrome vision - ~120 million - Sensitive to 1 photon - Can pool responses - Slow response time - Only operate in low-light conditions - Saturate quickly in lots of light - Take ~7 minutes to adjust (night vision) 29 Cones - detailed, color vision - ~6 million - Need many photons to activate, bright light - Fast response time - Fine details - Fast changes over time - Responsible for most daytime vision - Mostly packed into one region: Fovea 30 Fovea: where it’s all happening - Small circle on the retina, 1.5mm - Densely packed with cones - 200,000 cones/mm2 - Highest visual acuity - Reading: move your eyes so the text is centered in fovea 31 Peripheral vision: don’t get eaten - Few cones - Low acuity - Low perception of color - Lots of rods, good at night - At night: look at stars straight on vs slightly next to them. Brighter when you don’t look right at them! 32 Peripheral vision: don’t get eaten 33 Photoreceptors need change! 34 Fixational eye movement - Receptors adjust, lose sensitivity over time - Eye keeps moving to expose new parts to light - Microsaccades - Short linear movement - Sporadic - Ocular drift - Constant slow movement - Microtremors - Tiny vibrations - Synchronized between eyes - For seeing fine details 35 36 Brains (maybe) came after eyes! - Animals that rely on visual input but not brains - Eyes connect straight to muscle tissue - Some jellyfish - No reason to have a brain without sensory input - Brains Eyes coevolve - As the eyes get more complex, visual cortex expands - So maybe the reason you have a brain is because of your eyes! 37 Ganglia transmit info to brain - ~ 1 million of them - Different ganglia connect to different kinds of photoreceptors, sensitive to different things - M cells: depth, movement, orientation/position of objects - P cells: color, shape, fine details 38 Ganglia transmit info to brain 39 Visual cortex interprets data - More than 30 different substructures in the brain for processing visual data 40 Visual cortex interprets data - V1: primary visual cortex - Edge detection - Highly spatially sensitive - V2: secondary visual cortex - Size, color, shape, possibly memory - Sends signals onward to V3, V4, V5 - Sends strong feedback back to V1 - Many of these system functions are not well understood 41 Visual cortex is split (maybe) - Ventral/dorsal hypothesis - Information goes through V1 and V2 - Splits into streams for different purposes Dorsal Stream Primary Visual Cortex Ventral Stream 42 Ventral vs dorsal stream Factor Ventral system Dorsal system Function Recognition/identification Visually guided behaviour Sensitivity High spatial frequencies - High temporal frequencies - details motion Memory Long term stored Only very short-term storage representations Speed Relatively slow Relatively fast Consciousness Typically high Typically low Frame of reference Allocentric or object-centered Egocentric or viewer-centered Visual input Mainly foveal or parafoveal Across retina Monocular vision Generally reasonably small Often large effects e.g. effects motion parallax 43 What does this split mean? - Recognition and action are split! - Damage to dorsal system: - Can recognize objects - Poor visual control for tasks like grasping - Damage to ventral system - Cannot recognize objects - Can still manipulate them, grasping, etc. - Much of the information in the dorsal system is not consciously accessible 44 Blindsight: vision without sight 45 The brain and vision - Enormous processing power devoted to vision - Visual cortex is largest “system” in the brain - 30% of the cerebral cortex - ⅔ of the electrical activity - Lots of processing happening “subconsciously” 46 Case study: How the brain sees 3d - One eye - Focus - how much your lens must change to make object clear - Blur - objects that are blurry are at different depth - Parallax - observer or object moves, gets multiple views - Two eyes - Stereopsis - images from eyes are different - Convergence - where your eyes are pointing - Brain - Kinetic depth - infer 3d shape of moving objects - Occlusion - objects in front are closer - Familiar objects - you know how big a car is… - Shading - 3d shape from light/shadow cues 47 Case study: How the brain sees 3d 48 Case study: How the brain sees 3d 49 Case study: How the brain sees 3d 50 Case study: How the brain sees 3d 51 52 53 54 55 56 57 We don’t really understand vision - Visual cortex - highly studied part of the brain - Only rough idea of what different components do - New discoveries in vision all the time - Eye uses blinking to reset its rotational orientation - Visual cortex can make some “high-level” decisions 58 Is vision easy or hard for humans? - What do you think now? 59 Is vision easy or hard for humans? - What do you think now? 60 So what are we looking at anyway? - Electromagnetic radiation - Wave? Particle? - Photon - single particle of light - Visible light: ~400-700 nanometers - Why that range? Electric Field Magnetic Field 61 Visible light and the sun 62 Light is a combination of waves - Can be described as a sum of its parts - Relative strength of different frequencies 63 Sources of light are diverse! 64 “White” light - all wavelengths 65 Objects reflect only some light 66 Objects reflect only some light 67 Objects reflect only some light 68 Objects reflect only some light 69 What color is the object? - The “color” of an object depends on both the incident light and the objects reflectance: 70 Different illumination matters! 71 Different illumination matters! Under White Light Under Bluish Light Under Dark Blue Light Under Red Light Under Green Light Under Yellow Light 72 Case study: makeup application 73 Photoreceptors and light - Each receptor has a responsiveness curve - Receptors more responsive to some wavelengths, less responsive to others - Rods: peak around 498 nm - Cones: 3 kinds - Short: peak around 420 nm - Medium: peak around 530 nm - Long: peak around 560 nm 74 Photoreceptors and light 75 Cones and color - Our perception of color comes from cones - Different waveforms provoke different responses - Each cone has essentially one “output” - To calculate: - Multiply input waveform by response curve - Integrate area under the curve - The “color” we see is the relative activation of the 3 kinds of cones 76 All cones are not equal 77 Different wavelengths are brighter 78 This is hard to read This is easier to read 79 Many variations, what do they see? State Types of cone cells Approx. number of Carriers colors perceived Monochromacy 1 100 marine mammals, owl monkey, Australian sea lion, achromat primates Dichromacy 2 10,000 most terrestrial non-primate mammals, color blind primates Trichromacy 3 1 million most primates, especially great apes (such as humans), marsupials, some insects (such as honeybees) Tetrachromacy 4 100 million most reptiles, amphibians, birds and insects, rarely humans Pentachromacy 5 10 billion some insects (specific species of butterflies), some birds (pigeons for instance) 80 Color is our perception of waves - Not the actual wave itself - We only have 3 cones, have to represent color with just 3 outputs - Many waveforms look the same: metamers - Is this a problem?? 81 Metamers are great! - Imagine we could perfectly perceive waveforms: - To duplicate a color you would have to duplicate the wave - TVs would be really hard to make - Color printers would require thousands of inks - But not with the power of metamers! - Can recreate many colors just by selectively stimulating cones 82 CIE 1931 and Color Matching - Late 1920s William Wright and John Guild experimented with colors! (and people) - Subjects get controls to 3 “primary” lights - Show them a light - Subject adjusts their lights to match the given light 83 84 p1 p2 p3 85 p1 p2 p3 86 p1 p2 p3 87 88 p1 p2 p3 89 p1 p2 p3 90 p1 p2 p3 p1 p2 p3 p1 p2 p3 91 92 93 94 95 96 Results: - Given 3 primaries people can match any color - People select very similar distributions for a given color - Colors seem to follow nice, linear rules: Grassman’s laws! - A=B+C => A+D=B+C+D - A=B+C => nA=nB+nC - A=B+C and D=B+C => A=D - Light is combinations of individual wavelengths - If we can match any wavelength we can match any light 97 Now we can make a map of color 98 Linear colorspace - Pick some primaries - Can mix those primaries to match any color inside the triangle 99 “Theoretical” CIE RGB primaries 100 Practical sRGB primaries, MSFT 1996 101 MANY different colorspaces 102 What does this mean for computers? - We represent images as grids of pixels - Each pixel has a color, 3 components: RGB - Not every color can be represented in RGB! - Have to go out in the real world sometimes - RGB is made to trick humans, not be accurate - sRGB is not actually linear, gamma compressed - Humans see differences between dark tones more than bright - Compress light tones, expand dark tones, more efficient - Can represent color with 3 numbers - #ff00ff; (1.0, 0.0, 1.0); 255,0,255; etc…. 103 Grayscale - making color images not - We can simulate monochromatic images from RGB - Want a good approximation of how “bright” the image is without color information - (R+B+G/3) - looks weird - We should - Gamma decompress - Calculatight lightness - Gamma compress - We can just operate on sRGB - Typically ~.30R +.59G +.11B 104 RGB is a cube... 105 Hue, Saturation, Value: cylinder! 106 Hue, Saturation, Value - Different model based on perception of light - Hue: what color - Saturation: how much color - Value: how bright - Allows easy image transforms - Shift the hue - Increase saturation 107 108