History of Artificial Intelligence PDF
Document Details
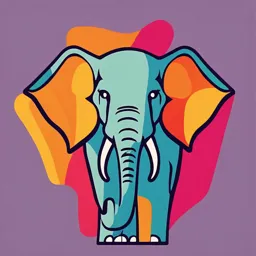
Uploaded by AstoundedComprehension6772
IU International University of Applied Sciences
Tags
Summary
This document provides an overview of the history of artificial intelligence, and examines notable figures and developments in the field, including philosophers like Aristotle and Leonardo da Vinci. It explores the philosophical and historical contexts that shaped the development of artificial intelligence.
Full Transcript
UNIT 1 HISTORY OF ARTIFICIAL INTELLIGENCE STUDY GOALS On completion of this unit, you will have learned … – how artificial intelligence has developed as a scientific discipline. – what paradigms have dominated public perception of the field at different times. – which notable advances a...
UNIT 1 HISTORY OF ARTIFICIAL INTELLIGENCE STUDY GOALS On completion of this unit, you will have learned … – how artificial intelligence has developed as a scientific discipline. – what paradigms have dominated public perception of the field at different times. – which notable advances are still relevant today. – what the history of artificial intelligence means for you in terms of learning new skills and contributing to society. 1. HISTORY OF ARTIFICIAL INTELLIGENCE Introduction This unit starts by providing an overview of artificial intelligence and then proceeds to explain some of the details that make up this new technology. It will take you back to the year 350 BC to illustrate how ideas about the artificial creation of intelligent processes have evolved across the ages. To date, artificial intelligence is an active research area in the field of cognitive psychology. Consequently, no single uncontroversial or uncontested definition of the term currently exists. An operational definition that is apposite with respect to the subject of this course can be stated as follows: intelligence is the ability to accomplish complex goals. In this vein, artificial intelligence (AI) can be framed as the totality of abilities used to accomplish complex goals that are achieved by machines. This broad definition implies perception, learning, problem solving, and decision-making. In practical terms, this means that com- puters that can perceive via sensing devices, apply reasoning, and then act in a rational manner are defined as exhibiting artificial intelligence. 1.1 Historical Developments Ancient Artificial Intelligence History A conventional starting point for the history of artificial intelligence tends to be around the 1950s when the notion of artificial intelligence was first applied to the nascent discipline of computer science. However, it is enlightening to take a broader view of the history of ideas related to artificial intelligence through a brief look at some of the key thinkers in the field dating back to 350 BC and the philosophical insights of Aristotle (Russell & Norvig, 2022). Aristotle (384—322 BC), Greek Philosophical insight: Artistotle formalized logical conclusions by fully enumerating all possible categorical syllogisms. Relation to artificial intelligence: Algorithms can be programmed to derive valid logical conclusions based on a given set of rules. Syllogism Aristotle taught logic at a school called the Lyceum. Syllogisms are rules for arriving at This is a form of logical workable conclusions from two or more propositions. A contemporary equivalent to the argumentation that applies deductive reason- enumeration of valid forms of logical derivation forms the foundation of logical program- ing to reach a conclusion ming languages. based on two or more propositions that are assumed to be true. 14 Leonardo da Vinci (1452—1519), Italian Philosophical insight: Da Vinci designed a hypothetical computing machine on paper. Relation to artificial intelligence: Progress in computing machinery is a necessary pre-con- dition for artificial intelligence. Da Vinci designed a computing machine with 13 registers, demonstrating that a black box can accept inputs and produce outputs based on a stored program in memory or mechan- ics. Thomas Hobbes (1588—1679), British Philosophical Insight: Hobbes derived the notion of the necessity of a social contract from a mechanistic understanding of the individual. Relation to artificial intelligence: Hobbes identified similarities between reasoning and computation in that humans employ operations similar to calculus in rational decision- making, such that they could be formalized, analogous to mathematics. René Descartes (1596—1650), French Philosophical Insight: Rationality and reason can be defined via mechanics and mathe- matics. Similar views on rational thought were held by Gottfried Wilhelm Leibniz (1646— 1716) based on earlier work by Ramon Llull (1232—1315). Relation to artificial intelligence: Objectives can be formulated in the form of equations. Objectives in linear programming or in artificial intelligence agents are defined mathemat- ically. Descartes described rationalism and materialism as two sides of the same coin. Arti- ficial intelligence aims for rational decisions arrived at mathematically. David Hume (1711—1776), Scottish Philosophical Insight: Hume completed foundational work on questions of logical induc- tion and the notion of causation. Relation to artificial intelligence: Hume linked the principles of learning with repeated exposure that, among others, manifests in the learning curve. The principle of inferring Learning curve patterns or relations in data via repeated exposure is key to many machine learning algo- This is a graphical repre- sentation showing how rithms. an increase in learning (measured on the vertical Recent Artificial Intelligence History axis) derives from greater experience (the horizon- tal axis). Recent history in artificial intelligence is commonly said to have started in 1956, the year of the seminal Dartmouth conference. The term artificial intelligence was first coined at this conference and a tentative definition of the concept proposed. We deal with this recent history in terms of key personalities, key organizations that supported the cause, and key concepts that broke previous limits. 15 Dartmouth conference Key personalities The Summer Research Project on Artificial Intelli- gence was held in 1956 at A recent history of artificial intelligence often begins by describing the contributions of Dartmouth College in individuals such as Alan Turing (1912—1954), John McCarthy (1927—2011), Marvin Minsky New Hampshire, USA. (1927—2016), and Noam Chomsky (1928—). The female programmers of the ENIAC (Elec- This six-week conference is considered to be the tronic Numerical Integrator and Computer), the first general-purpose computer, which birthplace of artificial began operation in 1946, should also be mentioned in this context (Schwartz, 2019). These intelligence as a research are notably, Kathleen McNulty, Frances Bilas, Betty Jean Jennings, Ruth Lichterman, Eliza- discipline. beth Snyder, and Marlyn Wescoff. Alan Turing was an English mathematician and computer scientist who became fascinated with the prospect of mechanizing and formalizing rational thought processes. It was Turing who famously came up with the eponymous Turing test in 1950. In this test, a machine is seen to exhibit intelligence if it is able to have a conversation with a human observer that the latter cannot distinguish from a conversation with another human. Automata John McCarthy was an American scientist who studied automata and first coined the term This refers to a self-oper- artificial intelligence. Together with IBM and the Massachusetts Institute of Technology ating machine or machine control mechanism (MIT), he made artificial intelligence a separate field of study. McCarthy is also credited designed to automatically with inventing the Lisp programming language in 1958, which was used in artificial intelli- follow a predetermined gence applications for 30 years in the areas of fraud detection, website design, and robot- sequence of operations or instructions. ics. In the 1960s, he also invented computer timesharing and founded the Stanford Artifi- cial Intelligence Laboratory, which played a pivotal role in research relating to human skills for machines, such as seeing, listening, and reasoning. Marvin Minsky was an early artificial intelligence researcher and cognitive scientist who, together with McCarthy, attended the first artificial intelligence conference at Dartmouth College. In 1959, they jointly founded the MIT Artificial Intelligence Laboratory. However, their collaboration ended after McCarthy moved to Stanford University, with Minsky remaining at MIT. Noam Chomsky is also worth a brief mention, more as a linguist and philosopher than as an artificial intelligence scientist. His contribution to artificial intelligence comes in the form of his criticism of social media and as a contributor to linguistics and cognition. Key institutions Key institutions involved in the development of artificial intelligence include universities such as Dartmouth College, the host of influential artificial intelligence conferences, and MIT, where many influential figures of early artificial intelligence research taught. Corpora- tions such as IBM and INTEL, and government research institutions such as the Defense Advanced Research Projects Agency (DARPA) in the United States, which funds basic dual technology research projects (i.e. projects with civilian as well as military purposes), have also played a critical role in the development of artificial intelligence. 16 Key ideas supporting the development of artificial intelligence Research in the areas of decision theory, game theory, neuroscience, and natural language processing have also contributed to the development of artificial intelligence. Decision theory combines probability (mathematics) and utility (economics) to frame artificial intelligence decisions in terms of economic benefit and uncertainty. Game theory was made famous by John von Neuman (1903—1957), an American-Hun- garian computer scientist, and Oskar Morgenstern (1902—1977), an American-German mathematician and game theorist. Their work led to rational agents learning strategies to solve games. Neuroscience is a body of knowledge about the brain that some artificial intelligence models try to emulate, especially the brain’s problem-solving and information storing abilities. Natural language processing (NLP) is a discipline at the confluence of linguistics and computer science that aims to analyze and process language in both written and spo- ken forms. High-level languages are closer to human language and allow programmers independ- ence from computing hardware’s instruction sets. Other artificial intelligence specific lan- guages are listed below: Lisp is one of the older computer programming languages developed by John McCar- thy. Its name derives from the words “list processing”. Lisp is uniquely able to process strings of characters. Although it dates back to the 1960s, it remains relevant today and was used for early artificial intelligence programming. Prolog is an early artificial intelligence programming language designed for solving logi- cal formulas and proving theorems. Python is a high-level, general purpose programming language that plays a large role in artificial intelligence today. It has been around since 1991. Being open-sourced, Python has a substantial library that coders (programmers) can use to create value quickly. Recent progress in artificial intelligence is linked to three main factors. The first is progress in the availability of massive amounts of data in many formats, called big data, which is Big data continuously increasing. The second is progress in the data processing capacity of com- This refers to datasets that exceed the capabili- puters, while the third relates to new insights gained through mathematics, philosophy, ties of conventional infor- cognitive science, and machine learning. mation processing tools, commonly characterized by the dimensions of vol- Key Trends in Artificial Intelligence ume, velocity, variety, and veracity. Some definitions also add value and valid- Investment in artificial intelligence is becoming exceedingly relevant for corporations, uni- ity. versities, and governments. There are hardly any aspects of society, the economy, and our own personal lives that are not already influenced by artificial intelligence technology. In particular, technology leaders, such as Apple, Amazon, Microsoft, Google, and important Chinese companies such as Baidu and Tencent are highly committed to research and development in artificial intelligence. Current developments can be classified into three major areas: 17 Assistive intelligence devices These include wearable health monitors that measure pulse, temperature, or blood sugar, and sensors used in automobiles that detect all kinds of mechanical and human-related conditions. These are then interpreted via artificial intelligence algorithms in order to pro- vide assistance services. Augmented intelligence In this field of research, artificial intelligence works alongside a human expert to provide task-related support in selected areas where machine performance is superior to human performance. The overall process is, however, driven by the human expert. An example is the assistance provided to physicians in prescribing medications to overcome the limita- tions of human medical knowledge by being able to consider all possible medical side effects in a few minutes. Another example is a warning signal given to a driver whose car is in the wrong lane. Autonomous intelligence This involves physical robotic actors, such as self-driving vehicles, moving warehouse robots, or the autonomous control of non-physical workflows and business processes. The overarching theme is that control is exerted, with decisions being made without human intervention. The prospect of artificial intelligence operating in the real world without human control is not without danger, posing important ethical questions. The reaction of autonomous vehicles when faced with life-and-death decisions is an obvious example. However, also seemingly more benign application domains such as the autonomous acceptance or denial of insurance claims is not without its perils considering the impact they may have on the economic prospects of the affected persons. The Future of Artificial Intelligence Assessing the future prospects or impact of a technology or field of research is always highly speculative and influenced by cognitive biases and previous experiences that may have lost their relevance for assessing the state of the field and its future prospects. Thus, no attempt is given here at predicting the long-term future of artificial intelligence. How- ever, it is prudent to examine a common pattern that emerges in the general uptake and development of innovations and how they relate to current topics in artificial intelligence and supporting technologies. Gartner (2018) captures the history of many innovations in the form of a hype curve. Hype curves are described in phases, as shown in the graph below. The x-axis represents time characterized by the following phases: a discovery phase, or a need in the marketplace, which triggers innovation a peak phase of inflated expectation, which is shorter than the other phases a period of disillusionment a period of enlightenment, which recognizes the value of an innovation a period of leveling off in which productivity takes hold and becomes the norm 18 Figure 1: Gartner Hype Cycle for Emerging Technologies, 2018 Source: Gartner, Svetlana Sicular and Kenneth Brant, 24 July 2018. 19 The technology hype curve bears some similarity to the bell-shaped curve of a normal dis- tribution with the exception that the right tail leads into a rising slope that eventually lev- els off. Several aspects of artificial intelligence are positioned along this curve. For exam- ple, on closer inspection we can observe the following trends: In the innovation trigger phase, knowledge graphs, neuromorphic hardware, AI PaaS, Artificial General Intelligence (the ability of a machine to perform humanlike intellectual tasks), Edge AI, and smart robots appear. At the peak phase of inflated expectations, or hype, we find deep neural networks that were the driver of new performance highs in many machine learning applications dur- ing the last five to seven years. In the disillusionment phase, that is going downhill, we find that Level 4 Autonomous Driving may experience defunding due to its failing to meet expectations. Nothing in the field of artificial intelligence has reached the plateau of productivity yet, which represents general acceptance and productive use of the technology. Governance of Artificial Intelligence and Regulatory Considerations Let us assume that artificial intelligence is in fact a broad technology revolution not defined by a single capability like self-driving cars. Rather, it is an amalgam of many parts, each moving through the same process at a different rate from different starting points. It can be expected that there will be winners and losers as artificial intelligence advances. Orderly growth requires ethical guidelines governing human behavior in commerce and national defense. The lack of rules governing labor in British factories during the Industrial Revolution, for example, led to unrest, suffering, and ultimately the utopian ideology of Communism. In the spirit of learning from our common history, rather than blindly repeat- ing it, it is now time to consider ethical guidelines for the use of artificial intelligence appli- cations. A practical concern for society is to understand the consequences of the potential misuse of artificial intelligence, which may be addressed through either government regulation or self-imposed rules. The European Union’s General Data Protection Regulation (GDPR 2016/679) is one of the first government-initiated steps to regulate data, which initially focused on ensuring the protection of privacy for individual consumers. Another initiative of the European Union is the EU Regulation on Artificial Intelligence, for which a first pro- posal was presented in April 2021 (Publications Office of the European Union, 2021). The aim of this regulation is to create a common legal framework for the development and use of AI-based systems, which at the same time strengthens the public's trust in these sys- tems by adequately taking into account fundamental rights, security and privacy. Self-regulation with an emphasis on ethics is currently being researched at MIT and Stan- ford University in the United States. Stanford University also recently established the Insti- tute for Human-Centered Artificial Intelligence (HAI) (pronounced “hi”), which is led among others by renowned AI researcher Fei-Fei Li. It serves as an interdisciplinary center combining computer scientists, neuroscientists, and legal scholars focusing on social responsibility. MIT’s College of Computing has similar objectives in promoting the posi- tives of artificial intelligence while preventing negative consequences. 20 Ethical issues are relevant to all commercial and military applications of artificial intelli- gence as well as to the working lives of employees subject to an economy highly influ- enced by artificial intelligence. Relevant considerations include the following: Ethics In the business arena, ethics can be defined in terms of distinguishing between right and wrong conduct and acting accordingly. The choices between the two are many and quan- tified in terms of degree; they are in constant conflict and involve personal and corporate collective consciences. Examples of the unethical use of artificial intelligence include those that support discriminatory behavior towards particular groups of people, take advantage of the disadvantaged, show unchecked zeal for winner-takes-all competition, or that lead to the disbanding of beneficial artificial intelligence applications due to insuf- ficient financial reward. Rights Rights pertain to the physical human body, the right to work, the right to hold property, and to happiness and fellowship. Defining documents for our understanding of rights are the national constitutions or basic laws of states, the United Nations Universal Declaration of Human Rights, and the Geneva Convention, among others. Artificial intelligence projects must observe these rights. In cases where they do not, projects must be changed so that they adhere with them. Governments Governments naturally play an important role in regulating technological advances due to their overarching regulatory and legislative functions. By definition, governments are tasked with the responsibility of preserving a body politic. Artificial intelligence is a sci- ence and, therefore, a common good. This implies that it does not exist without govern- ment control, and it will be regulated sooner or later, as has already occurred in the Euro- pean Union. However, the time lag between industrial innovation and its effective regulation is usually long, and therein lies the danger. Unintended outcomes Unintended outcomes resulting from artificial intelligence technologies pose a significant risk to society, which often result from a lack of both oversight and foresight. The absence of oversight implies a lack of human control once an algorithm has been deployed. Economics Economic considerations are also important. Relevant questions in this domain include the impact of artificial intelligence on the labor market and the unequal distribution of wealth. 21 Artificial Intelligence Viewed as a Revolution When we think of revolutions, violent political overthrows in favor of a new paradigm come to mind. Examples of this are the French Revolution (1789), which involved the top- pling of a monarchy in favor of liberty, equality, and fraternity, the Russian Revolution (1917), which replaced the Czar with Communism, and the American Revolution (1775— 1783), which ended colonialism in favor of a republic. Similarly, humanity has lived through technological and, consequently, economic revolu- tions in the past. Examples include the introduction of the power loom and the spinning jenny (circa 1770), which enabled the global cotton market, steam power, the telegraph and the telephone, the internal combustion engine, sewing machines, and production lines. The artificial intelligence revolution promises to be faster and deeper. Technological revolutions share the overthrow aspect of the term revolution, but they are not necessarily caused by discontent within the masses. Technological revolutions are the result of creative people tinkering and subsequently making discoveries that serve human interests and needs. Gutenberg’s (1440) discovery of the moveable printing process, which led to the broadscale distribution of information, literature, science, scholarship, and the growth of publishing as a business, is a prime example of an innovation that also repre- sented a technological revolution. Other technological revolutions include that brought on by Fritz Haber’s discovery of a workable process for the synthesis of ammonia in 1910 and Ernest Rutherford’s splitting of the atom in 1917. Haber’s discovery led to increases in agricultural yields, with ammonia used as a chemical fertilizer, supporting larger populations and economic growth. By con- trast, Rutherford introduced a new branch of physics which contributed to the develop- ment of the atomic bomb, ending World War II in the Pacific. Spread spectrum (or fre- quency-hopping) which was developed by Hedy Lamarr is also a technology that was originally invented to be used in World War II, i.e. to control torpedoes. Even today, it is still the basis for various technologies in the telecommunications sector, such as Blue- tooth. We are at the beginning of what is publicly described as an artificial intelligence technol- ogy revolution. We do not yet know who the innovators will be. However, we can be cer- tain that collaboration is occurring across the sciences, leading to a brave new world to which we will need to adapt. What the Industrial Revolution and the current revolution in artificial intelligence have in common is that both periods produced inventions that improved people’s lives. The Industrial Revolution used steam and electrical energy to power machinery for mass pro- duction, overcoming the limits of human strength. The artificial intelligence revolution is using computers to produce information-based tools to overcome the information pro- cessing limitations of the human brain. Landmark achievements of the Industrial Revolution include the transition from the steam engine to electrically powered trains and ships, the enhancement of agricultural output as a result of the introduction of mechanized farm machinery and chemical fertilizers, the 22 development of the telephone, and the creation pharmaceuticals, such as penicillin. As a result of the artificial intelligence revolution, we can expect the potential eradication of many common illnesses, more efficient modes of transportation, the development of advanced methods for fighting crime, while simultaneously enabling it with the same technology, and all manner of other discoveries, such as developments in military capabil- ities used for national defense and territorial dominance. It is worthwhile to observe that nearly all newly discovered technologies are based on someone else’s prior discovery, driven by both human needs and innate curiosity. Artificial intelligence is no exception. In ancient times, Finnish people improved the axe, a tool, in terms of its design and the materials used to build it in order to make harvesting trees more efficient. The axe led to better housing and fishing boats, improving people’s diet, ultimately leading to longer life expectancy. The axe is a tool. Artificial intelligence is also a tool that can help the current generation live better and longer. However, just as axes can be used to cause a great deal of damage, so too can artificial intelligence. We can therefore expect that the revolution in artificial intelligence will have both positive and negative consequences for society. 1.2 AI Winters The term “AI winter” was coined around 1984 by artificial intelligence researchers. It is a AI winter bit melodramatic, but it befits the culture of artificial intelligence which is known for its This is a period character- ized by a prolonged exuberance. The word “winter”is borrowed from the expression “nuclear winter”, an after- decrease in interest and effect of a hypothetical nuclear world war, in which ash would overwhelm the atmosphere funding in artificial intelli- and block sunshine from entering the Earth’s atmosphere for years, so nothing would gence research. grow, and temperatures would stay excessively cold. Applied to artificial intelligence, the term refers to a pronounced and prolonged reduction in interest and funding of artificial intelligence technology and research. Such a downturn occurs when it becomes clear that inflated expectations generated in times of exceeding optimism regarding novel techno- logical developments will not realistically be met. There have been two such AI winters, one between 1974—1980 and one between 1987—1993, give or take a few years in either direction. The first AI winter was caused by the non-delivery of promised outcomes in automated language translation and shortcomings in connectionist (network-based) artificial intelli- gence approaches. While the former Soviet Union and the United States were in a cold war, the need for automatic language translation in the West was also great, with not enough translators available to meet demand. Despite initially optimistic assessments to the contrary, early attempts at machine translation failed spectacularly—not in the least due to their inability to handle word ambiguities. Phrases such as “out of sight—out of mind” were translated to “blind idiot”, for example. The United States’ generous govern- ment-funded research was evaluated by the Automatic Language Processing Advisory Committee. It stated that machine translations were less accurate, more expensive, and slower than employing humans. Moreover, it had been shown that the Perceptron—a 23 Perceptron then popular early model of neural-inspired artificial intelligence—had severe shortcom- This is a very simple clas- ings that prevented it from representing even the simple logical function of Exclusive or sification algorithm. In its simplest form, a percep- (XOR). tron is a neural network with a single node. The second AI winter occurred when the artificial intelligence community was overcome by a feeling of general pessimism due to the perception that the industry was out of con- trol, had disappointing results, and would collapse. This perception was based on the demise of the Lisp machine business. Lisp machines were a type of computer that suppor- ted the Lisp language—a popular language for artificial intelligence projects. It became clear, however, that the earliest successful examples of expert systems, which were the main driver of the renewed interest in artificial intelligence during the years 1980—1987, could not be developed beyond a certain point. The reasons for the developmental ceiling were unmanageable growth in the associated fact databases as well as their unreliability with respect to unknown inputs. The counter argument to the notion of AI winters is that these periods of downturn were in fact a myth perpetrated by a few prominent academics, their organizations, and investors who had lost money. Between 1980 and 1990 artificial intelligence was deeply embedded in all kinds of routine processing operations, including credit cards. The fact that Lisp machines collapsed, and expert systems went out of fashion, does not suggest the demise of an entire industry. The Causes of AI Winters AI winters are defined as periods during which funding, research, and interest in the sub- ject significantly decline. The following conditions can contribute to an AI winter: Non-performance: All research is ultimately funded by an interested party, be that a government, a foundation, or by wealthy persons either as an investment or as an act of benevolence. When funded research does not produce promised results, funding even- tually ends. Hype, or the excessive exuberance of promising more than can be delivered, by defini- tion leads to non-performance. Cheaper alternatives than what is being pursued suddenly emerge. This invariably cau- ses sponsors to switch to a new approach, as was the case with Lisp machines. In 1985, the new head of the United States’ Defense Advanced Research Projects Agency (DARPA) only agreed to fund mission-oriented research. Prior to that, DARPA funded basic research projects that by definition were unrelated to any particular mission. Fed- eral Legislation (the Mansfield Amendment of 1969) mandated that DARPA only fund mission-oriented research, and it belatedly complied. Computing capacity: Successful artificial intelligence outcomes often depend on the processing of huge amounts of data, which require a large amount of memory capacity and high processing speed. Availability of data and computing capacity have to be in sync. They have not always been available at the same time. This can lead to the perception of project failure. 24 Actual project failures: When funded research fails, disappointments and cancelled con- tracts are often the result. This was the case with the DARPA funded Speech Under- standing Research (SUR) project in which Carnegie Mellon University failed. However, there is a good argument to be made that basic research will more likely fail than suc- ceed. As a technology, artificial intelligence cannot make big steps until two other technologies are in place: computing capacity in terms of data storage and processing speed and the availability of a large enough volume of data. AI Winter Remedies Research “winters” are not inherently bad, as reform is part of moving forward. This is true for research into artificial intelligence as well as other fields of study. Progress does not look like a hockey stick—that is, flat for a while and then straight up. Remedies for AI win- ters obviously lie in the reduction of factors that bring them about. This is hard to do in a free economy, as no one person or entity controls all these factors. However, one counter- weight to AI winters is competition between nation-states. Competition between nation-states, such as the United States and China today, the United States and the former Soviet Union prior to 1991, and Japan’s Fifth Generation Projects in 1981, has kept opposing nation-states engaged in artificial intelligence research. Engage- ment translates into funding, as artificial intelligence is a dual technology. As early as 1981, Japan’s Ministry of International Trade and Industry (MITI) funded a Fifth Generation Project for software and hardware to perform conversations, translate languages, inter- pret pictures, and perform humanlike reasoning. While obviously not meeting its promise, it got the attention of many governments. It is important to understand that most artificial intelligence technology is dual purpose—that is, artificial intelligence technologies have military value as well as a consumer value. For example, robots and drone aircraft can be used in military battlefields as well as to pick products in warehouses and deliver medical supplies to remote areas. All governments share legitimate interests in defense and the management of their economy in terms of employment and competition. The Next AI Winter The answer to the question of whether there will there be another AI winter is that nobody knows for sure. If we stick with the definition of an AI winter as a period of little or no research funding for artificial intelligence, it is possible that if a hyped concept such as singularity were to get funded, and then defunded as a result of non-performance, an AI Singularity winter could indeed result. However, one argument against AI winters is that many artifi- This refers to the moment when exponential growth cial intelligence technologies wind up being embedded within other fields, such as bioin- in machine intelligence formatics, assuming a different name as a result. The defunding of non-performing accelerates, resulting in projects can, therefore, actually be good because change and renewal are key compo- next-generation artificial intelligence that can use nents of a revolution. The reader is therefore free to decide whether the concept of AI win- its superior resources and ters matters or whether it is merely an interesting myth. capacities for self- improvement to create a subsequent version of ever-improved intelli- gence. 25 1.3 Notable Advances in Artificial Intelligence Having investigated the pronounced downturns in the history of artificial intelligence in the previous section, the focus now shifts to the prosperous times in between previous AI winters, their characteristic research topics, and their successes. Additionally, we will also examine important developments in adjacent fields of study and their relationship to pro- gress in artificial intelligence. 1956—1974: Nascent Artificial Intelligence The early years of artificial intelligence research were dominated by what is now called symbolic artificial intelligence. This approach tries to formalize thought processes as the manipulation of symbolic representations of information according to the rules of formal logic. Consequently, artificial intelligence systems of this era were predominantly con- cerned with the implementation of logical calculus. In this vein, they often implemented a variant of a search strategy in which solutions were arrived at by following a step-by-step procedure in which the steps either followed logically from the preceding state or system- atically tried to explore possible alternatives using backtracking to avoid dead ends. Dur- ing these years, the first attempts at processing natural language were also developed. Since both logical search methods and the first steps towards language processing focused on highly constrained settings and environments, initial successes could be ach- ieved. This so-called microworld approach to creating simplified working environments for the first artificial intelligence solutions also proved to be fruitful in computer vision and robot control. Parallel to these developments, the first theoretical models of neurons—the cells that make up mammalian brains—were developed as corresponding models of how these cells or computational units could interact in networks to implement simple logical functions. 1980—1987: Knowledge Representation While the first wave of artificial intelligence research primarily focused on logical infer- ence, the second wave was predominately characterized by trying to address the problem of knowledge representation. This shift in focus occurred due to the insight that intelligent behavior in day-to-day situations was largely reliant on common sense knowledge about how the world works rather than merely on logical inference. To capture this knowledge- based aspect of intelligence, the technological approach of expert systems was devised. The main feature of this technology was the attempt to systematically store domain rele- vant knowledge in knowledge databases and devise methods to effectively and efficiently navigate them. Moreover, a noticeable upturn in government funding occurred at the beginning of the 1980s, with the Fifth Generation Computer project of the Japanese Gov- ernment and the Alvey project in the United Kingdom being notable examples. Initial set- backs in the development of network and neurally-inspired approaches to artificial intelli- gence were also remedied by newly proposed network models and backpropagation as an effective training method for layered networks of computational units. 26 1993—Today: Learning from Data The 1990s saw some big advances in game artificial intelligence when the first computer system (IBM’s Deep Blue) beat the then world-champion Garry Kasparov. Apart from this substantial but narrow success, artificial intelligence methods have taken hold in engi- neering real-world solutions, and successful approaches in its subfields have gradually entered real-world applications, often without being explicitly referred to as artificial intel- ligence. Moreover, since the early 1990s, there has been an influx of ideas from mathemat- ics, statistics, decision theory, and operations research, which have contributed to artifi- cial intelligence becoming a mature and rigorous scientific discipline. In particular, the intelligent agent paradigm has gained substantial popularity. Here, the notion of the rational agent of economic theory combines with computer science notions of objects and modularity to form the idea of an intelligently acting entity. From this point of view, artifi- cial intelligence can be seen as the study of intelligent agents, thereby freeing it from the notion of imitating human intelligence to a broader study of intelligence in general. These aforementioned advances have been supported by a marked increase in computa- tional and data storage capabilities. This, together with the unprecedented increase in the volume, variety, and velocity of data generation, which occurred during the rise of the internet, has set artificial intelligence up for its current boom. The latest upturn in the pop- ularity of and interest in artificial intelligence research, which began in 2012, is predomi- nantly based on advances in connectionists machine learning models, known as deep learning. In deep learning, theoretical advances in the construction and adaptation of net- worked machine learning models are linked synergistically with the previously mentioned increase in information storage and processing capabilities and the presence of a larger corpora of data to train such models to achieve hitherto unseen levels of performance in many machine learning benchmark problems. In turn, these developments have revived interest in long-established learning models, such as reinforcement learning, paving the way for altogether new ideas, such as adversarial learning. Linguistics Linguistics can be described as the science of natural language in general. It encompasses the study of the structural (grammatical) and phonetic properties of interhuman commu- nication. Understanding language requires information about the subject matter and the context in which it is used. To this end, linguistics distinguishes between the following subfields (McGregor, 2015): Phonetics and phonology: studies speech sounds Morphology: deals with the structure of words Syntax: deals with the structure of sentences Semantics: studies the meaning of words and sentences Pragmatics: studies the use of language Noam Chomsky (1957) was instrumental in establishing this with his book Syntactic Struc- tures. One could go further and consider creativity and thought as related to linguistic arti- ficial intelligence given that all our ways of thinking are so intimately related to language as a form of representation. How, for example, can a child say something it has never said 27 before? In the field of artificial intelligence, we think of natural language as a medium of communication in which the context is a given. Language is, therefore, much more than representation. Cognition In the context of artificial intelligence, cognition refers to several different faculties, includ- ing sensing and perception, learning and understanding, reasoning and intelligence, and thinking and apprehension. The English word “recognition” reflects this. Much of our con- temporary understanding of cognition in artificial intelligence ultimately comes from a combination of several bodies of knowledge. In this case, the fields of psychology and arti- ficial intelligence combine. Psychology deals with experimentation on humans and ani- mals to form new theories and hypotheses; computer science deals with the behavioral modeling of what psychology has observed. Computer models of the brain receive a stim- ulus and make an internal representation of that new stimulus, which can in turn lead the brain to modify its internal representation. If it does, it can lead to actions being taken by the brain. If we want to model the brain, we have to get into it, virtually of course. This can be done by observation or introspection. Once we have a cognitive computer model that works well, the next step is to determine how the model makes decisions, understanding its rationale. It is important for the artificial intelligence industry to provide “explained decisions”, which means that the artificial intelligence model should be able to make its reasoning transparent to an outside observer. As artificial intelligence-based decisions are involved in exceedingly large areas of our lives, the ability to explain how decisions are made is demanded by people concerned with the ethical use of artificial intelligence. Nev- ertheless, this aspect is still lacking, particularly in deep learning-based approaches, which are currently quite popular. Gaming Gaming does not only mean gambling or playing games like tennis. In artificial intelli- gence, it is about learning, uncertainty, and probability. John Von Neuman (1903—1957) and Oskar Morgenstern (1902—1977) established game theory as a formal mathematical field of study. Subsequently, a comprehensive taxonomy of games was established, and in some cases even provably optimal rational gaming strategies were developed. Most games are played for entertainment or the challenge of winning, such as in chess, poker, checkers, and bridge. For all of these games, machines now play at a higher level than even the world’s best human experts. Decision theory and game theory are somewhat related. Decision theory addresses uncer- tainty and usefulness, or stated in another way, probability and utility. Game theory is characterized by the fact that player X’s moves affect player Y’s options. However, winning is not necessarily the objective in each case. Children who play games are not only amus- ing themselves and filling in time; they are also experimenting with options and thereby learning and finding out what works, how things work, with understanding developing from their observations. 28 An example of a zero-sum, perfect information, and yet highly complex game, is the ancient Asian board game known in the West under its Japanese name Go (WeiQi in Chi- nese, Baduk in Korean). Go is a 2,500-year-old two-player game in which the main objec- tive is to surround the most territory. Despite its simplicity in terms of the rule set, its finesse and complexity stem from the large game board that enables a combinatorial explosion of possible moves. Before 2016, it was believed that this combinatorial com- plexity (which prevents the successful application of methods used for games like check- ers and chess) would make it a domain characterized by the dominance of human players for the foreseeable future. However, in 2016 one of the world’s best Go players, the Korean Lee Sedol, played a 5-game match against AlphaGo, a Go-playing system developed by the Google-owned company DeepMind. This match ended in a resounding 4—1 win for AlphaGo—a system based on deep networks and reinforcement learning. Whereas the version used in the 2016 match used Go knowledge acquired by humans dur- ing the long history of the game by employing records from professional Go players for training, DeepMind has subsequently developed a system (named AlphaZero) that learns the game solely based on self-play, foregoing traditional Go knowledge altogether. Remarkably, the resulting system has come out even stronger and has subsequently played numerous games where it has surprised human experts with effective and efficient moves that were hitherto shunned by orthodox Go theory. Such an approach is particu- larly useful in policy research and the discovery of strategy options humans have not yet come up with. The Internet of Things (IoT) The Internet of Things (IoT) refers to interconnected computing devices that are embed- ded in homes, cars, on wearable devices, and phones. Such devices are typically used every day. In addition, such devices are enabled to send and receive data amongst them- selves and their users. This generates large amounts of data. The possibilities offered by AI are ideal for evaluating this data and providing further services based on it. It is important to remember that this data can be used for both productive and destructive purposes. A constructive application may take the form of medical reminders to take medication based on the physical measurements generated by wearable devices. A negative applica- tion could be the monitoring of data about the medications a person is taking in order to determine their health insurance rate. In other words, privacy violations and the ethics of data use are issues facing the use of artificial intelligence devices. While IoT does not qual- ify as artificial intelligence per se, it is linked to it. This is because IoT is about connecting machines and generating data, and the intelligent behavior of these machines is derived from artificial intelligence algorithms. Quantum Computing Quantum computing, like the Internet of Things, is a technological advancement that, when combined with artificial intelligence, enables innovation. The term “quantum com- puting” comes from the physical theory called quantum mechanics which deals with the strange behavior of sub-atomic particles. An electron, for example, can be in two differ- ence states (places) at the same time. In general, quantum mechanics posits that physical systems are characterized by a wave function describing the probabilities of the system in 29 any particular state. The goal of quantum computing is to build super computers that uti- lize these quantum properties of matter to implement novel algorithmic approaches that significantly outperform classical machines (see Giles, 2019). It can be reasoned that the probabilistic approach inherent in many artificial intelligence techniques and the ubiqui- tous use of optimization methods are well suited to the kind of information processing that quantum computers provide, putting it in a very promising position to profit from new technological advances in the field. SUMMARY This unit provided an initial overview of the field of artificial intelligence, examining notable discoveries that were instrumental in the develop- ment of the field, such as logical calculus, branch and search algorithms, knowledge representation in expert systems, and networked models of machine learning. It also took a look at failures and periods of stagna- tion along the way. The goal has been to demonstrate that during the historical develop- ment of artificial intelligence, different paradigms have at different times shaped public understanding of the field. Consequently, there is no hard and fast reason to believe that the currently popular paradigm of deep learning networks offers the final word on how to implement intelligent behavior into machines. Additionally, some adjacent fields to artificial intelligence, such as lin- guistics, gaming, the Internet of Things (IoT), and quantum computing have been touched upon to give a flavor of the broad array of fields that have either contributed to the development of artificial intelligence or have been influenced by it. 30